- Fish Genetics and Biotechnology Division, ICAR-Central Institute of Freshwater Aquaculture, Bhubaneswar, India
Labeo catla (catla) is the second most commercially important and widely cultured Indian major carp (IMC). It is indigenous to the Indo-Gangetic riverine system of India and the rivers of Bangladesh, Nepal, Myanmar, and Pakistan. Despite the availability of substantial genomic resources in this important species, detailed information on the genome-scale population structure using SNP markers is yet to be reported. In the present study, the identification of genome-wide single nucleotide polymorphisms (SNPs) and population genomics of catla was undertaken by re-sequencing six catla populations of riverine origin from distinct geographical regions. DNA isolated from 100 samples was used to perform genotyping-by-sequencing (GBS). A published catla genome with 95% genome coverage was used as the reference for mapping reads using BWA software. From a total of 472 million paired-end (150 × 2 bp) raw reads generated in this study, we identified 10,485 high-quality polymorphic SNPs using the STACKS pipeline. Expected heterozygosity (He) across the populations ranged from 0.162 to 0.20, whereas observed heterozygosity (Ho) ranged between 0.053 and 0.06. The nucleotide diversity (π) was the lowest (0.168) in the Ganga population. The within-population variation was found to be higher (95.32%) than the among-population (4.68%) variation. However, genetic differentiation was observed to be low to moderate, with Fst values ranging from 0.020 to 0.084, and the highest between Brahmani and Krishna populations. Bayesian and multivariate techniques were used to further evaluate the population structure and supposed ancestry in the studied populations using the structure and discriminant analysis of principal components (DAPC), respectively. Both analyses revealed the existence of two separate genomic clusters. The maximum number of private alleles was observed in the Ganga population. The findings of this study will contribute to a deeper understanding of the population structure and genetic diversity of wild populations of catla for future research in fish population genomics.
1 Introduction
Labeo catla (Hamilton, 1822), popularly known as “catla,” is the second most popular Indian major carp (IMC) (Murthy, 2002). This potamodromous fish is indigenous to the Indo-Gangetic riverine system of India and the rivers of Bangladesh, Nepal, Myanmar, and Pakistan (Reddy, 2005). It is widely cultured in polyculture systems of the Indian subcontinent because of its fast-growing nature, compatibility with other major carps, unique surface feeding behavior, good taste, and consumer preference (FAO, 2006–2020). At present, L. catla constitutes ∼3.4% of the global freshwater aquaculture production ($5 billion USD) (FAO, 2019). In the last 20 years, the culture of IMCs has intensified due to their high commercial value, increasing demand, and fast growth rate with 4,713,340 tons of production (FAO, 2000-2020). An extreme population decline trend of 87% in the wild capture of L. catla species has been observed by comparing the survey data from 1958 to 1994 (Payne et al., 2004) in Indian rivers, which may have been caused by overfishing and habitat alterations by construction of dams and pollution (Reddy, 2005). Wild populations are used as a source of genetic diversity for hatchery stock. To establish effective management and conservation guidelines, as well as develop genetic improvement programs for catla species, it is crucial to first assess genetic variations among populations using extensive information on the genetic structure of the wild populations of catla.
However, quite limited genetic information on studies pertaining to genetic variation in wild populations of catla is available using microsatellites (Alam and Islam, 2005; Ahmed and Abbas, 2018; Faroque et al., 2021), random amplified polymorphic DNA (RAPD) (Islam et al., 2005; Rahman et al., 2009), and mitochondrial DNA markers (Das et al., 2012; Sarmah and Sarmah, 2016; Behera et al., 2017). In addition to this, very few studies on genetic diversity employing SNP markers have been reported in the wild populations of catla (Hamilton et al., 2019). Nevertheless, the degree, pattern, and variation of the genetic structure in the present context are still poorly understood.
Being the most abundant molecular marker in the genomes of various vertebrate organisms, SNPs have been the markers of choice for a variety of applications, including population genomics. Over the last two decades, next-generation sequencing (NGS) technology has evolved, enabling the rapid and inexpensive identification of genome-wide SNP markers in any organism without access to prior genomic data. Furthermore, genotyping-by-sequencing (GBS) has become a potential genome-wide genotyping tool that employs an enzyme-based complexity reduction method to simultaneously discover and genotype molecular markers across the entire genome, with or without a reference (Elshire et al., 2011). The genome-wide SNPs discovered using the GBS technique have formed the basis of population structure and diversity studies (Nyinondi et al., 2020; Yang et al., 2020; Fagbemi et al., 2021), linkage map construction (Everett and Seeb, 2014; Uchino et al., 2018; Yin et al., 2020), QTL analyses (Uchino et al., 2018), genome-wide association studies for economically important traits, such as disease resistance (Correa et al., 2015; Gutierrez et al., 2015; Wang et al., 2017), and the application of genomic selection (Wolc et al., 2015; Wang et al., 2019; Luo et al., 2021; Luo et al., 2022; Wang et al., 2022).
In the present investigation, genome-wide SNPs were identified and population genomics analysis was performed in L. catla by genotyping-by-sequencing of six riverine catla populations from distinct geographical regions of India. The results of this study will enhance our knowledge in understanding genetic diversity and population structure and help in the conservation and management of wild populations of catla.
2 Materials and methods
2.1 Sample collection
Fish samples pertaining to riverine populations were collected from six Indian rivers viz, Cauvery (CAU), Krishna (KRN), Godavari (GOD), Mahanadi (MAH), Brahmani (BRM), and Ganga (GAN) (Table 1; Figure 1). The waters of the Cauvery River at Mysore are reported to have high metal contamination and altered water quality due to nearby agricultural and industrial activities (Susheela et al., 2014), as well as a damming effect due to the presence of the Krishna Raja Sagara Dam. Fish sampling from the Krishna River was performed at Vijayawada, where compromised water quality due to fly ash arising from the Vijayawada Thermal Power Station is reported (Rao and Ravindhranath, 2013). Moreover, the fish fauna of the Krishna River near the Prakasam Barrage is threatened due to several factors, including heavy harvesting of fish resources, competition and predation by introduced species, and habitat degradation due to pollutants (Jadhav et al., 2011). Rajahmundry was the only sampling site where the Water Quality Index (WQI) of the Godavari River was found to be fair (Sravya et al., 2017) and less environmental impact was observed. The Mahanadi River near Sambalpur in Odisha state is a major site for coal fields, while the Brahmani River near Barkote is moderately affected by industrial and agricultural runoffs (Nath et al., 2018). Samples were collected from the Ganga River at Patna, a state capital witnessing a colossal level of urbanization (Imam. 2020) along with air and water pollution due to rampant sand mining and brick kilns. Recently, a report on the shifting of the Ganga River by an average of 0.14 km per year from the city of Patna has alerted environmentalists to focus on its associated flora and fauna (Kumar et al., 2014).
Samples were collected from adult individuals ranging from 1 to 2 kg in weight. Natural hybrids of catla are known to be rare in the riverine populations. Even then, all sampled individuals were checked phenotypically, considering the shape of the head and mouth (Sarder et al., 2011). As the individuals ranged from 1 to 2 kg in weight, they were easily distinguishable. No hybrids were detected. Fin clips from 100 individuals were stored at −20°C in 95% ethyl alcohol until DNA extraction. All handling of fish was carried out following the guidelines for control and supervision of experiments on animals established by the Government of India and approved by the Institutional Animal Ethics Committee (AEC) of ICAR-CIFA.
2.2 DNA isolation
High-molecular weight (MW) genomic DNA (gDNA) was extracted from the fin clips using a “DNeasy Blood and Tissue Kit” (QIAGEN, Hilden, Germany), according to the manufacturer’s protocol. The integrity and concentration of the DNA were checked by 0.8% agarose gel electrophoresis and using a Nanodrop Biospectrometer (Eppendorf India Pvt. Ltd.). Samples with an adequate concentration (≥100 ng/μl) and quality (intact high-MW DNA) were selected. One GBS library was prepared using 100 samples.
2.3 GBS library construction and sequencing
Using Elshire et al.’s (2011) methodology with modifications, GBS data were generated. In brief, each 200 ng of DNA sample was digested with five units of the ApeK1 enzyme at 75°C for 4 h and cooled down to 4°C, followed by GBS library preparation. Unique barcodes and a “common” adapter were used for each individual ligation. After ligation, they were pooled and purified using AMpure DNA purification beads and the genomic fragments were then amplified in 50-µl volumes. GBS library purification was carried out using Agencourt AMPure XP SPRI magnetic beads (Beckman Coulter). The quality of the GBS library was assessed using the 4150 TapeStation System (Catalog: G2992AA, Agilent). The GBS library was sequenced using the Illumina HiSeq 2000 platform with paired-end (PE) reads of 150 × 2 bp.
