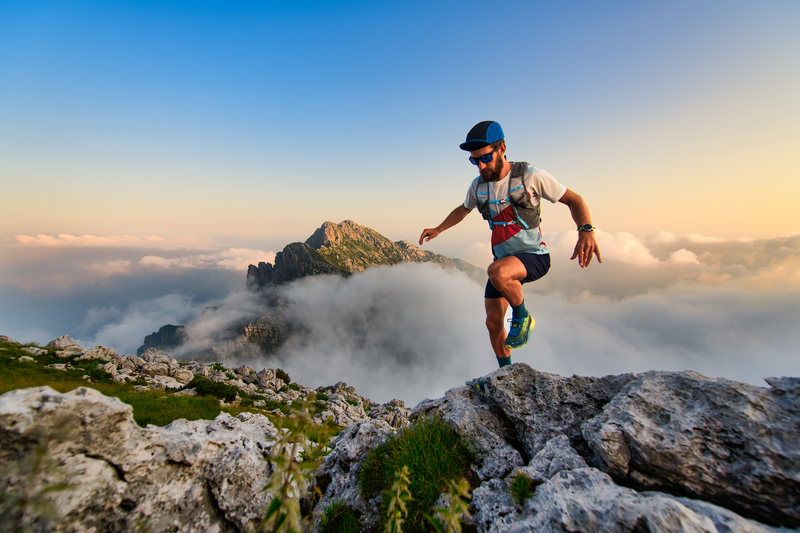
95% of researchers rate our articles as excellent or good
Learn more about the work of our research integrity team to safeguard the quality of each article we publish.
Find out more
EDITORIAL article
Front. Genet. , 14 April 2023
Sec. Evolutionary and Population Genetics
Volume 14 - 2023 | https://doi.org/10.3389/fgene.2023.1187687
This article is part of the Research Topic Cancer evolution View all 6 articles
Editorial on the Research Topic
Cancer evolution
Cancer is a complex disease (Donaldson et al., 2015; Yalcin et al., 2020) characterised by the breakdown of multicellular cooperation between somatic cells (Aktipis et al., 2015) that results in a set of convergent (Fortunato et al., 2017) traits known as the hallmarks of cancer (Hanahan, 2022). Cancer cells can proliferate extensively within a tissue, spread beyond the normal regulatory boundaries, and even colonise distant sites (Hanahan, 2022). Cancer progression is understood to be a complex Darwinian adaptive system (Greaves and Maley, 2012), with cancer cells acting as the equivalent of an asexually reproducing, unicellular quasi-species. Tumours are defined as large populations of genetically-diverse cell subpopulations competing for a limited number of nutrients while being selectively influenced by endogenous and exogenous factors. Because evolution and Darwinian selection are contingent and blind to the future, the outcome of this competition is the survival of clones well suited to flourish under specific conditions. Many clones dominant at one point in time may reach evolutionary dead ends and die out, while others may be able to persist. The level of genetic diversity found in cancer cannot be fully explained by Darwinian evolution, so non-Darwinian mechanisms have been proposed to account for cancer evolution (Vendramin et al., 2021). Neutral evolution, macroevolutionary changes, and the role of non-genetic determinants all appear to impact cancer progression significantly (Williams et al., 2016). The architecture of cancer seems sophisticated and intricate, and a thorough understanding of evolution is required for developing novel, evolutionary-informed therapeutic approaches (Gatenby and Brown, 2020). For this Research Topic, we gathered five studies covering three key aspects of cancer evolution.
Understanding the dynamics and possibly predicting cancer progression requires knowing how evolutionary forces alter the allele frequencies in somatic cells. Population genetic studies have generated critical insights in cancer evolution (Williams et al., 2019), including two of the articles in this Research Topic.
Luddy et al. investigated the principle of evolutionary triage (Gatenby et al., 2014), which connects the frequency of a gene mutation in a population with its contribution to cancer cell fitness. A mutation that improves fitness will spur cell proliferation, increasing the likelihood of its being found in a Research Topic of tumours. Instead, a mutation that does not affect fitness will only be detected at a frequency consistent with the underlying mutation rate. Luddy et al. used this framework to investigate genes associated with tumour-immune interactions in two lung adenocarcinoma cohorts with different molecular subtypes and found distinct convergent signatures of purifying selection. Targeting genes or molecular pathways under purifying selection may have therapeutic efficacy similar to targeting driver genes and may open up new therapeutic pathways (Gatenby and Brown, 2020).
