- 1State Key Laboratory for Managing Biotic and Chemical Threats to The Quality and Safety of Agro-products, Zhejiang Academy of Agricultural Sciences, Hangzhou, China
- 2Institute of Vegetables, Zhejiang Academy of Agricultural Sciences, Hangzhou, China
Genome-wide association studies have revealed that the regulation of gene expression bridges genetic variants and complex phenotypes. Profiling of the bulk transcriptome coupled with linkage analysis (expression quantitative trait locus (eQTL) mapping) has advanced our understanding of the relationship between genetic variants and gene regulation in the context of complex phenotypes. However, bulk transcriptomics has inherited limitations as the regulation of gene expression tends to be cell-type-specific. The advent of single-cell RNA-seq technology now enables the identification of the cell-type-specific regulation of gene expression through a single-cell eQTL (sc-eQTL). In this review, we first provide an overview of sc-eQTL studies, including data processing and the mapping procedure of the sc-eQTL. We then discuss the benefits and limitations of sc-eQTL analyses. Finally, we present an overview of the current and future applications of sc-eQTL discoveries.
1 Introduction
Over the past decades, genome-wide association studies (GWAS) have successfully identified thousands of genetic variants associated with over 100 common diseases (Visscher et al., 2017). However, the vast majority of these variants are in non-coding regions (Brodie et al., 2016) and exert their effect function by regulating gene expression. Expression quantitative trait locus (eQTL) mapping, which links genetic variants to the variation in gene expression, has largely been performed in bulk transcriptomic data generated by RNA-seq and microarray technologies. However, a significant proportion of GWAS loci cannot be explained by eQTL signals in bulk transcriptomic data, in which expression levels are averaged across all cells in a sample.
One solution to this problem is to study the regulation of gene expression at the cell-type-specific level (Knowles et al., 2017; Favé et al., 2018). Several previous studies in purified blood cell populations (Fairfax et al., 2012; Ishigaki et al., 2017; Donovan et al., 2020; Kim-Hellmuth et al., 2020; Yao et al., 2021) have already identified cell-type-specific regulation. The recent advent of scRNA-seq technology has revolutionized our ability to understand cell-type-specific gene expression by resolving complex cellular heterogeneity.
The single-cell expression quantitative trait locus (sc-eQTL) is emerging as a powerful tool to identify cell-type-specific regulation of gene expression. For example, a recent study performed eQTL mapping using single nuclei RNA-seq from 196 individuals in eight CNS cell types and identified 6,108 eGenes, 43% of which have cell-type-specific effects. The study provided new insights into the disease etiology and genetic mechanisms influencing neurological disorders (Bryois et al., 2022), demonstrating that sc-eQTL mapping provides a powerful approach to link genetic variants to complex diseases.
In this review, we aim to provide a comprehensive overview of sc-eQTL studies. We begin with an introduction to data processing and mapping procedures used in sc-eQTL analyses and provide details of the methods used in the analysis of the cell-type-specific regulation of gene expression. We then discuss the benefits of sc-eQTL studies compared to traditional eQTL analyses using bulk transcriptomic data. The limitations and challenges of sc-eQTL analyses are also discussed. Finally, we present a comprehensive overview of the current and future applications of sc-eQTL discoveries.
2 Evolution of sc-eQTL analyses: from an early approach to recent developments
The concept of cell-type-specific eQTLs was first introduced in 2013 in a study that measured 92 genes in 1,440 single cells from 15 individuals (Wills et al., 2013) to explore whether studying individual cells could provide greater mechanistic insights into how genetic variants quantitatively affect gene expression. However, the first large-scale genome-wide sc-eQTL study was performed in 2018 in eight major immune cell populations from 78,000 peripheral blood mononuclear cells (PBMCs) from 23 donors (Kang et al., 2018; Ma et al., 2022). This study was further expanded by identifying unfound cell-type-specific and co-expression eQTLs (van der Wijst et al., 2018) in 25,000 PBMCs from 45 donors. Similar sc-QTL studies using different single-cell transcriptomic technologies were also reported (Sarkar et al., 2019; Cuomo et al., 2020a; Mandric et al., 2020; Van Der Wijst et al., 2020; Figure 1). Single-cell transcriptomic technologies primarily fall into two categories: one that captures the full length of transcripts (e.g., Smart-seq2, MATQ-seq2, and SUPeR-seq) and another that captures the 3′/5′ends of transcripts. Full-length transcript sequencing allows for the detection of the complete transcriptome and the analysis of alternative splicing; its high cost and limited scalability makes it impractical for large-scale studies. In contrast, 3′/5′-end transcript sequencing, while less sensitive in detecting gene expression and alternative splicing, is more cost-effective and scalable and can, thus, accommodate more cells (Svensson et al., 2017; Chen et al., 2019). Recently, long-read sequencing technologies, such as PacBio and Oxford Nanopore, have emerged as powerful tools in the field, enabling the detection of full-length transcripts at high throughput and with high accuracy. These technologies are still in their infancy, but they hold great potential for expanding the capabilities of single-cell transcriptomic studies and can be expected to impact the sc-eQTL study.
Similar to eQTL analyses at the bulk level, gene regulation can be classified into two types: cis-regulation (local) and trans-regulation (distant). Most sc-eQTL studies have focused on cis-regulation due to the statistical power. In theory, cis-eQTLs can be mapped for all the genes measured in each cell. However, owing to the coverage of scRNA-seq, the identification of cis-eQTLs is currently only limited to cell-type levels. As a result, current sc-eQTL studies mainly attempt to identify cell-type-specific cis-eQTLs using single-cell transcriptomics (van der Wijst et al., 2018). To overcome the coverage issue of single-cell transcriptomic data and utilize expression levels measured by bulk transcriptomics, many computational deconvolution methods were developed to integrate single-cell and bulk transcriptomic data to identify cell-type-specific cis-eQTLs. However, a limitation of the deconvolution methods is that the analyzed cis-eQTLs were assigned to known cell types. Several studies also pointed out that the analysis of cis-eQTLs directly detected by single-cell transcriptomics outperforms deconvolution methods (Perez et al., 2022; Yazar et al., 2022).
3 Data processing for sc-eQTL mapping
While significant efforts have been made in the development of statistical methods for bulk transcriptomic data, most of these methods cannot be directly applied to sc-eQTL studies. This is because single-cell transcriptomic data have unique characteristics, such as zero-inflated gene expression. As a result, several crucial processing steps are needed to be performed before utilizing statistic methods developed for bulk RNA-seq studies on single-cell transcriptomic data.
3.1 Preprocessing single-cell transcriptomic data for eQTL mapping
The main processes involved in preparing single-cell transcriptomic data for eQTL mapping involve several key steps, including cell-level gene expression counting, quality control (QC), mean aggregation, covariate correlation procedures, and multiple testing corrections in the context of sc-eQTL mapping (Figure 2). A study by has provided optimized eQTL mapping workflows for single-cell studies (Cuomo et al., 2021).
The process starts with counting the cell-level gene expression, which can be obtained using a variety of different methods (Teng et al., 2016; Vieth et al., 2019; Chen et al., 2021). As for digital transcript quantification, transcripts from tag-based sequencing can be combined with UMI tags. UMI tags are a series of short sequences with specifically ordered bases; they are added to the ends of cDNAs during reverse transcription, and PCR products from the same cDNA would carry the same UMI molecule. Therefore, UMI tags can distinguish cDNA repeats from biology repeats. However, transcripts from full-length scRNA-seq cannot be combined with UMI molecules, which results in a lower quality of transcript counting based on full-length sequencing than that based on tag-based sequencing. However, MATQ-seq can produce full-length transcripts that can be combined with UMI molecules (Macosko et al., 2015).