2.4 Sequence data analysis and SNP genotyping
The quality of the data was checked with FastQC v0.11.8 (Andrews, 2010) and MultiQC v1.9 (Ewels et al., 2016) software. The fastp (Chen et al., 2018) program was used to remove the adapter, along with duplicate sequences and bases of poor quality (Q < 30) from the raw reads. The high-quality reads of each individual were mapped to the catla reference genome [GeneBank acc. no: GCA_012976165.1 (Sahoo et al., 2020)] using BWA v0.6.12 software with the “MEM” option (Li, 2013) and default parameters. The reference catla genome had an assembled genome size of 1.01 Gb, 5,245 scaffolds and >92% BUSCO completeness. SAMtools v0.1.19 (Li et al., 2009) was used to convert the SAM files into BAM and sort alignments by genomic coordinates. SNPs were discovered using the GSTACKS program with a default model (Marukilow) in STACKS v2.59 (Catchen et al., 2013; Rochette et al., 2019) with the parameters var-alpha = 0.05, gt-alpha = 0.05 (both default), and min-mapq = 30, to reduce the impact of misplaced reads. Subsequently, SNPs present in at least 75% of the individuals in each population (r) and across populations (R) were identified with a minor allele frequency of 0.05 using the POPULATIONS program of STACKS. Additionally, these SNPs were filtered again with mean depth >= 5 using VCFtools v0.1.12 (Danecek et al., 2011) (Table 2). The resultant SNPs were used for subsequent genetic analysis.
2.5 Genetic diversity analysis and effective population size
STACKS v2.59 (Rochette et al., 2019) software was used to assess the genetic variation metrics, such as mean expected heterozygosity (He) and observed heterozygosity (Ho), average inbreeding coefficients (FIS), nucleotide diversity (π) with their standard errors (SE), mean frequency of the most frequent allele (P), Hardy–Weinberg equilibrium (HWE) estimates, and pairwise Fst values across the six populations of catla. The pairwise Fst heatmap was constructed with Heatmapper software (Babicki et al., 2016). Furthermore, analyses of molecular variance (AMOVA) were performed to find out the distribution of genetic variations using Arlequin v3.5 software (Excoffier and Lischer, 2010). The effective population size (Ne) was estimated by using a molecular co-ancestry method (Nomura, 2008), as implemented in NeEstimator v2.01 (Do et al., 2014). PGDSpider software (Lischer and Excoffier, 2012) was used to convert the vcf file into a GENEPOP input file for NeEstimator.
2.6 Clustering and ancestry analysis
For visualization and insight into the existence of genetic clusters, principal component analysis (PCA) was carried out using the R tool v3.5 (R core team, 2018) with the Adegenet v2.1.1 package (Jombart, 2008; Jombart and Ahmed, 2011). The scatter plot was created in R using the “ggplot2″ package (Ginestet et al., 2011) with the first and second components. Thereafter, the genetic structure was analyzed through the multivariate approach called DAPC (Jombart et al., 2010) in the same R package that utilizes the number of principal components and discriminant axes to divide the sample into between-group and within-group components in order to maximize discrimination across populations. In DAPC, data are first converted using principle component analysis (PCA), and then, clusters are determined using discriminant analysis (DA). This approach offers a visual representation of the genetic difference in multivariate space among populations. Additionally, the probable population admixture was analyzed using Structure v.2.3.4 software (Hubisz et al., 2009) under correlated allele frequencies and admixture models (settings: K values = 1–10; burn-in length = 50,000; MCMC replicates = 200,000; iterations = 10). L(K) and ΔK statistics were inferred in both STRUCTURE HARVESTER (Earl and vonHoldt, 2012) and CLUMPAK (Kopelman et al., 2015) software applications to select the optimal number of genetic clusters (K). A UPGMA tree was also constructed to cluster the six catla populations using the R tool in the “ape” v5.6.2 (Paradis and Schliep, 2019) package.
2.7 Linkage disequilibrium
TASSEL v5.0 (Bradbury et al., 2007) software was used to analyze LD (r2) for the six catla populations with a sliding window size of 50. A non-linear regression curve was used to estimate LD decay, and the distance between SNPs at which the average r2 drops to 50% of the maximal LD value was used to compute the LD decay distance.
3 Results
3.1 SNP identification and genotyping
GBS libraries were sequenced, generating a total of 472 million raw data with 0.71 Gb of bases on average for each individual. Each randomly selected read was mapped to the sequences of various fish species including Cyprinus carpio and L. catla in the NCBI NT database, demonstrating the reliability of the reads. After removing reads with low base quality (Q < 30), on average, 96.91% of filtered reads were successfully mapped to a catla reference genome with approximately 278 million clean data (Supplementary Table S1), and an average of 2.78 million clean reads per individual were retained. After applying the filtering criteria that a locus must be present in more than 75% of the individuals in a population and across populations, the MAF must be greater than 0.05, and the mean depth must be >= 5, 10,485 putative SNP markers were selected for further genetic data analysis. The average depth of the 10,485 SNPs was 10. The STACKS output indicated the presence of 8,963 loci and 1–2 SNPs per locus.
3.2 Genetic diversity and population structure
In this study, the genetic diversity of six catla populations and the allele polymorphism of these groups were analyzed. Across all populations, the expected heterozygosity (He) ranged from 0.162 (GAN) to 0.20 (GOD) and observed heterozygosity (Ho) varied between 0.053 (GAN) and 0.06 (BRM). The nucleotide diversity (π) varied from 0.168 to 0.205 across the six populations, with the GAN population having the least value. The mean frequency of the most frequent allele (P) varied from 0.87 to 0.89 among the six populations of catla. The inbreeding coefficients (FIS) of the six populations of catla ranged from 0.382 to 0.637 and were highest in the GOD population (Table 3). Six populations with 77,216 to 78,421 loci out of 79,861 loci showed substantial deviations from the Hardy–Weinberg equilibrium (p < 0.05). Maximum numbers of private alleles were observed in the GAN population in comparison to other studied wild populations. Ne values were successfully estimated for 10,485 SNP markers with the highest value of 1.2 in the KRN population, whereas the lowest value was 0.8 in the CAU population (Supplementary Table S2).
The results of PCA scatter plots are shown in Figure 2. Most individuals from GOD and KRN were grouped together with abscissa values less than 0. Most individuals of BRM, MAH, and GAN together with few individuals from CAU were clustered on abscissa values greater than 0, while most individuals of CAU and GOD showed closer affinity toward the KRN cluster. Thereafter, DAPC further revealed the population structure with two main clusters (Figure 3A).
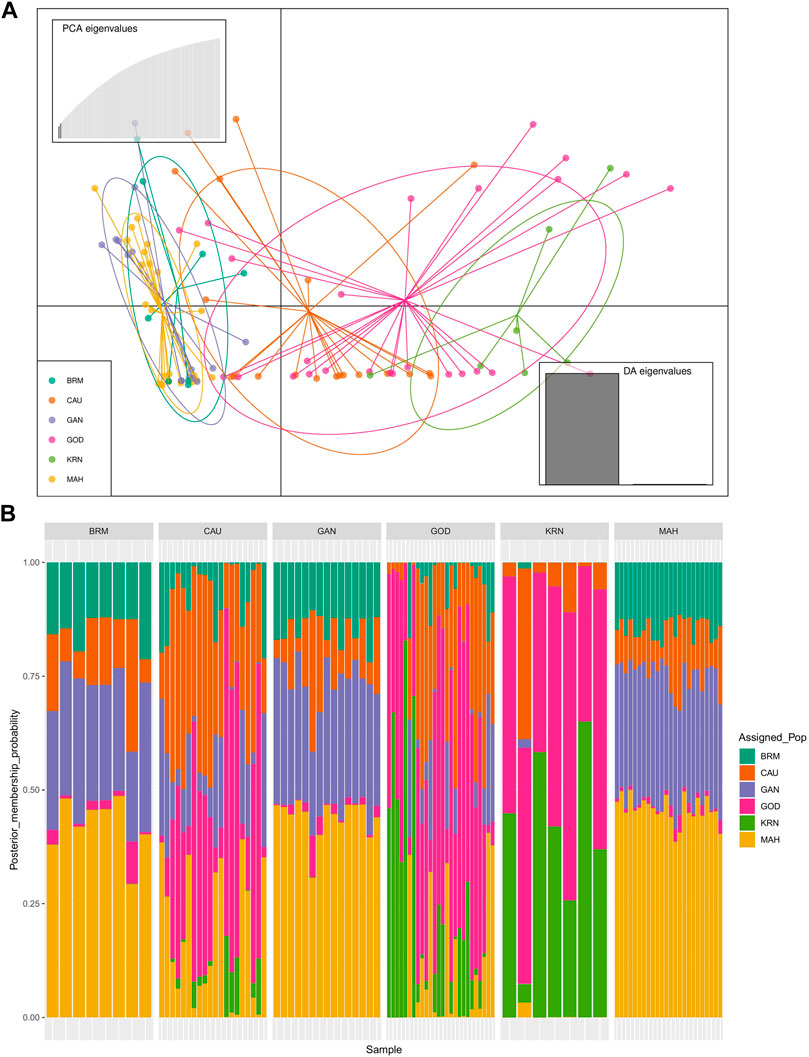
FIGURE 3. Discriminant analysis of principal components (DAPC) of six catla populations: (A) the scatter plot for all data with two linear discriminants explained 89.68% and 10.32% of the variation, respectively. (B) The membership probabilities of each individual in the two assigned clusters estimated by DAPC. The vertical bars correspond to the individuals of each population.