Kurpas and Kimmel developed two alternative evolution models and applied them to cancer data to test if cancer cells follow Darwinian evolution. Both models describe the combined fitness effect of driver and passenger mutations in cancer cells; the only difference is how cellular fitness affects the likelihood of occupying the space of a dying cell. In one model, population fitness is constant in the absence of mutations, consistent with Darwinian selection, whereas fitness is not constant in the alternative. The two models were fitted to breast cancer samples, and it was found that the model consistent with Darwinian selection fits the data better.
Molecular signatures can elucidate mechanisms of cancer progression and inform the clinical practice of many cancer types, perhaps most notably breast cancer (Lal et al., 2017).
Yan et al. developed a novel prognostic signature grounded on a set of necroptosis-related genes to predict breast cancer patient outcomes based on median risk scores. Patients above and below the median are classified as high-risk and low-risk, respectively. High-risk patients encounter low overall survival and worse predicted tumour, node, and metastasis stages.
Cisneros et al. studied the role of stress-induced mutagenesis (SIM) in cancer and introduced new statistical methods to identify its signature. Using somatic mutations found in tumour and normal tissue and SIM cell lines, they identified clusters of mutations consistent with stress-induced mutagenesis. These genetic clusters were less conserved in cancer, indicating a loss of regulatory control of SIM, and stress-induced mutagenesis was related to patient survival. This study shows that an evolutionary conserved adaptive mutation response, already present in bacteria, is a source of genomic instability that fuels cancer initiation, progression, and therapeutic resistance (Fitzgerald et al., 2017). This process may explain tumours’ ability to evolve under selective pressures, supporting evolutionary-based treatment strategies (Natterson-Horowitz et al., 2023).
Multi-omic studies explore the interaction between multiple biological factors to provide a more holistic view of biological processes and their relative effect on an outcome (Song et al., 2020). The development of a new multi-omics tool completes this Research Topic on cancer evolution.
Huzar et al. describe MOCA, a multi-omics toolkit for comparing gene expression and genetic evolution patterns in single cells and testing their consistency. MOCA was applied to published datasets and revealed links between genetic and phenotypic changes that can aid in understanding tumorigenesis, therapy resistance, and cancer progression. A significant shift toward single-cell technologies is expected in the coming years (Rozenblatt-Rosen et al., 2020), and MOCA will enable better exploitation of these upcoming datasets.
Cancer is an evolutionary process of somatic cells, and therefore the somatic evolutionary theory is critical to understanding and treating this major cause of death. The articles in this Research Topic emphasise and exemplify this fact. Evolutionary therapies are promising treatment strategies that use the most recent advances in somatic evolutionary theory to manipulate the evolution of cancer cells, controlling their growth or driving them to extinction. These advancements have the potential to improve the quality of life of cancer patients by transforming cancer into a chronic disease or, in some cases, eradicating it.
All listed authors contributed significantly, directly, and intellectually to the work and approved its publication.
This work was partly supported by US National Cancer Institute grants NIH R01 CA140657, NIH U54 CA217376, NIH U2C CA233254, and R21 CA257980 (DM). AA is supported by the International Fellowship for Outstanding Researchers Program administered by TUBITAK (grant number 118C197).
We thank the authors who contributed to this Research Topic and the expert reviewers for their timely work and efforts.
The authors declare that the research was conducted in the absence of any commercial or financial relationships that could be construed as a potential conflict of interest.
All claims expressed in this article are solely those of the authors and do not necessarily represent those of their affiliated organizations, or those of the publisher, the editors and the reviewers. Any product that may be evaluated in this article, or claim that may be made by its manufacturer, is not guaranteed or endorsed by the publisher.
Aktipis, C. A., Boddy, A. M., Jansen, G., Hibner, U., Hochberg, M. E., Maley, C. C., et al. (2015). Cancer across the tree of life: Cooperation and cheating in multicellularity. Philos. Trans. R. Soc. Lond. B Biol. Sci. 370. doi:10.1098/rstb.2014.0219
Donaldson, P., Daly, A., Ermini, L., and Bevitt, D. (2015). Genetics of complex disease. Garl. Sci. 2015, 450. doi:10.1201/9780429258688
Fitzgerald, D. M., Hastings, P. J., and Rosenberg, S. M. (2017). Stress-induced mutagenesis: Implications in cancer and drug resistance. Annu. Rev. Cancer Biol. 1, 119–140. doi:10.1146/annurev-cancerbio-050216-121919
Fortunato, A., Boddy, A., Mallo, D., Aktipis, A., Maley, C. C., and Pepper, J. W. (2017). Natural selection in cancer biology: From molecular snowflakes to trait hallmarks. Cold Spring Harb. Perspect. Med. 7, a029652. doi:10.1101/cshperspect.a029652
Gatenby, R. A., and Brown, J. S. (2020). Integrating evolutionary dynamics into cancer therapy. Nat. Rev. Clin. Oncol. 17, 675–686. doi:10.1038/s41571-020-0411-1
Gatenby, R. A., Cunningham, J. J., and Brown, J. S. (2014). Evolutionary triage governs fitness in driver and passenger mutations and suggests targeting never mutations. Nat. Commun. 5, 5499. doi:10.1038/ncomms6499
Greaves, M., and Maley, C. C. (2012). Clonal evolution in cancer. Nature 481, 306–313. doi:10.1038/nature10762
Hanahan, D. (2022). Hallmarks of cancer: New dimensions. Cancer Discov. 12, 31–46. doi:10.1158/2159-8290.CD-21-1059
Lal, S., McCart Reed, A. E., de Luca, X. M., and Simpson, P. T. (2017). Molecular signatures in breast cancer. Methods 131, 135–146. doi:10.1016/j.ymeth.2017.06.032
Natterson-Horowitz, B., Aktipis, A., Fox, M., Gluckman, P. D., Low, F. M., Mace, R., et al. (2023). The future of evolutionary medicine: Sparking innovation in biomedicine and public health. Front. Sci. 1. doi:10.3389/fsci.2023.997136
Rozenblatt-Rosen, O., Regev, A., Oberdoerffer, P., Nawy, T., Hupalowska, A., Rood, J. E., et al. (2020). The human tumor atlas network: Charting tumor transitions across space and time at single-cell resolution. Cell 181, 236–249. doi:10.1016/j.cell.2020.03.053
Song, M., Greenbaum, J., Luttrell, J., 4thZhou, W., Wu, C., Shen, H., et al. (2020). A review of integrative imputation for multi-omics datasets. Front. Genet. 11, 570255. doi:10.3389/fgene.2020.570255
Vendramin, R., Litchfield, K., and Swanton, C. (2021). Cancer evolution: Darwin and beyond. EMBO J. 40, e108389. doi:10.15252/embj.2021108389
Williams, M. J., Sottoriva, A., and Graham, T. A. (2019). Measuring clonal evolution in cancer with genomics. Annu. Rev. Genomics Hum. Genet. 20, 309–329. doi:10.1146/annurev-genom-083117-021712
Williams, M. J., Werner, B., Barnes, C. P., Graham, T. A., and Sottoriva, A. (2016). Identification of neutral tumor evolution across cancer types. Nat. Genet. 48, 238–244. doi:10.1038/ng.3489
Keywords: cancer evolution, evolutionary biology, somatic cell, cancer phylogenetics, tumor heterogeneity
Citation: Ermini L, Mallo D, Kleftogiannis D and Acar A (2023) Editorial: Cancer evolution. Front. Genet. 14:1187687. doi: 10.3389/fgene.2023.1187687
Received: 16 March 2023; Accepted: 05 April 2023;
Published: 14 April 2023.
Edited and reviewed by:
Samuel A. Cushman, United States Department of Agriculture (USDA), United StatesCopyright © 2023 Ermini, Mallo, Kleftogiannis and Acar. This is an open-access article distributed under the terms of the Creative Commons Attribution License (CC BY). The use, distribution or reproduction in other forums is permitted, provided the original author(s) and the copyright owner(s) are credited and that the original publication in this journal is cited, in accordance with accepted academic practice. No use, distribution or reproduction is permitted which does not comply with these terms.
*Correspondence: Luca Ermini, bHVjYS5lcm1pbmlAeWFob28uY28udWs=; Diego Mallo, ZG1hbGxvYWRAYXN1LmVkdQ==; Dimitrios Kleftogiannis, ZGltaXRyaW9zLmtsZWZ0b2dpYW5uaXNAdWliLm5v; Ahmet Acar, YWNhcmFAbWV0dS5lZHUudHI=
†These authors have contributed equally to this work and share first and senior authorship
Disclaimer: All claims expressed in this article are solely those of the authors and do not necessarily represent those of their affiliated organizations, or those of the publisher, the editors and the reviewers. Any product that may be evaluated in this article or claim that may be made by its manufacturer is not guaranteed or endorsed by the publisher.
Research integrity at Frontiers
Learn more about the work of our research integrity team to safeguard the quality of each article we publish.