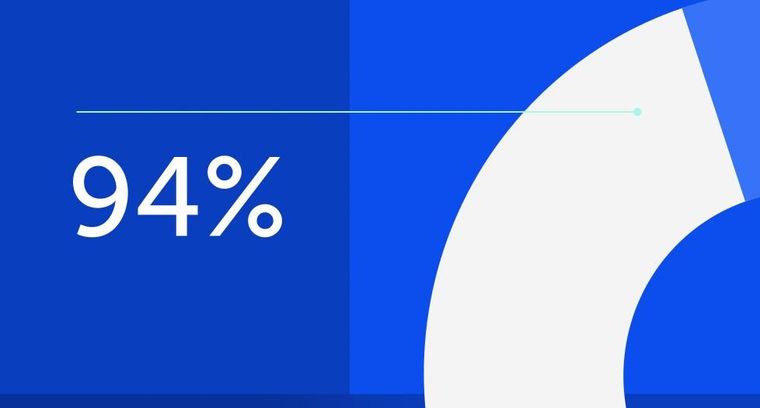
94% of researchers rate our articles as excellent or good
Learn more about the work of our research integrity team to safeguard the quality of each article we publish.
Find out more
ORIGINAL RESEARCH article
Front. Genet., 13 July 2023
Sec. RNA
Volume 14 - 2023 | https://doi.org/10.3389/fgene.2023.1214968
Introduction: The discovery of non-coding RNA (ncRNA) dates back to the pre-genomics era, but the progress in this field is still dynamic and leverages current post-genomics solutions. WWOX is a global gene expression modulator that is scarcely investigated for its role in regulating cancer-related ncRNAs. In bladder cancer (BLCA), the link between WWOX and ncRNA remains unexplored. The description of AP-2α and AP-2γ transcription factors, known as WWOX-interacting proteins, is more commonplace regarding ncRNA but still merits investigation. Therefore, this in vitro and in silico study aimed to construct an ncRNA-containing network with WWOX/AP-2 and to investigate the most relevant observation in the context of BLCA cell lines and patients.
Methods: RT-112, HT-1376, and CAL-29 cell lines were subjected to two stable lentiviral transductions. High-throughput sequencing of cellular variants (deposited in the Gene Expression Omnibus database under the GSE193659 record) enabled the investigation of WWOX/AP-2-dependent differences using various bioinformatics tools (e.g., limma-voom, FactoMineR, multiple Support Vector Machine Recursive Feature Elimination (mSVM-RFE), miRDB, Arena-Idb, ncFANs, RNAhybrid, TargetScan, Protein Annotation Through Evolutionary Relationships (PANTHER), Gene Transcription Regulation Database (GTRD), or Evaluate Cutpoints) and repositories such as The Cancer Genome Atlas (TCGA) and Cancer Cell Line Encyclopedia. The most relevant observations from cap analysis gene expression sequencing (CAGE-seq) were confirmed using real-time PCR, whereas TCGA data were validated using the GSE31684 cohort.
Results: The first stage of the whole study justified focusing solely on WWOX rather than on WWOX combined with AP-2α/γ. The most relevant observation of the developed ncRNA-containing network was LINC01137, i.e., long non-coding RNAs (lncRNAs) that unraveled the core network containing UPF1, ZC3H12A, LINC01137, WWOX, and miR-186-5p, the last three being a novel lncRNA/miRNA/mRNA axis. Patients’ data confirmed the LINC01137/miR-186-5p/WWOX relationship and provided a set of dependent genes (i.e., KRT18, HES1, VCP, FTH1, IFITM3, RAB34, and CLU). Together with the core network, the gene set was subjected to survival analysis for both TCGA-BLCA and GSE31684 patients, which indicated that the increased expression of WWOX or LINC01137 is favorable, similar to their combination with each other (WWOX↑ and LINC01137↑) or with MIR186 (WWOX↑/LINC01137↑ but MIR186↓).
Conclusion: WWOX is implicated in the positive feedback loop with LINC01137 that sponges WWOX-targeting miR-186-5p. This novel WWOX-containing lncRNA/miRNA/mRNA axis should be further investigated to depict its relationships in a broader context, which could contribute to BLCA research and treatment.
Even though the discovery of non-coding RNAs (ncRNAs) dates back to the pre-genomics era, the progress in this field is still dynamic. The current knowledge is three decades away from identifying the first microRNA (miRNA) or long non-coding RNA (lncRNA); the ncRNA-related field has thus achieved a relevant maturity and can leverage current post-genomics solutions. Nowadays, ncRNAs are classified as housekeeping or regulatory RNAs. Housekeepers include, e.g., ribosomal RNA (rRNA), small nuclear RNA (snRNA), and small nucleolar RNA (snoRNA), whereas regulatory ncRNAs encompass, e.g., miRNA, lncRNA, piwi-interacting RNA (piRNA), and circular RNA (circRNA) (Zhang P. et al., 2019). The implication of ncRNAs in human pathologies has been demonstrated in cardiovascular and neurological disorders or cancer (Lekka and Hall, 2018). For the latter, both the diagnosis and therapy benefit from using ncRNAs, such as miRNA, piRNA, and lncRNA (Le et al., 2021).
In recent years, our research focused on investigating the interplay between WWOX, a global modulator of gene expression, and AP-2α/AP-2γ transcription factors. Even though some experimental data confirm the role of these three proteins in regulating RNA interactomes, no literature review provided a synopsis of the current knowledge of WWOX. In contrast, most of the AP-2α/γ-related literature was summarized by us a few years ago (Kolat et al., 2019). Although a dozen experimental articles make up the reasonable collection, more research will presumably appear in the nearest future, especially since the involvement of ncRNAs in cancer is still a hot topic (Liu et al., 2021; Garbo et al., 2022; Han et al., 2022; Jiang et al., 2022). In one of our previous studies, some interesting observations were noted for WWOX and AP-2α/γ based on the cap analysis gene expression sequencing (CAGE-seq) of the bladder cancer (BLCA) cell lines. However, in that project, we mainly focused on signaling pathways regulated by protein-encoding genes (Kolat et al., 2022). Given that the implication of WWOX/AP-2 in ncRNA networks merits further investigation, this study aimed at constructing RNA interactomes containing WWOX and AP-2α/γ and then investigating the most relevant observation using data from bladder cancer cell lines and patients affected by this disease. The first stage of the whole study justified that the investigation of ncRNA interactomes should be focused solely on WWOX rather than on WWOX combined with AP-2α/γ. Later stages of this research indicated that WWOX is a significant factor in prolonging patient survival both individually and in combination with other genes. Interestingly, there was no research on WWOX and ncRNAs in bladder cancer (Table 1), placing our study at the beginning of such literature.
The culture of bladder cancer cell lines, RT-112, HT-1376, and CAL-29 (purchased from Deutsche Sammlung von Mikroorganismen und Zellkulturen [DSMZ], Brunswick, Germany), has been fully described in previous studies (Kolat et al., 2021a; Kolat et al., 2021b). Briefly, the RT-112 cell line was cultured in RPMI-1640 medium, HT-1376 in high-glucose DMEM, and CAL-29 in DMEM; all cell lines were supplemented with FBS, L-glutamine, and antibiotic—antimycotic and incubated under humidified conditions. Stable transduction was obtained using lentiviral overexpression systems for WWOX and AP-2α/γ (Kolat et al., 2021a; Kolat et al., 2021b). The antibiotic clone selection of the first transduction (WWOX) was conducted using puromycin, whereas G418 was used for selection during the second transduction (AP-2α or AP-2γ). For each cell line, six stable transductants were obtained: control/control (KK); control/AP-2α↑ (KA); control/AP-2γ↑ (KC); WWOX↑/control (WK); WWOX↑/AP-2α↑ (WA); and WWOX↑/AP-2γ↑ (WC). To confirm the efficiency of transductions, proteins were isolated using the RIPA lysis buffer with the addition of sodium orthovanadate, phosphatase inhibitor cocktail, and phenylmethylsulfonyl fluoride (Santa Cruz Biotechnology, Dallas, TX, United States). Subsequently, blotting for WWOX, AP-2α, and AP-2γ was performed in duplicate for each cellular variant, as described previously (Kolat et al., 2021a; Kolat et al., 2021b). In the current study, five comparison types (i.e., KA/KK, KC/KK, WK/KK, WA/KK, and WC/KK) were initially adopted from the previous research (Kolat et al., 2022), but the comparison scheme was updated once the workflow focused on WWOX, given the promising results for this protein compared to AP-2α and AP-2γ. From the second stage of this study, cellular variants with WWOX overexpression (represented by WK, WA, and WC) were collectively compared to those without WWOX overexpression (represented by KK, KA, and KC; denoted as the “native WWOX” group). The bladder cancer cell lines were treated as biological replicates of a specific cellular variant to limit the impact of tumor heterogeneity, similar to our previous study (Kolat et al., 2022).
