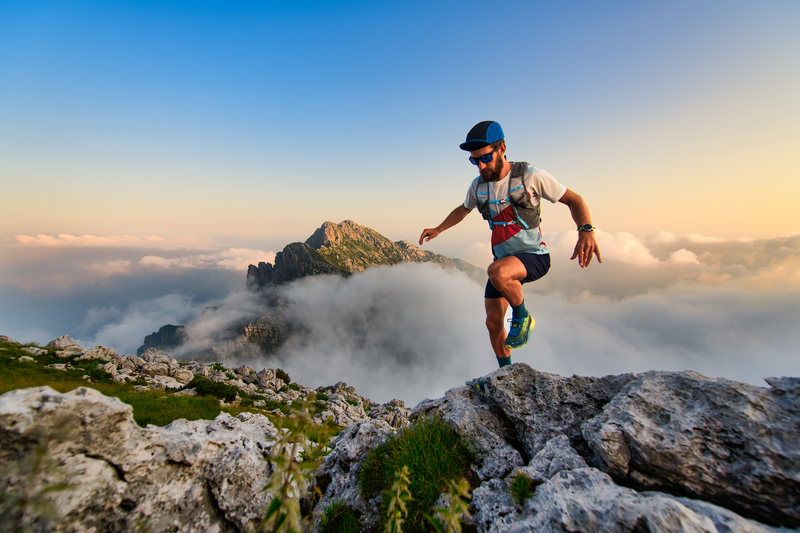
94% of researchers rate our articles as excellent or good
Learn more about the work of our research integrity team to safeguard the quality of each article we publish.
Find out more
ORIGINAL RESEARCH article
Front. Genet. , 04 April 2023
Sec. Evolutionary and Population Genetics
Volume 14 - 2023 | https://doi.org/10.3389/fgene.2023.1144221
This article is part of the Research Topic Genetic Basis of Adaptation to Extreme Environments Ⅱ View all 6 articles
Introduction: Heavy metal pollutants can have long lasting negative impacts on ecosystem health and can shape the evolution of species. The persistent and ubiquitous nature of heavy metal pollution provides an opportunity to characterize the genetic mechanisms that contribute to metal resistance in natural populations.
Methods: We examined variation in resistance to copper, a common heavy metal contaminant, using wild collections of the model organism Drosophila melanogaster. Flies were collected from multiple sites that varied in copper contamination risk. We characterized phenotypic variation in copper resistance within and among populations using bulked segregant analysis to identify regions of the genome that contribute to copper resistance.
Results and Discussion: Copper resistance varied among wild populations with a clear correspondence between resistance level and historical exposure to copper. We identified 288 SNPs distributed across the genome associated with copper resistance. Many SNPs had population-specific effects, but some had consistent effects on copper resistance in all populations. Significant SNPs map to several novel candidate genes involved in refolding disrupted proteins, energy production, and mitochondrial function. We also identified one SNP with consistent effects on copper resistance in all populations near CG11825, a gene involved in copper homeostasis and copper resistance. We compared the genetic signatures of copper resistance in the wild-derived populations to genetic control of copper resistance in the Drosophila Synthetic Population Resource (DSPR) and the Drosophila Genetic Reference Panel (DGRP), two copper-naïve laboratory populations. In addition to CG11825, which was identified as a candidate gene in the wild-derived populations and previously in the DSPR, there was modest overlap of copper-associated SNPs between the wild-derived populations and laboratory populations. Thirty-one SNPs associated with copper resistance in wild-derived populations fell within regions of the genome that were associated with copper resistance in the DSPR in a prior study. Collectively, our results demonstrate that the genetic control of copper resistance is highly polygenic, and that several loci can be clearly linked to genes involved in heavy metal toxicity response. The mixture of parallel and population-specific SNPs points to a complex interplay between genetic background and the selection regime that modifies the effects of genetic variation on copper resistance.
Heavy metals are naturally occurring elements enriched in some environments owing to industry and agriculture due to their usefulness as conductive materials (Karnchanawong and Limpiteeprakan, 2009) and as components of pesticides (Schutte et al., 1997; Roca et al., 2007). More than 5 million sites world-wide are polluted with dangerous levels of heavy metals and metalloids (He et al., 2015), and mismanagement of mining and industry waste as well as widespread pesticide use in agriculture are among the primary drivers of heavy metal pollution (Liu et al., 2018; Kang et al., 2020). When heavy metals become highly concentrated in soil and water, they pose a serious threat to ecosystem health (Li et al., 2019; Vareda et al., 2019). Additionally, because heavy metals are not biodegradable and persist and accumulate in contaminated sites (Sánchez-Chardi and Nadal, 2007; Ali et al., 2019; Ali and Khan, 2019), they can drive adaptation in populations. Indeed, variation in heavy metal resistance and adaptation has been linked to pollution in many species (e.g., Ashida, 1965; McNeilly, 1968; MacNair et al., 1993; Roelofs et al., 2006; Martins et al., 2007; Li et al., 2017; Kang et al., 2020; Li et al., 2021).
Numerous genes and pathways have the potential to contribute to metal resistance (Merritt and Bewick, 2017). For example, genes that regulate metabolism and homeostasis of biologically necessary heavy metals (such as copper and zinc) also interact with metals that have no biological role (such as cadmium and lead) (Olaniran et al., 2013; Calap-Quintana et al., 2017). Metallothioneins (Mtns), a small family of metal scavenger proteins present in prokaryotes and eukaryotes that likely arose through gene duplication events (Maroni and Watson, 1985; Moleirinho et al., 2011), fall into this category. Mtns primarily bind and sequester excess copper or zinc ions to reduce their intracellular concentration, but will also bind and thus help detoxify cadmium ions (Egli et al., 2003; Egli et al., 2006; Luo et al., 2020). Mtn sequence and expression variation has been previously linked to variation in metal resistance in insects and humans (Roelofs et al., 2006; Janssens et al., 2009; Adams et al., 2015; Catalán et al., 2016), suggesting they may play a role in adaptive responses to metal pollution.
Cytochrome p450s (Cyp450s), glutathione s transferases (Gsts), ABC-type transporters, and heat shock proteins (HSPs) all respond to a wide range of xenobiotic stressors and also have the potential to contribute to heavy metal resistance (Enayati et al., 2005; González-Guerrero et al., 2010; Gupta et al., 2010; Cao et al., 2017; Chain et al., 2019; Lu et al., 2021). For instance, in the mosquito Aedes aegypti, larval exposure to copper increased expression of cytochrome p450s, which ultimately led to greater resistance of larvae to insecticides (Poupardin et al., 2008). The potential for interplay between heavy metal pollution and chemicals used to reduce noxious mosquito populations has linked implications for both the evolution of the species as well as control of diseases that impact human populations (Poupardin et al., 2008; Riaz et al., 2009). Given the broad range of chemical stressors that induce them, Cyp450s, Gsts, HSPs, and Mtns have all been suggested as biomarkers of heavy metal pollution (Pyza et al., 1997; Tabrez and Ahmad, 2013; Saad et al., 2016) and both protein-coding and regulatory variation in or near these gene families could contribute to metal adaptation.
An important question is how frequently metal adaptation is conferred by a single major mutation as opposed to the aggregate effects of many loci. There are certainly well documented cases of adaptation to metals via mutations of large effect. For instance, populations of Mimulus guttatus growing in copper mine tailings in Copperopolis, CA, USA evolved copper resistance due to rapid evolution at the Tol1 locus (MacNair, 1983; Wright et al., 2013). In Saccharomyces cerevisiae, copper resistance was linked to copy number variation of the copper chelating CUP1 locus (Fogel and Welch, 1982; Karin et al., 1984). However, when variation in metal resistance has been examined using laboratory-derived populations the trait appears to be polygenic (Mahapatra et al., 2010; Ehrenreich et al., 2012; Zhou et al., 2016; Zhou et al., 2017; Evans et al., 2018; Everman et al., 2021), suggesting that single locus, large effect mutations that lead to adaptation may be rare. The fact that the laboratory populations used in most of these studies were naïve to metal stress motivated the present study: We examine multiple populations with varying exposure to metal pollution to better understand and characterize the genetic complexity underlying metal adaption in natural populations.
We characterized variation in copper resistance in multiple natural populations of D. melanogaster collected from sites influenced by local mining and agricultural activities. In addition, we characterized copper resistance in two independently derived wild-type Drosophila laboratory populations [Drosophila Synthetic Population Resource (DSPR) and the Drosophila Synthetic Reference Panel (DGRP)] to provide a comparison between our wild-collected populations and copper-naïve populations. We focused on copper because it is a common metal pollutant that is toxic at high concentrations (Olaniran et al., 2013; Zuily et al., 2022) but also one of the few heavy metals that is biologically essential (Calap-Quintana et al., 2017). Furthermore, Green et al. (2022) found that copper resistance varied in natural populations of Drosophila melanogaster and may be influenced by pollution associated with urbanization. D. melanogaster is an excellent model for assessing the impacts of copper pollution because populations are widely distributed in both contaminated and uncontaminated sites. In addition, there is extensive overlap of metal responsive genes between D. melanogaster and humans, and flies have been widely used as a model to characterize the genetic response to metal stress (Egli et al., 2006; Hua et al., 2011; Abnoos et al., 2013; Zhou et al., 2016; Calap-Quintana et al., 2017; Zhou et al., 2017; Everman et al., 2021).
We collected D. melanogaster from five sites that varied in heavy metal pollution level and found a positive association between copper resistance and the level of metal pollution at the site. We then used a pooled sequencing, bulked segregant analysis approach (Kelly and Hughes, 2019) to identify genetically variable loci associated with copper resistance in each population. We identified 288 SNPs and several potential candidate genes that were associated with variation in copper resistance. There was limited overlap in the genetic architecture of copper resistance in the wild-derived populations and the DGRP; however, overlap was more extensive between the wild-derived populations and the DSPR. Candidate genes included those that have been previously linked to copper metabolism, homeostasis, and toxicity as well as genes that represent novel candidates for copper resistance. By examining the genetic control of copper resistance in multiple wild-derived populations as well as against known copper-naïve DSPR and DGRP laboratory populations, we were able to identify loci that had similar effects across populations, as well as loci that had population-specific effects, which may depend on a combination of genetic background, selection intensity, and evolutionary history.
We collected flies from five field sites in the United States including two orchards (Duncan’s Berry Farm, DBF; Rees’ Fruit Farm, RFF), a small open-air market (Gilliland Orchard, GPO), and two mines (Anschutz Mine, AMM; Burra Burra Mine, BBM; Table 1 and Figure 1A). DBF is a small blueberry farm in Smithville, MO, USA and RFF is a fruit farm in Topeka, KS, USA that grows a variety of fruit including raspberries, apples, tomatoes, peaches, and apricots. Copper-containing pesticide use at the DBF and RFF sites is limited (personal comm. to ERE from DBF and RFF owners). The GPO site was in an open-air market in Cleveland, TN, USA, which does not have any on-site fruit production. During the collection period, the market was selling peaches grown in Hogback, SC, USA (∼240 km from the market, personal communication to ERE).
TABLE 1. Collection dates, number of D. melanogaster females collected at each site, and levels of replication for experiments.
FIGURE 1. Sites and traps used to collect Drosophila melanogaster. (A) Flies were collected from two orchards (DBF = Duncan’s Berry Farm; RFF = Rees’ Fruit Farm), two mine sites (AMM = Anschutz Mine; BBM = Burra Burra Mine), and one open-air market (GPO = Gilliland Orchard). (B) A representation of 16oz plastic bottles baited with banana, yeast, and water that were used to collect flies (created with BioRender.com). Small holes (4 mm diameter) were melted into the sides of bottles in a grid pattern, and a 15 cm wooden craft stick was provided to prevent flies from sticking in the bait or the side of the bottle.
AMM (known historically as the Madison Mine) is an active cobalt mine in Fredericktown, MO, USA and is one of several mines located in the lead belt of Missouri. AMM currently encompasses 0.59 km2 and has been linked to metal contamination of soil and water at levels of environmental concern (Rangen and Mosby, 2015). A remedial investigation conducted in 2011 found elevated levels of several metals, including copper, in sediment of the nearby Little St. Francis River chat pile (3.5 km from collection site) (Rangen and Mosby, 2015). Flies were collected outside the Anschutz Mine premises less than 6 m from a flowing mine shaft (contaminated ground water exiting a mine shaft opening). BBM is a decommissioned copper mine which operated from 1899–1958 (Illo, 2009) and was the largest of three primary mines in the Copper Basin Mining District in Tennessee (Poste, 1932). BBM is currently listed as an non-NPL (National Priorities List) Superfund Site (EPA, 2011). Lee et al. (2002) reported elevated levels of copper in addition to zinc, cobalt, nickel, lead, and cadmium in creeks (North Potato watershed, David’s Mill Creek) that flow through the Copper Basin region. Flies were collected approximately 0.2 km from the Burra Burra mine pit.
We collected flies from wild populations daily for 3–6 days between June and September (DBF flies were collected in 2018, all other populations were collected in 2019; Table 1) using ten 16oz clear plastic bottle traps baited with half of one banana mixed with approximately one teaspoon of yeast and 10 mL water (Figure 1B). We melted 4 mm diameter holes into the top half of the bottles with one hole approximately every centimeter in a 3 by 6 grid (Figure 1B). A 15 cm wooden craft stick (e.g., Carolina Biological 971662) was placed in each trap to provide an area for flies to land. Traps were suspended with string from trees or bushes so that they remained in the shade and were replaced daily. Individual gravid females were aspirated from traps into cornmeal-molasses-yeast vials to lay eggs for 5 days to obtain males for species verification and to obtain flies to establish cage populations. Cage populations (30 cm × 30 cm × 30 cm, Bugdorm-1) were established for the DBF, GPO, and BBM collections using 20 virgin females and males from each site-specific founder D. melanogaster female. Given the large number of females collected from the RFF site, we used 5 virgin females and males to establish the RFF population cage to avoid overcrowding. Since only 20 females were obtained from the AMM site, we did not establish a population cage and instead maintained isofemale strains that were used to measure copper resistance.
We also assessed copper resistance in a subset of the Drosophila Synthetic Population Resource (DSPR, 194 strains, (King et al., 2012)) and the Drosophila Genetic Reference Panel (DGRP, 152 strains, (Mackay et al., 2012)). The DSPR and DGRP are two large panels of D. melanogaster inbred strains. These strains were included as representatives of heavy metal-naïve populations as they are derived from ancestral populations with low likelihood of exposure to heavy metals and have been maintained in standard laboratory conditions for at least a decade (the DSPR strains were derived from a global sampling of individuals collected before 1970 and were inbred by 2009; DGRP females were collected from an open-air farmer’s market in 2003 (Mackay et al., 2012)).
We assessed copper resistance (our focal trait) and starvation resistance (a representation of a non-metal stress trait) in the wild-derived flies in the third generation removed from the field (after one generation of mating freely in population cages) to limit the opportunity for lab adaptation. DBF, RFF, GPO, and BBM were measured as populations for both traits. AMM, DGRP, and DSPR were measured as isofemale/inbred strains for copper resistance only. Starvation resistance was measured previously in the DSPR and DGRP populations (Everman et al., 2019). We did not measure starvation resistance in the AMM population because this population had relatively few founder females and was not included in subsequent sequencing experiment. Females were used in all experiments. We focused on females to facilitate comparison with previously published studies and because females are the homogametic sex, which simplifies population genetic analyses. Experimental females were obtained from population cages by collecting embryos on apple juice agar plates baited with yeast paste (equal parts yeast and water). Apple juice plates were placed in the cages overnight and embryos were collected the following morning by suspending embryos in 1x PBS and rinsing them into a 50 mL conical tube. After the embryos settled to the bottom of the tube, 12 uL aliquots of embryos were distributed into cornmeal-molasses-yeast food vials. To obtain experimental females from the AMM isofemale strains and the DSPR and DGRP strains, we allowed adult females to oviposit in cornmeal-molasses-yeast vials for 2 days before removing all adults. All vials containing embryos underwent development in the same incubator at 25°C and ∼50% humidity on a 12:12 L:D cycle.
Flies were allowed to eclose for 2 days and then were transferred to new food vials for 24 h to ensure most if not all experimental females were mated. Experimental females were sorted from males into groups of 20 over CO2 anesthesia, placed in new cornmeal-molasses-yeast food vials, and were allowed to recover for 24 h. Following recovery, experimental females were transferred without CO2 into vials containing either 1.8 g Instant Drosophila Media (Carolina Biological Supply Company 173200) hydrated with 8 mL 50 mM Copper (II) Sulfate (CuSO4; Sigma-Aldrich C1297) or starvation media (1.5% agar with media preservatives prepared as described in Everman et al. (2019)). Copper and starvation resistance were assessed by counting the number of dead individuals every 24 h. When approximately 150 copper-exposed females from the DBF, RFF, GPO, and BBM populations remained alive, exactly 150 individuals from each population were collected and frozen for DNA extraction (described below). Counts of females tested for each trait and each population are summarized in Table 1.
We calculated copper and starvation resistance as the average time (in hours) until death per vial. Resistance measured in the AMM, DSPR, and DGRP populations is reported as average lifespan per strain. All phenotype residuals were close to normally distributed and our sample sizes are large, so we proceeded with parametric tests since our tests are likely robust to slight deviations from model assumptions. Variation in copper and starvation resistance was assessed separately with one-way analyses of variance (ANOVA), testing the effect of population on variation in average lifespan. We used Tukey’s HSD post hoc comparisons to test for significant differences among populations.
To determine whether copper and starvation resistance may be phenotypically correlated, we used a general linear model to test for a correlation between mean strain-specific measures of the two stress traits in the DSPR and the DGRP. We could not determine whether the two stress traits were correlated within each of the wild-derived populations because each individual fly is genetically distinct (whereas the DSPR and DGRP are comprised of many isogenic strains that consist of genetically identical individuals). Instead, we calculated the mean stress resistance for each population and used a general linear model to test for a correlation between mean population-level copper and starvation resistance. This second analysis allowed us to address whether variation in starvation resistance among wild-derived populations followed a pattern like that of copper resistance.
We executed bulked segregant analysis (Michelmore et al., 1991; Pool, 2016; Kelly and Hughes, 2019) for the 4 wild-derived population cages (DBF, RFF, GPO, BMM), using a copper-resistant pool of 150 females (see above) and a matching control pool of 150 females randomly selected from the same generation of flies that were not exposed to copper. We made whole genome libraries for each of the eight pools of flies (copper-resistant and control pools of DBF, RFF, GPO, and BBM populations). DNA was extracted from batches of 15 females per pool using the Gentra Puregene kit (Qiagen) and then pooled at equal concentrations. Pool-specific barcoded libraries were generated using the NEBNext DNA Library Prep Reagent Set for Illumina (New England Biolabs) with size selection for 300-400bp inserts. Libraries were assessed with qPCR and were pooled at equimolar concentrations in the following combinations: library pool 1 = control and copper-resistant DBF pools; library pool 2 = control and copper-resistant RFF, GPO, and BBM pools). Paired-end 150bp reads from library pool 1 were sequenced over an NextSeq 550 High Output flowcell, resulting in 250x (DBF control pool) and 310x (DBF copper-resistant pool) coverage. Paired-end 150bp reads from library pool 2 were sequenced over an NextSeq2000 P2 flowcell, resulting in on average 131x coverage per pool (RFF control = 143x, RFF copper-resistant = 122x; GPO control = 124x, GPO copper-resistant = 124x; BBM control = 141x, BBM copper-resistant = 131x). Previous studies have demonstrated that use of sequencing data from multiple platforms does not introduce widespread systematic biases or issues related to variant calling (providing that similar platforms (Lam et al., 2012; Illumina, 2020) and the same quality filtering and variant calling procedures are used (Pan et al., 2022), see below). Library preparation and sequencing were completed at the University of Kansas Genome Sequencing Core.
Quality assessment and trimming of reads was completed with fastp [v. 0.20.1 (Chen et al., 2018)] using a mean quality score of 30 over a window size of 5bp. Filtered reads were aligned to the D. melanogaster reference genome (Release 6.41) using bwa mem (v. 0.7.17-r1188 (Li and Durbin, 2009)) with default parameters. Aligned reads were sorted, read pairs were identified, and duplicates were marked and removed with samtools [v. 1.11 (Li et al., 2009; Danecek et al., 2021)]. We used bcftools mpileup and call (v. 1.11 (Danecek et al., 2021)) to generate a BCF file containing genotype likelihoods for the eight aligned BAM files and to call variants. vcfutils.pl varFilter was used to filter SNP and indel variants using default parameters, generating a final VCF file containing variants from all pools and retaining 1,986,553 variants (203,673 of which were indels). VCF files were filtered to remove indels and variable sites with more than two segregating bases and to retain variable sites on the 5 major D. melanogaster chromosome arms (2R, 2L, 3R, 3L, X) with a minimum read depth of 100 and with a minimum allele frequency of 0.05 in at least one of the four populations. Post filtering, 692,822 SNPs remained. Small differences in error rate between the NextSeq 550 and NextSeq 2000 may impact detection of low frequency variants (Davis et al., 2021), but since we eliminate the bulk of these we do not anticipate a major impact of sequencing platform on our results.
To assess the shift in allele frequency at each variable site between the control and copper resistant pools of individuals, we first calculated the difference in arcsine square-root transformed allele frequency (z) between the two pools:
where Cu and Avg represent copper-resistant and control pools (Walsh and Lynch, 2018; Kelly and Hughes, 2019). Positive dz values indicate that the reference base is more common in the copper-resistant pool than in the control pool.
We detected copper resistance SNPs by first determining the likelihood of the data assuming that the SNP has no effect on resistance (Model 0). In this case,
where
LRT4 is fully general and does not distinguish between different patterns of allele frequency shift between pools. To refine our estimates for SNP effects, we fit two additional models (Models 1 and 2) that constrain responses across populations (see Figure 2; Table 2). The simplest case (Model 1) posits a strictly parallel response: The true
which is compared to the chi-square distribution with 1 df. Model 2 is a generalization of Model 1 and allows the parallel shift to differ in magnitude between two groups, populations with high copper exposure risk (BBM and GPO) and populations with low copper exposure risk (RFF and DBF). We tested this two-parameter model versus the null hypothesis using:
which is compared to the chi-square distribution with 2 df. To determine the best fitting model for each SNP initially identified as significant by LRT4, we compared the raw p values from the three LRT models (LRT1, LRT2, and LRT4; named using the number of parameters in each test) and assigned the model resulting in the lowest p-value. Five SNPs showed identical p values for more than one model, and in these cases we assigned the model yielding the highest log-likelihood value.
FIGURE 2. Conceptual representation of LRT models for significant SNPs. SNPs that shift in allele frequency between control and copper-resistant pools in the same way will give the lowest p-values using LRT1. LRT2 should most effectively identify parallel shifts in allele frequency between the two high copper resistance populations (BBM and GPO) versus the low copper resistance populations (DBF and RFF). Shifts in allele frequency that are population-specific will yield the lowest p-values using LRT4.
We used allele frequency estimates from the control pools of the DBF, RFF, GPO, and BBM populations to test for population differentiation using global FST values calculated with the R package poolfstat [v. 2.1.1 (Hivert et al., 2018)]. We also estimated FST for each SNP to identify regions of the genome that were enriched for SNPs that contribute to population differentiation. SNP-specific FST estimates were compared to the results of our bulked segregant analysis (BSA) to infer the history of natural selection on putative copper resistance loci.
The DGRP is a widely used Drosophila mapping population, and the full details on the generation, sequencing, and genome-wide association (GWA) mapping can be found from Mackay et al. (2012); Huang et al. (2014). The structure of the DGRP is distinct from the wild-collected populations examined in this study because it consists of fully inbred isogenic strains, allowing us to use GWA mapping to identify variants that are associated with copper resistance. The online DGRP2 GWA mapping tool (http://dgrp2gnets.ncsu.edu (Mackay et al., 2012)) accounts for Wolbachia infection and inversion status of the DGRP lines in the calculation of test statistics used to determine probabilities that the approximately 4 million variable sites are associated with phenotypic variation (Mackay et al., 2012; Huang et al., 2014). We assessed significance using a 5% false discovery rate threshold as well as the liberal threshold common in DGRP studies (p < 10−5) that is used because GWA using <200 lines is underpowered to detect variants with effects typical of highly polygenic traits (Vaisnav et al., 2014; Huang et al., 2020). The more permissive threshold (p < 10−5) facilitates comparison of our results with previously published studies and helps detect SNPs with true effects on copper resistance; however, the false positive rate is also inflated, so our results should be interpreted with this tradeoff in mind.
We estimated broad sense heritability for copper resistance in the DGRP by first estimating genetic and phenotypic variance from linear mixed model using the lme and varcomp functions in R (APE (Paradis et al., 2004), nlme (Pinheiro et al., 2017)) and then calculating the proportion of total variance explained by estimated genetic variance (Lynch and Walsh, 1998).
We assessed overlap in the genetic control of copper resistance in the DGRP with signals of copper-associated loci from our wild-derived populations (DBF, RFF, GPO, BBM) by comparing significant DGRP loci (p < 10−5) with those that shifted in allele frequency between the control and copper-resistant pools (significant for LRT1, LRT2, or LRT4). For SNPs that were within or near genes [within 3,000 bp, position information obtained from FlyBase (Thurmond et al., 2019)], this comparison was made at the gene level. For the remaining SNPs not associated with genes, and because linkage disequilibrium is low in D. melanogaster, we assessed overlap at the SNP level after converting DGRP SNP position annotation from Release 5 to Release 6 (Mackay et al., 2012; Thurmond et al., 2019). We also assessed overlap between copper-associated loci from the four wild-derived populations and previously resolved QTL intervals identified in the DSPR (Everman et al., 2021) by identifying QTL intervals within which BSA SNPs were found.
We carried out GO analysis using the Bioconductor annotation package org. Dm.eg.db (v. 3.14.0) (Carlson, 2019) and the R package GOstats (v. 2.60.0) (Falcon and Gentleman, 2007), which employs hypergeometric tests for overrepresentation of GO terms followed by multiple test correction. We obtained annotation and ontology information for the lists of genes using the D. melanogaster annotation tool available from biomaRt (v. 2.50.3 (Durinck et al., 2005)) via Ensembl (Cunningham et al., 2022) and the org.DM.eg.db R package.
Starvation resistance data for the DSPR and DGRP are available from Everman et al. (2019). Adult female 48-h copper resistance data for the DSPR are available from Everman et al. (2021). Phenotype data generated in this study are available from FigShare. All code and allele frequency data are available from accompanying Supplementary Materials deposited on FigShare (https://doi.org/10.6084/m9.figshare.22332775.v1). Sequence data are available from NCBI SRA BioProject PRJNA923720.
Adult female copper resistance (measured as average lifespan on copper containing food) was highly variable within and between all populations, with average lifespan ranging from 56.3 ± 0.95 s. e. to 114.2 ± 0.50 s. e. hours (Population: F6,1685 = 921.8, p < 0.0001; Figure 3A). Average copper resistance was also distinct in nearly every population; the only populations with similar levels of copper resistance were the DGRP and DBF populations (DBF vs. DGRP: adj p = 0.99) and the BBM and GPO populations (BBM vs. GPO: adj p = 0.94). All other pairwise comparisons of average copper resistance were significant (Tukey’s HSD; adj p < 0.0001; Figure 3A). Overall, average copper resistance was lowest in the DSPR laboratory population and highest in the wild-derived BBM and GPO populations (adj p < 0.0001; Figure 3A).
FIGURE 3. Copper and starvation resistance vary within and among populations. (A) Copper resistance significantly varied among populations (F6,1685 = 921.8, p < 0.0001), following a trend where resistance was higher in populations with closer proximity to sources of heavy metal contamination. Tukey’s HSD post hoc comparisons revealed that copper resistance was significantly different in all populations (adj p < 0.0001) except for the DGRP and DBF populations (adj p = 0.9) and the BBM and GPO populations (adj p = 0.9). (B) Starvation resistance also varied within and among populations. Tukey’s HSD post hoc comparisons revealed that starvation resistance was not different between the two laboratory populations (DSPR vs. DGRP, adj p = 0.94) or RFF, BBM, and GPO (all pairwise comparisons adj p > 0.64). Starvation resistance in the DBF population was significantly higher than the DSPR and DGRP and significantly lower than the other wild-derived populations (all pairwise comparisons adj p < 0.0001). In A and B, each point indicates either the strain-level mean (DSPR and DGRP, Table 1) or vial mean (N ≈ 20 females) resistance. (C) Population-level mean copper and starvation resistance were correlated with mean starvation resistance (F1,4 = 17.6, p = 0.014). (D) Starvation and copper resistance were correlated among DSPR strains (F1,191 = 31.3, p < 0.0001, adj R2 = 13.6%) as well among DGRP strains (E. F1,122 = 23.2, p < 0.0001, adj R2 = 15.3%). In (D, E), the black line shows the best fit line and shading indicates the 95% CI of the regression model.
Figure 3A shows variation in copper resistance that is consistent with selection for resistance to heavy metals, but this pattern could also be explained by variation in overall stress resistance among the populations. To address this, we measured starvation resistance in four of the wild-derived populations (DBF, RFF, BBM, GPO) and leveraged previously-published starvation data from the DSPR and DGRP laboratory populations (Everman et al., 2019). We treated starvation resistance as a representative non-metal stress trait that could contribute to copper resistance (i.e., through initial avoidance of copper-containing food). Starvation resistance was variable within and among populations (F5,2265 = 233.6, p < 0.0001; mean starvation resistance: 156.0 ± 0.71 s. e.–230.1 ± 1.9 s. e. hours; Figure 3B). However, population-level differences in starvation resistance were less distinct than for copper resistance. Variation in starvation resistance among the DSPR and DGRP strains nearly encompassed the range of starvation resistance observed for all other populations (Figure 3B). In contrast, variation in copper resistance in wild-derived populations extended beyond the range of copper resistance observed in either laboratory population (Figure 3A). Generally, average starvation resistance was significantly different between the laboratory and wild-derived populations, with the exception of DBF which was intermediate to the laboratory populations and BBM, RFF, and GPO. Among all populations, mean copper and starvation resistance were correlated (F1,4 = 17.6, p = 0.013, adj R2 = 76.8%, Figure 3C). However, the correlation between strain-specific copper and starvation resistance in the laboratory populations was modest, suggesting that starvation resistance explains less than 20% of variation in copper resistance when genetic variation can be controlled for (DSPR: F1,191 = 31.3, p < 0.0001, adj R2 = 13.6%, Figure 3D; DGRP: F1,122 = 23.2, p < 0.0001, adj R2 = 15.2%; Figure 3E). These pattens suggest that variation in copper resistance among populations is not solely due to overall patterns of stress resistance in the wild-derived populations.
We applied the bulked segregant analysis (BSA) tests to 692,822 SNPs. The most general model LRT4 identified 288 SNPs associated with copper resistance (Figure 4, top panel). Of these, LRT1, which tested for parallel shift in allele frequency in multiple populations, was chosen as the best fitting model for 70 SNPs. LRT2, which tested for a correspondence between exposure risk of populations and allele frequency shift, was the best fitting model for 23 SNPs. The remaining 195 SNPs were best fit by LRT4 indicating population-specific shifts in allele frequency between the copper-resistant and control pools (Figure 4; Table 2).
FIGURE 4. Copper resistance is influenced by multiple loci in wild-derived populations. The top panel shows 288 SNPs which shifted in allele frequency in a manner consistent with LRT1 (parallel shift, 70 SNPs), LRT2 (similar shift in high vs. low copper resistance populations, 23 SNPs), or LRT4 (population-specific shift, 195 SNPs). Points are partially transparent to show overlap of multiple SNPs. The bottom panel shows estimated FST values for each SNP. In both panels, SNPs are shaded according to which LRT model best fit their shift in allele frequency between the average and high copper resistance pools. 39 SNPs with population-specific effects on copper resistance (LRT4) also had elevated FST values, suggesting that these SNPs also contribute to population differentiation. In both plots, red horizontal dashed lines indicate thresholds; in the top panel the threshold indicates the 10% FDR cutoff used to identify significant SNPs. In the bottom panel the threshold is set at FST = 0.15 to differentiate SNPs with elevated FST estimates (Frankham et al., 2002).
Of the 288 SNPs significantly associated with copper resistance, 198 (68.8%) fell within 103 distinct genes or within 3000bp of 120 distinct genes (Table S1). Because a small number of SNPs fell within multiple overlapping genes, the total number of genes containing or near LRT-associated SNPs was 220. The remaining 90 SNPs were further than 3000bp from any gene. Forty-seven genes have more than one SNP within or near them, with a maximum of 31 SNPs falling within a single gene (Pzl; Supplementary Figure S2, Supplementary Table S1). Gene ontology analysis revealed enrichment of genes involved in a wide range of categories which included several related to mitochondrial function (Supplementary Figure S2). We did not observe enrichment of genes specifically related to metal homeostasis, detoxification, or toxicity.
We examined the distribution of maximum LRT values for each model as they varied across the genome in 100 kb intervals and found that higher maximum LRT values were more common near the centromere regions of chromosome 2R and 3R (Supplementary Figure S3). SNPs with significant LRT values tended to co-localize in peaks in these regions as well (Supplementary Figure S3). This finding is consistent with a previous study of parallel selection in Drosophila simulans (Kelly and Hughes, 2019), and supports patterns expected for regions of low recombination rates.
We calculated pairwise FST using allele frequencies estimated from the control pools of each natural population for the 692,822 SNPs to measure differentiation among the wild populations. Overall, global FST was low (FST = 0.005 ± 9.06 × 10−5), consistent with previous studies of genetic differentiation in D. melanogaster populations (Ives, 1945; Verspoor and Haddrill, 2011; Mateo et al., 2018). While low, pairwise FST estimates were significantly positive indicating that the four wild-derived populations are genetically distinct (Table 3).
Using a threshold of FST > 0.15 to indicate moderate to significant differentiation (Hartl and Clark, 1997; Frankham et al., 2002), SNP-wise FST estimates revealed 142 SNPs distributed across the five major chromosome arms that contribute to population differentiation (Figure 4, lower panel). Of these, 93 SNPs fell within genes (85 SNPs) or were within 3000bp of genes (8 SNPs) (Supplementary Table S2). Gene ontology analysis revealed enrichment of genes belonging to a diverse set of categories including “response to chemical” (GO term ID: 0042221) and others related to mitochondrial function and protein folding (Supplementary Figure S2). As with the BSA results, we did not observe any enrichment of SNPs with elevated FST in genes directly related to copper response. Like our BSA results, SNPs with high FST estimates tended to co-localize in peaks near centromeres and telomeres (Figure 4), and 39 of these SNPs were among those associated with copper resistance.
Notably, SNPs that were significantly associated with copper resistance in our BSA results (above) exhibited a tenfold increase in FST estimates relative to non-significant SNPs (LRT-significant SNPs average FST = 0.061 ± 0.006 s. e.; Non-significant SNPs FST = 0.005 ± 0.00002 s. e.). High FST of significant SNPs was largely driven by the farm-mine contrasts (LRT-significant SNPs average FST = 0.055) as the farm-farm contrasts resulted in lower levels of differentiation at significant sites (LRT-significant SNPs average FST = 0.033). In addition, BSA-SNPs with elevated Fst estimates usually had population-specific responses to copper resistance (i.e., they were most significant for LRT4; