- 1Program in Biology, Division of Science and Mathematics, New York University Abu Dhabi, Abu Dhabi, United Arab Emirates
- 2Centre National de Recherche et de Formation sur le Paludisme, Ouagadougou, Burkina Faso
The regulation of immune cell responses to infection is a complex process that involves various molecular mechanisms, including epigenetic regulation. DNA methylation has been shown to play central roles in regulating gene expression and modulating cell response during infection. However, the nature and extent to which DNA methylation is involved in the host immune response in human malaria remains largely unknown. Here, we present a longitudinal study investigating the temporal dynamics of genome-wide in vivo DNA methylation profiles using 189 MethylationEPIC 850 K profiles from 66 children in Burkina Faso, West Africa, sampled three times: before infection, during symptomatic parasitemia, and after malaria treatment. The results revealed major changes in the DNA methylation profiles of children in response to both Plasmodium falciparum infection and malaria treatment, with widespread hypomethylation of CpGs upon infection (82% of 6.8 K differentially methylated regions). We document a remarkable reversal of CpG methylation profiles upon treatment to pre-infection states. These changes implicate divergence in core immune processes, including the regulation of lymphocyte, neutrophil, and myeloid leukocyte function. Integrative DNA methylation-mRNA analysis of a top differentially methylated region overlapping the pro-inflammatory gene TNF implicates DNA methylation of TNF cis regulatory elements in the molecular mechanisms of TNF regulation in human malaria. Our results highlight a central role of epigenetic regulation in mounting the host immune response to P. falciparum infection and in response to malaria treatment.
1 Introduction
Despite the long history of malaria (Laveran, 1881; Poinar, 2005; Cox, 2010), the disease remains a major public health concern with an estimate of 619,000 deaths globally in 2021, 78.9% of which are children under the age of five in Sub-Saharan Africa (WHO, 2022). Human malaria is attributed to five species of Plasmodium, among which P. falciparum and P. vivax are the most studied due to their high prevalence in causing the disease (Perkins, 2014; Sato, 2021). Malaria has been challenging to eradicate due to the multi-layered host and parasite factors, their interaction with each other and with the environment (Idaghdour et al., 2012; Dieng et al., 2020). Host-parasite interactions often occur over relatively short evolutionary time scales, and it has been suggested that epigenetic and transcriptional mechanisms play important roles in these interactions in malaria (Gómez-Díaz et al., 2012; Idaghdour et al., 2012; Cheeseman and Weitzman, 2015). Understanding the nature and role of these molecular mechanisms in the context of how host immune cells respond to Plasmodium infection and to treatment with potential consequences on the course and outcome of infection is important to our overall understanding of how host–parasite interactions impact the pathology of the disease.
The role of epigenetic processes in complex diseases is becoming increasingly evident (Khyzha et al., 2017; Deng et al., 2019; Wang et al., 2019; Dobbs et al., 2022; Bouyahya et al., 2022; Ding et al., 2022; Kaimala et al., 2022). Epigenetic studies have begun to establish mechanistic links between epigenetic regulation and diseases susceptibility such as for rheumatoid arthritis (Liu et al., 2013) and type I diabetes (Rakyan et al., 2011) and also variation in immune-related gene expression traits (Lim et al., 2013; Obata et al., 2015), immune cell differentiation processes (Lai et al., 2013; Netea et al., 2015; Sellars et al., 2015; Rusek et al., 2018; Sun and Barreiro, 2020), immune memory genesis. For instance, Zhang and colleagues investigated the role of epigenetic mechanisms and DNA methylation in immune cell lineage differentiation and memory generation and reported specific DNA methylation patterns involved in the differentiation of memory cell subtypes with shared epigenetic regulation mechanisms across different immune cell lineages (Zhang et al., 2022). Despite the growing appreciation of the role of host epigenetics in the host immune response to pathogens such as Mycobacterium tuberculosis (Pennini et al., 2006; Gómez-Díaz et al., 2012; Cheeseman and Weitzman, 2015; Pacis et al., 2015), Leishmania donovani (Marr et al., 2014), and Toxoplasma gondii (Sabou et al., 2020), knowledge on the role of host epigenetic modifications in malaria is limited. Most malaria investigations published to date focused on the epigenetics of the parasite P. falciparum (Batugedara et al., 2017; Cortés and Deitsch, 2017; Gupta et al., 2017; Arama et al., 2018; Schrum et al., 2018). Investigations of host epigenetics in malaria were largely limited to candidate gene approaches including genes mediating host resistance/susceptibility (Stern et al., 2016; Lessard et al., 2017; Nisar et al., 2022), innate and adaptive immunity-related genes (Dobbs et al., 2020; Loiseau et al., 2020; Dobbs et al., 2022) and immune cell differentiation processes (Soon and Haque, 2018; Studniberg et al., 2022). Other human malaria studies have focused on the role of miRNAs in response to malaria (El-Assaad et al., 2011; Dkhil et al., 2017; Dieng et al., 2020; Gupta and Wassmer, 2021). We currently lack a comprehensive understanding of how P. falciparum infection impacts the host methylome during uncomplicated blood stage malaria.
In this study, we address this gap in knowledge by performing a longitudinal study to document and investigate the nature of genome-wide DNA methylation changes taking place in vivo in circulating immune cells of African children upon P. falciparum infection and treatment. We take advantage of a robust matched study design where the same group of children are sampled prior to infection, during symptomatic P. falciparum infection and 3 weeks after treatment. The results provided an unbiased view of the dynamics of methylation changes during the course of malaria infection and after treatment, identified the genomic regions impacted by these changes and highlighted epigenetic regulation as a major modulator of circulating immune cell function in human malaria.
2 Materials and methods
2.1 Study design and population
The study protocol and informed consent procedures were approved by the Institutional Review Board of New York University Abu Dhabi (UAE, protocol number 011–2015) and the Comité d’Ethique pour la Recherche en Santé au Burkina Faso (Ministry of Health, Burkina Faso; protocol number 2015-02-018). Assent for children older than 4 years of age and informed consent from parents or legal guardians of all study participants were obtained following the approved study protocol. Inclusion criteria are (i) residence in Bounouna and Nafona villages in the malaria-endemic Banfora district located 441 km from Ouagadougou the capital of Burkina Faso, West Africa, (ii) age between two and ten, (iii) no disease symptoms or chronic disease history (including sickle cell disease) based on medical records and physical assessment by a doctor at the CNRFP clinic in Banfora and (iv) no parasitemia at enrolment during the dry season (based on thick blood smear analysis and subsequently confirmed using RNASeq data). Transmission of malaria in Burkina Faso is markedly seasonal with a peak during the rainy season where around 60% of the total annual number of malaria episodes occurs (Tiono et al., 2013). Following enrollment, the study participants were followed on a weekly basis for up to 6 months. Phenotyping and sampling were done at three time points: Before Infection (BI), at the beginning of Symptomatic Parasitemia (SP), and 3 weeks After artemether/lumefantrine (Coartem) Treatment (AT) Figure 1A. In total, 150 children were recruited of which approximately 60 were carefully selected for the methylation study based on completeness of the data (Supplementary Data).
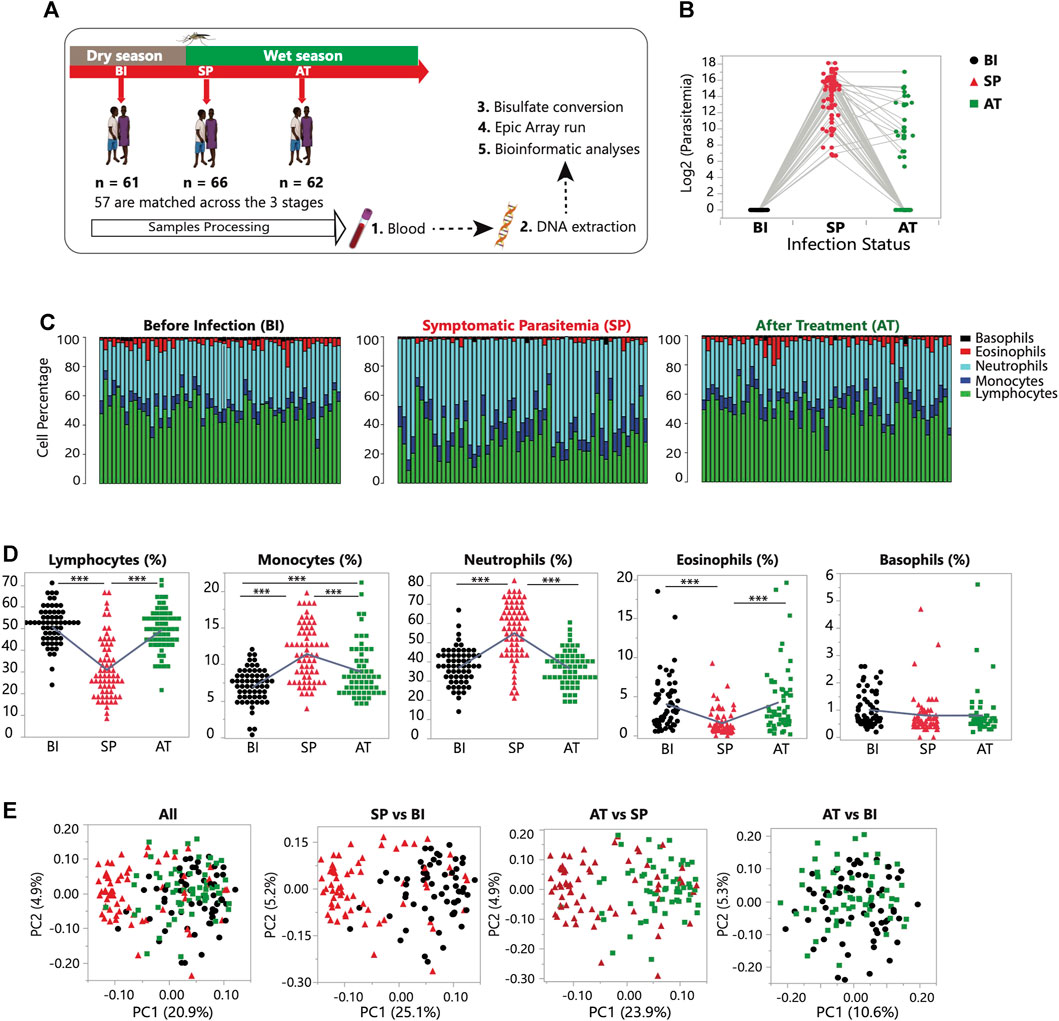
FIGURE 1. Study design and longitudinal dynamics of genome-wide DNA methylation profiles in malaria. (A) Study experimental design. The children were longitudinally sampled at three stages: Before Infection (BI, n = 61); Symptomatic Parasitemia (SP, n = 66) and After Treatment (AT, n = 62). (B) Parasitemia levels (log2) in the three stages profiled: Before Infection (n = 61, in black); Symptomatic Parasitemia (n = 66, in red) and After Treatment (n = 62, in green). The grey lines connect the matched samples. (C) Changes in the proportion of five white blood cell types during the course of the three stages sampled. Each bar represents one individual and the proportion of each cell type is shown for each stage. White blood cell count data was generated using the hematology analyzer Pentra C200 (HORIBA). (D) The percentage (%) of white blood cells (WBCs), including of lymphocytes, monocytes, neutrophils, eosinophils, and basophils, is plotted for each study participant in three groups: BI (n = 61), SP (n = 66), and AT (n = 62). Statistical significance for the mean difference between all pairs was tested using Tukey-Kramer HSD. The sign (***) indicates a p-value of <0.001. (E) Principal component analysis (PCA) of the DNA methylome for the three stages combined and for each pairwise comparison (SP vs. BI; SP vs. AT and AT vs. BI). Color and shape of the data point is referring to its infection status; BI = green square, SP = red triangle, and AT = black circle.
2.2 Blood samples and data collection
Blood samples (∼10 ml) and patient data were collected at the CNRFP clinic in Banfora. To minimize heterogeneity due to the circadian rhythm and technical reasons, blood samples were collected between 9:00 a.m. and 2:00 p.m. and processed immediately after collection following the same protocols throughout the various downstream experiments. One ml of blood was collected for DNA isolation. The remaining blood was used for standard thick blood smear analysis and to generate hemoglobin level and total cell count data including lymphocytes, neutrophils, monocytes, eosinophils and basophils. White blood cell count data was generated using the hematology analyzer Pentra C200 (HORIBA). Hemoglobin and G6PD genotypes were determined as previously described (Bougouma et al., 2012). Parasitaemia was calculated using manual counting of Giemsa-stained thin and thick blood smears and bright-field microscopy. Other covariates of interest such as age, sex and location were collected from all participants. Participant demography, biochemical and cell count data are summarized in Supplementary Data and Supplementary Table S1.
2.3 DNA isolation and methylation profiling
Genomic DNA was extracted from whole blood using Qiagen DNAeasy Blood and Tissue Kit (Qiagen) following the manufacturer’s instructions. DNA quality and concentration were checked using nanodrop and qubit instruments, respectively. A total of 50 ng of genomic DNA was used for genome-wide DNA methylation profiling at the Australian Research Genomics Facilities (ARGF, Melbourne) using Illumina’s Infinium MethylationEPIC (HM850 K) platform. Briefly, genomic DNA was bisulfite-converted using the EZ DNA Methylation kit (Zymo) then assayed by the Illumina Infinium MethylationEPIC BeadChip array that interrogates over 850,000 CpG methylation sites. Quality of the bead chip array was assayed by sample-independent and dependent controls for staining, extension, target removal, hybridization, bisulfite conversion, allele-specific extension and primer extension.
2.4 Data processing and quality control
Raw methylation intensities were processed in R (version 3.5.1) using the R/Bioconductor package minfi (Aryee et al., 2014). For each sample, the estimated methylation level at each CpG site is expressed as a beta value (β), which is the ratio of the methylated probe intensity to the overall intensity [i.e., calculated using the following formula C/(C + T +100), where C and T are the intensities of methylated and unmethylated signals, respectively (Pidsley et al., 2016)]. The produced β values were then adjusted using the 500-cell type-informative probes reported by Houseman et al. (2012) to account for differences in methylation arising from differences in cell type composition in whole-blood samples. β values were adjusted by using penalized regression to regress out the cell types using the function “adjust.beta” following the Houseman et al. (2012) procedure. The new adjusted β values were then logit transformed to the adjusted M values that were subsequently used for the statistical analyses. WBC composition data measured in the lab was also directly used as covariates to account for cell composition and the results were similar to those of the Houseman et al. (2012) method.
To reduce the non-biological differences between probes and to adjust for the different probe design types present in the 850 K architecture, the intensities of the signal were corrected for background fluorescence and technical variations using functional “funnorm” normalization implemented in minfi for both adjusted β and adjusted M values (Supplementary Figure S1). Then, stringent filtering was applied to filter out probes based on the following criteria: (i) probes overlapping single nucleotide polymorphism (SNP) with minor allele frequency (MAF) > 0.05 in 1 K Genome data (using the “dropLociWithSnps” function), (ii) probes on X and Y chromosomes, and (iii) cross-reactive probes as recommended and listed by Pidsley et al. (2016). This filtering resulted in a total of 794,698 CpG sites retained for downstream analyses.
2.5 DNA methylation data analysis
Methylation distribution data analysis and principal component analysis (PCA) were done on the full 794,698 CpG loci dataset using JMP Genomics (SAS Institute). Longitudinal changes in DNA methylation for the 794,698 CpG loci were assessed using DMRcate (Peters et al., 2015) for each infection status contrast: Before Infection vs. Symptomatic Parasitemia, Symptomatic Parasitemia vs. After Treatment, and After Treatment vs. Before Infection using the following linear mixed effect model; (∼Infection_Status + Age + Sex + Individual) (Supplementary Data). Age and sex effects are expected to be marginal given the matched study design nonetheless they were included in the model as covariates. Given the longitudinal nature of the data (i.e., repeated measures), the “Individual” effect was accounted for by including it in the analysis as a blocked parameter. The run settings for DMRcate were adjusted based on Mallik et al. (2019) which showed that power and precision of the DMRcate run were best achieved when lambda was set to 500, and parameter C to 5. The p-value cutoff measure for the identification of differentially methylated probes and regions was set to 0.001. Results were then corrected for multiple testing using the Benjamini–Hochberg method. The annotations of the methylation probes used in the analyses were obtained from the R/Bioconductor package IlluminaHumanMethylationEPICanno.ilm10b4. hg19 (package version 3.7). Visualizations of the temporal and spatial changes in DMPs and DMRs were plotted using the ggplot2 R package (version 3.4.0). DNA methylation age was estimated using the epigenetic clock model developed by Horvath et al. (2018) and implemented in the methyAge package in R 4.2.2 (R Core Team). The model uses 391 CpGs measured from six different tissues including whole blood from participants of a wide age range 0–94 years (Horvath et al., 2018). The model was chosen since our data was generated from children (median 4 years old; range; 2–9.1 years old) and the model has demonstrated better performance with children data (Wu et al., 2019; Kling et al., 2020; McEwen et al., 2020; Monasso et al., 2021). The model generated methylation age and acceleration values that were compared between the three infection stages investigated using ANOVA and Tukey’s HSD test.