In addition, Figure 3B shows the membership probabilities of each individual in various clusters. This bar graph provides a more organized picture of our data by comparing the population membership probability assignments with the populations to which they were first assigned. The individuals of BRM, MAH, and GAN populations were assigned to the same cluster, and most individuals of KRN populations were allocated to another cluster. CAU and GOD, on the other hand, had substantial admixture from all clusters. Furthermore, the Bayesian clustering algorithm STRUCTURE revealed the shared population ancestry of 100 individuals partitioned into two clusters at the optimal cluster value K = 3 (Figures 4A,B).
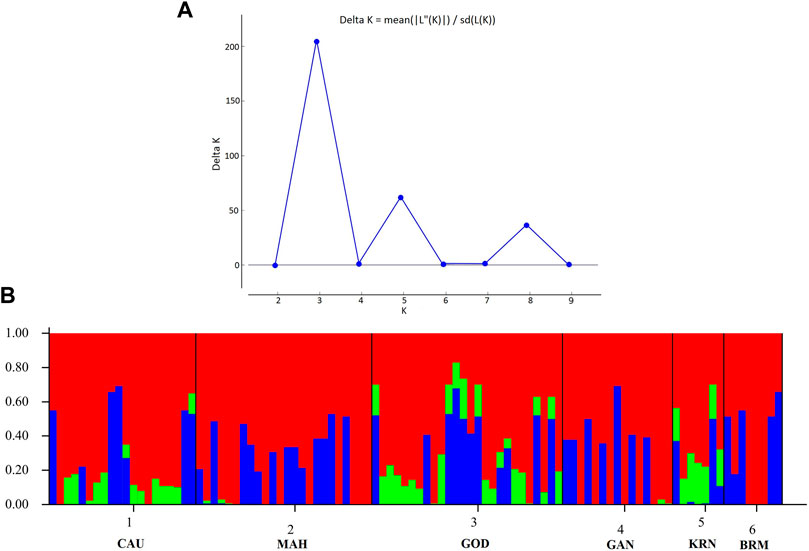
FIGURE 4. Clustering analysis using STRUCTURE. (A) The optimal cluster value (K) was k = 3 with ΔK. (B) The Structure analysis with admixture results for K = 3 with two clusters.
The UPGMA tree of the six populations (Figure 5) showed two genetically separate clusters, with almost all KRN and GOD individuals clustering together (cluster 1) and the remaining individuals aggregating into a second cluster (cluster 2). Individuals from MAH, BRM, and GAN made up most of the individuals in cluster 2.
3.3 Genetic differentiation and gene flow
The pairwise Fst values ranged from 0.0208 to 0.0845 for these six catla populations (Figure 6; Table 4). The maximum genetic difference (Fst = 0.0845) was found between the BRM and KRN populations. In contrast, the CAU and GOD populations had the least genetic difference (Fst = 0.0208). Furthermore, to study the genetic variations across the populations, AMOVA (Table 5) was performed. Within populations, variation was found to be higher (95.32%) in proportion. On the other hand, the variation among populations accounted for just 4.68% of the overall molecular variation in the studied populations.
3.4 Linkage disequilibrium
The whole-genome average maximum r2 value was found to be 0.2, which reduced to 0.1 at a distance of 472 bp. Hence, this distance is assumed to represent the whole-genome LD decay distance and LD decayed above this distance (Figure 7). Thus, no significant pairwise linkage disequilibrium was observed among the loci.
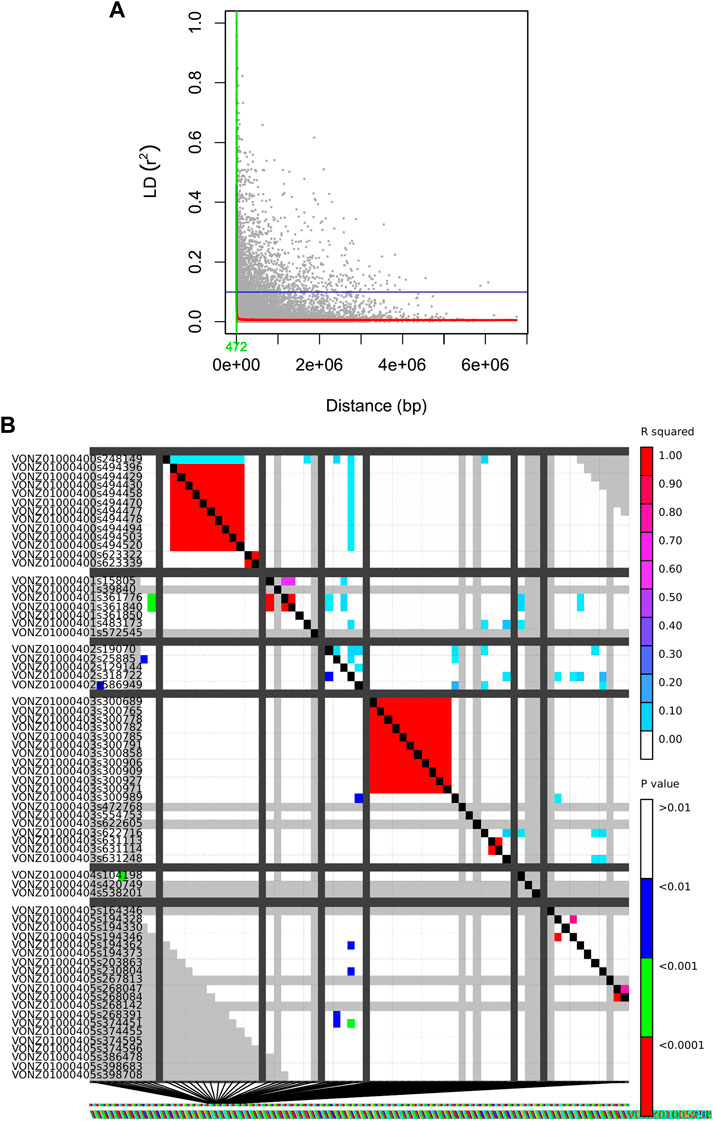
FIGURE 7. (A) Genome-wide LD decay plots. The x-axis shows the distance between SNPs in base pair, while the y-axis indicates the LD values in r2. Half LD and LD decay distance are shown by the horizontal lines and vertical lines, respectively. (B) The x and y axes are used to illustrate the pairwise LD scores of polymorphism sites. The values for R-squared are shown above the diagonal, while the associated values for P are shown below the diagonal. The results of a comparison between two sets of marker sites are displayed by each cell, with colour coding based on the occurrence of significant LD. In both diagonals, the substantial threshold levels are represented by a coloured barcode.
4 Discussion
4.1 SNP identification, genetic diversity, and population structure
Genotyping-by-sequencing resulted in 10,485 putative SNP markers from the 278 million filtered reads. Nucleotide diversity is an important indicator for assessing the DNA sequence diversity of a species or population (Goodall-Copestake et al., 2012). The nucleotide diversity (π) that was identified in this research falls within the range that has been reported in earlier studies involving freshwater fishes (Habib et al., 2012; Nyinondi et al., 2020; Zhou et al., 2021). All six catla populations showed relatively low nucleotide diversity, with the GAN population having the lowest value. Fast lineage shortening within founder populations leads to low nucleotide diversity, which is a feature of a narrow genetic structure (Grant and Bowen, 1998). Loss of allelic heterozygosity in our sampling sites may be due to habitat loss and fragmentation that led to inbreeding and selection (Malini and Rao, 2004; Jadhav et al., 2011; Kumar et al., 2020; Das et al., 2021). Another possible explanation for the absence of considerable allelic heterogeneity in the catla population may be the “stepping stone model of migration” (Felsenstein, 1997), which links effective gene flow to gene exchange among neighboring populations (Kumar et al., 2014; Das et al., 2021).
