- 1College of Animal Science and Technology, China Agricultural University, Beijing, China
- 2CAS Key Laboratory of Animal Ecology and Conservation Biology, Institute of Zoology, Chinese Academy of Sciences (CAS), Beijing, China
- 3University of Chinese Academy of Sciences (UCAS), Beijing, China
Goats with diverse economic phenotypic traits play an important role in animal husbandry. However, the genetic mechanisms underlying complex phenotypic traits are unclear in goats. Genomic studies of variations provided a lens to identify functional genes. In this study, we focused on the worldwide goat breeds with outstanding traits and used whole-genome resequencing data in 361 samples from 68 breeds to detect genomic selection sweep regions. We identified 210–531 genomic regions with six phenotypic traits, respectively. Further gene annotation analysis revealed 332, 203, 164, 300, 205, and 145 candidate genes corresponding with dairy, wool, high prolificacy, poll, big ear, and white coat color traits. Some of these genes have been reported previously (e.g., KIT, KITLG, NBEA, RELL1, AHCY, and EDNRA), while we also discovered novel genes, such as STIM1, NRXN1, LEP, that may be associated with agronomic traits like poll and big ear morphology. Our study found a set of new genetic markers for genetic improvement in goats and provided novel insights into the genetic mechanisms of complex traits.
Introduction
As one of the earliest domesticated animals, goats profoundly affect human society (Porter, 1996; Pringle, 1998). Then, human beings reshaped the morphology, physiology, and behavior of goats by domestication, selection, and dispersal with humans (Larson and Fuller, 2014). Goats have formed a wealth of breed resources adapted to different natural environments and human needs in the past 10,000 years (Pereira and Amorim, 2010; Zheng et al., 2020), exhibited specialized phenotypes (e.g., coat color, horn, ear), and provided diverse productions (e.g., milk, fiber) (Skapetas and Bampidis, 2016). For the animals, those traits selected in a specific direction may be imprinted obvious characteristics in the genome (Grossman et al., 2010; Li et al., 2020; Mariadassou et al., 2020; LI et al., 2022; Seo et al., 2022).
Recent studies by integrating whole genome datasets and ancient DNA information have identified candidate genome regions and genes during goat domestication (Alberto et al., 2018; Daly et al., 2018; Zheng et al., 2020). The availability of whole-genome datasets provides a lens to uncover the genetic mechanism underlying phenotypic traits. Many candidate genes or selective regions were identified in recent studies by selective sweep or whole-genome association analysis (GWAS) using re-sequencing datasets and whole-genome SNPs arrays (Guo et al., 2018; Islam et al., 2020; Luigi-Sierra et al., 2020; Talouarn et al., 2020; Wang K. et al., 2020; Gu et al., 2022). Nevertheless, most of those studies focused on given breeds or included breeds restricted in geographic regions, and little is known regarding the genetic mechanisms of diverse phenotypic traits of goats on a worldwide scale. Here, we collected samples on the global scale with various phenotypes and aimed to conduct a genome-wide analysis to identify genomic regions associated with the phenotypic traits underlying recently strong selection. The identification of genes associated with varied agronomic traits across worldwide goats facilitates prospective molecular breeding endeavors.
Materials and methods
Genotypic and phenotypic data
We collected 361 samples including 68 domestic goat breeds with typical phenotypic traits (Supplementary Table S1). Whole-genome resequencing datasets of 361 samples were retrieved from the National Center for Biotechnology Information (NCBI) (Supplementary Table S1). The raw reads were filtered with Trimmomatic v0.39 (Bolger et al., 2014), and filtered reads were aligned to the goat reference genome (ARS1) by the Burrows-Wheeler Aligner v0.7.17 (Li and Durbin, 2009) with default parameters. Then we carried out the GATK Best Practices Workflows to call short variations. We filtered duplicates by the MarkDuplicates module with Picard v2.18.12 (http://broadinstitute.github.io/picard/) and detected short variations using the GATK v4.2.3.0 HaplotypeCaller module (McKenna et al., 2010) in individual level. The raw GVCF files of each sample were merged using the CombineGVCFs and detected for short variations using the GenotypeGVCFs. In this study, we only selected SNP by the SelectVariants module in GATK. The raw SNPs were firstly filtered by VariantFiltering module of the GATK with the parameters “QUAL <30.0 || QD < 2.0 || MQ < 40.0 || FS > 60.0 || SOR >3.0 || MQRankSum < −12.5 || ReadPosRankSum < −8.0”. We further identified high quality SNPs using the following criteria: (i) biallelic SNPs, (ii) autosome SNPs, (iii) minor allele frequency (MAF) > 0.05, (iv) call rate >90%. The above analyses were performed by VCFtools v0.1.14 (Danecek et al., 2011).