The Extracol reagent (EURx, Poland) was used to isolate total RNA, and extraction was performed according to the manufacturer’s protocol. The RNA integrity number (RIN) was determined using the Agilent 2100 Bioanalyzer (Agilent Technologies, Santa Clara, CA, United States); the quality threshold was set at RIN >7.0. The total RNA was then reverse-transcribed using random primers (CAGE Library Preparation Kit; K.K. DNAFORM, Yokohama, Japan). The 5′-cap-located ribose diols in RNAs were oxidized and biotinylated. Following cap-trapping, streptavidin beads enabled RNA/cDNA hybrid selection. RNA digestion using RNase I/H was followed by linker ligation to 5′-and 3′-cDNA ends, enabling the construction of double-stranded cDNA libraries. Sequencing was performed using the NextSeq 500 sequencer (Illumina, San Diego, CA, United States) using 75 nt single-end reads. Deposited data from the nAnTi-CAGE experiment are held under the accession number GSE193659 in the Gene Expression Omnibus (GEO) database. The quality of the obtained data was evaluated via the FastQC tool (v0.11.9). The Burrows–Wheeler Alignment tool (v0.7.17) was used for mapping the tags to the hg38 human genome. Unmapped reads were processed by implementing hierarchical indexing for spliced alignment of transcripts (v2.0.5). Following modified Paraclu (from the RECLU pipeline) for tag clustering, BEDTools (v2.12) was used to extract regions with a 90% overlap between replicates. Clusters that did not meet the requirements of the irreproducible discovery rate of ≥0.1 and >200 bp were omitted.
All currently known ncRNAs were acquired from the Human Genome Organization Gene Nomenclature Committee (HGNC). This list was applied to our GEO record (GSE193659) to analyze only ncRNA data in the subsequent step. Differential expression analysis (DEA) was performed via the limma package (v3.42.2), with the voom () function to make CAGE-seq data useful for limma. The calcNormFactors () function was used to preprocess data, and low-expressed genes were filtered out (tags with ≥5 counts per million in ≥1 library were retained). After the voom transformation, the model was fitted in limma using weighted least squares via the lmFit () function. The log2 fold-change (log2FC) values were obtained for each comparison via the makeContrasts () function with default parameters. Empirical Bayesian smoothing of standard errors preceded the extraction of differentially expressed genes (log2FC >1 and p < 0.05) via the topTable () function.
The Venn diagram was prepared using the venn R package (v1.9) and modified visually using Inkscape (v1.1) to include item labels. Heatmaps were visualized using the Heatmapper that utilizes ggplot2, d3heatmap, and gplot R packages (Babicki et al., 2016). An average linkage served as the clustering method, whereas Spearman’s rank correlation was applied as the distance measurement method. A dendrogram was visualized via clustering to rows and columns. The genes that were intersected using the Venn diagram and presented on the heatmap served as active variables in the multiple factor analysis (MFA) together with features representing WWOX/AP-2 expression statuses (native or overexpressed) among RT-112, HT-1376, and CAL-29 cell lines. Spatial partitioning across Dim1 and Dim2 components was visualized using FactoMineR and factoextra R packages.
Genes identified from the differential expression analysis were visualized alongside WWOX/AP-2 in the form of a network using Cytoscape (v3.7), which served as a tool for collecting data from a few ncRNA-related databases: miRDB (Wong and Wang, 2015), Arena-Idb (Bonnici et al., 2018), miRNet (Chang et al., 2020), LncRRIsearch (Fukunaga et al., 2019), snoDB (Bouchard-Bourelle et al., 2020), and RISE (Gong et al., 2018). In order to acquire the most relevant interactions, these servers were employed with various parameters that are summarized in the following sentences. miRDB was searched using miRNA names; only targets with the highest score were selected (e.g., if the highest available target score on a scale of 1–100 was 99, only targets with this score were selected and not the targets with a score of ≤98). Arena-Idb was queried by ncRNA names, and all interactions were acquired due to their low amount (typically four targets per ncRNA). miRNet lacked the targets for most input genes, so a similar approach was followed as in Arena-Idb; the GraphML files were downloaded via the “ncRNAs” query list. LncRRIsearch was queried with Ensembl gene ID and an energy threshold of −16 kcal/mol. Together with snoDB, RISE was used to acquire data on RNA–RNA interactions for snoRNAs; databases were searched using snoRNA names that directly provided results in a tabular form.
The part of a network that was directly connected to WWOX/AP-2 (henceforth denoted as the “interconnected network”) was subjected to functional annotations via the Database for Annotation, Visualization, and Integrated Discovery (DAVID) (Sherman et al., 2022) and Protein Annotation Through Evolutionary Relationships (PANTHER) (Thomas et al., 2022). DAVID was employed with Benjamini–Hochberg (BH) adjusted p-values to search among the “GOTERM_BP/MF/CC_DIRECT” ontology and pathways from Reactome/KEGG/WikiPathways, whereas the PANTHER overrepresentation test was annotated only using “PANTHER pathways” with Fisher’s exact test. DAVID was also used to acquire the entire annotation clusters, among which the two best clusters were selected owing to their higher enrichment scores than the remaining clusters. At this study stage, the emphasis was put on WWOX, considering the results acquired through the methodological workflow so far (see Sections 3.1 and 3.2 for more details). Genes from the interconnected network were subjected to Multiple Support Vector Machine Recursive Feature Elimination (mSVM-RFE) (Duan et al., 2005) that enabled the selection of best features (i.e., genes) distinguishing WWOX-overexpressing cellular variants from those with native WWOX expression. Feature ranking was executed via svmRFE () with k-fold cross-validation (CV) of k = 10 to include a multiplicity of mSVM-RFE and halve.above = 100. In order to allow SVM fitting, the R package e1071 was included in the R environment. Top features across all folds were obtained using writeFeatures () with a list of genes ordered by the ascending AvgRank value (the lower the number, the better the features). The expression of the most relevant genes in cellular variants with different WWOX levels was visualized using the vioplot (v0.4.0) R package.
One of the most relevant ncRNAs from mSVM-RFE (i.e., LINC01137) was selected as the initial point for further WWOX-related research since it was the most credible result to unravel the direct lncRNA/miRNA/mRNA axis that contains WWOX (see Section 3.2. for details). Primarily, the genomic location of LINC01137 was visualized through the UCSC Genome Browser (UCSC stands for the University of California, Santa Cruz). Transcription factors (TFs) that are top interactors of the WWOX protein were acquired from the literature (Hussain et al., 2018) and further investigated for their binding sites near the genomic location of LINC01137 via the Gene Transcription Regulation Database (GTRD v20.06) (Yevshin et al., 2019). Similar to the previous methodology section, the gene expression was visualized using the vioplot R package; the genes in close proximity to the genomic location of LINC01137, as well as the WWOX-interacting TFs, were included. The ENCORI database (Li et al., 2014) was employed to perform pairwise RNA–RNA co-expression analysis between selected ncRNAs. Subsequently, the common function (top similarly regulated pathways) of the involved messenger RNA (mRNA)-encoding genes (data for ncRNAs were unavailable) was predicted via correlation-based gene set enrichment analysis (corGSEA) under the Correlation AnalyzeR server (Miller and Bishop, 2021) assisted by a few literature datasets, FANTOM6 database, and Molecular Signatures Database (MSigDB).
Total RNA was extracted using the Extracol reagent (EURx, Poland) according to the manufacturer’s guidelines. Using ImProm-II™ reverse transcriptase (Promega, Madison, WI, United States), 10 mg of total RNA was reverse-transcribed into cDNA. After diluting each cDNA sample with DEPC water to a total volume of 140 μL, 2 μL was used for real-time quantitative PCR (GoTaq® qPCR Master Mix, Promega). Each cellular variant was measured in triplicate using the LightCycler® 480 (Roche Applied Science) with initial denaturation at 95°C for 2 min, followed by 45 cycles of 95°C for 30 s and annealing at 60°C for 30 s. Primer sequences for references and genes of interest are presented in Supplementary Table S1. The amplification of specific transcripts was confirmed by the melting curves at the end of each PCR. The relative expression level was determined using appropriate references: H3F3A, RPLP0, and RPS17 were used for protein-encoding genes (UPF1, RBM22, and ZC3H12A), whereas U6 served as an endogenous control for the expression of selected ncRNAs (LINC01137, MIR6732, and MIR186). The Universal Human Reference RNA (Stratagene, La Jolla, CA, United States) was applied as a calibrator. LinRegPCR (v2021.2) was implemented to determine the baseline and measurements of real-time qPCR efficiency. The relative expression level was calculated using the Pfaffl method (Pfaffl, 2001).