2.6 Gene set enrichment analysis
We identified gene ontology (GO) cellular component, molecular functions and biological processes pathways that were significantly enriched for DMRs at cut-off p-value <0.01 using the gometh function of the missMethyl R package (version 1.10.0 gometh function) (Phipson et al., 2016). The gometh function included an adjustment for the underlying distribution of probes on the array to remove bias due to unbalanced distribution of probes per gene interrogated in the array. Cluego (Lee et al., 2005), available through the program Cytoscape (version 3.71) (Shannon et al., 2003), was then used to quantify enrichment scores and significance.
2.7 Correlation between TNF expression and methylation level
The expression levels of TNF (IQR normalized) of 40 children sampled at three time points, Before Infection, during Symptomatic Parasitemia and After Treatment were obtained from an RNA-seq dataset (Dieng et al., 2020; Abdrabou et al., 2021) generated from the same blood samples as the methylation dataset. This matched dataset provided an opportunity to assess the association between methylation and expression level of TNF. Normalized TNF levels of 40 children were correlated (Pearson method) with their matched adjusted beta values of 13 CpGs averaged located on the DMR that overlaps with the TNF gene locus. Expression-methylation correlations were tested separately for each of the three infection stage groups.
3 Results
Over a 3-month period, we recruited and sampled longitudinally a pediatric cohort of children in Banfora district in Burkina Faso. Phenotypic, clinical data and blood specimens were collected at three time points: Before Infection (BI), during Symptomatic Parasitemia (SP) and 3 weeks After Treatment (AT) Figures 1A, B. White blood cells (WBCs) are a central component of the immune system and their composition and response to the infection can modulate the course of the disease. Proportions of the five major WBCs (lymphocytes, monocytes, neutrophils, basophils, and eosinophils) were analyzed to assess the nature of changes taking place in response to P. falciparum infection and treatment (Figures 1C, D). Proportions of each cell type were compared between the three stages (Figure 1C). As the children transition to the SP stage, significant differences in the counts of monocytes, lymphocytes, neutrophils and eosinophils (p < 0.001) were observed. In particular, we highlight the significant depletion of the proportion of lymphocytes during the SP stage, and the increase in the proportions of monocytes and neutrophils (Figures 1C, D; Supplementary Table S2). In response to treatment, significant changes in cell proportions are observed again but in the opposite direction to the response to the infection (Figure 1D). Comparing the Before Infection (BI) and After Treatment (AT) groups shows that in general after treatment the children recover their pre-infection WBCs proportions with no significant differences observed.
3.1 Longitudinal dynamics of methylation profiles throughout the course of infection
To infer the correlation structure in the data, we performed principal component analysis (PCA) using the entire DNA methylation dataset that consists of normalized 794,698 CpG sites methylation levels from 189 samples representing the three infection stages (BI, SP and AT) (Supplementary Figure S1, S2). The first methylation principal component (PC1), indicating the variation and degree of differentiation among the three infection stages, explains ∼20% of the total variance and clearly captures the effect of P. falciparum infection on host DNA methylation profiles (Figure 1E; Supplementary Figure S2). PCA of pair groups captured the gradual temporal changes of P. falciparum infection (Figure 1E). Variance component analysis shows that infection status accounts for 41.8% of the total variance in PC1.
3.1.1 Differentially methylated positions (DMPs)
To evaluate the genome-wide DNA methylation differences and establish the direction of the changes in whole blood DNA during the course of P. falciparum infection, we performed differential methylation analyses on the 794,698 CpG sites in 189 samples. We used a mixed regression model that accounts for age, sex, and the individual effect (see Methods for details) to identify differentially methylated positions (DMPs) and regions (DMRs). We identified ∼79.9 K (10%), ∼73.2 K (9%) and 9 K (1%) DMPs passing the experiment-wide significance threshold (FDR <0.01) between the contrasts Symptomatic Parasitemia (SP) vs Before Infection (BI), After treatment (AT) vs Symptomatic Parasitemia (SP) and After Treatment (AT) vs Before Infection (BI), respectively, (Table 1). The temporal dynamics of DNA methylation profiles are shown in Figure 2. We note that 18 children did not clear the parasites from their blood after treatment. Differential methylation analysis between this group and the children who cleared the parasite revealed no differentially methylated CpGs (FDR <0.01) and only 11 CpGs at FDR <0.05 (Supplementary Figure S3).
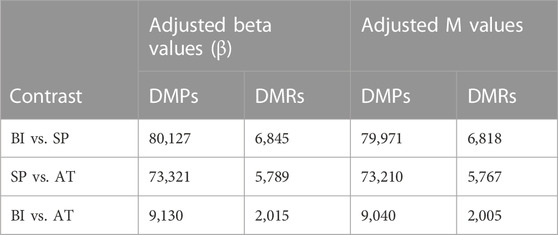
TABLE 1. Number of differentially methylated positions (DMPs) and regions (DMRs) for both adjusted beta and M values obtained using DMRcate.
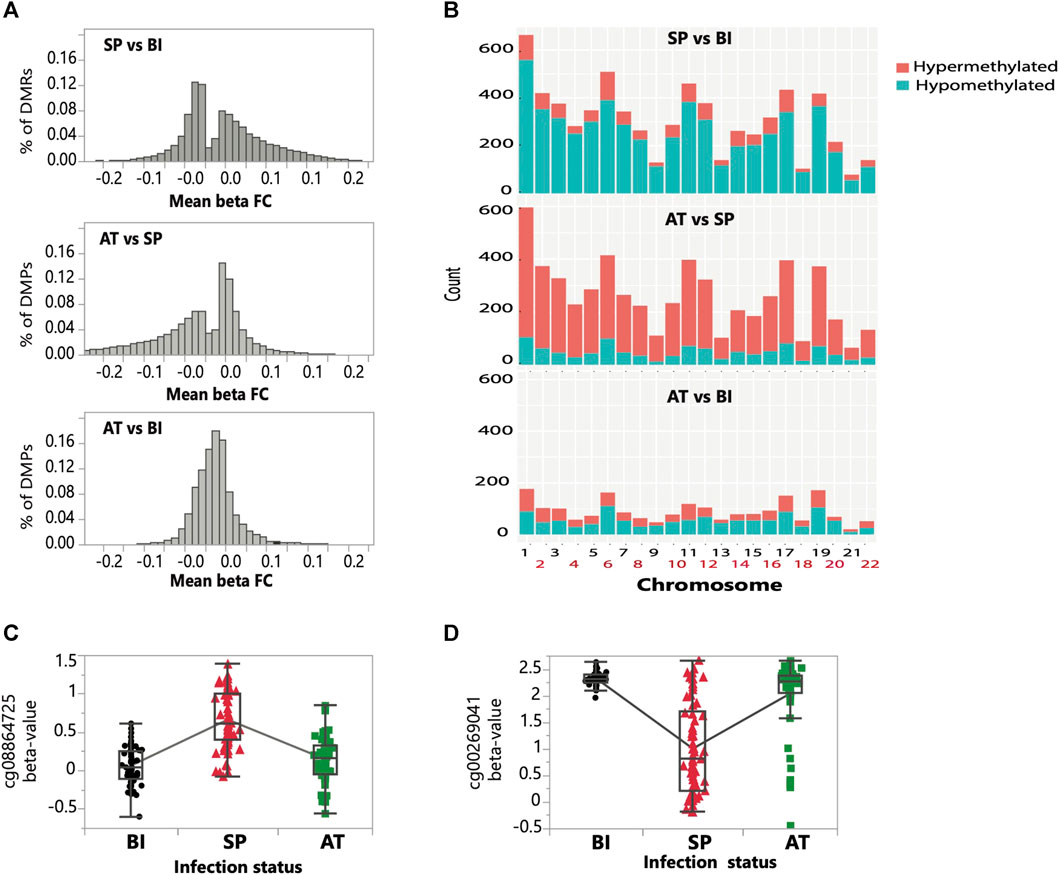
FIGURE 2. Genome-wide distribution of differentially methylated positions and regions throughout the three infection stages. (A) Distribution of hypermethylated (greater than zero) and hypomethylated (lower than zero) differentially methylated positions (DMPs) for the three pairwise comparisons (SP vs. BI; SP vs. AT, and BI vs. AT). (B) Chromosomal distribution of the counts of hypermethylated and hypomethylated differentially methylated regions (DMRs) for the three pairwise comparisons (BI vs. SP, SP vs. AT, and BI vs. AT). The bar height reflects the density of DMRs for each chromosome. The blue and red colors on the bars represent the counts of hypo- and hyper-methylated DMRs within the chromosome, respectively. (C, D) Examples of temporal changes in methylation of two randomly selected CpGs of opposite trend. (C) a CpG which has an increase in methylation in SP compared to BI and AT stages, and (D) a CpG which has an decrease in methylation in SP compared to BI and AT stages.