The total 361 samples were classified into six pairs of populations according to six important economic traits (Table 1). 88 dairy type individuals, 19 non-dairy type individuals, 24 wool type individuals, 22 wild type individuals, 14 high prolificacy individuals, 25 low prolificacy individuals, 31 poll individuals, 15 horn individuals, 14 big ear individuals, 22 small ear individuals, 45 white coat individuals, and 37 black coat individuals were obtained (Table 1).
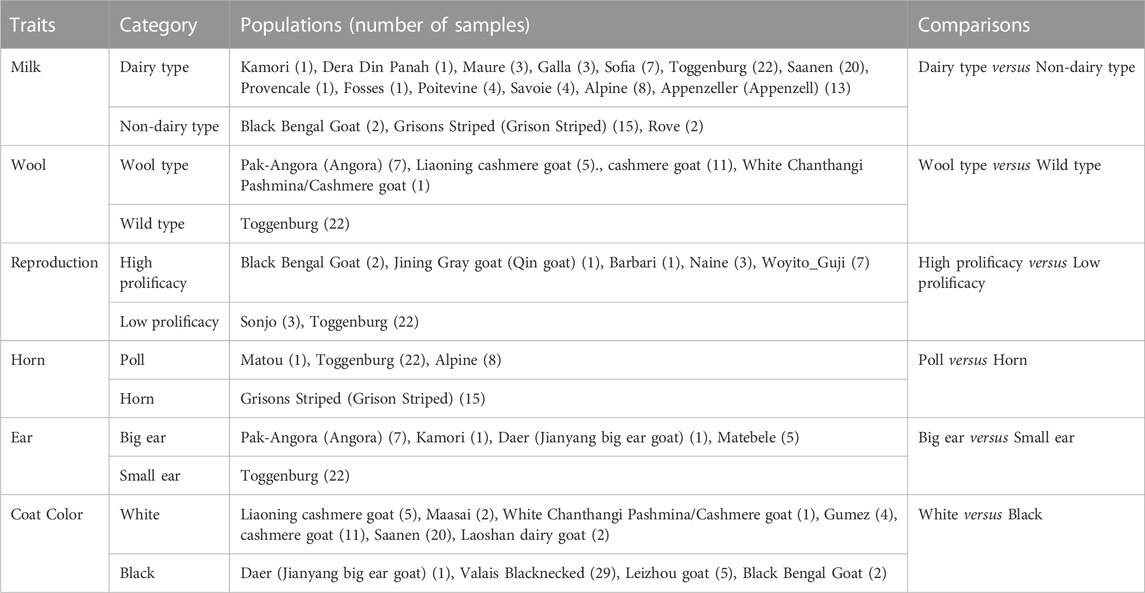
TABLE 1. Information from worldwide goat breeds used to detect selective signals associated with specific traits.
Genomic selection signals analysis
To identify the genomic signatures of selection in domestic goats with special phenotypes, we carried out two approaches. We calculated the pairwise FST values (Weir and Cockerham, 1984) between pairwise populations with contrasting phenotypes (Table 1). Further, we calculated ln (θπ ratios) (θπ-Control/θπ-Case) of pairwise populations with contrasting phenotypes to detect changes in genetic diversity due to artificial selection (Yang et al., 2016). The above analyses were all performed by VCFtools v0.1.14 (Danecek et al., 2011) with a 50 kb sliding window and a 25 kb sliding step across chromosomes. The top 5% FST values and θπ ratios were considered as candidate selective sweeps regions.
Candidate gene analysis
The candidate genomic regions were annotated using SNPeff v.5.1 (Cingolani et al., 2012) based on the goat reference genome (ARS1). Gene Ontology (GO) term enrichment and Kyoto Encyclopedia of Genes and Genomes (KEGG) pathway analyses of a candidate gene set were carried out by a statistical overrepresentation test with the default setting using the clusterProfiler 4.0 package in the R program (Wu et al., 2021). Categories with the threshold of adjusted p-value <0.05 after the Bonferroni correction were defined as significantly enriched terms and pathways.