Promising results were observed after implementing the workflow from previous methodological sections that directed the study to certify the observations in a broader context, i.e., using data from additional BLCA cell lines and bladder cancer patients. The DepMap portal (depmap.org/portal/; accessed on 10 January 2023) served as the source of the Cancer Cell Line Encyclopedia (CCLE) dataset that includes files from RNA sequencing of tumor in vitro models. Data on bladder cancer were acquired from two files from the dataset: “CCLE_RNAseq_rsem_genes_tpm_20180929.txt” and “CCLE_miRNA_20180525.gct.” Cell lines featuring the intended expression profile (i.e., with higher expression of WWOX and LINC01137 but lower MIR186 expression) were selected using the UpSetR package, which provides a scalable matrix-based alternative to Venn diagrams for performing intersection analysis and presenting the size or properties of sets (Conway et al., 2017). A similar procedure was executed in terms of bladder cancer patients, the cohort of which was acquired from The Cancer Genome Atlas (TCGA) via the Broad Institute Firehose (gdac.broadinstitute.org/; accessed on 14 January 2023) and The Atlas of ncRNA in Cancer (TANRIC) (Li et al., 2015). Together with our GEO dataset (GSE193659), the filtered data from the CCLE (henceforth denoted as “CCLE-BLCA”) and TCGA (henceforth denoted as “TCGA-BLCA”) were analyzed using MFA (similar to Section 2.4.) to reduce the multidimensional expression data of genes from the representative gene sets (see Section 3.4. for details).
Except for the TCGA-BLCA cohort, the study workflow so far utilized data from cancer cell lines, making the patients’ data unconfirmed at this stage. Therefore, the validation cohort was searched, taking into account the presence of at least 50 patients and at least two clinical endpoints, such as overall survival (OS), disease-specific survival (DSS), or progression-free interval (PFI). These requirements were chosen to facilitate and enrich one of the subsequent steps, i.e., the survival analysis that was performed using survival and survminer R packages. Eventually, GSE31684 was selected as a validation cohort with regard to the MFA from the previous section; clinical and expression data were acquired from GEO. Survival endpoints were evaluated on patients from GSE31684 and TCGA-BLCA using optimal cutoff values for the expression of a single gene [via our in-house Evaluate Cutpoints tool (Ogluszka et al., 2019)] or a few genes at once combined into the signature (via the which() function and further survival R package). Hazard ratios were visualized using the forestmodel R package with default parameters.
TargetScan (McGeary et al., 2019) was employed to identify possible binding between WWOX mRNA and miRNA of interest. Once the database was searched using the option “enter a human gene symbol,” the targeting of WWOX mRNA was evaluated using a table containing, e.g., context++ score (CS) for each miRNA–mRNA pair. Subsequently, the ncFANs tool (Zhang et al., 2021) was used to investigate the pairing between lncRNAs and miRNAs, as well as to receive additional data on the co-expression and formation of RNA–DNA triple helices (together with the number of triplex-forming oligonucleotides [TFOs]). The server was accessed via the ncFANs-NET module and queried using the lncRNA of interest, i.e., LINC01137. The ncFANs-NET module was built using large-scale sequencing data from public databases; thus, it should be more robust and valid. The co-expression network was selected with the “overall cancer” method and correlation coefficient ≥0.5, whereas the centric regulatory network was built with a miRanda score of ≥140 to reveal potential miRNA response elements (MREs) in LINC01137. Selected MREs were examined using the RNAhybrid tool (Rehmsmeier et al., 2004) to estimate the interaction’s minimum free energy (MFE).
The normality of the distribution was determined by the Shapiro–Wilk test; the statistical significance was evaluated with an unpaired t-test or Mann–Whitney test. The results with a p-value less than 0.05 were considered statistically significant.
The methodology described previously is summarized alongside the main study findings in Figure 1. This allowed us to visualize which sections of methods or results correspond to each other and to which stage of the study they were assigned. The individual parts of the results and their discussion are presented in the following sections.
FIGURE 1. Overview of the methodology and results in consecutive stages of the current study. The first column represents the methodology, whereas the second summarizes the main results. Rows correspond to study stages. The workflow is depicted to facilitate the interpretation of a particular section. For each rectangle, the corresponding sections from the main text are indicated.
Initially, the differentially expressed ncRNA-encoding genes (DEGs) among cellular variants (KK, KA, KC, WK, WA, and WC) were visualized (Figure 2A), and their intersection for all pairwise comparisons [adapted from our previous research (Kolat et al., 2022)] was analyzed (Figure 2B). In total, 16 lncRNAs, nine miRNAs, three snoRNAs, and two snRNAs were identified. The central clade on the row dendrogram (marked with purple in Figure 2A) suggested that about half of DEGs stably depend on the presence of WWOX overexpression. The Venn diagram certified that WWOX might be superior to AP-2α/γ in regulating ncRNAs, the intersection between comparisons adapted from the previous research study, even if sparse, is only present for WK/KK, WA/KK, and WC/KK. The multidimensionality reduction provided a general view of the samples, which enabled grouping them based on the expression profile of WWOX and AP-2α/γ. Ultimately, this prompted us to focus solely on WWOX instead of WWOX combined with AP-2 [the latter was the case in the previous research (Kolat et al., 2022)] since the cellular variants were distinguished by WWOX regardless of whether it was analyzed alone or in combination with AP-2α/γ (Figure 2C). Even if the interaction between AP-2α/γ and ncRNAs is known (Kolat et al., 2019), this regulation might be subordinate to WWOX influence, as described specifically for AP-2α/γ (Kolat et al., 2021a) or regarding the general superiority of WWOX function (Pospiech et al., 2018). With regard to ncRNA data from our CAGE-seq experiment, it appears that investigating WWOX instead of AP-2α/γ is a relevant approach. Thus, the cellular variants with WWOX overexpression (represented by WK, WA, and WC) will be collectively compared to those without WWOX overexpression (represented by KK, KA, and KC) beginning from the consecutive part of the study (as shown in Figure 1).
FIGURE 2. Initial comparison of cellular variants. (A) Differential expression analysis revealed that the expression profile of about half of the DEGs (central clade marked with purple) stably differed between WWOX-overexpressing variants (WK, WA, and WC) and those with native WWOX expression (KK, KA, and KC). (B) Intersection analysis suggested that WWOX might be superior to AP-2α/γ in regulating ncRNAs since the intersection between comparisons is present only for WK/KK, WA/KK, and WC/KK. (C) Multiple factor analysis ultimately revealed that the WWOX profile is more important for DEGs irrespective of whether analyzed alone or in combination with AP-2α/γ.
Elaboration of a network using the list of differentially expressed ncRNAs and various databases (see Section 2.5.) provided a comprehensive view of RNA interactomes (Figure 3A) for WWOX, as well as AP-2α and AP-2γ (these were also included to verify an assumption from the previous section, i.e., whether WWOX is more important than AP-2α/γ in discriminating DEGs). At first glance, some of the ncRNAs from the central clade on the row dendrogram (Figure 2A) generated a shared network with WWOX/AP-2 (only the part being an interconnected network is visible in Figure 3A; the complete scheme with divided subnetworks is shown in Supplementary Figure S1). The most prevalent group of ncRNAs regarded as the input (framed with a solid line) included lncRNAs, but some miRNAs, snoRNAs, and snRNAs were also present. Annotation clustering of Gene Ontology revealed that the gene expression regulation behind the interconnected network might be involved in angiogenesis and proliferation (Figure 3B); our cellular variants were previously confirmed to affect these two processes diversely (Kolat et al., 2021a; Kolat et al., 2022). The complete functional annotation is shown in Supplementary Table S2.
FIGURE 3. RNA interactome visualization. (A) An interconnection overview confirmed that 12 DEGs form a network with WWOX and AP-2α/γ (see Supplementary Figure S1 for the complete scheme with divided subnetworks). (B) Gene Ontology using annotation clusters revealed that the network might regulate angiogenesis and proliferation. (C) Recursive feature elimination indicated that the two best genes (HIPK1-AS1 and LINC01137) are part of an interconnected network. (D) Expression differences of the top four DEGs from mSVM-RFE suggested that only LINC01137 can directly reveal the WWOX-containing lncRNA/miRNA/mRNA axis since its expression increases in WWOX-overexpressing variants (i.e., WK, WA, and WC). (E) Synopsis that justifies proceeding with LINC01137-related investigation.