Approximately three-quarters (59.2 K) of the total identified DMPs (i.e., ∼79.9 K) were shared by two pairwise comparisons (Symptomatic Parasitemia vs. Before Infection and After treatment vs. Symptomatic Parasitemia) and less than 1% (El-Assaad et al., 2011) were shared by the three groups (Supplementary Figure S4A). Through examining the methylation status and trend of DMPs (hypo vs. hyper methylation) between pairwise comparisons (Symptomatic Parasitemia vs. Before Infection and After Treatment vs. Symptomatic Parasitemia), we observed a switch from an overly hypomethylation state between the Symptomatic Parasitemia vs. Before Infection pairwise comparison, to an overly hypermethylation state between the After Treatment vs. Symptomatic Parasitemia pairwise comparison (Figure 2A). This was additionally highlighted by the Venn diagram which revealed significant overlaps of DMPs shifting from hypo to hyper-methylated states when comparing the Symptomatic Parasitemia vs. Before Infection and After Treatment vs. Symptomatic Parasitemia pairwise groups (Supplementary Figure S4B, C). This finding clearly shows reversal of the direction of methylation change following malaria treatments. This is consistent with the observation that the overall methylation state of children before infection and after treatment is similar (Figure 1E; Figure 2A). The number and magnitude of changes observed between states (Before Infection to Symptomatic Parasitemia and Symptomatic Parasitemia to After Treatment) were similar and largely correspond of reversal of methylation states which resulted in limited differences between After Treatment and Before Infection states (Figure 2A). Examples of these patterns of methylation reversal are shown for two CpG sites in Figures 2C, D.
3.1.2 Differentially methylated regions (DMRs)
Expending the differential methylation analysis from CpG sites to DMRs, we identified ∼6.8K, ∼5.7K, and ∼ 2 K DMRs passing the experiment-wide significance threshold (FDR <0.01) between Symptomatic Parasitemia (SP) vs. Before Infection (BI), After Treatment (AT) vs. SP and AT vs. BI, respectively (Table 1). The temporal dynamic trend of methylation profiles of DMRs shows a similar pattern to that of DMPs (Figure 2B). While around 82% (5.6 K) of DMRs during infection (from Before Infection to Symptomatic Parasitemia state) were hypomethylated, we observed approximately 80% (4.6 K) of DMRs to be hypermethylated after treatment (from Symptomatic Parasitemia to After Treatment states). This finding confirms the inferred restoration of the pre-infection DNA methylation states. This was further confirmed when After Treatment and Before Infection methylation states were compared and showed low levels of methylation changes between the two states (Figure 2B). Plotting the density of DMRs across chromosomes for each of the three pairwise comparisons (SP vs. BI, AT vs. SP and AT vs. BI) while accounting for the CpG probe content in the EPIC array showed that the distribution of DMRs across chromosomes is not random with most changes taking place in chromosomes 1, 6, 11, 17, and 19 (Supplementary Figure S5). We highlight in particular the case of chromosome 6 that harbors the HLA region being one of the most impacted by methylation changes in our dataset. Collectively, our DMPs and DMRs analyses show that P. falciparum infection and malaria treatment have a significant impact on the DNA methylation states of children. Particularly noteworthy is the finding that the majority of global methylation changes occurring during the acute phase of the disease (Symptomatic Parasitemia) revert back to their pre-infection (Before Infection) states after treatment.
Next, to assess the effect of Plasmodium infection and malaria treatment on epigenetic age, DNA methylation age was calculated using the estimator developed by Horvath et al. (2018). The model performed well with correlations between DNA methylation age and chronological age >0.8 (p < 0.0001) in all three stages of the infection (Supplementary Figure S6A). The results show no statistically significant differences in group’s epigenetic age and acceleration means (Supplementary Figures S6B, C).
3.2 Gene set enrichment analysis
Gene set enrichment analysis of differentially methylated regions revealed the impact of the methylation changes observed on immunological pathways in particular immunoglobulin production, leukocytes activation, myeloid leukocyte activation, neutrophil degranulation and T cell activation (Figure 3). Interestingly, the same pathways impacted upon infection were impacted in response to treatment which aligns with the DNA methylation reversal trend observed in our quantitative temporal dynamic analysis of DMPs and DMRs. Collectively, differential methylation and enrichment analyses show that global methylation patterns that are disrupted in response to P. falciparum infection are restored quantitatively and qualitatively leading to the restoration of the pre-infection functional and immunological states following malaria treatment.
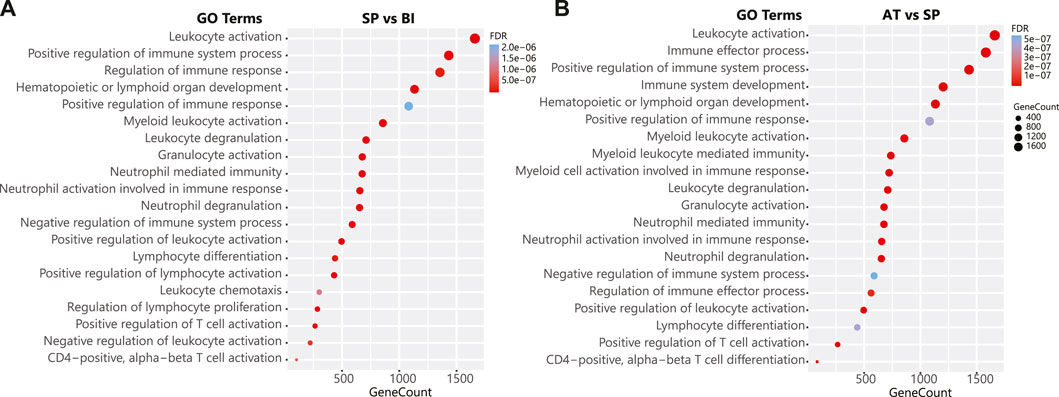
FIGURE 3. Pathway enrichment of differential immunological responses to malaria infection. (A) The top 20 statistically significant (FDR <0.01) pathways enriched for the contrast Symptomatic Parasitemia (SP) vs. Before Infection (BI). (B) The top 20 statistically significant (FDR <0.01) pathways enriched for the contrast After treatment (AT) vs. Symptomatic Parasitemia (SP). The y-axis indicates the pathway name, x-axis and the bubble size indicate the number of genes in each pathway. The color bar indicates the corrected FDR p-value, blue represents higher values (less significant), red represents lower values (more significant). Pathways are ranked based on their gene count. Pathway enrichment analysis was done using the gometh function of the missMethyl R package (version 1.10.0 gometh function). Grouping pathways to a non-redundant list was performed using ClueGO. For the full list of the statistically significant pathways see Supplementary Tables S1H, I.
3.3 Correlation between TNF expression and methylation levels of associated CpGs
To gain insights into the effects of global methylation on gene expression regulation during P. falciparum malaria, we performed a focused analysis of the top most significant perturbation observed on chromosome 6 which implicates the TNF locus (Figure 4A). Allelic variation in this locus and closeby genes have been associated with severe outcomes following Plasmodium infection in multiple populations (Kwiatkowski, 1990; McGuire et al., 1994; Clark Taane et al., 2009). The DMR identified in our analysis is located right upstream of the TNF locus and contains 13 CpGs (Figure 4A). The 13 CpG sites show consistent hypomethylation in Symptomatic Parasitemia and a remarkable reversion of methylation state after malaria treatment (Figures 4A, B). We observed changes of TNF transcript abundance that are consistent with a model of local methylation regulation of TNF gene expression where hypomethylation in Symptomatic Parasitemia results in upregulation of TNF expression that then drops to pre-infection levels as the CpG site regain their methylation marks (Figure 4B). To test if methylation levels within each infection stage correlate with variation of TNF expression, we performed correlation analysis between the adjusted beta values of the 13 CpGs averaged and TNF expression levels in the three stages separately: Before Infection (n = 40), during Symptomatic Parasitemia infection (n = 40), and After Treatment (n = 40). The results show a significant negative correlation in the Symptomatic Parasitemia (p-value = 0.002) and After Treatment (p-value = 0.025) groups. In the Before Infection group, the trend was negative but not statistically significant (Figure 4C). Combined, these results support the hypothesis that the dynamic methylation changes taking place in the DMR result in changes in TNF gene expression levels that increase during symptomatic parasitemia and revert back to pre-infection levels. These results implicate methylation in the molecular mechanisms of TNF regulation in human malaria.