Results and discussion
We obtained 28.56 million SNPs after filtering in 361 samples. We implemented selection screening in 6 pairs of populations (Table 1). 515, 303, 210, 531, 261, and 217 genomic regions were detected based on the overlap of the top 5% θπratio and FST for milk, wool, reproduction, horn, ear, and coat color traits, respectively. We further annotated those genomic regions and detected a set of novel and previously reported functional genes (Figures 1, 2; Supplementary Figure S1-S4; Supplementary Table S2-S7). 332 genes within 307 candidate selective sweep regions were identified to be associated with dairy traits (Supplementary Table S2). LPL was found to be associated with milk fat content in goats (Badaoui et al., 2007). Additionally, BCAR3, ART3, GABRG2, JAK2, and ABTB2 were functionally associated with milk traits in cows (Gao et al., 2017; Khan et al., 2019; Zhou et al., 2019; Peters et al., 2021; Buaban et al., 2022). Similarly, MRPL47, ACTL6A, NDUFB5, and BDH1 were identified as being related to milk yield in buffalo (El-Halawany et al., 2017; Du et al., 2019). We further investigated the functions of those genes by GO and KEGG analyses and found a significant overrepresentation of genes involved in GO terms, such as transporter complex, transmembrane transporter complex, and ion channel complex (Figure 3A). The GO term “ion channel” involved in the modulation of milk production by controlling mammary gland fluid flow in dairy cows (Cai et al., 2018). In the wool type versus wild type pair population, 203 genes within 188 candidate genomic regions were annotated and may be involved in the wool trait regulation mechanism (Supplementary Table S3). One of the strongest selective sweep signatures was situated in (neurobachin) NBEA, which encodes a neuron-specific multidomain protein of 327 kDa (Wang et al., 2000). Although the gene was a novel gene for goats, it had been reported to be associated with wool production in Chinese merino sheep (Wang et al., 2014). We detected a significant overrepresentation of genes in three GO and one KEGG terms involved Phospholipase C (PLC) activity (Figure 3B), and the reduction of PLC activity led to downregulation of keratin expression in mice hair, resulting in hair hypotrichosis and diverse hair anomalies (Nakamura et al., 2003; Nakamura et al., 2008). For the high prolificacy versus low prolificacy pair population, we obtained 164 functional genes within 142 genomic regions (Supplementary Table S4). RELL1, KIT, and KITLG were identified as candidate genes associated with prolificacy (Supplementary Figure S2). RELL1 was reported to be associated with the number of stillborn in pigs (Onteru et al., 2012), the single nucleotide polymorphisms (SNPs) in KIT and KITLG were found to be associated with the litter size of goats and sheep (An et al., 2012; Yuan et al., 2019; Wang et al., 2020a). Further analysis showed the significant overrepresentation of genes in 12 GO terms, which are mainly associated with Second-messenger-mediated signaling and the activity of phospholipase (Supplementary Table S5A). The cyclic AMP governs the synthesis and secretion of reproductive hormones, particularly gonadotropins (Mukherjee and Mayo, 2000), and mediates the impacts of follicle-stimulating hormone and luteinizing hormone on the development of ovarian follicles and ovulation (Riccetti et al., 2018). 300 candidate genes were identified to be associated with poll traits (Supplementary Table S5). The highest peak of θπratio and FST is situated in STIM1 and NRXN1, with STIM1 involved in store-operated Ca2+ channels (Roos et al., 2005) and NRXN1 binding neuroligins to form (Ca2+)-dependent neurexin/neuroligin complexes (Siddiqui et al., 2010) (Supplementary Figure S2). They both likely play important roles in neurodevelopment and behavior (Ching et al., 2010; Zheng et al., 2020). Further, GO enrichment analysis suggested a significant overrepresentation of genes involved in enamel mineralization (Supplementary Figure S5B). Enamel mineralization is involved in the process of producing and fortifying tooth enamel, the outermost layer of the tooth, which serves as a protective barrier (Vaissier Welborn, 2020), which may also have a correlation with the development and formation of the horn. In the big ear versus small ear goats, 205 genes within 188 genomic regions were identified to be associated with the big ear trait (Supplementary Table S6). In addition, we found a novel gene with big ear morphology in the goat. Furthermore, we detected a significant overrepresentation of genes involved in G protein−coupled purinergic nucleotide receptor activity, purinergic nucleotide receptor activity, and nucleotide receptor activity (Supplementary Figure S5C), potentially exerting an influence on ear morphology. Overall, 145 genes within 146 genomic regions were identified in the white coat color versus black coat color pair population (Supplementary Table S7). The two genes AHCY, and EDNRA were identified to be involved in the regulation of coat color (Menzi et al., 2016; Nazari-Ghadikolaei et al., 2018) (Supplementary Figure S4). We also detected novel genes involved with coat colors in goats, such as GPR22, SOX5, CLEC12B, AIM1, ITFG1, and LDLRAD4 (Table 2).