Subsequently, the entire network from Supplementary Figure S1 was examined to indicate the most relevant genes that distinguish WWOX-overexpressing variants (i.e., WK, WA, and WC) from those without WWOX overexpression (i.e., KK, KA, and KC). The mSVM-RFE algorithm ranked the genes according to their AvgRank value (the lower the number, the better the features), indicating that the top genes include HIPK1-AS1, LINC01137, LINC01063, and SNED1-AS1, which all encode lncRNAs (Figure 3C). Expression of these genes was shown on separate scales (Figure 3D), emphasizing that only the expression of LINC01137 was elevated in WWOX-overexpressing variants. Moreover, LINC01063 and SNED1-AS1 were not a part of the interconnected network visualized in Figure 3A and had noticeably worse AvgRank values than HIPK1-AS1 and LINC01137. Increased expression of LINC01137 during WWOX overexpression suggests that LINC01137 might be able to unravel a direct WWOX-containing lncRNA/miRNA/mRNA axis (Figure 3E) because the expression of lncRNA and mRNA in such axes is oftentimes positively correlated (Pacholewska and Sung, 2019; Song et al., 2022). In summary, LINC01137 was selected as the most relevant gene because a) it was a part of the interconnected network, b) it had been ranked as one of the best genes according to mSVM-RFE, c) its expression change between cellular variants was statistically significant, and d) it was increased in WWOX-overexpressing variants so that it might be able to unravel a direct WWOX-containing lncRNA/miRNA/mRNA axis. The following section is dedicated to identifying the mechanism by which WWOX upregulates LINC01137.
Insights into the LINC01137–WWOX relationship began with an investigation of the genomic location of LINC01137. Based on the UCSC Genome Browser (Figure 4A, top panel), one can perceive that this locus is worth investigating since LINC01137 is located near MIR6732, the miRNA-encoding gene that was also identified during the differential expression analysis (Figures 2A, B; WK vs. KK comparison). LINC01137 shares the promoter with ZC3H12A, a gene encoded on the plus (or positive-sense) strand of DNA, and only LINC01137 is encoded on the minus (or negative-sense) strand. Given that the sequence of both genes does not overlap, they are controlled under the so-called divergent promoter, i.e., a bidirectional region that enables the co-regulation of divergent transcription (Seila et al., 2009; Warman et al., 2021). It was justifiable to profoundly investigate the close proximity of the LINC01137 locus with regard to the related genes (MIR6732 and ZC3H12A) and transcription factors binding to this locus [as for TFs, our decision was to avail the protein interactome of WWOX (Hussain et al., 2018) to search among top interactors]. Two candidate transcription factors, RBM22 and UPF1, were evaluated for their binding sites near the divergent promoter (Figure 4A, bottom panel). Although binding sites of RBM22 were more prevalent, their localization overlapped with ZC3H12A and not LINC01137. At the same time, the RBM22 binding site that was the closest to LINC01137 was outpaced by the binding site of UPF1 which completely overlapped with the location of a divergent promoter. This suggests that UPF1 might be involved in the WWOX-dependent upregulation of LINC01137 and simultaneous RBM22-independent co-regulation of ZC3H12A. Similar to LINC01137 (Figure 3D), our CAGE-seq data certified that UPF1 and ZC3H12A are also significantly upregulated in WWOX-overexpressing cellular variants (i.e., WK, WA, and WC); however, no statistical significance was noted for RBM22 and MIR6732 (Figure 4B). Altogether, LINC01137 appears to be a good direction for further WWOX-related research studies, provided that it is possible to identify an miRNA that would complete the RNA axis. Candidate miRNAs were proposed based on the network visualized in Figure 3A; miR-186 and miR-6515 were found between LINC01137 and WWOX. After genes encoding these two miRNAs were correlated with LINC01137 among BLCA patients, the statistically significant negative correlation between LINC01137 and MIR186 was noted (Figure 4C). In order to verify all observations described in this section, we decided to perform real-time PCR; the results were concordant for LINC01137, MIR186, ZC3H12A, and UPF1 (Figure 4D). Although slight deviations were observed for RBM22, this did not affect the further study stages that focused entirely on ZC3H12A, UPF1, WWOX, LINC01137, and miR-186, especially the last three being an example of the lncRNA/miRNA/mRNA axis. Henceforth, the relationships between ZC3H12A, UPF1, WWOX, LINC01137, and miR-186 were denoted as the “core network.” Before focusing on the LINC01137/miR-186/WWOX axis, an additional enrichment analysis was performed for WWOX, UPF1, and ZC3H12A to predict their functional similarities (due to the limitations of Correlation AnalyzeR: only protein-encoding genes were investigated via this tool). Two annotations, namely, the double-strand break repair (DSBR) and smoothened (Smo) signaling pathway, were found to be repeated in the corGSEA of WWOX and UPF1, as well as WWOX and ZC3H12A (Supplementary Figure S2). Their usefulness in facilitating the workflow is explained in the following section.
FIGURE 4. Insights into LINC01137 and related genes or molecules. (A) The genomic location of LINC01137 revealed that it shares a divergent promoter with ZC3H12A (top). Binding sites of two WWOX-interacting transcription factors, namely, RBM22 and UPF1, indicated that one binding site of UPF1 overlaps with a divergent promoter location (bottom). (B) Expression differences of investigated genes using CAGE-seq data suggested that UPF1 might be involved in WWOX-dependent upregulation of LINC01137 and simultaneous RBM22-independent co-regulation of ZC3H12A. (C) Correlation between LINC01137 and potentially related miRNAs (acquired from the interconnected network from Figure 3A) implies that LINC01137 can be negatively correlated with MIR186. (D) Expression differences of investigated genes using real-time quantitative PCR data aligned with CAGE-seq findings.
The study was directed to certify the results in a broader context. In addition to RT-112, HT-1376, and CAL-29 cell lines, we decided to employ the CCLE and TCGA, which served as sources of BLCA data from cell lines and patients, respectively. To avoid excessive reduction of the group size, an emphasis was put on the LINC01137/miR-186/WWOX axis. The samples with specific expression profiles (i.e., high expression of WWOX and LINC01137 but low MIR186 expression; the second group with an opposite profile) were initially extracted. This enabled the selection of seven BLCA cell lines from the CCLE repository and 71 patients from TCGA-BLCA (Figure 5A). Afterward, each dataset was subjected to subsidiary MFA to identify the most relevant genes that explain the differences between opposite expression profiles (Supplementary Figure S3 for CCLE-BLCA; Supplementary Figure S4 for TCGA-BLCA; Supplementary Figure S5 for GSE193659). Five independent analyses were performed for each dataset; the number of analyses was dictated by separate biological processes that were included on the basis of the core network function. Thus, two corGSEA annotations related to ZC3H12A and UPF1 (i.e., DSBR and Smo signaling) were represented by the Reactome gene set R-HSA-5693606 and the MSigDB gene set M14890, respectively. The other three gene sets from MSigDB—M5902, M4627, and M10617—represented the apoptosis, proliferation, and inflammatory response, respectively, i.e., the processes that were determined on the basis of the literature data on core network genes. Namely, the interaction between ZC3H12A and UPF1 is known to orchestrate the cleavage of stem–loop-containing mRNAs during the early phase of inflammation (Mino et al., 2019). On the other hand, the role of miR-186 is widely described in terms of cell proliferation (Xiang et al., 2020). Data on LINC01137 functionality are scarce, which prompted us to access the FANTOM6 database and select the most significant annotation, i.e., the regulation of apoptosis; this is in line with a prominent role of WWOX in this process (Chen et al., 2021; Taouis et al., 2021). The intersection between datasets (Supplementary Figure S6) allowed us to identify genes that were further evaluated for their appropriateness in differentiating expression profiles (Supplementary Figure S7).
FIGURE 5. Investigation of the relationship between LINC01137, MIR186, and WWOX. (A) Relevant cell lines and patients from CCLE-BLCA and TCGA-BLCA were temporarily selected to facilitate the implementation of the definitive MFA (see Supplementary Figures S3–S7 for intermediate stages of the study workflow). (B,C) Definitive multiple factor analysis of patients and cell lines from CCLE-BLCA, TCGA-BLCA, and GSE193659 confirmed the positive correlation between WWOX and LINC01137 and a negative correlation between MIR186 and WWOX or LINC01137. All samples with various expression profiles, marked with black, blue, and red in subfigure (A), are included in the last two subfigures.
Ultimately, seven genes (i.e., KRT18, HES1, VCP, FTH1, IFITM3, RAB34, and CLU) met all requirements that stemmed from the analyses presented in Supplementary Figures S3–S7. These requirements were as follows: a) well explanation of the aforementioned expression profiles in CCLE-BLCA, TCGA-BLCA, and GSE193659 datasets; b) an intersection between all three datasets; and c) no distortion of the examined expression profile. Expression of the aforementioned genes (alongside LINC01137, MIR186, and WWOX) served as an input to the definitive MFA that was performed to summarize what GSE193659, CCLE-BLCA, and TCGA-BLCA share in common. Compared to subsidiary MFAs, all cell lines and patients were included in the definitive MFA (i.e., not only the one marked with blue or red in Figure 5A, but also the samples with heterogeneous expression profiles marked with black). Despite the inclusion of additional samples, the positive correlation between WWOX and LINC01137 was still evident, alongside the negative correlation between WWOX and MIR186 or LINC01137 and MIR186 (Figure 5B). The same is visible when distributing all samples within Dim1 and Dim2, with the best results for extremes of expression (Figure 5C).