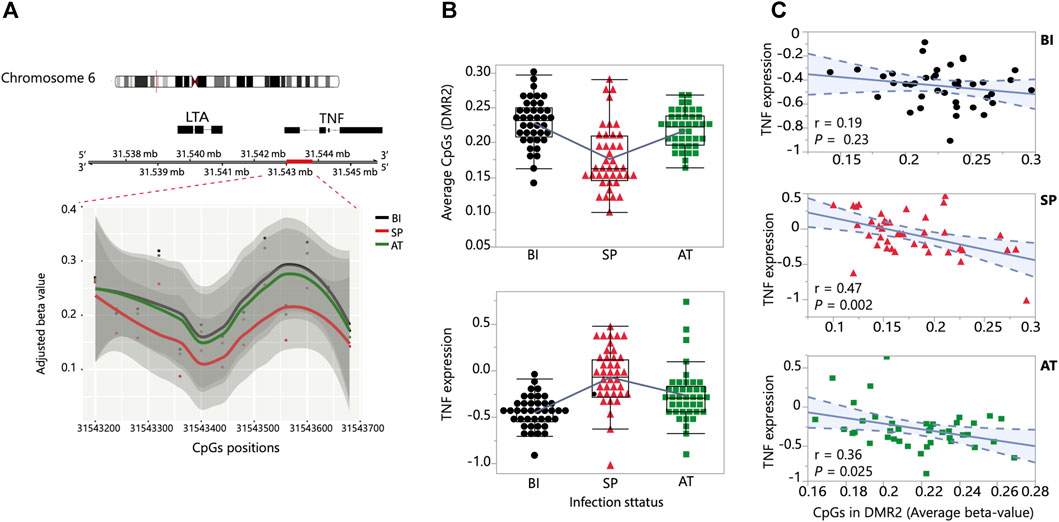
FIGURE 4. DNA methylation association with TNF expression. (A) A TNF-associated DMR (red bar) on chromosome 6 showing the temporal change in methylation state. The y-axis and x-axis show the adjusted beta-values and the positions of the CpGs in the DMR, respectively. (B) Temporal changes in methylation (top) and TNF expression (bottom) of the 40 children sampled at the three stages: Before Infection, Symptomatic Parasitemia and After Treatment (BI, SP and AT). (C) Correlation plots of the association between the CpGs adjusted beta values and level of TNF expression at the three stages: Before Infection (BI, black), Symptomatic Parasitemia (SP, red) and After Treatment (AT, green).
4 Discussion
Methylation of DNA plays a central role in gene regulation by controlling the accessibility of DNA to transcription factors and RNA polymerase. DNA methylation is a relatively stable epigenetic mark (Kim and Costello, 2017). However, under certain cellular environments (i.e., innate immune cells facing a parasitic infection), DNA methylation levels can change rapidly (Pacis et al., 2019). Although changes of DNA methylation in response to infection has received increased attention in the past years (Poulin and Thomas, 2008; Gómez-Díaz et al., 2012; Wenzel and Piertney, 2014; Deng et al., 2019; Dobbs et al., 2022; Bouyahya et al., 2022; Ding et al., 2022; Kaimala et al., 2022), little is known about the epigenetic response taking place in response to the malarial parasite P. falciparum. In this study, we generated the first matched genome-wide DNA methylation data at three time points in malaria. To our knowledge, this is the first report documenting host temporal DNA methylome changes in malaria.
Both supervised and unsupervised analysis of methylation data demonstrate significant changes of circulating immune cell methylation profiles. We document these major changes at DMP and DMR scales. During the symptomatic parasitemia stage, most of the DMPs and DMRs are hypomethylated. The relationship between DNA methylation and gene expression is complex but in general, hypomethylation is associated with increased transcription levels (Feinberg and Tycko, 2004; Nishigaki et al., 2005; Jones, 2012). Given the need for a rapid response of circulating immune cells to Plasmodium infection, the observed global hypomethylation response is expected and results in global transcriptional responses as previously reported in malaria (Idaghdour et al., 2012; Dieng et al., 2020). General hypomethylation was also reported to affect intergenic regions of DNA, including transposable elements, which can lead to changes in chromosomal stability (Wöhrle et al., 2001; Nichol and Pearson, 2002). Our results are consistent with other studies that have reported frequent occurrence of both global and localized DNA hypomethylation across a range of diseases including various types of cancers such as pancreas cancers (Sato et al., 2003), colon cancers (Frigola et al., 2005), breast cancer (Piotrowski et al., 2006), and neoplasia (Santini et al., 2001). Additionally, hypomethylation has been observed in other conditions such as Crohn’s disease (Somineni et al., 2019) and bacterial infection of human dendritic cells (Pacis et al., 2015). The methylation profile of circulating immune cells has undergone a significant reversal to the largely hypermethylated pre-infection state following treatment, with the majority of the DMPs and DMRs becoming hypermethylated. A study on pediatric patients with Crohn’s disease found similar DNA methylation patterns in blood samples, including changes in CpG methylation that disappeared with treatment of inflammation (Somineni et al., 2019). That said, a number of DMPs and DMRs are exceptions to this trend and suggest that P. falciparum infection and/or drug treatment leave an epigenetic footprint in the genomes of circulating immune cells. Regions that did not revert back may act as informed enhancers, potentially allowing for faster transcriptional responses to future infections. We speculate that the observed dynamic methylation changes in response to infection and treatment leave epigenetic signatures that could impact the host response to future infections.
The spatial density of DMPs and DMRs, accounting for the distribution of CpG sites on the EPIC array, revealed that chromosomes 1, 6, 11, 17, and 19 are the most subject to these changes. These chromosomes have the highest densities of immune genes indicating the occurrence of major epigenetic regulation of immune response. The majority of DMRs overlapped with gene bodies and promoters of master immune genes (i.e., HLA-DQB1, HLA-A, HHLA2, CD1C, IFNA1, IFNK, LY9, TNFSF18 and others). Notably and as anticipated, the highest number of DMRs implicating immune genes was observed during the symptomatic parasitemia phase, reflecting a systematic epigenetically-regulated transcriptional response to P. falciparum infection. Enrichment analysis of DMRs implicated several core immunological pathways that are crucial for the activation and regulation of immune response against malaria infection. The top impacted processes include immunoglobulin production, neutrophil degranulation, T cell activation and myeloid leukocyte differentiation, migration and chemotaxis. These processes play important roles in the pathogenesis of malaria (Sun and Chakrabarti, 1985; Tecchio and Cassatella, 2016; Aitken et al., 2018; Silveira et al., 2018) and potentially in the development of protective immunity (Uchechukwu et al., 2017). It is worth noting that the global methylation changes impacted non-immune system-related genes supporting the view that parasite infection affects various cellular processes beyond strict immune responses (Wenzel and Piertney, 2014).
Changes in DNA methylation profiles of cis and trans regulatory elements are predicted to result in changes of gene expression levels. Our TNF-focused integrative CpG methylation-TNF mRNA analysis implicates methylation in the molecular mechanisms of TNF regulation in human malaria. TNF is a vital component of the innate and adaptive immune systems and has been linked to the recruitment and enhancement of monocytes, neutrophils, and lymphocytes during inflammation triggered by malaria infection (Mackay et al., 1993; Pasparakis et al., 1996; Dobbs et al., 2022). Several previous studies have reported TNF cis regulatory allelic variation as well as altered levels of TNF expression associated with severe malaria infection (Henrici et al., 2021; Mahittikorn et al., 2022). Our integrative analysis identified the hypomethylated CpG sites that are most likely mediating the observed upregulation of TNF expression in response to P. falciparum expression. Hypermethylation of the same CpG sites is likely the mediator of downregulation of TNF expression observed following treatment. The matched nature of both our transcriptomic and methylation datasets enabled us to draw these inferences on the impact of DNA methylation changes on TNF gene expression.
In summary, we present the first genome-wide matched DNA methylation profiles of malarial children before infection, during symptomatic parasitemia and after treatment and provide evidence of widespread impact of P. falciparum on DNA methylation of circulating immune cells. Knowledge of epigenetic regulation of the human host to P. falciparum infection adds another layer of complexity to host-parasite interactions but offers also new insights into potential novel therapeutic approaches in malaria as has been done in other diseases.
Data availability statement
The 850k EPIC DNA methylation data is deposited in GEO under the following ID: GSE230850. URL: https://www.ncbi.nlm.nih.gov/geo/query/acc.cgi?acc=GSE230850.
Ethics statement
The studies involving human participants were reviewed and approved by the Board of New York University Abu Dhabi (UAE, protocol number 011-2015) and the Comité d’Ethique pour la Recherche en Santé au Burkina Faso (Ministry of Health, Burkina Faso; protocol number 2015-02-018). Written informed consent to participate in this study was provided by the participants’ legal guardian/next of kin.
Author contributions
Conceptualization: YI, AD, and DA. Methodology: AD, IS, MMD, SSS, SS, AmD, AB, SC, and SBS. Formal analysis: DA, AD, VM, and MMD. Writing—Original draft preparation: DA, AD. Writing—Review and editing: YI, AD, DA, and MMD. Supervision: IS, SBS, and YI. Project administration: IS and YI. Funding acquisition: YI. All authors contributed to the article and approved the submitted version.
Funding
This work was funded by New York University Abu Dhabi Grant AD105 to YI.