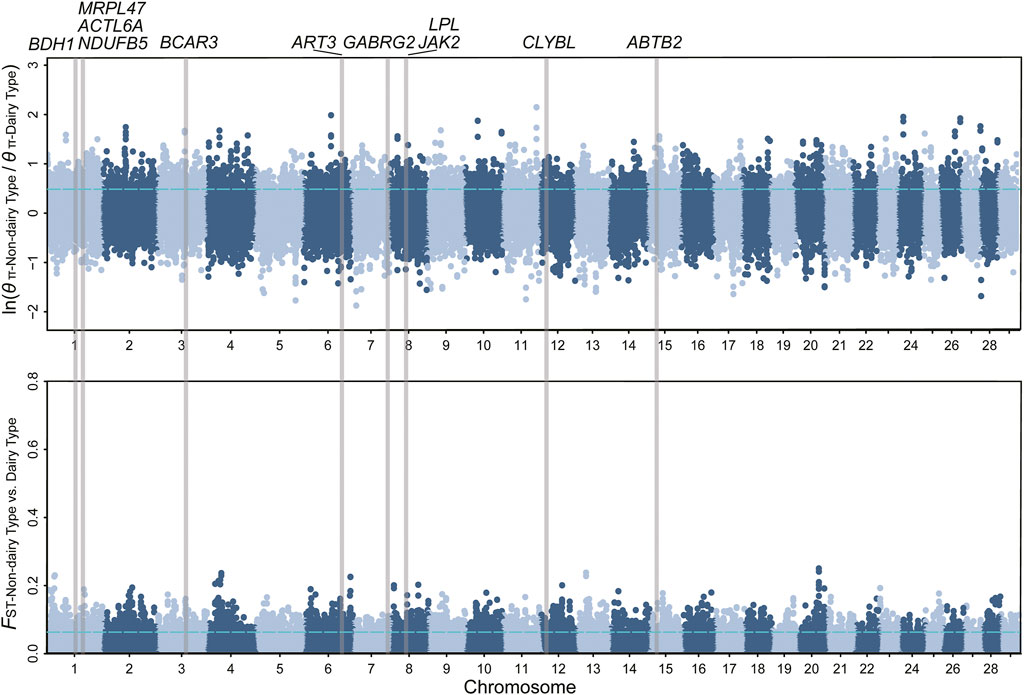
FIGURE 1. Manhattan plot of θπ ratios and FST for milk traits. The vertical gray thick lines indicated the position of selected genes detected in our study. The values of the top 5% threshold (FST = 0.063, θπ ratio = 0.488) are denoted by blue horizontal dashed lines.
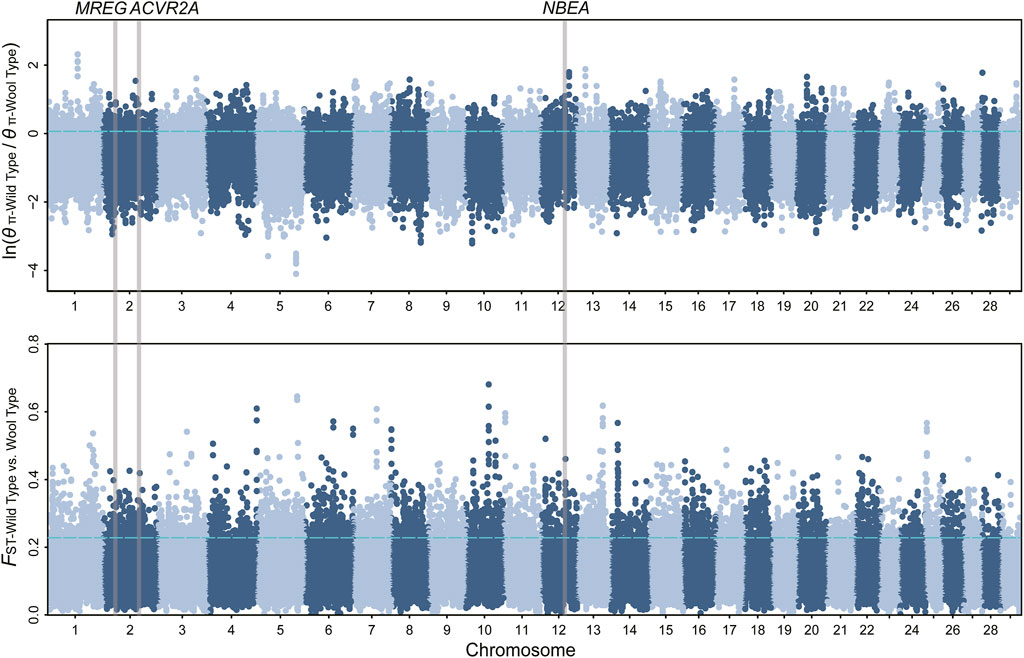
FIGURE 2. Manhattan plot of θπ ratio and FST for wool traits. The vertical gray thick lines indicated the position of selected genes detected in our study. The top 5% threshold (FST = 0.228, θπ ratio = 0.064) values are denoted by blue horizontal dashed lines.