Except for the TCGA-BLCA cohort, the study workflow so far has used data from cancer cell lines, making the verification of results on independent cohorts justifiable. The selection of the appropriate dataset (see Section 2.9. for details) revealed the GSE31684 cohort from the GEO, in which the definitive MFA from the previous section was validated. The results indicated that despite the lack of an evident correlation between MIR186 and LINC01137, the WWOX gene still correlated positively with LINC01137 and negatively with MIR186 (Supplementary Figure S8). Together with WWOX, LINC01137, and MIR186, the remaining genes from the definitive MFA (i.e., KRT18, HES1, VCP, FTH1, IFITM3, RAB34, and CLU) were subsequently subjected to survival analysis that included not only the evaluation of each gene separately but also the gene signatures. As for the gene signatures, although we predominantly investigated various WWOX/LINC01137/MIR186 expression combinations, a separate part was dedicated to the entire core network and two genes that presented congruent results in two MFA correlation circles (Figure 5B; Supplementary Figure S8): KRT18 (positively correlated to MIR186) and CLU (positively correlated to WWOX). The entire set of Kaplan–Meier survival curves is visualized in Supplementary Figure S9 for TCGA-BLCA and Supplementary Figure S10 for GSE31684, whereas the simplified summary for both cohorts, including the hazards ratio, in a tabular form is shown in Supplementary Table S3. For instance, patients had superior OS when the expression of WWOX, LINC01137, ZC3H12A, and UPF1 was high but MIR186 expression was low, compared to the opposite profile (Supplementary Table S3; Supplementary Figure S9). For clarity and the given primary purpose of this section, only the data for WWOX, LINC01137, MIR186, and the related gene signatures are presented in Figure 6. High WWOX expression was found to prolong DSS and PFI; a similarity was found in terms of LINC01137 and OS or PFI. It is also encouraging to note statistically significant results for the WWOX/MIR186 ratio, where patients with a high ratio (i.e., with higher expression of WWOX but lower MIR186) survive longer than those with opposite ratios, as estimated using OS. Furthermore, a high LINC01137/MIR186 ratio improved DSS and PFI. The gene/gene ratio was used for MIR186 and either WWOX or LINC01137 due to their supposedly opposite characteristics. However, in order to analyze the relationship between WWOX and LINC01137, we selected patients having either high or low expression of these two genes at once. The DSS-related example is visualized in the last row of Figure 6; the intersected patients constituted at least 70% of the reference group, a group that determined the maximum overlap due to its size. The remaining equivalents for OS and PFI are visualized in Supplementary Figures S9, S10.
FIGURE 6. Survival analysis of WWOX, LINC01137, MIR186, and the related gene signatures. The bottom-right graph represents the figure’s legend. Investigated groups are marked with red and blue on the left side of each row. Except for the last row, the columns represent OS, DSS, and PFI. The last row visualizes an example of the UpSetR-created intersection of patients (DSS endpoint) having either high WWOX and high LINC01137 (red) or the exact opposite (blue). A complete set of survival curves are visualized in Supplementary Figure S9 (TCGA-BLCA) and Supplementary Figure S10 (GSE31684), whereas the simplified summary with hazard ratios is presented in Supplementary Table S3.
The prognostic significance of WWOX has been evaluated in different tumors and various clinical endpoints (Zelazowski et al., 2011; Wen et al., 2017; Kaluzinska et al., 2021), including the statistically significant prediction of BLCA progression (Ramos et al., 2008), which aligns with our observations. Concerning LINC01137, the literature lacks data on BLCA (Li H. J. et al., 2021) and is inconsistent. For example, Huang and Huang (2021) proposed that LINC01137 was associated with poorer overall survival of patients with non-small-cell lung cancer. However, Lin et al. (2018) performed a prognostic meta-analysis on the same tumor, which revealed that LINC01137 is highly expressed in patients with low risk scores. Further research on this lncRNA is advisable, especially since the ratio of LINC01137 between tumor and normal specimens depends on the type of cancer tissue (Du et al., 2021). Lastly, the patient’s survival worsened when miR-186 was overexpressed in pancreatic ductal adenocarcinoma (Zhang et al., 2015) and esophageal cancer (Xue et al., 2021); however, no statistical significance was noted in luminal A breast cancer (Denkiewicz et al., 2019). As for BLCA, miR-186 was found to be the critical exosome-derived molecule that can induce natural killer cell dysfunction (Huyan et al., 2022), the process that entails the diminished production of effector cytokines and facilitates immune surveillance escape (Zhang W. et al., 2022).
To ultimately demonstrate the relationship between WWOX, LINC01137, MIR186, and two other genes from the core network (i.e., ZC3H12A and UPF1), we employed a few tools and collected the available data from the literature. First, the direct interaction between WWOX and UPF1 proteins is possible via the proline-rich motif of UPF1 (1005PPGY1008) and the first tryptophan domain of WWOX (WW1) (Hussain et al., 2018). The recognition of proline-rich motifs by the WW1 domain is a common manner by which WWOX interacts with many protein partners (Abu-Odeh et al., 2014). Subsequently, in this network, UPF1 binds to the divergent promoter of LINC01137 and ZC3H12A, as was investigated using GTRD. Expression changes in cellular variants suggest that UPF1 upregulates these two genes. Afterward, LINC01137 can act as an miRNA sponge, inhibiting the degradation of WWOX mRNAs carried out by miR-186. Nevertheless, there was still a need to specify which mature strand from the miR-186 stem–loop could target the mRNA of WWOX and whether the same strand can be sponged by LINC01137. The TargetScan database confirmed the possibility of miR-186-5p (but not -3p) binding to 3′-UTR of WWOX mRNA via 5'CAAAGAAU3' and 5'AUUCUUUA3', respectively (CS of −0.19). Since the seed site was stringent, one can infer that mRNA will be degraded (Saito and Saetrom, 2010; Mullany et al., 2016). On the other hand, the potential binding of miR-186 and LINC01137 with the specific sequence was not confirmed straightforwardly in any database. To compensate for this, we employed the ncFANs tool to calculate the miRanda score between LINC01137 and any miR-186 mature sequence, determining the possible region of their interaction. The results revealed that MREs for miR-186-5p (but not -3p) are present in the 1838–1861 positions of LINC01137 with a miRanda score of 150.00. Within this location (i.e., in the 1854–1861 position of lncRNAs), the RNAhybrid tool indicated the 7mer-m8 site of LINC01137 (5'AUUCUUUU3') that matches the same sequence of miR-186-5p (5'CAAAGAAU3') as WWOX. MFE of binding between LINC01137 and miR-186-5p was −16.6 kcal/mol [similar works accept a threshold of around −15 kcal/mol (Liu et al., 2013; Dandare et al., 2021)]. Since LINC01137 was the molecule that facilitated the unraveling of relationships, we employed other modules of the ncFANs tool to further investigate if any other dependencies with the rest of the core network exist. Interestingly, not only was LINC01137 confirmed to co-express with ZC3H12A (r > 0.5; FDR <0.05; certifying their co-regulation under the divergent promoter), but it also forms the RNA–DNA triple helix (also known as triplex) in the genomic location of WWOX using 14 TFOs [typically, TFOs are 12–28 in length (Li et al., 2022)]. Such structures can repress or induce gene expression (Alecki and Vera, 2022; Cecconello et al., 2022; Warwick et al., 2023), but the latter can be assumed in our case. The module evaluating triple helices also returned one TFO within the ZC3H12A gene, which should be noted for future studies. In general, these aspects should be profoundly investigated by more sophisticated methods such as triplex-seq (Warwick et al., 2022). Altogether, relationships in the core network are visualized in Figure 7.
FIGURE 7. Visualization of the core network. The WWOX protein can interact with the UPF1 transcription factor to upregulate ZC3H12A and LINC01137 genes. The latter gene encodes lncRNA that sponges miR-186-5p and recognizes WWOX mRNA. Moreover, LINC01137 is involved in a positive feedback loop with WWOX owing to its ability to form an RNA–DNA triplex in the genomic location of WWOX.
Determining the influence of WWOX on the ncRNA interactome in bladder cancer is still in its infancy; the subject merited investigation and was inaugurated by the current study. Following the construction of the ncRNA-containing network, various bioinformatics tools utilized the data from cell lines and patients, proposing a novel WWOX-related axis from the RNA interactome of bladder cancer. In this axis, WWOX is involved in the positive feedback loop with LINC01137, i.e., the lncRNA that sponges WWOX-targeting miR-186-5p. It is advisable to perform subsequent research to depict the relationships in a broader context, which may confer benefits to the clinic.