In addition to nucleotide diversity, heterozygosity is also a key determinant of population diversity in a given species. In this study, low levels of heterozygosity were observed across all populations. The observed heterozygosity was lower than the expected heterozygosity, which is similar to the findings of Faroque et al. (2021) and Alam and Islam. (2005) in L. catla and Alam et al. (2009) in L. rohita using microsatellite DNA markers. However, our result showed a small difference between the mean He and Ho. Observations made by Hamilton et al. (2019) were at most similar for wild populations of catla using SNP markers. This indicates that, over time, the rivers containing wild populations of L. catla have experienced a decrease in genetic diversity, but the specific reasons for this are not clear. The loss in river population is thought to be caused by unregulated fishing methods, including the overexploitation of seed, inadequate and inconsistent river flow as a result of the construction of dams, and water contamination by human activities (Alam et al., 2009). Heterozygosity losses may also be the consequence of inbreeding, selection, and decline in breeding populations due to intermixing of diversified genetic stocks or the Wahlund effect (Hartl and Clark, 1997).
For a long time, rivers have been used for industrial development, electricity production, fishing, and agriculture near highly urbanized areas. The major effects of urbanization on river systems include habitat loss and fragmentation, altered water quality (due to an increase in the loading of pollutants, fertilizers, and sediment), and biological invasion (Simpson et al., 2016; Hammerschlag et al., 2017). Similar incidents were observed at our sampling locations. Rapid urbanization around the industrial areas of Sambalpur and Cuttack near the Mahanadi River has been polluting the river water with sewerage, industrial effluents, and biomedical waste (Swain et al., 2017). Industrial and agricultural runoffs (Nath et al., 2018), as well as effluents from the Rourkela Steel Plant and other chemical industries in the river bed, moderately affect Brahmani River water quality near Barkote (Swain et al., 2017). Rajahmundry was the only sampling site where the Water Quality Index (WQI) of the Godavari River was found to be fair and less environmental impact was noted (Sravya et al., 2017). According to Susheela et al. (2014), the Cauvery River at Mysore has an alarming level of metal pollution and altered water quality due to nearby agricultural and industrial activities and a damming effect due to the presence of the Krishna Raja Sagara Dam. Additionally, the Ganga River at Patna recorded a colossal level of urbanization (Imam, 2020) along with air and water pollution as a result of rampant sand mining and brick kilns. This may be the reason for the lower diversity of the GAN population compared with other sampled wild populations. At the same time, compromised water quality due to fly ash arising from the Vijayawada Thermal Power Station has been reported (Rao and Ravindhranath, 2013). Also, the fish fauna of the Krishna River near the Prakasam Barrage is in danger due to a number of issues, such as intensive fishing, competition and predation from invasive species, and habitat degradation from pollution (Jadhav et al., 2011). Dam-induced delta erosion affects estuarine fisheries and reduces the biodiversity of estuarine flora and fauna, which leads to effects on the effective population size and inbreeding levels (Malini and Rao, 2004). Habitat loss, fragmentation, and hydrological alteration arise from excessive sand mining, encroachment, and siltation on river beds of the Saruali and Rengali dams (Das et al., 2021). An observation on the migration of fish from their natural habitat resulting in inbreeding and selection due to the presence of the Hirakud Dam has been reported (Kumar et al., 2020), which is a major concern. The absence of freshwater in the estuaries and the destruction of mangrove forests have caused a decline in fisheries in the Cauvery, Krishna, Godavari, Mahanadi, and Brahmani estuaries. Salinity variations brought on by water obstruction (Dandekar, 2012) and biological invasion of alien fish due to the Krishna Godavari River linking project at Vijayawada in Nagarjuna Sagar Dam, Rajahmundry in the Dowleswaram Barrage, and dams at Patna (Dandekar, 2012; Singh et al., 2013; Babu and Padmavathi, 2016) are also affecting fish heterozygosity and nucleotide diversity.
The inbreeding coefficients (FIS) of six populations of catla indicate increased homozygosity in GOD, as compared to other studied wild populations (Table 3). The occurrence of non-random mating or population division is frequently indicated by high FIS readings (Allendorf et al., 2007). In this investigation, the resulting alleles were found to be polymorphic (Table 3). When the most frequent allele’s frequency was less than or equal to 0.99, then that region was defined as a polymorphic locus (Hartl and Clark, 1997). The observed unexpected maximum private allele in the GAN population at any SNP loci argues against the effective ongoing gene flow. This unexpected outcome may be due to the small sampling size or it may show maximum differentiation within a population due to the random mutation (Hartl and Clark, 1997), which thus requires further investigation.
Effective population size (Ne), usually less than the census population size, influences genetic diversity in a population. Our results showed lower levels of Ne ranging from 0.8 to 1.2, and similar observations were reported by Escalante et al. (2020) and Diaz et al. (2021). Inbreeding and genetic drift are expected to reduce genetic diversity as Ne decreases (Frankham, 2005). In this study, the CAU population had the lowest Ne (0.8) with relatively lower nucleotide diversity (0.178) and observed heterozygosity (0.055) than all other populations, resulting in a moderately higher FIS value (0.57). However, this is not always true in populations in which genetic diversity is altered by external influences. For example, despite decreases in Ne (0.9) in the GOD population, there was no decline in the nucleotide diversity (0.205) and observed heterozygosity (0.059). Despite having a higher FIS value (0.637), genetic diversity seemed to be maintained through immigration and other events, such as the recent artificial interlinking of rivers. A similar observation was obtained in Atlantic salmon (Salmo salar) (Consuegra et al., 2005; Fraser et al., 2007). In addition to this, the GOD population at Rajahmundry has been reported to have a fair Water Quality Index (WQI) and lower pollution levels (Sravya et al., 2017), indicating less influence on genetic diversity. The BRM population had a higher level of Ne (1.1), indicating higher genetic diversity. Estimated genetic indices of the BRM population showed a similar pattern, with the highest Ho (0.06) among populations under study. Similar findings were obtained for the KRN population, which had the highest effective population size (1.2) with a higher Ho (0.059) among populations. The most concerning populations in our studies were those of Mahanadi and Ganga. Among six populations, the GAN population had the lowest Ne (0.9), the lowest Ho (0.053), and the lowest nucleotide diversity (0.168) with a moderately higher inbreeding (0.428) value. Similar observations were seen in the MAH population, which had a relatively lower Ne (0.9) and a lower nucleotide diversity (0.174) and Ho (0.054). The observed reduction in heterozygosity could be attributed to the enormous pollution due to industrial activities, sand mining, and the release of agricultural runoffs in the Ganga River (Imam, 2020). In addition, activities related to coal mines and water pollution by sewerage and biomedical waste from two highly urbanized industrial cities such as Sambalpur and Cuttack have adversely affected the genetic diversity in the MAH population. Furthermore, habitat fragmentation due to the presence of the Hirakud Dam has also been reported (Swain et al., 2017). Thus, GAN and MAH populations require attention and efficient management for the conservation of wild populations of catla.
In this study, the PCA indicated no substantial grouping by populations or sampling regions as these samples of catla populations, to some extent, were leading to the probability of gene pool mixing as a result of anthropogenic activities. Specific reasons in relation to the anthropogenic activities can be attributed to rigorous aquaculture activities, such as seed production in hatcheries, in which unregulated hybrid fish production might pose a threat in terms of genetic pool contamination. Another major concern in the Indian context is the significant inbreeding rate in hatcheries. Hatchery-produced inbred individuals escaping to the natural environment may also cause the gene pool to be adversely affected (Alam et al., 2009; Mandal et al., 2014). Nevertheless, it seems that there are two major clusters: the first cluster contains GOD and KRN, while the second cluster has the other remaining populations. The results of the PCA and DAPC can be seen to be very similar, with an organized picture created using the membership probabilities of each individual in the DAPC, in which the individuals from the BRM, MAH, and GAN populations are all in the same cluster, while the individuals from the KRN population fall into a separate cluster and individuals from the CAU and GOD populations are admixed. The overlap in clusters and the lack of significant distances between groups shown by the multivariate DAPC may be explained by high gene flow and the mixing events that have taken place in individuals within the populations under study. Traditionally, for structure analysis, the most probable K is chosen by applying the highest value of L(K), but in the majority of cases, L(K) tends to marginally rise even after the genuine K has been attained. As a result, we computed ΔK according to Evanno et al. (2005) as well. At the most likely value of K, a clearer peak can be presented using ΔK (Figure 4A). Ancestral proportions at K = 3 indicated that the 100 individuals were partitioned into two clusters (Figure 4B). The STRUCTURE analysis result is similar to the DAPC result, which shows that six populations were divided into two main clusters. No significant population structure was observed in catla, as reported by Hamilton et al. (2019) and Das Mahapatra et al. (2018). Furthermore, ancestry analysis revealed the occurrence of mixing among the aforementioned groups. This could be due to the intermixing of individuals between rivers through human intervention. Another possible explanation for this phenomenon might be that the L. catla species taken from distinct river basins in the current study had a similar ancestral gene pool, for which at least historical connections existed across different rivers (Daniels, 2001). The distribution of river systems has the greatest influence on the population structure of freshwater species. The result of the UPGMA tree of the six populations of catla also showed two genetic clusters among the six populations, which was in line with the results of the PCA and DAPC. Similar results were shown in L. catla (Rahman et al., 2009; Ali et al., 2015) and L. rohita (Islam and Alam, 2004) using RAPD analysis, and also in L. rohita (Alam et al., 2009) using microsatellite DNA markers among the Halda, Padma, and Jamuna rivers, respectively.