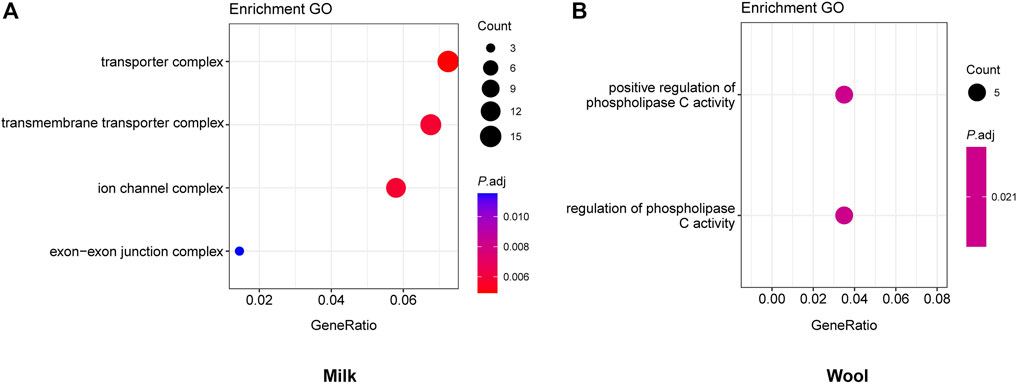
FIGURE 3. GO enrichment analyses for milk (A) and wool (B), with the significant (p.adj <0.05) GO terms.
In summary, we collected diverse goats’ germplasm resources and explored functional genes of important phenotypical traits. Our study confirmed previous results and also identified some novel genes involved in the regulation of specific traits. Our study provides deep insights into the genetic mechanisms of complex traits and genetic markers for genetic improvement in goats.
Data availability statement
The datasets presented in this study can be found in online repositories. The names of the repository/repositories and accession number(s) can be found in the article/Supplementary Material.
Ethics statement
Ethical review and approval was not required for the animal study because All data used in this study is publicly available.
Author contributions
F-HL conceived and designed the project. XW, J-NJ, and D-FW collected and analyzed the data. XW, J-NJ, and F-HL wrote the manuscript. All authors reviewed and approved the final manuscript.
Funding
This work was supported by the Natural Science Foundation of China (Nos.32061133010, 31972527) and the National Key Research and Development Program-Key Projects (2021YFD1300900). We thank Meng-Hua Li for his helpful comments and revisions.
Conflict of interest
The authors declare that the research was conducted in the absence of any commercial or financial relationships that could be construed as a potential conflict of interest.
Publisher’s note
All claims expressed in this article are solely those of the authors and do not necessarily represent those of their affiliated organizations, or those of the publisher, the editors and the reviewers. Any product that may be evaluated in this article, or claim that may be made by its manufacturer, is not guaranteed or endorsed by the publisher.
Supplementary material
The Supplementary Material for this article can be found online at: https://www.frontiersin.org/articles/10.3389/fgene.2023.1173017/full#supplementary-material
References
Alberto, F. J., Boyer, F., Orozco-terWengel, P., Streeter, I., Servin, B., de Villemereuil, P., et al. (2018). Convergent genomic signatures of domestication in sheep and goats. Nat. Commun. 9 (1), 813. doi:10.1038/s41467-018-03206-y
An, X., Hou, J., Li, G., Song, Y., Wang, J., Chen, Q., et al. (2012). Polymorphism identification in the goat KITLG gene and association analysis with litter size. Anim. Genet. 43 (1), 104–107. doi:10.1111/j.1365-2052.2011.02219.x
Badaoui, B., Serradilla, J., Tomas, A., Urrutia, B., Ares, J., Carrizosa, J., et al. (2007). Short communication: Identification of two polymorphisms in the goat lipoprotein lipase gene and their association with milk production traits. J. Dairy Sci. 90 (6), 3012–3017. doi:10.3168/jds.2006-409
Bolger, A. M., Lohse, M., and Usadel, B. (2014). Trimmomatic: A flexible trimmer for illumina sequence data. Bioinformatics 30 (15), 2114–2120. doi:10.1093/bioinformatics/btu170
Buaban, S., Lengnudum, K., Boonkum, W., and Phakdeedindan, P. (2022). Genome-wide association study on milk production and somatic cell score for Thai dairy cattle using weighted single-step approach with random regression test-day model. J. Dairy Sci. 105 (1), 468–494. doi:10.3168/jds.2020-19826
Cai, J., Wang, D., and Liu, J. (2018). Regulation of fluid flow through the mammary gland of dairy cows and its effect on milk production: A systematic review. J. Sci. Food Agric. 98 (4), 1261–1270. doi:10.1002/jsfa.8605
Ching, M. S., Shen, Y., Tan, W. H., Jeste, S. S., Morrow, E. M., Chen, X., et al. (2010). Deletions of NRXN1 (neurexin-1) predispose to a wide spectrum of developmental disorders. Am. J. Med. Genet. Part B Neuropsychiatric Genet. 153 (4), 937–947. doi:10.1002/ajmg.b.31063