Acknowledgments
We would like to thank the children who participated in the study and their families and all the individuals who facilitated sample collection and clinical work in Burkina Faso.
Conflict of interest
The authors declare that the research was conducted in the absence of any commercial or financial relationships that could be construed as a potential conflict of interest.
Publisher’s note
All claims expressed in this article are solely those of the authors and do not necessarily represent those of their affiliated organizations, or those of the publisher, the editors and the reviewers. Any product that may be evaluated in this article, or claim that may be made by its manufacturer, is not guaranteed or endorsed by the publisher.
Supplementary material
The Supplementary Material for this article can be found online at: https://www.frontiersin.org/articles/10.3389/fgene.2023.1197933/full#supplementary-material
References
Abdrabou, W., Dieng, M. M., Diawara, A., Sermé, S. S., Almojil, D., Sombié, S., et al. (2021). Metabolome modulation of the host adaptive immunity in human malaria. Nat. Metab. 3 (7), 1001–1016. doi:10.1038/s42255-021-00404-9
Aitken, E. H., Alemu, A., and Rogerson, S. J. (2018). Neutrophils and malaria. Front. Immunol. 9, 3005. doi:10.3389/fimmu.2018.03005
Arama, C., Quin, J. E., Kouriba, B., Östlund Farrants, A-K., Troye-Blomberg, M., and Doumbo, O. K. (2018). Epigenetics and malaria susceptibility/protection: A missing piece of the puzzle. Front. Immunol. 9, 1733. doi:10.3389/fimmu.2018.01733
Aryee, M. J., Jaffe, A. E., Corrada-Bravo, H., Ladd-Acosta, C., Feinberg, A. P., Hansen, K. D., et al. (2014). Minfi: A flexible and comprehensive bioconductor package for the analysis of Infinium DNA methylation microarrays. Bioinformatics 30 (10), 1363–1369. doi:10.1093/bioinformatics/btu049
Batugedara, G., Lu, X. M., Bunnik, E. M., and Le Roch, K. G. (2017). The role of chromatin structure in gene regulation of the human malaria parasite. Trends Parasitol. 33 (5), 364–377. doi:10.1016/j.pt.2016.12.004
Bougouma, E. C., Tiono, A. B., Ouédraogo, A., Soulama, I., Diarra, A., Yaro, J-B., et al. (2012). Haemoglobin variants and Plasmodium falciparum malaria in children under five years of age living in a high and seasonal malaria transmission area of Burkina Faso. Malar. J. 11 (1), 154. doi:10.1186/1475-2875-11-154
Bouyahya, A., Mechchate, H., Oumeslakht, L., Zeouk, I., Aboulaghras, S., Balahbib, A., et al. (2022). The role of epigenetic modifications in human cancers and the use of natural compounds as epidrugs: Mechanistic pathways and pharmacodynamic actions. Biomolecules 12 (3), 367. doi:10.3390/biom12030367
Cheeseman, K., and Weitzman, J. B. (2015). Host–parasite interactions: An intimate epigenetic relationship. Cell. Microbiol. 17 (8), 1121–1132. doi:10.1111/cmi.12471
Clark Taane, G., Diakite, M., Auburn, S., Campino, S., Fry Andrew, E., Green, A., et al. (2009). Tumor necrosis factor and lymphotoxin-alpha polymorphisms and severe malaria in African populations. J. Infect. Dis. 199 (4), 569–575. doi:10.1086/596320
Cortés, A., and Deitsch, K. W. (2017). Malaria epigenetics. Cold Spring Harb. Perspect. Med. 7 (7), a025528. doi:10.1101/cshperspect.a025528
Cox, F. E. (2010). History of the discovery of the malaria parasites and their vectors. Parasit. Vectors 3 (1), 5. doi:10.1186/1756-3305-3-5
Deng, Q., Luo, Y., Chang, C., Wu, H., Ding, Y., and Xiao, R. (2019). The emerging epigenetic role of CD8+T cells in autoimmune diseases: A systematic review. Front. Immunol. 10, 856. doi:10.3389/fimmu.2019.00856
Dieng, M. M., Diawara, A., Manikandan, V., Tamim El Jarkass, H., Sermé, S. S., Sombié, S., et al. (2020). Integrative genomic analysis reveals mechanisms of immune evasion in P. falciparum malaria. Nat. Commun. 11 (1), 5093. doi:10.1038/s41467-020-18915-6
Ding, Q., Gao, Z., Chen, K., Zhang, Q., Hu, S., and Zhao, L. (2022). Inflammation-related epigenetic modification: The bridge between immune and metabolism in type 2 diabetes. Front. Immunol. 13, 883410. doi:10.3389/fimmu.2022.883410
Dkhil, M. A., Al-Quraishy, S. A., Abdel-Baki, A-A. S., Delic, D., and Wunderlich, F. (2017). Differential miRNA expression in the liver of balb/c mice protected by vaccination during crisis of Plasmodium chabaudi blood-stage malaria. Front. Microbiol. 7, 2155. doi:10.3389/fmicb.2016.02155
Dobbs, K. R., Crabtree, J. N., and Dent, A. E. (2020). Innate immunity to malaria—the role of monocytes. Immunol. Rev. 293 (1), 8–24. doi:10.1111/imr.12830
Dobbs, K. R., Dent, A. E., Embury, P., Ogolla, S., Koech, E., Midem, D., et al. (2022). Monocyte epigenetics and innate immunity to malaria: Yet another level of complexity? Int. J. Parasitol. 52 (11), 717–720. doi:10.1016/j.ijpara.2022.07.001
El-Assaad, F., Hempel, C., Combes, V., Mitchell, A. J., Ball, H. J., Kurtzhals, J. A. L., et al. (2011). Differential MicroRNA expression in experimental cerebral and noncerebral malaria. Infect. Immun. 79 (6), 2379–2384. doi:10.1128/IAI.01136-10
Feinberg, A. P., and Tycko, B. (2004). The history of cancer epigenetics. Nat. Rev. Cancer 4 (2), 143–153. doi:10.1038/nrc1279
Frigola, J., Solé, X., Paz, M. F., Moreno, V., Esteller, M., Capellà, G., et al. (2005). Differential DNA hypermethylation and hypomethylation signatures in colorectal cancer. Hum. Mol. Genet. 14 (2), 319–326. doi:10.1093/hmg/ddi028
Gómez-Díaz, E., Jordà, M., Peinado, M. A., and Rivero, A. (2012). Epigenetics of host–pathogen interactions: The road ahead and the road behind. Plos Pathog. 8 (11), e1003007. doi:10.1371/journal.ppat.1003007
Gupta, H., Chaudhari, S., Rai, A., Bhat, S., Sahu, P. K., Hande, M. H., et al. (2017). Genetic and epigenetic changes in host ABCB1 influences malaria susceptibility to Plasmodium falciparum. PLOS ONE 12 (4), e0175702. doi:10.1371/journal.pone.0175702
Gupta, H., and Wassmer, S. C. (2021). Harnessing the potential of miRNAs in malaria diagnostic and prevention. Front. Cell. Infect. Microbiol. 11, 793954. doi:10.3389/fcimb.2021.793954
Henrici, R. C., Sautter, C. L., Bond, C., Opoka, R. O., Namazzi, R., Datta, D., et al. (2021). Decreased parasite burden and altered host response in children with sickle cell anemia and severe anemia with malaria. Blood Adv. 5 (22), 4710–4720. doi:10.1182/bloodadvances.2021004704
Horvath, S., Oshima, J., Martin, G. M., Lu, A. T., Quach, A., Cohen, H., et al. (2018). Epigenetic clock for skin and blood cells applied to Hutchinson Gilford Progeria Syndrome and ex vivo studies. Aging 10 (7), 1758–1775. doi:10.18632/aging.101508
Houseman, E. A., Accomando, W. P., Koestler, D. C., Christensen, B. C., Marsit, C. J., Nelson, H. H., et al. (2012). DNA methylation arrays as surrogate measures of cell mixture distribution. BMC Bioinforma. 13 (1), 86. doi:10.1186/1471-2105-13-86
Idaghdour, Y., Quinlan, J., Goulet, J-P., Berghout, J., Gbeha, E., Bruat, V., et al. (2012). Evidence for additive and interaction effects of host genotype and infection in malaria. Proc. Natl. Acad. Sci. 109 (42), 16786–16793. doi:10.1073/pnas.1204945109
Jones, P. A. (2012). Functions of DNA methylation: Islands, start sites, gene bodies and beyond. Nat. Rev. Genet. 13 (7), 484–492. doi:10.1038/nrg3230
Kaimala, S., Kumar, C. A., Allouh, M. Z., Ansari, S. A., and Emerald, B. S. (2022). Epigenetic modifications in pancreas development, diabetes, and therapeutics. Med. Res. Rev. 42 (3), 1343–1371. doi:10.1002/med.21878
Khyzha, N., Alizada, A., Wilson, M. D., and Fish, J. E. (2017). Epigenetics of atherosclerosis: Emerging mechanisms and methods. Trends Mol. Med. 23 (4), 332–347. doi:10.1016/j.molmed.2017.02.004
Kim, M., and Costello, J. (2017). DNA methylation: An epigenetic mark of cellular memory. Exp. Mol. Med. 49 (4), e322–e. doi:10.1038/emm.2017.10
Kling, T., Wenger, A., and Carén, H. (2020). DNA methylation-based age estimation in pediatric healthy tissues and brain tumors. Aging 12 (21), 21037–21056. doi:10.18632/aging.202145
Kwiatkowski, D. (1990). Tumour necrosis factor, fever and fatality in falciparum malaria. Immunol. Lett. 25 (1-3), 213–216. doi:10.1016/0165-2478(90)90117-9
Lai, A. Y., Mav, D., Shah, R., Grimm, S. A., Phadke, D., Hatzi, K., et al. (2013). DNA methylation profiling in human B cells reveals immune regulatory elements and epigenetic plasticity at Alu elements during B-cell activation. Genome Res. 23 (12), 2030–2041. doi:10.1101/gr.155473.113
Laveran, A. (1881). Nature parasitaire des accidents de l'impaludisme: Description d'un nouveau parasite trouvé dans le sang des malades atteints de fièvre palustre. J.-B. Baillière. 93, 1–8.