In brief, the six populations of L. catla were divided into two clusters based on the results of PCA, DAPC, STRUCTURE, and the UPGMA tree, with a moderate degree of genetic divergence. According to the outcomes of genetic admixture and phylogenetic analysis, a significant number of individuals from the BRM, MAH, and GAN populations of catla were clustered together and it was not possible to entirely differentiate the two clusters due to the presence of genetic overlap. In particular, the majority of individuals originating from GOD populations displayed a closer kinship to the KRN populations.
4.2 Population genetic differentiation and gene flow
The pairwise Fst values ranged from 0.0208 to 0.0845 for these six catla populations, showing low-to-moderate levels of genetic differentiation. A similar observation was made by Das et al. (2012) using mtDNA markers; low SNP marker diversity and lack of genetic differentiation were reported by Hamilton et al. (2019). In general, Fst values ranging from 0.05 to 0.15 suggest a moderate level of genetic differentiation between populations (Wright, 1978). The maximum genetic difference (Fst = 0.0845) was found between the BRM and KRN populations. In contrast, the CAU and GOD populations had the least genetic difference (Fst = 0.0208). The Fst value revealed that KRN samples were significantly different from the BRM, GAN, and MAH samples, whereas BRM samples were observed to be substantially different from GAN. Smaller genetic distance and high gene flow might be the possible reasons for reduced genetic differentiation between the CAU and GOD populations. Our sample of SNPs, which shows low-to-moderate molecular marker differentiation across rivers, revealed minimal genetic differentiation because of adaptive selection or drift and suggested that there had been continuous gene flow amongst the analyzed river basins (Hamilton et al., 2019). As with many other Indo-Malayan fish, L. catla is thought to have invaded India during the Eocene epoch through the Indo-Brahma River, which flows from Assam to the Arabian Sea in a westerly direction, confirming a common ancestry of catla species in the rivers of India (Daniels, 2001). Additionally, in the lack of connectivity among the rivers, low-to-moderate genetic differentiation is most likely the outcome of gene flow brought about by species introduction into waterways, unethical aquaculture practices, environmental catastrophes, and others. Furthermore, AMOVA results revealed that within-population variation was higher (95.32%) in proportion than that in among-population variation (4.68%), which coincides with the results obtained by Hamilton et al. (2019), Sahoo et al. (2018), and Das et al. (2012), and is possibly because of the increased gene flow among the populations (Slatkin and Barton, 1989; Allendorf et al., 2012). This kind of gene flow may lower genetic differentiation and account for the substantial within-population variability (Ferguson et al., 1995), as seen in L. catla samples from six river basins. The fixation index (Fst = 0.04681; p = 0.00000 ± 0.00000) revealed minimal genetic variation among populations (Weir and Cockerham, 1984; Excoffier et al., 1992).
4.3 Linkage disequilibrium
In this study, no significant pairwise linkage disequilibrium was observed among the loci, which is similar to the findings obtained by Das Mahaptra et al. (2018). Our results indicated LD to be entirely decayed at r2 < ∼ 0.2 as it approaches equilibrium. This, along with LD decay at r2 = half decay distance, is a widely used criterion in the literature (Hudson, 2004; VanLiere and Rosenberg, 2008; Vos et al., 2017). An admixed population structure can sometimes lead to reduced levels of linkage disequilibrium (LD) between genetic variants. An admixed population is a population that has arisen from the interbreeding of two or more ancestral populations. As per our result, LD between these variants may be reduced or even eliminated in the admixed population. Furthermore, it is important to note that while admixture can lead to reduced levels of LD between certain genetic variants, the effects of admixture on LD can be complex and context-dependent.
5 Conclusion
In the present investigation, 10,485 genome-wide SNPs were identified using the GBS method for genome-scale analysis in six riverine populations of L. catla. Low genetic diversity was observed with low-to-moderate levels of pairwise genetic differentiation in the six catla populations. Most of the genetic variations were found within populations. Both Bayesian and multivariate techniques divided L. catla into two clusters. The results of this study will help in the conservation and management of wild populations of catla.
Data availability statement
The datasets presented in this study can be found in online repositories. The names of the repository/repositories and accession number(s) can be found at: https://www.ncbi.nlm.nih.gov/, PRJNA886474.
Ethics statement
The animal study was reviewed and approved by the Institutional Animal Ethics Committee (AEC) of ICAR-CIFA.
Author contributions
BS: conceptualization, methodology, data curation, sample collection, tissue extraction and DNA isolation, formal analysis, investigation, and writing—original draft. GD: helped with sample collection, tissue extraction, and DNA isolation. NP: helped in DNA isolation. PN: helped in formal analysis. LS: writing—reviewing and editing. JS: conceptualization. PM: resources. UU: resources. PD: conceptualization, supervision, project administrator, and writing—review and editing.
Funding
The work presented was financially supported by the Department of Biotechnology, Govt. of India, New Delhi, under the Center of Excellence program (No: BT/AAQ/3/SP13797/2017).
Acknowledgments
The authors acknowledge the financial support by the Department of Biotechnology, Govt. of India, New Delhi, under the Center of Excellence program. Authors are thankful to the Director, ICAR-CIFA, Bhubaneswar, for providing the facilities to work under the project.
Conflict of interest
The authors declare that the research was conducted in the absence of any commercial or financial relationships that could be construed as a potential conflict of interest.
Publisher’s note
All claims expressed in this article are solely those of the authors and do not necessarily represent those of their affiliated organizations, or those of the publisher, the editors, and the reviewers. Any product that may be evaluated in this article, or claim that may be made by its manufacturer, is not guaranteed or endorsed by the publisher.
Supplementary material
The Supplementary Material for this article can be found online at: https://www.frontiersin.org/articles/10.3389/fgene.2023.1166385/full#supplementary-material
References
Ahmed, T., and Abbas, K. (2018). Patterns of genetic variability in natural and hatchery populations of Catla catla based on microsatellite DNA markers. Pak. J. Agric. Sci. 55 (4), 929. doi:10.21162/PAKJAS/18.7237
Alam, M., Jahan, M., Hossain, M., and Islam, M. (2009). Population genetic structure of three major river populations of rohu, Labeo rohita (Cyprinidae: Cypriniformes) using microsatellite DNA markers. Genes & Genomics 31 (1), 43–51. doi:10.1007/bf03191137
Alam, M. S., and Islam, M. S. (2005). Population genetic structure of Catla catla (Hamilton) revealed by microsatellite DNA markers. Aquaculture 246 (1-4), 151–160. doi:10.1016/j.aquaculture.2005.02.012
Ali, M. R., Rahi, M. L., Islam, S. S., Shah, M. S., and Shams, F. I. (2015). Genetic variability assay of different strains of Catla catla. Int. J. Life Sci. 9 (1), 37–42. doi:10.3126/ijls.v9i1.11924
Allendorf, F. W., Luikart, G. H., and Aitken, S. N. (2007). Conservation and the genetics of populations. Mammalia 2007, 189–197. doi:10.1515/MAMM.2007.038
Allendorf, F. W., Luikart, G. H., and Aitken, S. N. (2012). Conservation and the genetics of populations. 2nd edition. Oxford: Wiley Blackwell Publishing.
Andrews, S. (2010). Babraham bioinformatics-FastQC: A quality control tool for high throughput sequence data. Available at: http://www.bioinformatics.bbsrc.ac.uk/projects/fastqc.
Babicki, S., Arndt, D., Marcu, A., Liang, Y., Grant, J. R., Maciejewski, A., et al. (2016). Heatmapper: Web-enabled heat mapping for all. Nucleic Acids Res. 44 (1), W147–W153. doi:10.1093/nar/gkw419
Babu, R., and Padmavathi, P. (2016). Interlinking of Krishna and Godavari rivers: An ecological study. Int. J. Fish. Aquatic Stud. 4, 593.
Behera, B. K., Kunal, S. P., Baisvar, V. S., Meena, D. K., Panda, D., Pakrashi, S., et al. (2017). Genetic variation in wild and hatchery population of Catla catla (Hamilton, 1822) analyzed through mtDNA cytochrome b region. Mitochondrial DNA Part A 29 (1), 126–131. doi:10.1080/24701394.2016.1253072
Bradbury, P. J., Zhang, Z., Kroon, D. E., Casstevens, T. M., Ramdoss, Y., and Buckler, E. S. (2007). Tassel: Software for association mapping of complex traits in diverse samples. Bioinformatics 23 (19), 2633–2635. doi:10.1093/bioinformatics/btm308
Catchen, J., Hohenlohe, P. A., Bassham, S., Amores, A., and Cresko, W. A. (2013). Stacks: An analysis tool set for population genomics. Mol. Ecol. 22 (11), 3124–3140. doi:10.1111/mec.12354
Chen, S., Zhou, Y., Chen, Y., and Gu, J. (2018). fastp: an ultra-fast all-in-one FASTQ preprocessor. Bioinformatics 34 (17), i884–i890. doi:10.1093/bioinformatics/bty560
Consuegra, S., Verspoor, E., Knox, D., and García de Leániz, C. (2005). Asymmetric gene flow and the evolutionary maintenance of genetic diversity in small, peripheral Atlantic salmon populations. Conserv. Genet. 6, 823–842. doi:10.1007/s10592-005-9042-4
Correa, K., Lhorente, J. P., López, M. E., Bassini, L., Naswa, S., Deeb, N., et al. (2015). Genome-wide association analysis reveals loci associated with resistance against Piscirickettsia salmonis in two Atlantic salmon (Salmo salar L.) chromosomes. BMC genomics 16 (1), 854–859. doi:10.1186/s12864-015-2038-7
Dandekar, P. (2012). Damaged rivers, collapsing fisheries: Impacts of dams on riverine fisheries in India. Available at: http://sandrp.in/dams/Impacts of Dams on Riverine Fisheries in India Parineeta Dandekar Sep 2012.pdf. [Accessed March 27, 2015].
Danecek, P., Auton, A., Abecasis, G., Albers, C. A., Banks, E., DePristo, M. A., et al. (2011). The variant call format and VCFtools. Bioinformatics 27 (15), 2156–2158. doi:10.1093/bioinformatics/btr330
Daniels, R. R. (2001). Endemic fishes of the western ghats and the satpura hypothesis. Curr. Sci. 81 (3), 240–244.
Das Mahapatra, K., Sahoo, L., Saha, J. N., Murmu, K., Rasal, A., Nandanpawar, P., et al. (2018). Establishment of base population for selective breeding of catla (Catla catla) depending on phenotypic and microsatellite marker information. J. Genet. 97, 1327–1337. doi:10.1007/s12041-018-1034-5
Das, M. K., Samanta, S., Sudheesan, D., Naskar, M., Bandopadhyay, M., Paul, S., et al. (2021). Fish diversity, community structure and ecological integrity of river Brahmani. J. Inland Fish. Soc. India. 48, 1–13. doi:10.47780/jifsi.48.1.2016.116278
Das, R., Mohindra, V., Singh, R. K., Lal, K. K., Punia, P., Masih, P., et al. (2012). Intraspecific genetic diversity in wild Catla catla (Hamilton, 1822) populations assessed through mtDNA cytochrome b sequences. J. Appl. Ichtology 28, 280. doi:10.1111/j.1439-0426.2011.01911.x
Diaz, B. G., Zucchi, M. I., Alves-Pereira, A., de Almeida, C. P., Moraes, A. C. L., Vianna, S. A., et al. (2021). Genome-wide SNP analysis to assess the genetic population structure and diversity of Acrocomia species. PLoS One 16(7), e0241025. doi:10.1371/journal.pone.0241025
Do, C., Waples, R. S., Peel, D., Macbeth, G. M., Tillett, B. J., and Ovenden, J. R. (2014). NeEstimator v2: Re-implementation of software for the estimation of contemporary effective population size (Ne) from genetic data. Mol. Ecol. Resour. 14 (1), 209–214. doi:10.1111/1755-0998.12157
Earl, D. A., and VonHoldt, B. M. (2012). Structure HARVESTER: A website and program for visualizing STRUCTURE output and implementing the Evanno method. Conserv. Genet. Resour. 4, 359–361. doi:10.1007/s12686-011-9548-7
Elshire, R. J., Glaubitz, J. C., Sun, Q., Poland, J. A., Kawamoto, K., Buckler, E. S., et al. (2011). A robust, simple genotyping-by-sequencing (GBS) approach for high diversity species. PLoS One 6, e19379. doi:10.1371/journal.pone.0019379
Escalante, M. A., Perrier, C., García-De León, F. J., Ruiz-Luna, A., Ortega-Abboud, E., and Manel, S. (2020). Genotyping-by-sequencing reveals the effects of riverscape, climate and interspecific introgression on the genetic diversity and local adaptation of the endangered Mexican golden trout (Oncorhynchus chrysogaster). Conserv. Genet. 21, 907–926. doi:10.1007/s10592-020-01297-z
Evanno, G., Regnaut, S., and Goudet, J. (2005). Detecting the number of clusters of individuals using the software STRUCTURE: A simulation study. Mol. Ecol. 14 (8), 2611–2620. doi:10.1111/j.1365-294X.2005.02553.x
Everett, M. V., and Seeb, J. E. (2014). Detection and mapping of QTL for temperature tolerance and body size in Chinook salmon (Oncorhynchus tshawytscha) using genotyping by sequencing. Evol. Appl. 7 (4), 480–492. doi:10.1111/eva.12147
Ewels, P., Magnusson, M., Lundin, S., and Käller, M. (2016). MultiQC: Summarize analysis results for multiple tools and samples in a single report. Bioinformatics 32 (19), 3047–3048. doi:10.1093/bioinformatics/btw354
Excoffier, L., and Lischer, H. E. (2010). Arlequin suite ver 3.5: A new series of programs to perform population genetics analyses under linux and windows. Mol. Ecol. Resour. 10 (3), 564–567. doi:10.1111/j.1755-0998.2010.02847.x
Excoffier, L., Smouse, P. E., and Quattro, J. (1992). Analysis of molecular variance inferred from metric distances among DNA haplotypes: Application to human mitochondrial DNA restriction data. Genetics 131 (2), 479–491. doi:10.1093/genetics/131.2.479
Fagbemi, M. N. A., Pigneur, L. M., Andre, A., Smitz, N., Gennotte, V., Michaux, J. R., et al. (2021). Genetic structure of wild and farmed Nile tilapia (Oreochromis niloticus) populations in Benin based on genome-wide SNP technology. Aquaculture 535, 736432. doi:10.1016/j.aquaculture.2021.736432
FAO (2006–2020). Fisheries and aquaculture topics. Statistics - introduction. Rome: Topics Fact Sheets. FAO Fisheries and Aquaculture Department.
FAO. (2000-2020). Fisheries and aquaculture. Global aquaculture production quantity. Available at: https://www.fao.org/fishery/statistics-query/en/aquaculture/aquaculture_quantity. [Accessed March 19, 2023].
Faroque, M. A., Minar, M. H., Nesa, N. U., Sarder, M. R. I., and Mollah, M. F. A. (2021). Genetic characterisation of wild catla (Catla catla, Hamilton) populations using microsatellite DNA markers. Bangladesh J. Fish. Res. 33 (2), 167–176. doi:10.52168/bjf.2021.33.19
Felsenstein, J. (1997). “Population differentiation and evolutionary processes,” in Genetic effects of straying of non-native hatchery fish into natural populations. Editor W. S. Grant (Washington, DC: U. S. Dept. Comm., NOAA tech. Mem.), 31–43.
Ferguson, A., Taggart, J. B., Prodohl, P. A., McMeel, O., Thompson, C., Stone, C., et al. (1995). The application of molecular markers to the study and conservation of fish populations, with special reference to Salmo. Salmo. J. Fish. Biol. 47, 103–126. doi:10.1111/j.1095-8649.1995.tb06048.x
Frankham, R. (2005). Genetics and extinction. Biol. Conserv. 126 (2), 131–140. doi:10.1016/j.biocon.2005.05.002
Fraser, D. J., Jones, M. W., McParland, T. L., and Hutchings, J. A. (2007). Loss of historical immigration and the unsuccessful rehabilitation of extirpated salmon populations. Conserv. Genet. 8, 527–546. doi:10.1007/s10592-006-9188-8
Ginestet, C. (2011). ggplot2: elegant graphics for data analysis. J. R. Stat. Soc. Ser. A 174, 50–56. doi:10.1038/hdy.2012.12
Goodall-Copestake, W. P., Tarling, G. A., and Murphy, E. (2012). On the comparison of population-level estimates of haplotype and nucleotide diversity: A case study using the gene cox1 in animals. Heredity 109 (1), 50–56. doi:10.1038/hdy.2012.12
Grant, W. A. S., and Bowen, B. W. (1998). Shallow population histories in deep evolutionary lineages of marine fishes: Insights from sardines and anchovies and lessons for conservation. J. Hered. 89, 415–426. doi:10.1093/jhered/89.5.415
Gutierrez, A. P., Yáñez, J. M., Fukui, S., Swift, B., and Davidson, W. S. (2015). Genome-wide association study (GWAS) for growth rate and age at sexual maturation in Atlantic salmon (Salmo salar). PloS one 10 (3), e0119730. doi:10.1371/journal.pone.0119730
Habib, M., Lakra, W. S., Mohindra, V., Lal, K. K., Punia, P., Singh, R. K., et al. (2012). Assessment of ATPase 8 and ATPase 6 mtDNA sequences in genetic diversity studies of Channa marulius (Channidae: Perciformes). Proc. Natl. Acad. Sci. India Sect. B- Biol. Sci. 82, 497–501. doi:10.1007/s40011-012-0061-x
Hamilton, F. (1822). An account of the fishes found in the River Ganges and its branches. Edinburg: Archibald Constable and Company. 405.
Hamilton, M. G., Mekkawy, W., and Benzie, J. A. (2019). Sibship assignment to the founders of a Bangladeshi Catla catla breeding population. Genet. Sel. Evol. 51 (1), 17–18. doi:10.1186/s12711-019-0454-x
Hammerschlag, N., Meyer, C. G., Grace, M. S., Kessel, S. T., Sutton, T. T., Harvey, E. S., et al. (2017). Shining a light on fish at night: An overview of fish and fisheries in the dark of night, and in deep and polar seas. Bull. Mar. Sci. 93 (2), 253–284. doi:10.5343/bms.2016.1082
Hartl, D. L., and Clark, A. G. (1997). Principles of population genetics. 3rd edition. Sunderlands, Massachusetts: Sinauer Associates, Inc, 542.
Hubisz, M. J., Falush, D., Stephens, M., and Pritchard, J. K. (2009). Inferring weak population structure with the assistance of sample group information. Mol. Ecol. Resour. 9, 1322–1332. doi:10.1111/j.1755-0998.2009.02591.x
Hudson, R. (2004). “Linkage disequilibrium and recombination,” in Handbook of statistical genetics. Chapter 22. John Wiley Sons, Ltd. doi:10.1002/0470022620.bbc23
Imam, S. (2020). Population growth and urbanization in Bihar: A district level analysis. Int. J. Sci. Res. 2020, 2319–7064. doi:10.21275/SR21814213203
Islam, M. S., Ahmed, A. S. I., Azam, M. S., and Alam, M. S. (2005). Genetic analysis of three river populations of Catla catla (Hamilton) using randomly amplified polymorphic DNA markers. Asian-australas. J. Anim. Sci. 18 (4), 453–457. doi:10.5713/ajas.2005.453
Islam, M. S., and Alam, M. S. (2004). Randomly amplified polymorphic DNA analysis of four different populations of the Indian major carp, Labeo rohita (Hamilton). J. Appl. Ichthyol. 20 (5), 407–412. doi:10.1111/j.1439-0426.2004.00588.x
Jadhav, B. V., Kharat, S. S., Raut, R. N., Paingankar, M., and Dahanukar, N. (2011). Freshwater fish fauna of koyna river, northern western ghats, India. J. Threat. Taxa 3 (1), 1449–1455. doi:10.11609/jott.o2613.1449-55
Jombart, T. (2008). adegenet: a R package for the multivariate analysis of genetic markers. Bioinformatics 24, 1403–1405. doi:10.1093/bioinformatics/btn129
Jombart, T., and Ahmed, I. (2011). Adegenet 1.3-1: new tools for the analysis of genome-wide SNP data. Bioinformatics 27, 3070–3071. doi:10.1093/bioinformatics/btr521
Jombart, T., Devillard, S., and Balloux, F. (2010). Discriminant analysis of principal components: A new method for the analysis of genetically structured populations. BMC Genet. 11, 94. doi:10.1186/1471-2156-11-94
Kopelman, N. M., Mayzel, J., Jakobsson, M., Rosenberg, N. A., and Mayrose, I. (2015). Clumpak: A program for identifying clustering modes and packaging population structure inferences across K. Mol. Ecol. Resour. 15 (5), 1179–1191. doi:10.1111/1755-0998.12387
Kumar, S., Sarthi, P. P., Kumar, P., and Ghosh, S. (2014). “Study of changing path of the Ganga through LISS III satellite data,” in International conference on science and technology. Bihar Science Conference Patna, Bihar, India, December 23rd-25th, 2014.
Kumar, S. T., Kumar, S. S., and Charan, G. B. (2020). Fish diversity of Mahanadi River (Odisha part), threats and conservation measures. Int. J. Life Sci. 8 (2), 355–371.
Li, H. (2013). Aligning sequence reads, clone sequences and assembly contigs with BWA-MEM. arXiv preprint arXiv:1303.399.
Li, H., Handsaker, B., Wysoker, A., Fennell, T., Ruan, J., Homer, N., et al. (2009). The sequence alignment/map format and SAMtools. Bioinformatics 25(16), pp.2078–2079. doi:10.1093/bioinformatics/btp352
Lischer, H. E., and Excoffier, L. (2012). PGDSpider: An automated data conversion tool for connecting population genetics and genomics programs. Bioinformatics 28 (2), 298–299. doi:10.1093/bioinformatics/btr642
Luo, Z., Yu, Y., Bao, Z., Xiang, J., and Li, F. (2022). Evaluation of genomic selection for high salinity tolerance traits in Pacific white shrimp Litopenaeus vannamei. Aquaculture 557, 738320. doi:10.1016/j.aquaculture.2022.738320
Luo, Z., Yu, Y., Xiang, J., and Li, F. (2021). Genomic selection using a subset of SNPs identified by genome-wide association analysis for disease resistance traits in aquaculture species. Aquaculture 539, 736620. doi:10.1016/j.aquaculture.2021.736620
Malini, B. H., and Rao, K. N. (2004). Coastal erosion and habitat loss along the Godavari delta front-a fallout of dam construction (?). Curr. Sci. 87 (9), 1232–1236.
Mandal, T., Sharma, B. B., and Dash, G. (2014). Parasitic disease management strategies in the carp hatcheries of West Bengal, India. Int. J. Adv. Sci. Tech. Res. 4, 156–164.
Murthy, H. S. (2002). Indian major carps. Nutrient requirements and feeding of finfish for aquaculture. Wallingford, United Kingdom: CABI Publishing, 262–272.
Nath, T. K., Tripathy, B., and Das, A. (2018). A study of water quality of River Brahmani, Odisha (India) to assess its potability. Int. J. Eng. Res. Technol. 7 (7), 301–311.
Nomura, T. (2008). Estimation of effective number of breeders from molecular coancestry of single cohort sample. Evol. Appl. 1 (3), 462–474. doi:10.1111/j.1752-4571.2008.00015.x
Nyinondi, C. S., Mtolera, M. S., Mmochi, A. J., Lopes Pinto, F. A., Houston, R. D., de Koning, D. J., et al. (2020). Assessing the genetic diversity of farmed and wild Rufiji tilapia (Oreochromis urolepis urolepis) populations using ddRAD sequencing. Ecol. Evol. 10 (18), 10044–10056. doi:10.1002/ece3.6664
Paradis, E., and Schliep, K. (2019). Ape 5.0: an environment for modern phylogenetics and evolutionary analyses in R. Bioinformatics 35 (3), 526–528. doi:10.1093/bioinformatics/bty633
Payne, A. I., Sinha, R., Singh, H. R., and Huq, S. (2004). “A review of Ganga basin: Its fish and fisheries,” in Proceedings of the second International symposium on the management of large Rivers for fisheries, sustaining livelihood and biodiversity in the new millennium Editors R. Welcomme, and T. Petr (Bangkok, Thailand: RAP publication), I. 2004/16.
Rahman, S. M., Khan, M. R., Islam, S., and Alam, S. (2009). Genetic variation of wild and hatchery populations of the catla Indian major carp (Catla catla Hamilton 1822: Cypriniformes, Cyprinidae) revealed by RAPD markers. Genet. Mol. Biol. 32 (1), 197–201. doi:10.1590/S1415-47572009005000013
Rao, Y. H., and Ravindhranath, K. (2013). Thermal and heavy metal ions pollution assessment in near by water bodies of Vijayawada thermal power station. Chem. Asian J. 25 (3). doi:10.14233/ajchem.2013.13133
Reddy, P. V. G. K. (2005). Carp genetic resources of India, carp genetic resources for aquaculture in Asia. Malaysia: World Fish Center publishing Inc, 39–53.
Rochette, N. C., Rivera-Colón, A. G., and Catchen, J. M. (2019). Stacks 2: Analytical methods for paired-end sequencing improve RADseq-based population genomics. Mol. Ecol. 28 (21), 4737–4754. doi:10.1111/mec.15253
Sahoo, L., Das, P., Sahoo, B., Das, G., Meher, P. K., Udit, U. K., et al. (2020). The draft genome of Labeo catla. BMC Res. Notes. 13 (1), 411–413. doi:10.1186/s13104-020-05240-w
Sahoo, L., Mohanty, M., Meher, P. K., Murmu, K., Sundaray, J. K., and Das, P. (2018). Population structure and genetic diversity of hatchery stocks as revealed by combined mtDNA fragment sequences in Indian major carp, Catla catla. Mitochondrial DNA Part A 30 (2), 289–295. doi:10.1080/24701394.2018.1484120
Sarder, M. R. I., Yeasin, M., Jewel, M. Z. H., Khan, M. M. R., and Simonsen, V. (2011). Identification of Indian major carps (Catla catla, Labeo rohita and Cirrhinus cirrhosus) and their hybrids by phenotypic traits, allozymes and food habits. Asian Fish. Sci. 24 (1), 49–61. doi:10.33997/j.afs.2011.24.1.005
Sarmah, B., and Sarmah, B. (2016). mtDNA cytochrome-b gene assisted genetic diversity study of Catla catla. Int. J. Fish. Aquat. Stud. 4, 268–272.
Simpson, S. D., Radford, A. N., Nedelec, S. L., Ferrari, M. C., Chivers, D. P., McCormick, M. I., et al. (2016). Anthropogenic noise increases fish mortality by predation. Nat. Commun. 7 (1), 10544. doi:10.1038/ncomms10544
Singh, A. K., Kumar, D., Srivastava, S. C., Ansari, A., Jena, J. K., and Sarkar, U. K. (2013). Invasion and impacts of alien fish species in the Ganga River, India. Aquat. Ecosyst. Health Manag. 16 (4), 408–414. doi:10.1080/14634988.2013.857974
Slatkin, M., and Barton, N. H. (1989). A comparison of three indirect methods for estimating average levels of gene flow. Evol 43 (7), 1349–1368. doi:10.1111/j.1558-5646.1989.tb02587.x
Sravya, P., Kumar, M. R., Sreejani, T., and Rao, G. S. (2017). Multivariate statistical analysis of Godavari river water quality for irrigation purposes at Rajahmundry and Dhawaleswaram, AP. Int. J. Civ. Struct. Environ. Infrastructure Eng. Res. Dev. 7, 2249–6866. ISSN (E): 2249-7978.
Susheela, S., Srikantaswamy, S., Shiva Kumar, F. D., Gowda, F. A., and Jagadish, F. K. (2014). Study of Cauvery river water pollution and its impact on socio-economic status around KRS Dam, Karnataka, India. J. Earth Sci. Geotech. Eng. 4 (2), 91–109.
Swain, A. K., Jena, M., Das, B., and Swain, R. (2017). “Concern over pollution in two major rivers of Odisha, India: A case study of Mahanadi and Brahmani rivers,” in Proceeding of the National conference on Advances in Water Resource and Environment Research, Qingdao, China, 29-30 June, 2017, 23.
Uchino, T., Hosoda, E., Nakamura, Y., Yasuike, M., Mekuchi, M., Sekino, M., et al. (2018). Genotyping-by-sequencing for construction of a new genetic linkage map and QTL analysis of growth-related traits in Pacific bluefin tuna. Aquac. Res. 49 (3), 1293–1301. doi:10.1111/are.13584
VanLiere, J. M., and Rosenberg, N. A. (2008). Mathematical properties of the r2 measure of linkage disequilibrium. Theor. Popul. Biol. 74 (1), 130–137. doi:10.1016/j.tpb.2008.05.006
Vos, P. G., Paulo, M. J., Voorrips, R. E., Visser, R. G., van Eck, H. J., and van Eeuwijk, F. A. (2017). Evaluation of LD decay and various LD-decay estimators in simulated and SNP-array data of tetraploid potato. Theor. Appl. Genet. 130 (1), 123–135. doi:10.1007/s00122-016-2798-8
Wang, L., Liu, P., Huang, S., Ye, B., Chua, E., Wan, Z. Y., et al. (2017). Genome-wide association study identifies loci associated with resistance to viral nervous necrosis disease in Asian seabass. Mar. Biotechnol. 19 (3), 255–265. doi:10.1007/s10126-017-9747-7
Wang, Q., Yu, Y., Zhang, Q., Zhang, X., Huang, H., Xiang, J., et al. (2019). Evaluation on the genomic selection in Litopenaeus vannamei for the resistance against Vibrio parahaemolyticus. Aquaculture 505, 212–225. doi:10.1016/j.ijbiomac.2019.05.101
Wang, Z., Hu, H., Sun, T., Li, X., Lv, G., Bai, Z., et al. (2022). Genomic selection for improvement of growth traits in triangle sail mussel (Hyriopsis cumingii). Aquaculture 561, 738692. doi:10.1016/j.aquaculture.2022.738692
Weir, B. S., and Cockerham, C. C. (1984). Estimating F-statistics for the analysis of population structure. Evol 38, 1358–1370. doi:10.1111/j.1558-5646.1984.tb05657.x
Wolc, A., Zhao, H. H., Arango, J., Settar, P., Fulton, J. E., O’sullivan, N. P., et al. (2015). Response and inbreeding from a genomic selection experiment in layer chickens. Genet. Sel. Evol. 47 (1), 59. doi:10.1186/s12711-015-0133-5
Wright, S. (1978). “Evolution and the genetics of populations,” in Variability within and among natural population (Chicago, IL: University of Chicago Press), 4.
Yang, T. Y., Gao, T. X., Meng, W., and Jiang, Y. L. (2020). Genome-wide population structure and genetic diversity of Japanese whiting (Sillago japonica) inferred from genotyping-by-sequencing (GBS): Implications for fisheries management. Fish. Res. 225, 105501. doi:10.1016/j.fishres.2020.105501
Yin, X., Arias-Pérez, A., Kitapci, T. H., and Hedgecock, D. (2020). High-density linkage maps based on Genotyping-by-Sequencing (GBS) confirm a chromosome-level genome assembly and reveal variation in recombination rate for the Pacific oyster Crassostrea gigas. G3 Genes, Genomes, Genet. 10 (12), 4691–4705. doi:10.1534/g3.120.401728
Keywords: single nucleotide polymorphisms (SNPs), genotyping-by-sequencing (GBS), Labeo catla, population genomics, genetic differentiation
Citation: Sahoo B, Das G, Nandanpawar P, Priyadarshini N, Sahoo L, Meher PK, Udit UK, Sundaray JK and Das P (2023) Genetic diversity and genome-scale population structure of wild Indian major carp, Labeo catla (Hamilton, 1822), revealed by genotyping-by-sequencing. Front. Genet. 14:1166385. doi: 10.3389/fgene.2023.1166385
Received: 15 February 2023; Accepted: 10 April 2023;
Published: 09 May 2023.
Edited by:
Diogo Teruo Hashimoto, São Paulo State University, BrazilReviewed by:
Vito Antonio Mastrochirico Filho, Universidade Estadual Paulista, BrazilManojit Bhattacharya, Fakir Mohan University, India
Ajaya Kumar Rout, Central Inland Fisheries Research Institute (ICAR), India
Copyright © 2023 Sahoo, Das, Nandanpawar, Priyadarshini, Sahoo, Meher, Udit, Sundaray and Das. This is an open-access article distributed under the terms of the Creative Commons Attribution License (CC BY). The use, distribution or reproduction in other forums is permitted, provided the original author(s) and the copyright owner(s) are credited and that the original publication in this journal is cited, in accordance with accepted academic practice. No use, distribution or reproduction is permitted which does not comply with these terms.
*Correspondence: Paramananda Das, cGRhczc3QGhvdG1haWwuY29t