Cingolani, P., Platts, A., Wang, L. L., Coon, M., Nguyen, T., Wang, L., et al. (2012). A program for annotating and predicting the effects of single nucleotide polymorphisms, SnpEff: SNPs in the genome of Drosophila melanogaster strain w1118; iso-2; iso-3. fly 6 (2), 80–92. doi:10.4161/fly.19695
Daly, K. G., Maisano Delser, P., Mullin, V. E., Scheu, A., Mattiangeli, V., Teasdale, M. D., et al. (2018). Ancient goat genomes reveal mosaic domestication in the Fertile Crescent. Science 361 (6397), 85–88. doi:10.1126/science.aas9411
Danecek, P., Auton, A., Abecasis, G., Albers, C. A., Banks, E., DePristo, M. A., et al. (2011). The variant call format and VCFtools. Bioinformatics 27 (15), 2156–2158. doi:10.1093/bioinformatics/btr330
Du, C., Deng, T., Zhou, Y., Ye, T., Zhou, Z., Zhang, S., et al. (2019). Systematic analyses for candidate genes of milk production traits in water buffalo (Bubalus Bubalis). Anim. Genet. 50 (3), 207–216. doi:10.1111/age.12739
El-Halawany, N., Abdel-Shafy, H., Abd-El-Monsif, A. S., Abdel-Latif, M. A., Al-Tohamy, A. F., and Abd El-Moneim, O. M. (2017). Genome-wide association study for milk production in Egyptian buffalo. Livest. Sci. 198, 10–16. doi:10.1016/j.livsci.2017.01.019
Gao, Y., Jiang, J., Yang, S., Hou, Y., Liu, G. E., Zhang, S., et al. (2017). CNV discovery for milk composition traits in dairy cattle using whole genome resequencing. BMC Genomics 18, 265. doi:10.1186/s12864-017-3636-3
Grossman, S. R., Shylakhter, I., Karlsson, E. K., Byrne, E. H., Morales, S., Frieden, G., et al. (2010). A composite of multiple signals distinguishes causal variants in regions of positive selection. Science 327 (5967), 883–886. doi:10.1126/science.1183863
Gu, B., Sun, R., Fang, X., Zhang, J., Zhao, Z., Huang, D., et al. (2022). Genome-wide association study of body conformation traits by whole genome sequencing in dazu black goats. Animals 12 (5), 548. doi:10.3390/ani12050548
Guo, J., Tao, H., Li, P., Li, L., Zhong, T., Wang, L., et al. (2018). Whole-genome sequencing reveals selection signatures associated with important traits in six goat breeds. Sci. Rep. 8 (1), 10405. doi:10.1038/s41598-018-28719-w
Islam, R., Liu, X., Gebreselassie, G., Abied, A., Ma, Q., and Ma, Y. (2020). Genome-wide association analysis reveals the genetic locus for high reproduction trait in Chinese Arbas Cashmere goat. Genes and Genomics 42, 893–899. doi:10.1007/s13258-020-00937-5
Khan, M. Z., Wang, D., Liu, L., Usman, T., Wen, H., Zhang, R., et al. (2019). Significant genetic effects of JAK2 and DGAT1 mutations on milk fat content and mastitis resistance in Holsteins. J. Dairy Res. 86 (4), 388–393. doi:10.1017/S0022029919000682
Larson, G., and Fuller, D. Q. (2014). The evolution of animal domestication. Annu. Rev. Ecol. Evol. Syst. 45, 115–136. doi:10.1146/annurev-ecolsys-110512-135813
Li, H., and Durbin, R. (2009). Fast and accurate short read alignment with Burrows–Wheeler transform. Bioinformatics 25 (14), 1754–1760. doi:10.1093/bioinformatics/btp324
Li, X., Yang, J., Shen, M., Xie, X.-L., Liu, G.-J., Xu, Y.-X., et al. (2020). Whole-genome resequencing of wild and domestic sheep identifies genes associated with morphological and agronomic traits. Nat. Commun. 11 (1), 2815. doi:10.1038/s41467-020-16485-1
Li, Y.-D., Xue, B., Xin, L., Wang, W.-j., Li, Z.-W., Ning, W., et al. (2022). Integration of genome-wide association study and selection signatures reveals genetic determinants for skeletal muscle production traits in an F2 chicken population. J. Integr. Agric. 21 (7), 2065–2075. doi:10.1016/s2095-3119(21)63805-4