The datasets presented in this study can be found in online repositories. The names of the repository/repositories and accession number(s) can be found at: https://www.ncbi.nlm.nih.gov/geo/; GSE193659.
DK and AB conceptualized the article. DK, ŻK-K, KK, MO, EP, and AB established the methodology. AB supervised the article. DK and ŻK-K visualized the results. DK wrote the original draft. DK, ŻK-K, KK, MO, EP, and AB reviewed and edited the article. All authors contributed to the article and approved the submitted version.
This research was funded by both the Medical University of Lodz (grant number: 503/0-078-02/503-01-001-19-00) and the National Science Centre of Poland (grant number 2016/21/B/NZ2/01823). The funding bodies had no role in the study design, collection, analysis, and interpretation of data and in writing the manuscript.
The authors declare that the research was conducted in the absence of any commercial or financial relationships that could be construed as a potential conflict of interest.
All claims expressed in this article are solely those of the authors and do not necessarily represent those of their affiliated organizations, or those of the publisher, the editors, and the reviewers. Any product that may be evaluated in this article, or claim that may be made by its manufacturer, is not guaranteed or endorsed by the publisher.
The Supplementary Material for this article can be found online at: https://www.frontiersin.org/articles/10.3389/fgene.2023.1214968/full#supplementary-material
Abu-Odeh, M., Bar-Mag, T., Huang, H., Kim, T., Salah, Z., Abdeen, S. K., et al. (2014). Characterizing WW domain interactions of tumor suppressor WWOX reveals its association with multiprotein networks. J. Biol. Chem. 289 (13), 8865–8880. doi:10.1074/jbc.M113.506790
Alecki, C., and Vera, M. (2022). Role of nuclear non-canonical nucleic acid structures in organismal development and adaptation to stress conditions. Front. Genet. 13, 823241. doi:10.3389/fgene.2022.823241
Babicki, S., Arndt, D., Marcu, A., Liang, Y., Grant, J. R., Maciejewski, A., et al. (2016). Heatmapper: Web-enabled heat mapping for all. Nucleic Acids Res. 44 (1), W147–W153. doi:10.1093/nar/gkw419
Bonnici, V., Caro, G., Constantino, G., Liuni, S., D'Elia, D., Bombieri, N., et al. (2018). Arena-idb: A platform to build human non-coding RNA interaction networks. BMC Bioinforma. 19 (10), 350. doi:10.1186/s12859-018-2298-8
Bouchard-Bourelle, P., Desjardins-Henri, C., Mathurin-St-Pierre, D., Deschamps-Francoeur, G., Fafard-Couture, E., Garant, J. M., et al. (2020). snoDB: an interactive database of human snoRNA sequences, abundance and interactions. Nucleic Acids Res. 48 (D1), D220–D225. doi:10.1093/nar/gkz884
Cecconello, A., Magro, M., Vianello, F., and Simmel, F. C. (2022). Rational design of hybrid DNA-RNA triplex structures as modulators of transcriptional activity in vitro. Nucleic Acids Res. 50 (22), 13172–13182. doi:10.1093/nar/gkac1131
Chang, L., Zhou, G., Soufan, O., and Xia, J. (2020). miRNet 2.0: network-based visual analytics for miRNA functional analysis and systems biology. Nucleic Acids Res. 48 (W1), W244–W251. doi:10.1093/nar/gkaa467
Chen, T., Gao, F., Feng, S., Yang, T., and Chen, M. (2015). MicroRNA-134 regulates lung cancer cell H69 growth and apoptosis by targeting WWOX gene and suppressing the ERK1/2 signaling pathway. Biochem. Biophys. Res. Commun. 464 (3), 748–754. doi:10.1016/j.bbrc.2015.07.021
Chen, Y. A., Sie, Y. D., Liu, T. Y., Kuo, H. L., Chou, P. Y., Chen, Y. J., et al. (2021). Normal cells repel WWOX-negative or -dysfunctional cancer cells via WWOX cell surface epitope 286-299. Commun. Biol. 4 (1), 753. doi:10.1038/s42003-021-02271-2
Conway, J. R., Lex, A., and Gehlenborg, N. (2017). UpSetR: an R package for the visualization of intersecting sets and their properties. Bioinformatics 33 (18), 2938–2940. doi:10.1093/bioinformatics/btx364
Dandare, A., Rabia, G., and Khan, M. J. (2021). In silico analysis of non-coding RNAs and putative target genes implicated in metabolic syndrome. Comput. Biol. Med. 130, 104229. doi:10.1016/j.compbiomed.2021.104229
Denkiewicz, M., Saha, I., Rakshit, S., Sarkar, J. P., and Plewczynski, D. (2019). Identification of breast cancer subtype specific MicroRNAs using survival analysis to find their role in transcriptomic regulation. Front. Genet. 10, 1047. doi:10.3389/fgene.2019.01047
Du, Y., Yang, H., Li, Y., Guo, W., Zhang, Y., Shen, H., et al. (2021). Long non-coding RNA LINC01137 contributes to oral squamous cell carcinoma development and is negatively regulated by miR-22-3p. Cell. Oncol. (Dordr) 44 (3), 595–609. doi:10.1007/s13402-021-00586-0
Duan, K. B., Rajapakse, J. C., Wang, H., and Azuaje, F. (2005). Multiple SVM-RFE for gene selection in cancer classification with expression data. IEEE Trans. Nanobioscience 4 (3), 228–234. doi:10.1109/tnb.2005.853657
Fabbri, M., Garzon, R., Cimmino, A., Liu, Z., Zanesi, N., Callegari, E., et al. (2007). MicroRNA-29 family reverts aberrant methylation in lung cancer by targeting DNA methyltransferases 3A and 3B. Proc. Natl. Acad. Sci. U. S. A. 104 (40), 15805–15810. doi:10.1073/pnas.0707628104
Fukunaga, T., Iwakiri, J., Ono, Y., and Hamada, M. (2019). LncRRIsearch: A web server for lncRNA-RNA interaction prediction integrated with tissue-specific expression and subcellular localization data. Front. Genet. 10, 462. doi:10.3389/fgene.2019.00462
Gao, K., Yin, J., and Dong, J. (2016). Deregulated WWOX is involved in a negative feedback loop with microRNA-214-3p in osteosarcoma. Int. J. Mol. Med. 38 (6), 1850–1856. doi:10.3892/ijmm.2016.2800
Garbo, S., Maione, R., Tripodi, M., and Battistelli, C. (2022). Next RNA therapeutics: The mine of non-coding. Int. J. Mol. Sci. 23 (13), 7471. doi:10.3390/ijms23137471
Gong, J., Shao, D., Xu, K., Lu, Z., Lu, Z. J., Yang, Y. T., et al. (2018). Rise: A database of RNA interactome from sequencing experiments. Nucleic Acids Res. 46 (D1), D194–D201. doi:10.1093/nar/gkx864
Han, J. B., Huang, M. L., Li, F., Yang, R., Chen, S. M., and Tao, Z. Z. (2020). MiR-214 mediates cell proliferation and apoptosis of nasopharyngeal carcinoma through targeting both WWOX and PTEN. Cancer Biother Radiopharm. 35 (8), 615–625. doi:10.1089/cbr.2019.2978
Han, Q., Wang, M., Dong, X., Wei, F., Luo, Y., and Sun, X. (2022). Non-coding RNAs in hepatocellular carcinoma: Insights into regulatory mechanisms, clinical significance, and therapeutic potential. Front. Immunol. 13, 985815. doi:10.3389/fimmu.2022.985815
Hua, H. W., Jiang, F., Huang, Q., Liao, Z., and Ding, G. (2015). MicroRNA-153 promotes Wnt/β-catenin activation in hepatocellular carcinoma through suppression of WWOX. Oncotarget 6 (6), 3840–3847. doi:10.18632/oncotarget.2927
Huang, Y. J., and Huang, C. J. (2021). Construction of a 5 immune-related lncRNA-based prognostic model of NSCLC via bioinformatics. Med. Baltim. 100 (37), e27222. doi:10.1097/MD.0000000000027222
Hung, P. S., Chuang, F. J., Chen, C. Y., Chou, C. H., Tu, H. F., and Lo, S. S. (2020). miR-187* enhances SiHa cervical cancer cell oncogenicity via suppression of WWOX. Anticancer Res. 40 (3), 1427–1436. doi:10.21873/anticanres.14084
Hussain, T., Lee, J., Abba, M. C., Chen, J., and Aldaz, C. M. (2018). Delineating WWOX protein interactome by tandem affinity purification-mass spectrometry: Identification of top interactors and key metabolic pathways involved. Front. Oncol. 8, 591. doi:10.3389/fonc.2018.00591
Huyan, T., Gao, L., Gao, N., Wang, C., Guo, W., Zhou, X., et al. (2022). miR-221-5p and miR-186-5p are the critical bladder cancer derived exosomal miRNAs in natural killer cell dysfunction. Int. J. Mol. Sci. 23 (23), 15177. doi:10.3390/ijms232315177
Jiang, Y., Zhao, L., Wu, Y., Deng, S., Cao, P., Lei, X., et al. (2022). The role of NcRNAs to regulate immune checkpoints in cancer. Front. Immunol. 13, 853480. doi:10.3389/fimmu.2022.853480
Kaluzinska, Z., Kolat, D., Bednarek, A. K., and Pluciennik, E. (2021). PLEK2, RRM2, gcsh: A novel WWOX-dependent biomarker triad of glioblastoma at the crossroads of cytoskeleton reorganization and metabolism alterations. Cancers (Basel) 13 (12), 2955. doi:10.3390/cancers13122955
Khawaled, S., Suh, S. S., Abdeen, S. K., Monin, J., Distefano, R., Nigita, G., et al. (2019). WWOX inhibits metastasis of triple-negative breast cancer cells via modulation of miRNAs. Cancer Res. 79 (8), 1784–1798. doi:10.1158/0008-5472.CAN-18-0614
Khawaled, S., Nigita, G., Distefano, R., Oster, S., Suh, S. S., Smith, Y., et al. (2020). Pleiotropic tumor suppressor functions of WWOX antagonize metastasis. Signal Transduct. Target Ther. 5 (1), 43. doi:10.1038/s41392-020-0136-8
Kolat, D., Kaluzinska, Z., Bednarek, A. K., and Pluciennik, E. (2019). The biological characteristics of transcription factors AP-2α and AP-2γ and their importance in various types of cancers. Biosci. Rep. 39 (3), BSR20181928. doi:10.1042/BSR20181928
Kolat, D., Kaluzinska, Z., Bednarek, A. K., and Pluciennik, E. (2021a). Corrigendum: Fragile gene WWOX guides tfap2a/TFAP2C-dependent actions against tumor progression in grade II bladder cancer. Front. Oncol. 11, 735435. doi:10.3389/fonc.2021.735435
Kolat, D., Kaluzinska, Z., Bednarek, A. K., and Pluciennik, E. (2021b). WWOX loses the ability to regulate oncogenic AP-2γ and synergizes with tumor suppressor AP-2α in high-grade bladder cancer. Cancers (Basel) 13 (12), 2957. doi:10.3390/cancers13122957
Kolat, D., Kaluzinska, Z., Bednarek, A. K., and Pluciennik, E. (2022). Determination of WWOX function in modulating cellular pathways activated by AP-2α and AP-2γ transcription factors in bladder cancer. Cells 11 (9), 1382. doi:10.3390/cells11091382
Le, P., Romano, G., Nana-Sinkam, P., and Acunzo, M. (2021). Non-coding RNAs in cancer diagnosis and therapy: Focus on lung cancer. Cancers (Basel) 13 (6), 1372. doi:10.3390/cancers13061372
Lekka, E., and Hall, J. (2018). Noncoding RNAs in disease. FEBS Lett. 592 (17), 2884–2900. doi:10.1002/1873-3468.13182
Li, J. H., Liu, S., Zhou, H., Qu, L. H., and Yang, J. H. (2014). starBase v2.0: decoding miRNA-ceRNA, miRNA-ncRNA and protein-RNA interaction networks from large-scale CLIP-Seq data. Nucleic Acids Res. 42, D92–D97. doi:10.1093/nar/gkt1248
Li, J., Han, L., Roebuck, P., Diao, L., Liu, L., Yuan, Y., et al. (2015). Tanric: An interactive open platform to explore the function of lncRNAs in cancer. Cancer Res. 75 (18), 3728–3737. doi:10.1158/0008-5472.CAN-15-0273
Li, C., Zhou, Z., Ren, C., Deng, Y., Peng, F., Wang, Q., et al. (2022). Triplex-forming oligonucleotides as an anti-gene technique for cancer therapy. Front. Pharmacol. 13, 1007723. doi:10.3389/fphar.2022.1007723
Li, H. J., Gong, X., Li, Z. K., Qin, W., He, C. X., Xing, L., et al. (2021). Role of long non-coding RNAs on bladder cancer. Front. Cell. Dev. Biol. 9, 672679. doi:10.3389/fcell.2021.672679
Li, H. X., Liu, F., and Xia, J. H. (2021). Effects of miR-670-5p on proliferation, migration and invasion of lung cancer cells. Zhongguo Ying Yong Sheng Li Xue Za Zhi 37 (5), 500–505. doi:10.12047/j.cjap.6102.2021.046
Lin, T., Fu, Y., Zhang, X., Gu, J., Ma, X., Miao, R., et al. (2018). A seven-long noncoding RNA signature predicts overall survival for patients with early stage non-small cell lung cancer. Aging (Albany NY) 10 (9), 2356–2366. doi:10.18632/aging.101550
Liu, C., Mallick, B., Long, D., Rennie, W. A., Wolenc, A., Carmack, C. S., et al. (2013). CLIP-based prediction of mammalian microRNA binding sites. Nucleic Acids Res. 41 (14), e138. doi:10.1093/nar/gkt435
Liu, C. J., Shen, W. G., Peng, S. Y., Cheng, H. W., Kao, S. Y., Lin, S. C., et al. (2014). miR-134 induces oncogenicity and metastasis in head and neck carcinoma through targeting WWOX gene. Int. J. Cancer 134 (4), 811–821. doi:10.1002/ijc.28358
Liu, C. G., Li, J., Xu, Y., Li, W., Fang, S. X., Zhang, Q., et al. (2021). Long non-coding RNAs and circular RNAs in tumor angiogenesis: From mechanisms to clinical significance. Mol. Ther. Oncolytics 22, 336–354. doi:10.1016/j.omto.2021.07.001
McGeary, S. E., Lin, K. S., Shi, C. Y., Pham, T. M., Bisaria, N., Kelley, G. M., et al. (2019). The biochemical basis of microRNA targeting efficacy. Science 366 (6472), eaav1741. doi:10.1126/science.aav1741
Miller, H. E., and Bishop, A. J. R. (2021). Correlation AnalyzeR: Functional predictions from gene co-expression correlations. BMC Bioinforma. 22 (1), 206. doi:10.1186/s12859-021-04130-7
Mino, T., Iwai, N., Endo, M., Inoue, K., Akaki, K., Hia, F., et al. (2019). Translation-dependent unwinding of stem-loops by UPF1 licenses Regnase-1 to degrade inflammatory mRNAs. Nucleic Acids Res. 47 (16), 8838–8859. doi:10.1093/nar/gkz628
Mullany, L. E., Herrick, J. S., Wolff, R. K., and Slattery, M. L. (2016). MicroRNA seed region length impact on target messenger RNA expression and survival in colorectal cancer. PLoS One 11 (4), e0154177. doi:10.1371/journal.pone.0154177
Ogluszka, M., Orzechowska, M., Jedroszka, D., Witas, P., and Bednarek, A. K. (2019). Evaluate Cutpoints: Adaptable continuous data distribution system for determining survival in Kaplan-Meier estimator. Comput. Methods Programs Biomed. 177, 133–139. doi:10.1016/j.cmpb.2019.05.023
O'Leary, V. B., Hain, S., Maugg, D., Smida, J., Azimzadeh, O., Tapio, S., et al. (2017). Long non-coding RNA PARTICLE bridges histone and DNA methylation. Sci. Rep. 7 (1), 1790. doi:10.1038/s41598-017-01875-1
Pacholewska, A., and Sung, M. H. (2019). lncRNA expression predicts mRNA abundance. Epigenomics 11 (10), 1121–1128. doi:10.2217/epi-2019-0003
Pfaffl, M. W. (2001). A new mathematical model for relative quantification in real-time RT-PCR. Nucleic Acids Res. 29 (9), e45. doi:10.1093/nar/29.9.e45
Pospiech, K., Pluciennik, E., and Bednarek, A. K. (2018). WWOX tumor suppressor gene in breast cancer, a historical perspective and future directions. Front. Oncol. 8, 345. doi:10.3389/fonc.2018.00345
Ramos, D., Abba, M., Lopez-Guerrero, J. A., Rubio, J., Solsona, E., Almenar, S., et al. (2008). Low levels of WWOX protein immunoexpression correlate with tumour grade and a less favourable outcome in patients with urinary bladder tumours. Histopathology 52 (7), 831–839. doi:10.1111/j.1365-2559.2008.03033.x
Rehmsmeier, M., Steffen, P., Hochsmann, M., and Giegerich, R. (2004). Fast and effective prediction of microRNA/target duplexes. RNA 10 (10), 1507–1517. doi:10.1261/rna.5248604
Saito, T., and Saetrom, P. (2010). MicroRNAs--targeting and target prediction. N. Biotechnol. 27 (3), 243–249. doi:10.1016/j.nbt.2010.02.016
Seila, A. C., Core, L. J., Lis, J. T., and Sharp, P. A. (2009). Divergent transcription: A new feature of active promoters. Cell. Cycle 8 (16), 2557–2564. doi:10.4161/cc.8.16.9305
Sherman, B. T., Hao, M., Qiu, J., Jiao, X., Baseler, M. W., Lane, H. C., et al. (2022). David: A web server for functional enrichment analysis and functional annotation of gene lists (2021 update). Nucleic Acids Res. 50 (W1), W216–W221. doi:10.1093/nar/gkac194
Song, Y., Nie, L., Wang, M., Liao, W., Huan, C., Jia, Z., et al. (2022). Differential expression of lncRNA-miRNA-mRNA and their related functional networks in new-onset type 2 diabetes mellitus among Chinese rural adults. Genes. (Basel) 13 (11), 2073. doi:10.3390/genes13112073
Taouis, K., Driouch, K., Lidereau, R., and Lallemand, F. (2021). Molecular functions of WWOX potentially involved in cancer development. Cells 10 (5), 1051. doi:10.3390/cells10051051
Thomas, P. D., Ebert, D., Muruganujan, A., Mushayahama, T., Albou, L. P., and Mi, H. (2022). Panther: Making genome-scale phylogenetics accessible to all. Protein Sci. 31 (1), 8–22. doi:10.1002/pro.4218
Wang, X. H., Gan, C. Z., and Xie, J. Y. (2018). Inhibition of miR-24 suppresses malignancy of human non-small cell lung cancer cells by targeting WWOX in vitro and in vivo. Thorac. Cancer 9 (12), 1583–1593. doi:10.1111/1759-7714.12824
Warman, E. A., Forrest, D., Guest, T., Haycocks, J., Wade, J. T., and Grainger, D. C. (2021). Widespread divergent transcription from bacterial and archaeal promoters is a consequence of DNA-sequence symmetry. Nat. Microbiol. 6 (6), 746–756. doi:10.1038/s41564-021-00898-9
Warwick, T., Seredinski, S., Krause, N. M., Bains, J. K., Althaus, L., Oo, J. A., et al. (2022). A universal model of RNA.DNA:DNA triplex formation accurately predicts genome-wide RNA-DNA interactions. Brief. Bioinform 23 (6), bbac445. doi:10.1093/bib/bbac445
Warwick, T., Brandes, R. P., and Leisegang, M. S. (2023). Computational methods to study DNA:DNA:RNA triplex formation by lncRNAs. Noncoding RNA 9 (1), 10. doi:10.3390/ncrna9010010
Wen, J., Xu, Z., Li, J., Zhang, Y., Fan, W., Wang, Y., et al. (2017). Decreased WWOX expression promotes angiogenesis in osteosarcoma. Oncotarget 8 (37), 60917–60932. doi:10.18632/oncotarget.17126
Wong, N., and Wang, X. (2015). miRDB: an online resource for microRNA target prediction and functional annotations. Nucleic Acids Res. 43, D146–D152. doi:10.1093/nar/gku1104
Xiang, Y., Tian, Q., Guan, L., and Niu, S. S. (2020). The dual role of miR-186 in cancers: Oncomir battling with tumor suppressor miRNA. Front. Oncol. 10, 233. doi:10.3389/fonc.2020.00233
Xu, F., Wang, B., Liu, M., Liu, T., and Zhang, R. (2019). A long non-coding RNA TSLD8 inhibits hepatocellular carcinoma by stabilizing WWOX. Biochem. Biophys. Res. Commun. 516 (2), 526–532. doi:10.1016/j.bbrc.2019.06.043
Xu, D., Liu, X., Wu, J., Wang, Y., Zhou, K., Chen, W., et al. (2020). LncRNA WWOX-AS1 sponges miR-20b-5p in hepatocellular carcinoma and represses its progression by upregulating WWOX. Cancer Biol. Ther. 21 (10), 927–936. doi:10.1080/15384047.2020.1806689
Xue, J., Jia, E., Ren, N., and Xin, H. (2021). Identification of prognostic miRNA biomarkers for esophageal cancer based on the cancer genome Atlas and gene expression Omnibus. Med. Baltim. 100 (7), e24832. doi:10.1097/MD.0000000000024832
Yevshin, I., Sharipov, R., Kolmykov, S., Kondrakhin, Y., and Kolpakov, F. (2019). Gtrd: A database on gene transcription regulation-2019 update. Nucleic Acids Res. 47 (D1), D100–D105. doi:10.1093/nar/gky1128
Zelazowski, M. J., Pluciennik, E., Pasz-Walczak, G., Potemski, P., Kordek, R., and Bednarek, A. K. (2011). WWOX expression in colorectal cancer--a real-time quantitative RT-PCR study. Tumour Biol. 32 (3), 551–560. doi:10.1007/s13277-010-0150-5
Zhang, Z. L., Bai, Z. H., Wang, X. B., Bai, L., Miao, F., and Pei, H. H. (2015). miR-186 and 326 predict the prognosis of pancreatic ductal adenocarcinoma and affect the proliferation and migration of cancer cells. PLoS One 10 (3), e0118814. doi:10.1371/journal.pone.0118814
Zhang, Y., Bu, D., Huo, P., Wang, Z., Rong, H., Li, Y., et al. (2021). ncFANs v2.0: an integrative platform for functional annotation of non-coding RNAs. Nucleic Acids Res. 49 (W1), W459–W468. doi:10.1093/nar/gkab435
Zhang, P., Wu, W., Chen, Q., and Chen, M. (2019). Non-coding RNAs and their integrated networks. J. Integr. Bioinform 16 (3), 20190027. doi:10.1515/jib-2019-0027
Zhang, W., Zhao, Z., and Li, F. (2022). Natural killer cell dysfunction in cancer and new strategies to utilize NK cell potential for cancer immunotherapy. Mol. Immunol. 144, 58–70. doi:10.1016/j.molimm.2022.02.015
Zhang, X., Zhong, B., Zhang, W., Wu, J., and Wang, Y. (2019). Circular RNA CircMTO1 inhibits proliferation of glioblastoma cells via miR-92/WWOX signaling pathway. Med. Sci. Monit. 25, 6454–6461. doi:10.12659/MSM.918676
Zhang, Y., Yin, C., Wei, C., Xia, S., Qiao, Z., Zhang, X. W., et al. (2022). Exosomal miR-625-3p secreted by cancer-associated fibroblasts in colorectal cancer promotes EMT and chemotherapeutic resistance by blocking the CELF2/WWOX pathway. Pharmacol. Res. 186, 106534. doi:10.1016/j.phrs.2022.106534
Keywords: WWOX, LINC01137, miR-186, long non-coding RNA, microRNA, cap analysis gene expression sequencing, bioinformatics, bladder cancer
Citation: Kołat D, Kałuzińska-Kołat Ż, Kośla K, Orzechowska M, Płuciennik E and Bednarek AK (2023) LINC01137/miR-186-5p/WWOX: a novel axis identified from WWOX-related RNA interactome in bladder cancer. Front. Genet. 14:1214968. doi: 10.3389/fgene.2023.1214968
Received: 30 April 2023; Accepted: 30 June 2023;
Published: 13 July 2023.
Edited by:
Federico Zambelli, University of Milan, ItalyReviewed by:
Xianglai Jiang, Ningxia Medical University, ChinaCopyright © 2023 Kołat, Kałuzińska-Kołat, Kośla, Orzechowska, Płuciennik and Bednarek. This is an open-access article distributed under the terms of the Creative Commons Attribution License (CC BY). The use, distribution or reproduction in other forums is permitted, provided the original author(s) and the copyright owner(s) are credited and that the original publication in this journal is cited, in accordance with accepted academic practice. No use, distribution or reproduction is permitted which does not comply with these terms.
*Correspondence: Damian Kołat, ZGFtaWFuLmtvbGF0QHVtZWQubG9kei5wbA==
Disclaimer: All claims expressed in this article are solely those of the authors and do not necessarily represent those of their affiliated organizations, or those of the publisher, the editors and the reviewers. Any product that may be evaluated in this article or claim that may be made by its manufacturer is not guaranteed or endorsed by the publisher.
Research integrity at Frontiers
Learn more about the work of our research integrity team to safeguard the quality of each article we publish.