Lee, Y-I., Ho, J-M., and Chen, M-S. “Clugo: A clustering algorithm for automated functional annotations based on gene ontology,” in Fifth IEEE International Conference on Data Mining (ICDM'05), Houston, TX, USA, November 27-30, 2005 (IEEE).
Lessard, S., Gatof, E. S., Beaudoin, M., Schupp, P. G., Sher, F., Ali, A., et al. (2017). An erythroid-specific ATP2B4 enhancer mediates red blood cell hydration and malaria susceptibility. J. Clin. Investigation 127 (8), 3065–3074. doi:10.1172/JCI94378
Lim, P. S., Li, J., Holloway, A. F., and Rao, S. (2013). Epigenetic regulation of inducible gene expression in the immune system. Immunology 139 (3), 285–293. doi:10.1111/imm.12100
Liu, Y., Aryee, M. J., Padyukov, L., Fallin, M. D., Hesselberg, E., Runarsson, A., et al. (2013). Epigenome-wide association data implicate DNA methylation as an intermediary of genetic risk in rheumatoid arthritis. Nat. Biotechnol. 31 (2), 142–147. doi:10.1038/nbt.2487
Loiseau, C., Cooper, M. M., and Doolan, D. L. (2020). Deciphering host immunity to malaria using systems immunology. Immunol. Rev. 293 (1), 115–143. doi:10.1111/imr.12814
Mackay, F., Loetscher, H., Stueber, D., Gehr, G., and Lesslauer, W. (1993). Tumor necrosis factor alpha (TNF-alpha)-induced cell adhesion to human endothelial cells is under dominant control of one TNF receptor type, TNF-R55. J. Exp. Med. 177 (5), 1277–1286. doi:10.1084/jem.177.5.1277
Mahittikorn, A., Mala, W., Srisuphanunt, M., Masangkay, F. R., Kotepui, K. U., Wilairatana, P., et al. (2022). Tumour necrosis factor-α as a prognostic biomarker of severe malaria: A systematic review and meta-analysis. J. Travel Med. 29 (4), taac053. doi:10.1093/jtm/taac053
Mallik, S., Odom, G. J., Gao, Z., Gomez, L., Chen, X., and Wang, L. (2019). An evaluation of supervised methods for identifying differentially methylated regions in Illumina methylation arrays. Briefings Bioinforma. 20 (6), 2224–2235. doi:10.1093/bib/bby085
Marr, A. K., MacIsaac, J. L., Jiang, R., Airo, A. M., Kobor, M. S., and McMaster, W. R. (2014). Leishmania donovani infection causes distinct epigenetic DNA methylation changes in host macrophages. Plos Pathog. 10 (10), e1004419. doi:10.1371/journal.ppat.1004419
McEwen, L. M., O’Donnell, K. J., McGill, M. G., Edgar, R. D., Jones, M. J., MacIsaac, J. L., et al. (2020). The PedBE clock accurately estimates DNA methylation age in pediatric buccal cells. Proc. Natl. Acad. Sci. 117 (38), 23329–23335. doi:10.1073/pnas.1820843116
McGuire, W., Hill, A. V. S., Allsopp, C. E. M., and Greenwoodt, B. M. (1994). Variation in the TNF-a promoter region associated with susceptibility to cerebral malaria. Nature 371, 508–511.
Monasso, G. S., Jaddoe, V. W. V., Küpers, L. K., and Felix, J. F. (2021). Epigenetic age acceleration and cardiovascular outcomes in school-age children: The Generation R Study. Clin. Epigenetics 13 (1), 205. doi:10.1186/s13148-021-01193-4
Netea, M. G., Latz, E., Mills, K. H. G., and O'Neill, L. A. J. (2015). Innate immune memory: A paradigm shift in understanding host defense. Nat. Immunol. 16 (7), 675–679. doi:10.1038/ni.3178
Nichol, K., and Pearson, A. C. E. (2002). CpG methylation modifies the genetic stability of cloned repeat sequences. Genome Res. 12 (8), 1246–1256. doi:10.1101/gr.74502
Nisar, S., Torres, M., Thiam, A., Pouvelle, B., Rosier, F., Gallardo, F., et al. (2022). Identification of ATP2B4 regulatory element containing functional genetic variants associated with severe malaria. Int. J. Mol. Sci. 23 (9), 4849. doi:10.3390/ijms23094849
Nishigaki, M., Aoyagi, K., Danjoh, I., Fukaya, M., Yanagihara, K., Sakamoto, H., et al. (2005). Discovery of aberrant expression of R-RAS by cancer-linked DNA hypomethylation in gastric cancer using microarrays. Cancer Res. 65 (6), 2115–2124. doi:10.1158/0008-5472.CAN-04-3340
Obata, Y., Furusawa, Y., and Hase, K. (2015). Epigenetic modifications of the immune system in health and disease. Immunol. Cell. Biol. 93 (3), 226–232. doi:10.1038/icb.2014.114
Pacis, A., Mailhot-Léonard, F., Tailleux, L., Randolph, H. E., Yotova, V., Dumaine, A., et al. (2019). Gene activation precedes DNA demethylation in response to infection in human dendritic cells. Proc. Natl. Acad. Sci. 116 (14), 6938–6943. doi:10.1073/pnas.1814700116
Pacis, A., Tailleux, L., Morin, A. M., Lambourne, J., MacIsaac, J. L., Yotova, V., et al. (2015). Bacterial infection remodels the DNA methylation landscape of human dendritic cells. Genome Res. 25 (12), 1801–1811. doi:10.1101/gr.192005.115
Pasparakis, M., Alexopoulou, L., Episkopou, V., and Kollias, G. (1996). Immune and inflammatory responses in TNF alpha-deficient mice: A critical requirement for TNF alpha in the formation of primary B cell follicles, follicular dendritic cell networks and germinal centers, and in the maturation of the humoral immune response. J. Exp. Med. 184 (4), 1397–1411. doi:10.1084/jem.184.4.1397
Pennini, M. E., Pai, R. K., Schultz, D. C., Boom, W. H., and Harding, C. V. (2006). Mycobacterium tuberculosis 19-kDa lipoprotein inhibits IFN-γ-Induced chromatin remodeling of MHC2TA by TLR2 and MAPK signaling. J. Immunol. 176 (7), 4323–4330. doi:10.4049/jimmunol.176.7.4323
Perkins, S. L. (2014). Malaria's many mates: Past, present, and future of the systematics of the order haemosporida. J. Parasitol. 100 (1), 11–25. doi:10.1645/13-362.1
Peters, T. J., Buckley, M. J., Statham, A. L., Pidsley, R., Samaras, K., V Lord, R., et al. (2015). De novo identification of differentially methylated regions in the human genome. Epigenetics Chromatin 8 (1), 6. doi:10.1186/1756-8935-8-6
Phipson, B., Maksimovic, J., and Oshlack, A. (2016). missMethyl: an R package for analyzing data from Illumina’s HumanMethylation450 platform. Bioinformatics 32 (2), 286–288. doi:10.1093/bioinformatics/btv560
Pidsley, R., Zotenko, E., Peters, T. J., Lawrence, M. G., Risbridger, G. P., Molloy, P., et al. (2016). Critical evaluation of the Illumina MethylationEPIC BeadChip microarray for whole-genome DNA methylation profiling. Genome Biol. 17 (1), 208. doi:10.1186/s13059-016-1066-1
Piotrowski, A., Benetkiewicz, M., Menzel, U., De Ståhl, T. D., Mantripragada, K., Grigelionis, G., et al. (2006). Microarray-based survey of CpG islands identifies concurrent hyper- and hypomethylation patterns in tissues derived from patients with breast cancer. Genes., Chromosomes Cancer 45 (7), 656–667. doi:10.1002/gcc.20331
Poinar, G. (2005). Plasmodium dominicana n. sp. (plasmodiidae: Haemospororida) from tertiary Dominican amber. Syst. Parasitol. 61 (1), 47–52. doi:10.1007/s11230-004-6354-6
Poulin, R., and Thomas, F. (2008). Epigenetic effects of infection on the phenotype of host offspring: Parasites reaching across host generations. Oikos 117 (3), 331–335. doi:10.1111/j.2007.0030-1299.16435.x
Rakyan, V. K., Beyan, H., Down, T. A., Hawa, M. I., Maslau, S., Aden, D., et al. (2011). Identification of type 1 diabetes–associated DNA methylation variable positions that precede disease diagnosis. PLoS Genet. 7 (9), e1002300. doi:10.1371/journal.pgen.1002300
Rusek, P., Wala, M., Druszczyńska, M., and Fol, M. (2018). Infectious agents as stimuli of trained innate immunity. Int. J. Mol. Sci. 19 (2), 456. doi:10.3390/ijms19020456
Sabou, M., Doderer-Lang, C., Leyer, C., Konjic, A., Kubina, S., Lennon, S., et al. (2020). Toxoplasma gondii ROP16 kinase silences the cyclin B1 gene promoter by hijacking host cell UHRF1-dependent epigenetic pathways. Cell. Mol. Life Sci. 77 (11), 2141–2156. doi:10.1007/s00018-019-03267-2
Santini, V., Kantarjian, H. M., and Issa, J-P. (2001). Changes in DNA methylation in neoplasia: Pathophysiology and therapeutic implications. Ann. Intern. Med. 134 (7), 573–586. doi:10.7326/0003-4819-134-7-200104030-00011
Sato, N., Maitra, A., and Fukushima, N. (2003). Frequent hypomethylation of multiple genes overexpressed in pancreatic ductal adenocarcinoma. Cancer Res. 63 (14), 4158–4166.
Sato, S. (2021). Plasmodium—A brief introduction to the parasites causing human malaria and their basic biology. J. Physiological Anthropol. 40 (1), 1. doi:10.1186/s40101-020-00251-9
Schrum, J. E., Crabtree, J. N., Dobbs, K. R., Kiritsy, M. C., Reed, G. W., Gazzinelli, R. T., et al. (2018). Cutting edge: Plasmodium falciparum induces trained innate immunity. J. Immunol. 200 (4), 1243–1248. doi:10.4049/jimmunol.1701010
Sellars, M., Huh, J. R., Day, K., Issuree, P. D., Galan, C., Gobeil, S., et al. (2015). Regulation of DNA methylation dictates Cd4 expression during the development of helper and cytotoxic T cell lineages. Nat. Immunol. 16 (7), 746–754. doi:10.1038/ni.3198
Shannon, P., Markiel, A., Ozier, O., Baliga, N. S., Wang, J. T., Ramage, D., et al. (2003). Cytoscape: A software environment for integrated models of biomolecular interaction networks. Genome Res. 13 (11), 2498–2504. doi:10.1101/gr.1239303
Silveira, E. L. V., Dominguez, M. R., and Soares, I. S. (2018). To B or not to B: Understanding B cell responses in the development of malaria infection. Front. Immunol. 9, 2961. doi:10.3389/fimmu.2018.02961
Somineni, H. K., Venkateswaran, S., Kilaru, V., Marigorta, U. M., Mo, A., Okou, D. T., et al. (2019). Blood-Derived DNA methylation signatures of Crohn's disease and severity of intestinal inflammation. Gastroenterology 156 (8), 2254–2265. doi:10.1053/j.gastro.2019.01.270
Soon, M. S. F., and Haque, A. (2018). Recent insights into CD4+ Th cell differentiation in malaria. J. Immunol. 200 (6), 1965–1975. doi:10.4049/jimmunol.1701316
Stern, E. N., Lessard, S., Schupp, P. G., Sher, F., Lettre, G., and Bauer, D. E. (2016). An essential erythroid-specific enhancer of ATP2B4 associated with red blood cell traits and malaria susceptibility. Blood 128 (22), 1250. doi:10.1182/blood.v128.22.1250.1250
Studniberg, S. I., Ioannidis, L. J., Utami, R. A. S., Trianty, L., Liao, Y., Abeysekera, W., et al. (2022). Molecular profiling reveals features of clinical immunity and immunosuppression in asymptomatic P. falciparum malaria. Mol. Syst. Biol. 18 (4), e10824. doi:10.15252/msb.202110824
Sun, S., and Barreiro, L. B. (2020). The epigenetically-encoded memory of the innate immune system. Curr. Opin. Immunol. 65, 7–13. doi:10.1016/j.coi.2020.02.002
Sun, T., and Chakrabarti, C. (1985). Schizonts, merozoites, and phagocytosis in falciparum malaria. Ann. Clin. Lab. Sci. 15 (6), 465–469.
Tecchio, C., and Cassatella, M. A. (2016). Neutrophil-derived chemokines on the road to immunity. Seminars Immunol. 28 (2), 119–128. doi:10.1016/j.smim.2016.04.003
Tiono, A. B., Guelbeogo, M. W., Sagnon, N. F., Nébié, I., Sirima, S. B., Mukhopadhyay, A., et al. (2013). Dynamics of malaria transmission and susceptibility to clinical malaria episodes following treatment of Plasmodium falciparum asymptomatic carriers: Results of a cluster-randomized study of community-wide screening and treatment, and a parallel entomology study. BMC Infect. Dis. 13 (1), 535. doi:10.1186/1471-2334-13-535
Uchechukwu, C., Priscella, C. E., Egondu, I., and Florence, O. (2017). The role of myeloid cells in immunity to malaria: A review. J. Clin. Cell. Immunol. 8 (4), 1000519. doi:10.4172/2155-9899.1000519
Wang, H., Lou, D., and Wang, Z. (2019). Crosstalk of genetic variants, allele-specific DNA methylation, and environmental factors for complex disease risk. Front. Genet. 9, 695. doi:10.3389/fgene.2018.00695
Wenzel, M. A., and Piertney, S. B. (2014). Fine-scale population epigenetic structure in relation to gastrointestinal parasite load in red grouse (Lagopus lagopus scotica). Mol. Ecol. 23 (17), 4256–4273. doi:10.1111/mec.12833
Wöhrle, D., Salat, U., Hameister, H., Vogel, W., and Steinbach, P. (2001). Demethylation, reactivation, and destabilization of human fragile X full-mutation alleles in mouse embryocarcinoma cells. Am. J. Hum. Genet. 69 (3), 504–515. doi:10.1086/322739
Wu, X., Chen, W., Lin, F., Huang, Q., Zhong, J., Gao, H., et al. (2019). DNA methylation profile is a quantitative measure of biological aging in children. Aging 11 (22), 10031–10051. doi:10.18632/aging.102399
Keywords: malaria, Plasmodium falciparum, DNA methyaltion, methylome, host parasite interactions, TNF
Citation: Almojil D, Diawara A, Soulama I, Dieng MM, Manikandan V, Sermé SS, Sombié S, Diarra A, Barry A, Coulibaly SA, Sirima SB and Idaghdour Y (2023) Impact of Plasmodium falciparum infection on DNA methylation of circulating immune cells. Front. Genet. 14:1197933. doi: 10.3389/fgene.2023.1197933
Received: 31 March 2023; Accepted: 02 June 2023;
Published: 04 July 2023.
Edited by:
Subhashree Nayak, National Institute of Arthritis and Musculoskeletal and Skin Diseases (NIH), United StatesReviewed by:
Mark Nicholas Cruickshank, University of Western Australia, AustraliaZe Zhang, Dartmouth College, United States
Copyright © 2023 Almojil, Diawara, Soulama, Dieng, Manikandan, Sermé, Sombié, Diarra, Barry, Coulibaly, Sirima and Idaghdour. This is an open-access article distributed under the terms of the Creative Commons Attribution License (CC BY). The use, distribution or reproduction in other forums is permitted, provided the original author(s) and the copyright owner(s) are credited and that the original publication in this journal is cited, in accordance with accepted academic practice. No use, distribution or reproduction is permitted which does not comply with these terms.
*Correspondence: Youssef Idaghdour, eW91c3NlZi5pZGFnaGRvdXJAbnl1LmVkdQ==
†Present address: Aïssatou Diawara, Global Institute for Disease Elimination, Abu Dhabi, United Arab Emirates
‡These authors have contributed equally to this work and share first authorship