Luigi-Sierra, M. G., Landi, V., Guan, D., Delgado, J. V., Castelló, A., Cabrera, B., et al. (2020). A genome-wide association analysis for body, udder, and leg conformation traits recorded in Murciano-Granadina goats. J. Dairy Sci. 103 (12), 11605–11617. doi:10.3168/jds.2020-18461
Mariadassou, M., Ramayo-Caldas, Y., Charles, M., Féménia, M., Renand, G., and Rocha, D. (2020). Detection of selection signatures in Limousin cattle using whole-genome resequencing. Anim. Genet. 51 (5), 815–819. doi:10.1111/age.12982
McKenna, A., Hanna, M., Banks, E., Sivachenko, A., Cibulskis, K., Kernytsky, A., et al. (2010). The genome analysis toolkit: A MapReduce framework for analyzing next-generation DNA sequencing data. Genome Res. 20 (9), 1297–1303. doi:10.1101/gr.107524.110
Menzi, F., Keller, I., Reber, I., Beck, J., Brenig, B., Schütz, E., et al. (2016). Genomic amplification of the caprine EDNRA locus might lead to a dose dependent loss of pigmentation. Sci. Rep. 6 (1), 28438. doi:10.1038/srep28438
Mukherjee, A., and Mayo, K. E. (2000). Regulation of inhibin subunit gene expression by gonadotropins and cAMP in ovarian granulosa cells. Gene Eng. Endocrinol. 22, 277–306. doi:10.1385/1-59259-221-x:277
Nakamura, Y., Fukami, K., Yu, H., Takenaka, K., Kataoka, Y., Shirakata, Y., et al. (2003). Phospholipase Cdelta1 is required for skin stem cell lineage commitment. EMBO J. 22 (12), 2981–2991. doi:10.1093/emboj/cdg302
Nakamura, Y., Ichinohe, M., Hirata, M., Matsuura, H., Fujiwara, T., Igarashi, T., et al. (2008). Phospholipase C-delta1 is an essential molecule downstream of Foxn1, the gene responsible for the nude mutation, in normal hair development. FASEB J. 22 (3), 841–849. doi:10.1096/fj.07-9239com
Nazari-Ghadikolaei, A., Mehrabani-Yeganeh, H., Miarei-Aashtiani, S. R., Staiger, E. A., Rashidi, A., and Huson, H. J. (2018). Genome-wide association studies identify candidate genes for coat color and mohair traits in the Iranian Markhoz goat. Front. Genet. 9, 105. doi:10.3389/fgene.2018.00105
Onteru, S. K., Fan, B., Du, Z. Q., Garrick, D. J., Stalder, K. J., and Rothschild, M. F. (2012). A whole-genome association study for pig reproductive traits. Anim. Genet. 43 (1), 18–26. doi:10.1111/j.1365-2052.2011.02213.x
Peters, S. O., Kızılkaya, K., Ibeagha-Awemu, E. M., Sinecen, M., and Zhao, X. (2021). Comparative accuracies of genetic values predicted for economically important milk traits, genome-wide association, and linkage disequilibrium patterns of Canadian Holstein cows. J. Dairy Sci. 104 (2), 1900–1916. doi:10.3168/jds.2020-18489
Pringle, H. (1998). The slow birth of agriculture. Science 282, 1446–1446. doi:10.1126/science.282.5393.1446
Riccetti, L., Sperduti, S., Lazzaretti, C., Casarini, L., and Simoni, M. (2018). The cAMP/PKA pathway: Steroidogenesis of the antral follicular stage. Minerva Ginecol. 70 (5), 516–524. doi:10.23736/S0026-4784.18.04282-X
Roos, J., DiGregorio, P. J., Yeromin, A. V., Ohlsen, K., Lioudyno, M., Zhang, S., et al. (2005). STIM1, an essential and conserved component of store-operated Ca2+ channel function. J. Cell Biol. 169 (3), 435–445. doi:10.1083/jcb.200502019
Seo, D., Lee, D. H., Jin, S., Won, J. I., Lim, D., Park, M., et al. (2022). Long-term artificial selection of Hanwoo (Korean) cattle left genetic signatures for the breeding traits and has altered the genomic structure. Sci. Rep. 12 (1), 6438. doi:10.1038/s41598-022-09425-0
Siddiqui, T. J., Pancaroglu, R., Kang, Y., Rooyakkers, A., and Craig, A. M. (2010). LRRTMs and neuroligins bind neurexins with a differential code to cooperate in glutamate synapse development. J. Neurosci. 30 (22), 7495–7506. doi:10.1523/JNEUROSCI.0470-10.2010
Skapetas, B., and Bampidis, V. (2016). Goat production in the world: present situation and trends. Livest. Res. Rural Dev. 28, 200.