QC steps should be performed at the cell level to remove low-quality cells and normalize data to remove technical variations in the sequencing depth per cell. Batch corrections should also be used to remove poor-quality batches. A study by (Luecken and Theis, 2019) provides an overview of their best practices. Moreover, Xue et al. (2023) proposed a new guideline to optimize the number of latent variables for bulk data batch-effect correction tools, such as probabilistic estimation of expression residuals (PEER) and principal component analysis (PCA), thereby improving the power of sc-eQTL discovery. A list of methods/tools on data transformation, scaling/normalization, and batch effect correction are provided in Table 1 and Supplementary Table S1. Among batch effect correction methods in Table 1, some are linear methods (e.g., limma and ComBat) and some belong to NN-based methods (e.g., fastMNN, Scanorama, and Seurat). The four methods (WaVE, scMerge, scVI, and LIGER) in Table 1 can handle normalization and batch correction together (Chu et al., 2022). (Tran et al., 2020) compared 14 batch effect correction methods in five scenarios. In general, the tools Harmony, LIGER, and Seurat 3 perform well in batch processing. When correcting batch effects for unknown cell types, LIGER is preferred. However, the runtime of LIGER is comparatively long. Seurat 3 enables the handling of large datasets, but requires a longer runtime. To perform downstream DEG analysis well, the scMerge tool is recommended.
After quality control, it is necessary to perform clustering and cell-type assignment for scRNA-seq data (Cuomo et al., 2021). Major clustering tools for scRNA-seq data are based on the combination of basic clustering methods, which contain feature selection and dimensionality reduction, k-means, hierarchical clustering, and so on. Feature selection can identify genes with the highest variance. Dimensionality reduction projects data into a low-dimensional space, trying to preserve the original pairwise distances between points in the data as much as possible. Principal component analysis is one of the classical dimensionality reduction methods. Many methods, including Euclidean distance, cosine similarity, Pearson’s correlation, Spearman’s correlation, and so on, can be used to calculate the distance between points in a lower-dimensional space. K-means iteratively identifies k-cluster centers (centroids), and each cell in scRNA-seq data is assigned to the closest centroid. K-means can deal with large datasets but is not guaranteed to find the global minimum, and additionally, it is biased toward identifying equal-sized clusters, while omitting rare cell types. Another widely used clustering algorithm is hierarchical clustering, which combines individual cells into larger clusters or divides clusters into smaller groups. A visible disadvantage of hierarchical clustering is the high cost of time and memory for a large dataset. Community detection is a variant of clustering and is especially applied to graphs. This method identifies groups of nodes that are densely connected. An advantage of graph-based methods is that they do not need to specify the number of clusters.
As a single clustering method has notable disadvantages, many tools, including clustering modules, are based on a combination of several basic clustering methods. For example, clustering modules in Scanpy (Wolf et al., 2018), Seurat (Hao et al., 2021), PhenoGraph (Levine et al., 2015), SC3 (Kiselev et al., 2017; Kiselev et al., 2019), CIDR (Lin et al., 2017), pcaReduce (Žurauskienė and Yau, 2016), and TSCAN (Ji and Ji, 2016) are based on a combination of PCA and other basic clustering methods. SIMLR (Wang et al., 2018) is based on data-driven dimensionality reduction and k-means. GiniClust (Jiang et al., 2016) is based on DBSCAN; mpath and SINCERA (Guo et al., 2015) are based on hierarchical clustering; BackSPIN (Zeisel et al., 2015) is based on biclustering; RaceID3 (Grün et al., 2015) is based on k-means; and SNN-Cliq is graph-based. So, there are several user-friendly clustering tools available today. However, they have been developed for solving certain problems and it is impossible for them to be suitable for all situations.
Choosing suitable clustering and cell-type assignment algorithms for scRNA-seq data is vital (Luecken and Theis, 2019). The identification or classification of a cell into the right type or state is especially important (Van Der Wijst et al., 2020). For example, developed a clustering method based on sorting points into neighborhoods (SPIN) (Tsafrir et al., 2005). Some methods identify cell types through unsupervised clustering, such as pcaReduce and SC3. A major challenge in cell-type profiling is to identify rare cell types. A developed algorithm named rare cell-type identification (RaceID) infers abundant cell types by k-means clustering followed by systematic outlier screening (Grün et al., 2015). GiniClust detects rare cell types from single-cell gene expression data with the Gini index (Jiang et al., 2016), and GiniClust2, the upgraded version of GiniClust, is a cluster-aware weighted ensemble clustering method for cell-type detection (Tsoucas and Yuan, 2018). A newly developed tool, CellSIUS, can provide the sensitive and specific detection of rare cell populations from complex scRNA-seq data (Wegmann et al., 2019). Mean aggregation of gene expression across cells for each cell type is typically conducted by averaging gene profiles across cell types. Cell or cell-type-specific eQTLs can be mapped using eQTL mapping methods, developed especially for scRNA-seq data (Figure 2).
3.2 Methods used for sc-eQTL mapping
After preprocessing single-cell transcriptomic data, eQTL mapping is applied to identify genetic variants regulating gene expression at the single-cell-type level. Mapping can be carried out through various methods, including some sc-eQTL-specific tools (Table 2) and bulk eQTL mapping methods (Supplementary Table S2). These methods can be classified into two categories: parametric and non-parametric methods. Parametric methods, such as linear regression and ANOVA, assume that gene expression follows the normal distribution, Poisson distribution, or negative binomial distribution and use gene expression as the dependent variable, and genotypes as independent variables (Gatti et al., 2009; Shabalin, 2012). In contrast, non-parametric methods, such as the Krux method, are considered more robust and do not rely on any distribution assumption (Qi et al., 2014). Each tool presented in Table 2 has specific advantages. For example, SCeQTL (R package) utilizes zero-inflated negative binomial regression for eQTL mapping in scRNA-seq data (Hu et al., 2020). eQTLsingle can discover eQTLs solely through scRNA-seq data, without the use of genomic data (Ma et al., 2022). FastGxC is an efficient and powerful tool for mapping context-specific eQTLs in scRNA-seq data (Lu et al., 2021). Lastly, scTBLDA considers information across cell types, which is often ignored by methods that use summary statistics within cell types (Gewirtz et al., 2022).
Similar to traditional bulk eQTL mapping, the effects of covariates are typically removed from a sc-eQTL analysis to improve the sensitivity and interpretability of genetic associations in population-scale expression data. For example, a recent cell-type-specific eQTL in fibroblasts and fibroblast-derived iPSC types used different covariates and probabilistic estimation of expression residual factors (Shabalin, 2012; Neavin et al., 2021). Additionally, Xue et al. (2023) highlighted three key differences between bulk data and scRNA-seq pseudo-bulk data and provided a new guideline for selecting the optimal number of latent variables for bulk data batch-effect correction tools. This guideline has the potential to significantly improve sc-eQTL discovery and is an important contribution to the field.
The method specifically developed for sc-eQTL mapping can efficiently identify context-specific genetic variants regulating gene expression at the cell-type-specific level. For example, a method called FastGxC enables the construction of context-specific eQTL maps and has the potential to increase precision in identifying GWAS variants by three-fold compared to conventional eQTL mapping methods (Lu et al., 2021).