Talouarn, E., Bardou, P., Palhière, I., Oget, C., Clément, V., Tosser-Klopp, G., et al. (2020). Genome wide association analysis on semen volume and milk yield using different strategies of imputation to whole genome sequence in French dairy goats. BMC Genet. 21 (1), 19–13. doi:10.1186/s12863-020-0826-9
Vaissier Welborn, V. (2020). Enamel synthesis explained. Proc. Natl. Acad. Sci. 117 (36), 21847–21848. doi:10.1073/pnas.2014394117
Wang, J.-J., Zhang, T., Chen, Q.-M., Zhang, R.-Q., Li, L., Cheng, S.-F., et al. (2020a). Genomic signatures of selection associated with litter size trait in Jining Gray goat. Front. Genet. 11, 286. doi:10.3389/fgene.2020.00286
Wang, K., Kang, Z., Jiang, E., Yan, H., Zhu, H., Liu, J., et al. (2020b). Genetic effects of DSCAML1 identified in genome-wide association study revealing strong associations with litter size and semen quality in goat (Capra hircus). Theriogenology 146, 20–25. doi:10.1016/j.theriogenology.2020.01.079
Wang, X., Herberg, F. W., Laue, M. M., Wüllner, C., Hu, B., Petrasch-Parwez, E., et al. (2000). Neurobeachin: A protein kinase A-anchoring, beige/Chediak-higashi protein homolog implicated in neuronal membrane traffic. J. Neurosci. 20 (23), 8551–8565. doi:10.1523/JNEUROSCI.20-23-08551.2000
Wang, Z., Zhang, H., Yang, H., Wang, S., Rong, E., Pei, W., et al. (2014). Genome-wide association study for wool production traits in a Chinese Merino sheep population. PloS One 9 (9), e107101. doi:10.1371/journal.pone.0107101
Weir, B. S., and Cockerham, C. C. (1984). Estimating F-statistics for the analysis of population structure. Evolution 38, 1358–1370.
Wu, T., Hu, E., Xu, S., Chen, M., Guo, P., Dai, Z., et al. (2021). clusterProfiler 4.0: A universal enrichment tool for interpreting omics data. Innovation 2 (3), 100141. doi:10.1016/j.xinn.2021.100141
Yang, J., Li, W.-R., Lv, F.-H., He, S.-G., Tian, S.-L., Peng, W.-F., et al. (2016). Whole-genome sequencing of native sheep provides insights into rapid adaptations to extreme environments. Mol. Biol. Evol. 33 (10), 2576–2592. doi:10.1093/molbev/msw129
Yuan, Z., Zhang, J., Li, W., Wang, W., Li, F., and Yue, X. (2019). Association of polymorphisms in candidate genes with the litter size in two sheep breeds. Animals 9 (11), 958. doi:10.3390/ani9110958
Zheng, Z., Wang, X., Li, M., Li, Y., Yang, Z., Wang, X., et al. (2020). The origin of domestication genes in goats. Sci. Adv. 6 (21), eaaz5216. eaaz5216. doi:10.1126/sciadv.aaz5216
Keywords: goat, artificial selection, phenotypic traits, whole-genome scan, candidate genes
Citation: Wan X, Jing J-N, Wang D-F and Lv F-H (2023) Whole-genome selective scans detect genes associated with important phenotypic traits in goat (Capra hircus). Front. Genet. 14:1173017. doi: 10.3389/fgene.2023.1173017
Received: 24 February 2023; Accepted: 03 April 2023;
Published: 18 April 2023.
Edited by:
Ran Di, Chinese Academy of Agricultural Sciences, ChinaReviewed by:
Weimin Wang, Gansu Agricultural University, ChinaQiuyue Liu, Chinese Academy of Sciences (CAS), China
Copyright © 2023 Wan, Jing, Wang and Lv. This is an open-access article distributed under the terms of the Creative Commons Attribution License (CC BY). The use, distribution or reproduction in other forums is permitted, provided the original author(s) and the copyright owner(s) are credited and that the original publication in this journal is cited, in accordance with accepted academic practice. No use, distribution or reproduction is permitted which does not comply with these terms.
*Correspondence: Feng-Hua Lv, lvfenghua@cau.edu.cn
†These authors have contributed equally to this work