Compared to conventional eQTL mapping methods, sc-eQTL mapping strategies face the challenge of excessive zeros in single-cell transcriptomic data (Delmans and Hemberg, 2016; Miao et al., 2018; Hu et al., 2020). To address this challenge, the R package SCeQTL uses zero-inflated negative binomial regression for the sc-eQTL analysis to detect the gene expression variation and distinguish between “status difference” and “expression level difference” (Hu et al., 2020). Some recent approaches also take into account the dynamic pseudotime-defined cell types for the sc-eQTL analysis (Cuomo et al., 2020b), which have been shown to uncover new eQTL variants. In addition, the eQTLsingle tool was developed to discover eQTLs solely with single-cell transcriptomic data and detect mutations from single-cell transcriptomic data as genotypic data (Ma et al., 2022).
4 Advantages and limitations of sc-eQTL mapping
4.1 Advantages of sc-eQTL mapping compared to bulk eQTL methods
Single-cell transcriptomic data provide several advantages in exploring the genetic architecture of gene regulation. The ability of detecting cell types and cell states in an unbiased manner using single-cell transcriptomic data makes sc-eQTL mapping a powerful tool for studying the genetic architecture of gene regulation (Grün et al., 2015; Villani et al., 2017; Hernández et al., 2018; Karamitros et al., 2018; Guerrero-Juarez et al., 2019; Umans et al., 2020).The advantages of sc-eQTL mapping include the following: 1) discovery of cell-type-specific eQTLs, 2) identification of eQTLs regulating lowly expressed genes, and 3) detection cell-type-specific eQTLs in different spatiotemporal states. We discuss these advantages in detail in the following sections (Figure 3).
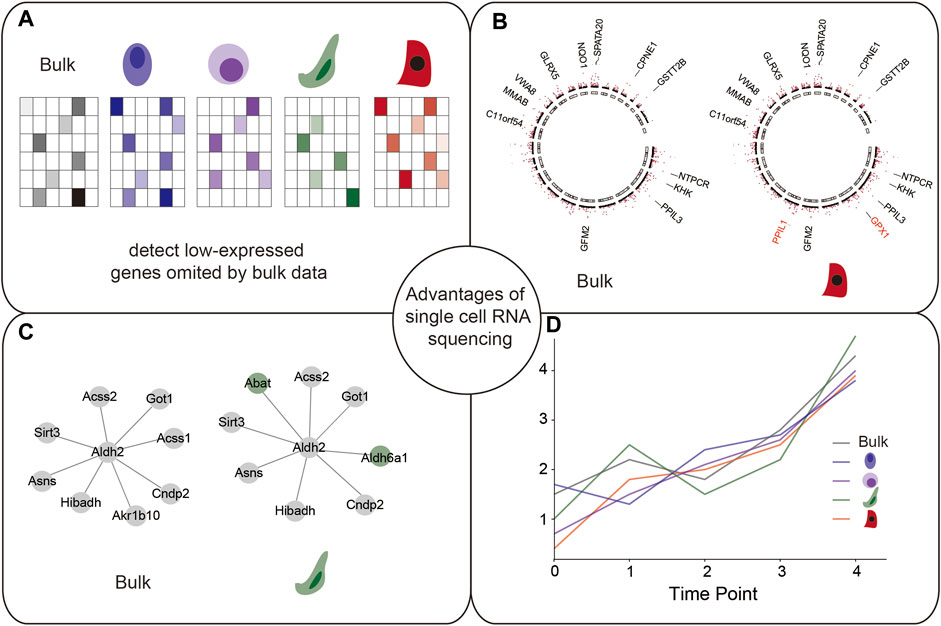
FIGURE 3. Advantages of scRNA-seq data, including (A) Identifying cell-type-specific eQTLs; (B) identifying low-expressed genes; (C) identifying cell-type-specific co-expression networks; and (D) identifying cell-type-specific eQTLs in different spatiotemporal states.
4.1.1 Discovery of cell-type-specific eQTLs that are diluted in bulk RNA-seq
Single-cell transcriptomic data offer a powerful tool to uncover cell-type-specific eQTLs that are diluted in bulk transcriptomic data. Cell-type-specific cis-eQTLs identified by bulk RNA-seq data are biased to known cell types, while the ones identified by scRNA-seq data can be assigned to novel cell types. Multiple studies have demonstrated this advantage. For example, a study discovered 379 cis-eQTLs (287 genes), of which 48 cis-eQTLs (38 genes) were only detected in specific cell types, not in any eQTLs from bulk RNA-seq data (van der Wijst et al., 2018). Another study on human skin fibroblasts showed that a majority of discovered eGenes were predominantly cell-type-specific and could only be identified in one fibroblast type or one iPSC type (Neavin et al., 2021). These findings suggest a high degree of cell-type-specific gene regulations detected in the sc-eQTL analysis that cannot be captured by bulk QTL mapping. Hence, sc-eQTL can be used to improve the eQTL detection when compared to bulk RNA-seq.
4.1.2 Identification of eQTLs regulating lowly expressed genes that are omitted by bulk data
Compared with bulk RNA-seq data, scRNA-seq data allow the estimation of the variability in gene expression across individual cells (Brennecke et al., 2013) and provide a new angle on how genetics may impact disease pathogenesis. For example, owing to the low expression of TSPAN13 in abundant CD4+ T cells, cis-eQTL rs2272245 was not identified in the bulk RNA-seq dataset (Zhernakova et al., 2017), but it significantly affected the low expressed gene TSPAN13 in cis (p = 2.21 × 10−6) in the scRNA-seq data analysis. This shows that the bulk RNA-seq-based cis-eQTL analysis loses power in the identification of cell-type-specific loci affecting lowly expressed genes (van der Wijst et al., 2018).
4.1.3 Detection of cell-state-specific eQTLs while bulk data lose this power
scRNA-seq data enable the simultaneous estimation of the composition and expression profiles of discrete cell populations, such as their activation states (van der Wijst et al., 2018). scRNA-seq data provide a flexible unbiased approach that has increased their resolution to define cell states along continuous dynamic processes, in which the eQTL effects manifest themselves (Cuomo et al., 2020a). In an elegant study by, the authors derived 126 iPSC cell lines from 125 donors in the HipSci project (Kilpinen et al., 2017) and harvested the cells immediately before differentiation (iPSCs) and at the mesendoderm and definitive endoderm stage of differentiation (Cuomo et al., 2020b). They found that over 30% of the identified eQTLs were specific to a single stage. Moreover, 349 eQTL variants identified during differentiation stages were novel and not previously identified in bulk RNA-Seq from iPSCs or GTEx tissues, and they also illustrated that eQTLs can modulate the timing of expression changes in response to differentiation (Cuomo et al., 2020a). Altogether the study demonstrated that the identification of eQTLs at distinct time points in the development allows the discovery of novel regulatory relationships.
In a study by the mapped eQTLs in memory T cells from 259 Peruvian individuals revealed more than 2,000 eQTLs, whose presence and function varied according to the transcriptomic state of T cells. So, they demonstrated that DNA sequence variation at a particular location in the genome may influence the expression of a given gene in some T-cell states but not in others (Nathan et al., 2022).
Another study by Yazar et al. (2022) identified cell-state-dependent eQTLs in B cells transitioning from naïve to memory states. In an example with rs9927852 and MAF, the expression of MAF increased with a high cytotoxic cell-state score and remained relatively constant with low cell-state scores. So, they demonstrated that two independent eQTLs have opposite effects on the expression of the same gene in different cell states. The above two studies emphasize the complexity of genome regulation in immune cells, and scRNA-seq increases the resolution of the identified eQTLs (Yazar and Powell, 2022).
4.2 Limitations of scRNA-seq in eQTL mapping
Despite the many benefits of sc-eQTL mapping, as shown previously, several limitations have also been noted in recent studies. These limitations include the following: 1) less power in identifying eQTLs, 2) high cost of scRNA sequencing, and 3) technical noises in scRNA-seq data.
4.2.1 Less power in identifying eQTLs
sc-eQTL mapping provides a detailed annotation of the eQTL effects across diverse cell types and cell states, enabling a better interpretation of the context-specific role of individual genetic variants (Cuomo et al., 2020b). However, owing to increased experimental noise, sc-eQTL mapping has lower power to discover eQTLs compared to bulk RNA-seq data. Thus, scRNA-seq data require larger sample sizes to identify the same number of eQTLs as bulk data (Sarkar et al., 2019). For instance, scRNA-seq studies by and Perez et al., 2022 identified less than 15 cell types, whereas Ota et al., 2021 identified 28 cell types in bulk RNA-seq data (Ota et al., 2021; Perez et al., 2022; Yazar et al., 2022). As a result, if the same sample size is used for scRNA-seq, a lower number of cis-eQTLs will be detected in scRNA-seq data compared to bulk data.
4.2.2 High cost of scRNA sequencing
The second limitation of the sc-eQTL study is the high cost associated with scRNA-seq, which is a relatively expensive method for gene expression analysis. While a typical bulk RNA-sequencing experiment requires up to 20 million sequencing reads per sample, scRNA-seq needs a much higher coverage, typically 50,000 to 150,000 reads per cell. A simple scRNA-seq experiment would include thousands of cells, with hundreds of thousands of reads. For example, to detect one thousand reads per cell, it needs to detect 50–150 million reads per sample, where the number of reads captured in scRNA-seq is 2.5–7.5 times larger than that in bulk RNA-seq. Therefore, scRNA-seq needs much more memory and storage space than bulk RNA-seq experiments.
4.2.3 Noise in the scRNA-seq dataset
scRNA-seq data are high dimensional and complex. When compared to traditional bulk RNA-seq, scRNA-seq needs to amplify genetic material in each cell to meet the requirements of sequencing platforms. The amplification processes bring many technical problems, such as a notable amplification bias and low genome coverage in DNA amplification, so the clustering and homogenization analysis strategies used in bulk RNA-seq cannot be used directly in scRNA-seq data analyses. As a result, there are many differences in various cells and platforms, and library sizes vary greatly between each other. So, there is much more noises in scRNA-seq data, which demand a series of pretreatment steps before the scRNA-seq data analysis.
4.3 Strategies to overcome the limitations of scRNA-seq in mapping eQTLs
4.3.1 Decreasing the cost of scRNA-seq
One of the main limitations of scRNA-seq is its high cost. However, with the development of cost-effective multiplexed workflows, that limitation has been significantly mitigated, enabling a broader adoption of population-scale scRNA-seq and cell-type-specific eQTL studies (van der Wijst et al., 2018; Zhang et al., 2018; Cuomo et al., 2020a). Through a series of simulations, Igor M. et al. demonstrated that by increasing the sample size and number of cells per individual while decreasing coverage, it was possible to reduce the cost of the scRNA-seq experiment by half (or even more), while maintaining the same statistical power. Furthermore, they provided a practical guideline for designing cell-type-specific eQTLs (Mandric et al., 2020).
4.3.2 Developing methods for deconvoluting bulk RNA-seq signals into different cell types
The high cost of single-cell transcriptomic sequencing has led to the development of several deconvolution methods to estimate the cell-type level gene expression from the bulk mRNA expression. These deconvolution methods, such as DeconRNAseq (Gong and Szustakowski, 2013), CIBERSORT (Newman et al., 2015), CIBERSORTx (Newman et al., 2019), BSEQ-sc (Baron et al., 2016), TIMER (Li et al., 2016), MuSiC (Qin et al., 2021), DSA (Zhong et al., 2013), and MMAD (Liebner et al., 2014), have been compared and discussed in recent literature (Avila Cobos et al., 2020; Jin and Liu, 2020). For instance, CIBERSORTx extends CIBERSORT to infer cell-type-specific gene expression profiles without physical cell isolation. Detailed information on the deconvolution methods is listed in Table 3. These tools are highly useful in re-analyzing both existing and new bulk RNA-seq datasets to identify and interpret the role of cell-type-specific eQTLs in complex diseases. The most widely used bulk deconvolution methods (i.e., OLS, nnls, RLR, FARDEEP, and CIBERSORT) and the three methods that use the scRNA-seq data as a reference (i.e., DWLS, MuSiC, and SCDC) achieved median RMSE values lower than 0.05 (Avila Cobos et al., 2020).
4.3.3 Batch effect correction and normalization to reduce high technical noise in scRNA-seq
Reducing high technical noise in scRNA-seq data remains a challenge. The noise can arise from differences in the sequencing platform, sequencing depth, amplification bias, RNA capture efficiency, and dropout events. Current noise reduction methods for scRNA-seq data include correcting the batch effect and normalization of the sequencing data. Recently, a comprehensive study evaluated 28 noise reduction methods and tools using 55 real and simulated datasets (Chu et al., 2022). However, it was noted that no single method can be used for all scRNA-seq experiments. The advantages and pitfalls of typical methods for batch effect correction and normalization are listed in Table 4. Therefore, the selection of an appropriate method needs caution and depends on the study design. Additionally, increasing the sample size is a feasible strategy for reducing experimental noise in scRNA-seq.
5 Conclusion and future directions
In conclusion, this review provided an overview of the recent advances in the study of the genetic regulation of gene expression through single-cell eQTL mapping. We also discussed how to perform sc-eQTL mapping and the advantages of scRNA-seq for sc-eQTL mapping and its challenges and limitations. While sc-eQTL analysis is still in its infancy stage, it offers great potential for advancing our understanding of the genetic regulation of gene expression.
In future, the advent of single-cell transcriptomics will lead to significant advancements in the understanding of the genetic regulation of gene expression. sc-eQTL studies have revealed many previously undetected cell-type-specific eQTLs that provide new insights into disease biology. With the decrease in single-cell transcriptomic sequencing costs, sc-eQTL studies will identify new genetic variants that regulate gene expression. Furthermore, the integration of QTL signals from multi-omics at the single-cell level and spatial data can improve the resolution of gene regulation at different omics levels.
Author contributions
JL contributed to the conception and design of the manuscript and the writing and data collection. XW and YC contributed to the writing and data collection. GC, JW, and XS contributed to the writing and editing All authors contributed to the article and approved the submitted version.
Funding
This study was supported by the Key Research and Development Program of Zhejiang (2021C02052) and the Natural Science Foundation of Zhejiang Province (LY20C150004).
Conflict of interest
The authors declare that the research was conducted in the absence of any commercial or financial relationships that could be construed as a potential conflict of interest.
Supplementary material
The Supplementary Material for this article can be found online at: https://www.frontiersin.org/articles/10.3389/fgene.2023.1182579/full#supplementary-material
References
Altboum, Z., Steuerman, Y., David, E., Barnett-Itzhaki, Z., Valadarsky, L., Keren-Shaul, H., et al. (2014). Digital cell quantification identifies global immune cell dynamics during influenza infection. Mol. Syst. Biol. 10 (2), 720. doi:10.1002/msb.134947
Avila Cobos, F., Alquicira-Hernandez, J., Powell, J. E., Mestdagh, P., and De Preter, K. (2020). Benchmarking of cell type deconvolution pipelines for transcriptomics data. Nat. Commun. 11 (1), 5650. doi:10.1038/s41467-020-19015-1
Baron, M., Veres, A., Wolock, S. L., Faust, A. L., Gaujoux, R., Vetere, A., et al. (2016). A single-cell transcriptomic map of the human and mouse pancreas reveals inter- and intra-cell population structure. Cell Syst. 3 (4), 346–360. doi:10.1016/j.cels.2016.08.011
Brennecke, P., Anders, S., Kim, J. K., Kołodziejczyk, A. A., Zhang, X., Proserpio, V., et al. (2013). Accounting for technical noise in single-cell RNA-seq experiments. Nat. Methods 10 (11), 1093–1095. doi:10.1038/nmeth.2645
Brodie, A., Azaria, J. R., and Ofran, Y. (2016). How far from the SNP may the causative genes be? Nucleic Acids Res. 44 (13), 6046–6054. doi:10.1093/nar/gkw500
Bryois, J., Calini, D., Macnair, W., Foo, L., Urich, E., Ortmann, W., et al. (2022). Cell-type-specific cis-eQTLs in eight human brain cell types identify novel risk genes for psychiatric and neurological disorders. Nat. Neurosci. 25 (8), 1104–1112. doi:10.1038/s41593-022-01128-z
J. Chambers, T. Hastie, and D. Pregibon (Editors) (1990). Statistical models in S. Compstat; 1990 1990 (Heidelberg: Physica-Verlag HD). doi:10.1201/9780203738535
Chen, G., Ning, B., and Shi, T. (2019). Single-cell RNA-seq technologies and related computational data analysis. Front. Genet. 10, 317. doi:10.3389/fgene.2019.00317
Chen, W., Zhao, Y., Chen, X., Yang, Z., Xu, X., Bi, Y., et al. (2021). A multicenter study benchmarking single-cell RNA sequencing technologies using reference samples. Nat. Biotechnol. 39 (9), 1103–1114. doi:10.1038/s41587-020-00748-9
Chu, S-K., Zhao, S., Shyr, Y., and Liu, Q. (2022). Comprehensive evaluation of noise reduction methods for single-cell RNA sequencing data. Briefings Bioinforma. 23 (2), bbab565. doi:10.1093/bib/bbab565
Cuomo, A. S. E., Alvari, G., Azodi, C. B., McCarthy, D. J., and Bonder, M. J. (2021). Optimizing expression quantitative trait locus mapping workflows for single-cell studies. Genome Biol. 22 (1), 188. doi:10.1186/s13059-021-02407-x
Cuomo, A. S. E., Seaton, D. D., McCarthy, D. J., Martinez, I., Bonder, M. J., Garcia-Bernardo, J., et al. (2020b). Publisher Correction: Single-cell RNA-sequencing of differentiating iPS cells reveals dynamic genetic effects on gene expression. Nat. Commun. 11 (1), 1572. doi:10.1038/s41467-020-15098-y
Cuomo, A. S. E., Seaton, D. D., McCarthy, D. J., Martinez, I., Bonder, M. J., Garcia-Bernardo, J., et al. (2020a). Single-cell RNA-sequencing of differentiating iPS cells reveals dynamic genetic effects on gene expression. Nat. Commun. 11 (1), 810. doi:10.1038/s41467-020-14457-z
Delmans, M., and Hemberg, M. (2016). Discrete distributional differential expression– (D3E) - a tool for gene expression analysis of single-cell RNA-seq data. BMC Bioinforma. 17 (1), 110. doi:10.1186/s12859-016-0944-6
Dong, M., Thennavan, A., Urrutia, E., Li, Y., Perou, C. M., Zou, F., et al. (2021). Scdc: Bulk gene expression deconvolution by multiple single-cell RNA sequencing references. Briefings Bioinforma. 22 (1), 416–427. doi:10.1093/bib/bbz166
Donovan, M. K. R., D’Antonio-Chronowska, A., D’Antonio, M., and Frazer, K. A. (2020). Cellular deconvolution of GTEx tissues powers discovery of disease and cell-type associated regulatory variants. Nat. Commun. 11 (1), 955. doi:10.1038/s41467-020-14561-0
Du, R., Carey, V., and Weiss, S. T. (2019). deconvSeq: deconvolution of cell mixture distribution in sequencing data. Bioinformatics 35 (24), 5095–5102. doi:10.1093/bioinformatics/btz444
Eraslan, G., Simon, L. M., Mircea, M., Mueller, N. S., and Theis, F. J. (2019). Single-cell RNA-seq denoising using a deep count autoencoder. Nat. Commun. 10 (1), 390. doi:10.1038/s41467-018-07931-2
Fairfax, B. P., Makino, S., Radhakrishnan, J., Plant, K., Leslie, S., Dilthey, A., et al. (2012). Genetics of gene expression in primary immune cells identifies cell type–specific master regulators and roles of HLA alleles. Nat. Genet. 44 (5), 502–510. doi:10.1038/ng.2205
Favé, M-J., Lamaze, F. C., Soave, D., Hodgkinson, A., Gauvin, H., Bruat, V., et al. (2018). Gene-by-environment interactions in urban populations modulate risk phenotypes. Nat. Commun. 9 (1), 827. doi:10.1038/s41467-018-03202-2
Friedman, J., Hastie, T., and Tibshirani, R. (2010). Regularization paths for generalized linear models via coordinate descent. J. Stat. Softw. 33 (1), 1–22. doi:10.18637/jss.v033.i01
Gatti, D. M., Sypa, M., Rusyn, I., Wright, F. A., and Barry, W. T. (2009). Safegui: Resampling-based tests of categorical significance in gene expression data made easy. Bioinformatics 25 (4), 541–542. doi:10.1093/bioinformatics/btn655
Gaujoux, R., and Seoighe, C. (2013). CellMix: A comprehensive toolbox for gene expression deconvolution. Bioinformatics 29 (17), 2211–2212. doi:10.1093/bioinformatics/btt351
Gewirtz, A. D., Townes, F. W., and Engelhardt, B. E. (2022). Expression QTLs in single-cell sequencing data. bioRxiv. doi:10.1101/2022.08.14.503915
Gong, T., and Szustakowski, J. D. (2013). DeconRNASeq: A statistical framework for deconvolution of heterogeneous tissue samples based on mRNA-seq data. Bioinformatics 29 (8), 1083–1085. doi:10.1093/bioinformatics/btt090
Grün, D., Lyubimova, A., Kester, L., Wiebrands, K., Basak, O., Sasaki, N., et al. (2015). Single-cell messenger RNA sequencing reveals rare intestinal cell types. Nature 525 (7568), 251–255. doi:10.1038/nature14966
Guerrero-Juarez, C. F., Dedhia, P. H., Jin, S., Ruiz-Vega, R., Ma, D., Liu, Y., et al. (2019). Single-cell analysis reveals fibroblast heterogeneity and myeloid-derived adipocyte progenitors in murine skin wounds. Nat. Commun. 10 (1), 650. doi:10.1038/s41467-018-08247-x
Guo, M., Wang, H., Potter, S. S., Whitsett, J. A., and Xu, Y. (2015). Sincera: A pipeline for single-cell RNA-seq profiling analysis. PLoS Comput. Biol. 11 (11), e1004575. doi:10.1371/journal.pcbi.1004575
Haghverdi, L., Lun, A. T. L., Morgan, M. D., and Marioni, J. C. (2018). Batch effects in single-cell RNA-sequencing data are corrected by matching mutual nearest neighbors. Nat. Biotechnol. 36 (5), 421–427. doi:10.1038/nbt.4091
Hao, Y., Hao, S., Andersen-Nissen, E., Mauck, W. M., Zheng, S., Butler, A., et al. (2021). Integrated analysis of multimodal single-cell data. Cell 184 (13), 3573–3587.e29. doi:10.1016/j.cell.2021.04.048
Hao, Y., Yan, M., Heath, B. R., Lei, Y. L., and Xie, Y. (2019). Fast and robust deconvolution of tumor infiltrating lymphocyte from expression profiles using least trimmed squares. PLoS Comput. Biol. 15 (5), e1006976. doi:10.1371/journal.pcbi.1006976
Hernández, P. P., Strzelecka, P. M., Athanasiadis, E. I., Hall, D., Robalo, A. F., Collins, C. M., et al. (2018). Single-cell transcriptional analysis reveals ILC-like cells in zebrafish. Sci. Immunol. 3 (29), eaau5265. doi:10.1126/sciimmunol.aau5265
Hie, B., Bryson, B., and Berger, B. (2019). Efficient integration of heterogeneous single-cell transcriptomes using Scanorama. Nat. Biotechnol. 37 (6), 685–691. doi:10.1038/s41587-019-0113-3
Hu, Y., Xi, X., Yang, Q., and Zhang, X. (2020). SCeQTL: an R package for identifying eQTL from single-cell parallel sequencing data. BMC Bioinforma. 21 (1), 184. doi:10.1186/s12859-020-3534-651
Hunt, G. J., Freytag, S., Bahlo, M., and Gagnon-Bartsch, J. A. (2019). dtangle: accurate and robust cell type deconvolution. Bioinformatics 35 (12), 2093–2099. doi:10.1093/bioinformatics/bty926
Ishigaki, K., Kochi, Y., Suzuki, A., Tsuchida, Y., Tsuchiya, H., Sumitomo, S., et al. (2017). Polygenic burdens on cell-specific pathways underlie the risk of rheumatoid arthritis. Nat. Genet. 49 (7), 1120–1125. doi:10.1038/ng.3885
Jew, B., Alvarez, M., Rahmani, E., Miao, Z., Ko, A., Garske, K. M., et al. (2020). Accurate estimation of cell composition in bulk expression through robust integration of single-cell information. Nat. Commun. 11 (1), 1971. doi:10.1038/s41467-020-15816-6
Ji, Z., and Ji, H. (2016). Tscan: Pseudo-time reconstruction and evaluation in single-cell RNA-seq analysis. Nucleic Acids Res. 44 (13), e117. doi:10.1093/nar/gkw430
Jiang, L., Chen, H., Pinello, L., and Yuan, G. C. (2016). GiniClust: Detecting rare cell types from single-cell gene expression data with Gini index. Genome Biol. 17 (1), 144. doi:10.1186/s13059-016-1010-4
Jin, H., and Liu, Z. (2020). A comparative study of deconvolution methods for RNA-seq data under a dynamic testing landscape. bioRxiv, 418640. doi:10.1101/2020.12.09.418640
Johnson, W. E., Li, C., and Rabinovic, A. (2007). Adjusting batch effects in microarray expression data using empirical Bayes methods. Biostatistics 8 (1), 118–127. doi:10.1093/biostatistics/kxj037
Kang, H. M., Subramaniam, M., Targ, S., Nguyen, M., Maliskova, L., McCarthy, E., et al. (2018). Multiplexed droplet single-cell RNA-sequencing using natural genetic variation. Nat. Biotechnol. 36 (1), 89–94. doi:10.1038/nbt.4042
Karamitros, D., Stoilova, B., Aboukhalil, Z., Hamey, F., Reinisch, A., Samitsch, M., et al. (2018). Single-cell analysis reveals the continuum of human lympho-myeloid progenitor cells. Nat. Immunol. 19 (1), 85–97. doi:10.1038/s41590-017-0001-2
Kilpinen, H., Goncalves, A., Leha, A., Afzal, V., Alasoo, K., Ashford, S., et al. (2017). Common genetic variation drives molecular heterogeneity in human iPSCs. Nature 546 (7658), 370–375. doi:10.1038/nature22403
Kim-Hellmuth, S., Aguet, F., Oliva, M., Muoz-Aguirre, M., Kasela, S., Wucher, V., et al. (2020). Cell type–specific genetic regulation of gene expression across human tissues. Science 369 (6509), eaaz8528. doi:10.1126/science.aaz8528
Kiselev, V. Y., Andrews, T. S., and Hemberg, M. (2019). Challenges in unsupervised clustering of single-cell RNA-seq data. Nat. Rev. Genet. 20 (5), 273–282. doi:10.1038/s41576-018-0088-9
Kiselev, V. Y., Kirschner, K., Schaub, M. T., Andrews, T., Yiu, A., Chandra, T., et al. (2017). SC3: Consensus clustering of single-cell RNA-seq data. Nat. Methods 14 (5), 483–486. doi:10.1038/nmeth.4236
Korsunsky, I., Millard, N., Fan, J., Slowikowski, K., Zhang, F., Wei, K., et al. (2019). Fast, sensitive and accurate integration of single-cell data with Harmony. Nat. Methods 16 (12), 1289–1296. doi:10.1038/s41592-019-0619-0
Knowles, D. A., Davis, J. R., Edgington, H., Raj, A., Favé, M-J., Zhu, X., et al. (2017). Allele-specific expression reveals interactions between genetic variation and environment. Nat. Methods 14 (7), 699–702. doi:10.1038/nmeth.4298
Levine, J. H., Simonds, E. F., Bendall, S. C., Davis, K. L., Amir el, A. D., Tadmor, M. D., et al. (2015). Data-driven phenotypic dissection of AML reveals progenitor-like cells that correlate with prognosis. Cell 162 (1), 184–197. doi:10.1016/j.cell.2015.05.047
Li, B., Severson, E., Pignon, J. C., Zhao, H., Li, T., Novak, J., et al. (2016). Comprehensive analyses of tumor immunity: Implications for cancer immunotherapy. Genome Biol. 17 (1), 174. doi:10.1186/s13059-016-1028-7
Liebner, D. A., Huang, K., and Parvin, J. D. (2014). Mmad: Microarray microdissection with analysis of differences is a computational tool for deconvoluting cell type-specific contributions from tissue samples. Bioinformatics 30 (5), 682–689. doi:10.1093/bioinformatics/btt566
Lin, P., Troup, M., and Ho, J. W. (2017). Cidr: Ultrafast and accurate clustering through imputation for single-cell RNA-seq data. Genome Biol. 18 (1), 59. doi:10.1186/s13059-017-1188-0
Liu, J., Gao, C., Sodicoff, J., Kozareva, V., Macosko, E. Z., and Welch, J. D. (2020). Jointly defining cell types from multiple single-cell datasets using LIGER. Nat. Protoc. 15 (11), 3632–3662. doi:10.1038/s41596-020-0391-8
Lopez, R., Regier, J., Cole, M. B., Jordan, M. I., and Yosef, N. (2018). Deep generative modeling for single-cell transcriptomics. Nat. Methods 15 (12), 1053–1058. doi:10.1038/s41592-018-0229-2
Lotfollahi, M., Wolf, F. A., and Theis, F. J. (2019). scGen predicts single-cell perturbation responses. Nat. Methods 16 (8), 715–721. doi:10.1038/s41592-019-0494-8
Lu, A., Thompson, M., Gordon, M. G., Dahl, A., Ye, C. J., Zaitlen, N., et al. (2021). Fast and powerful statistical method for context-specific QTL mapping in multi-context genomic studies. bioRxiv. doi:10.1101/2021.06.17.448889
Luecken, M. D., and Theis, F. J. (2019). Current best practices in single-cell RNA-seq analysis: A tutorial. Mol. Syst. Biol. 15 (6), e8746. doi:10.15252/msb.20188746
Ma, T., Li, H., and Zhang, X. (2022). Discovering single-cell eQTLs from scRNA-seq data only. Gene 829, 146520. doi:10.1016/j.gene.2022.146520
Macosko, E. Z., Basu, A., Satija, R., Nemesh, J., Shekhar, K., Goldman, M., et al. (2015). Highly parallel genome-wide expression profiling of individual cells using nanoliter droplets. Cell 161 (5), 1202–1214. doi:10.1016/j.cell.2015.05.002
Mandric, I., Schwarz, T., Majumdar, A., Hou, K., Briscoe, L., Perez, R., et al. (2020). Optimized design of single-cell RNA sequencing experiments for cell-type-specific eQTL analysis. Nat. Commun. 11 (1), 5504. doi:10.1038/s41467-020-19365-w
McCarthy, D. J., Campbell, K. R., Lun, A. T. L., and Wills, Q. F. (2017). Scater: Pre-processing, quality control, normalization and visualization of single-cell RNA-seq data in R. Bioinformatics 33 (8), 1179–1186. doi:10.1093/bioinformatics/btw777
Miao, Z., Deng, K., Wang, X., and Zhang, X. (2018). DEsingle for detecting three types of differential expression in single-cell RNA-seq data. Bioinformatics 34 (18), 3223–3224. doi:10.1093/bioinformatics/bty332
Mullen, K. M., and Stokkum, I. H. M. (2012). nnls: the Lawson-Hanson algorithm for non-negative least squares (NNLS). R Package Version 14.
Nathan, A., Asgari, S., Ishigaki, K., Valencia, C., Amariuta, T., Luo, Y., et al. (2022). Single-cell eQTL models reveal dynamic T cell state dependence of disease loci. Nature 606 (7912), 120–128. doi:10.1038/s41586-022-04713-1
Neavin, D., Nguyen, Q., Daniszewski, M. S., Liang, H. H., Chiu, H. S., Wee, Y. K., et al. (2021). Single cell eQTL analysis identifies cell type-specific genetic control of gene expression in fibroblasts and reprogrammed induced pluripotent stem cells. Genome Biol. 22 (1), 76. doi:10.1186/s13059-021-02293-3
Newman, A. M., Liu, C. L., Green, M. R., Gentles, A. J., Feng, W., Xu, Y., et al. (2015). Robust enumeration of cell subsets from tissue expression profiles. Nat. Methods 12 (5), 453–457. doi:10.1038/nmeth.3337
Newman, A. M., Steen, C. B., Liu, C. L., Gentles, A. J., Chaudhuri, A. A., Scherer, F., et al. (2019). Determining cell type abundance and expression from bulk tissues with digital cytometry. Nat. Biotechnol. 37 (7), 773–782. doi:10.1038/s41587-019-0114-2
Ota, M., Nagafuchi, Y., Hatano, H., Ishigaki, K., Terao, C., Takeshima, Y., et al. (2021). Dynamic landscape of immune cell-specific gene regulation in immune-mediated diseases. Cell 184 (11), 3006–3021.e17. doi:10.1016/j.cell.2021.03.056
Perez, R. K., Gordon, M. G., Subramaniam, M., Kim, M. C., Hartoularos, G. C., Targ, S., et al. (2022). Single-cell RNA-seq reveals cell type-specific molecular and genetic associations to lupus. Science 376 (6589), eabf1970. doi:10.1126/science.abf1970
Polański, K., Young, M. D., Miao, Z., Meyer, K. B., Teichmann, S. A., and Park, J. E. (2020). BBKNN: Fast batch alignment of single cell transcriptomes. Bioinformatics 36 (3), 964–965. doi:10.1093/bioinformatics/btz625
Qi, J., Foroughi Asl, H., Björkegren, J., and Michoel, T. (2014). kruX: matrix-based non-parametric eQTL discovery. BMC Bioinforma. 15 (1), 11. doi:10.1186/1471-2105-15-11
Qin, Y., Huttlin, E. L., Winsnes, C. F., Gosztyla, M. L., Wacheul, L., Kelly, M. R., et al. (2021). A multi-scale map of cell structure fusing protein images and interactions. Nature 600 (7889), 536–542. doi:10.1038/s41586-021-04115-9
Racle, J., de Jonge, K., Baumgaertner, P., Speiser, D. E., and Gfeller, D. (2017). Simultaneous enumeration of cancer and immune cell types from bulk tumor gene expression data. eLife 6, e26476. doi:10.7554/eLife.26476
Ripley, B., Venables, B., Bates, D. M., Hornik, K., Gebhardt, A., and Firth, D. (2022). Support functions and datasets for venables and ripley's MASS [R package MASS version 7, 3–58.
Risso, D., Perraudeau, F., Gribkova, S., Dudoit, S., and Vert, J.-P. (2018). A general and flexible method for signal extraction from single-cell RNA-seq data. Nat. Commun. 9 (1), 284. doi:10.1038/s41467-017-02554-5
Ritchie, M. E., Phipson, B., Wu, D., Hu, Y., Law, C. W., Shi, W., et al. (2015). Limma powers differential expression analyses for RNA-sequencing and microarray studies. Nucleic Acids Res. 43 (7), e47–e47. doi:10.1093/nar/gkv007
Sarkar, A. K., Tung, P. Y., Blischak, J. D., Burnett, J. E., Li, Y. I., Stephens, M., et al. (2019). Discovery and characterization of variance QTLs in human induced pluripotent stem cells. PLoS Genet. 15, e1008045. doi:10.1371/journal.pgen.1008045
Shabalin, A. A. (2012). Matrix eQTL: Ultra fast eQTL analysis via large matrix operations. Bioinformatics 28 (10), 1353–1358. doi:10.1093/bioinformatics/bts163
Svensson, V., Natarajan, K. N., Ly, L. H., Miragaia, R. J., Labalette, C., Macaulay, L. C., et al. (2017). Power analysis of single-cell RNA-sequencing experiments. Nat. Methods 14, 381–387. doi:10.1038/nmeth.4220
Teng, M., Love, M. I., Davis, C. A., Djebali, S., Dobin, A., Graveley, B. R., et al. (2016). A benchmark for RNA-seq quantification pipelines. Genome Biol. 17 (1), 74. doi:10.1186/s13059-016-0940-1
Tran, H. T. N., Ang, K. S., Chevrier, M., Zhang, X. M., Lee, N. Y. S., Goh, M., et al. (2020). A benchmark of batch-effect correction methods for single-cell RNA sequencing data. Genome Biol. 21, 12. doi:10.1186/s13059-019-1850-9
Tsafrir, D., Tsafrir, I., Ein-Dor, L., Zuk, O., Notterman, D. A., and Domany, E. (2005). Sorting points into neighborhoods (SPIN): Data analysis and visualization by ordering distance matrices. Bioinformatics 21 (10), 2301–2308. doi:10.1093/bioinformatics/bti329
Tsoucas, D., Dong, R., Chen, H., Zhu, Q., Guo, G., and Yuan, G-C. (2019). Accurate estimation of cell-type composition from gene expression data. Nat. Commun. 10 (1), 2975. doi:10.1038/s41467-019-10802-z
Tsoucas, D., and Yuan, G-C. (2018). GiniClust2: A cluster-aware, weighted ensemble clustering method for cell-type detection. Genome Biol. 19 (1), 58. doi:10.1186/s13059-018-1431-3
Umans, B. D., Battle, A., and Gilad, Y. (2020). Where are the disease-associated eQTLs? Trends Genet. 37 (2), 109–124. doi:10.1016/j.tig.2020.08.009
van der Wijst, M. G. P., Brugge, H., de Vries, D. H., Deelen, P., Swertz, M. A., Franke, L., et al. (2018). Single-cell RNA sequencing identifies celltype-specific cis-eQTLs and co-expression QTLs. Nat. Genet. 50 (4), 493–497. doi:10.1038/s41588-018-0089-9
Van Der Wijst, M. G. P., De Vries, D. H., Groot, H. E., Trynka, G., Hon, C. C., Bonder, M. J., et al. (2020). The single-cell eQTLGen consortium. elife 9, e52155. doi:10.7554/eLife.52155
Vieth, B., Parekh, S., Ziegenhain, C., Enard, W., and Hellmann, I. (2019). A systematic evaluation of single cell RNA-seq analysis pipelines. Nat. Commun. 10 (1), 4667. doi:10.1038/s41467-019-12266-7
Villani, A-C., Satija, R., Reynolds, G., Sarkizova, S., Shekhar, K., Fletcher, J., et al. (2017). Single-cell RNA-seq reveals new types of human blood dendritic cells, monocytes, and progenitors. Science 356 (6335), eaah4573. doi:10.1126/science.aah4573
Visscher, P. M., Wray, N. R., Zhang, Q., Sklar, P., McCarthy, M. I., Brown, M. A., et al. (2017). 10 Years of GWAS discovery: Biology, function, and translation. Am. J. Hum. Genet. 101 (1), 5–22. doi:10.1016/j.ajhg.2017.06.005
Wang, B., Ramazzotti, D., De Sano, L., Zhu, J., Pierson, E., and Batzoglou, S. (2018). Simlr: A tool for large-scale genomic analyses by multi-kernel learning. Proteomics 18 (2), 1700232. doi:10.1002/pmic.201700232
Wang, X., Park, J., Susztak, K., Zhang, N. R., and Li, M. (2019). Bulk tissue cell type deconvolution with multi-subject single-cell expression reference. Nat. Commun. 10 (1), 380. doi:10.1038/s41467-018-08023-x
Wegmann, R., Neri, M., Schuierer, S., Bilican, B., Hartkopf, H., Nigsch, F., et al. (2019). CellSIUS provides sensitive and specific detection of rare cell populations from complex single-cell RNA-seq data. Genome Biol. 20 (1), 142. doi:10.1186/s13059-019-1739-7
Wills, Q. F., Livak, K. J., Tipping, A. J., Enver, T., Goldson, A. J., Sexton, D. W., et al. (2013). Single-cell gene expression analysis reveals genetic associations masked in whole-tissue experiments. Nat. Biotechnol. 31 (8), 748–752. doi:10.1038/nbt.2642
Wolf, F. A., Angerer, P., and Theis, F. J. (2018). Scanpy: Large-scale single-cell gene expression data analysis. Genome Biol. 19 (1), 15. doi:10.1186/s13059-017-1382-0
Xue, A., Yazar, S., Neavin, D., and Powell, J. E. (2023). Pitfalls and opportunities for applying latent variables in single-cell eQTL analyses. Genome Biolology 24 (1), 33. doi:10.1186/s13059-023-02873-5
Yao, Z., van Velthoven, C. T. J., Nguyen, T. N., Goldy, J., Sedeno-Cortes, A. E., Baftizadeh, F., et al. (2021). A taxonomy of transcriptomic cell types across the isocortex and hippocampal formation. Cell 184 (12), 3222–3241.e26. doi:10.1016/j.cell.2021.04.021
Yazar, S., Alquicira-Hernandez, J., Wing, K., Senabouth, A., Gordon, M. G., Andersen, S., et al. (2022). Single-cell eQTL mapping identifies cell type–specific genetic control of autoimmune disease. Science 376 (6589), eabf3041. doi:10.1126/science.abf3041
Yazar, S., and Powell, J. E. (2022). Single-cell expression quantitative trait loci: T-Cell immunology teams up with statistical genetics. Immunol. Cell Biol. 100 (8), 588–590. doi:10.1111/imcb.12577
Zeisel, A., Muñoz-Manchado, A. B., Codeluppi, S., Lönnerberg, P., La Manno, G., Juréus, A., et al. (2015). Brain structure. Cell types in the mouse cortex and hippocampus revealed by single-cell RNA-seq. Science 347 (6226), 1138–1142. doi:10.1126/science.aaa1934
Zhang, T., Choi, J., Kovacs, M. A., Shi, J., Xu, M., Goldstein, A. M., et al. (2018). Cell-type-specific eQTL of primary melanocytes facilitates identification of melanoma susceptibility genes. Genome Res. 28 (11), 1621–1635. doi:10.1101/gr.233304.117
Zhernakova, D. V., Deelen, P., Vermaat, M., van Iterson, M., van Galen, M., Arindrarto, W., et al. (2017). Identification of context-dependent expression quantitative trait loci in whole blood. Nat. Genet. 49 (1), 139–145. doi:10.1038/ng.3737
Zhong, Y., Wan, Y. W., Pang, K., Chow, L. M., and Liu, Z. (2013). Digital sorting of complex tissues for cell type-specific gene expression profiles. BMC Bioinforma. 14, 89. doi:10.1186/1471-2105-14-89
Keywords: sc-eQTL, cell-type-specific, genetic variants, scRNA-seq, bulk RNA-seq
Citation: Luo J, Wu X, Cheng Y, Chen G, Wang J and Song X (2023) Expression quantitative trait locus studies in the era of single-cell omics. Front. Genet. 14:1182579. doi: 10.3389/fgene.2023.1182579
Received: 10 March 2023; Accepted: 26 April 2023;
Published: 22 May 2023.
Edited by:
Shizhong Xu, University of California, Riverside, United StatesReviewed by:
Marc Jan Bonder, European Molecular Biology Laboratory Heidelberg, GermanyMaud Fagny, Institut National de recherche pour l’agriculture, l’alimentation et l’environnement (INRAE), France
Copyright © 2023 Luo, Wu, Cheng, Chen, Wang and Song. This is an open-access article distributed under the terms of the Creative Commons Attribution License (CC BY). The use, distribution or reproduction in other forums is permitted, provided the original author(s) and the copyright owner(s) are credited and that the original publication in this journal is cited, in accordance with accepted academic practice. No use, distribution or reproduction is permitted which does not comply with these terms.
*Correspondence: Jie Luo, bHVvamllQG1haWwuemFhcy5hYy5jbg==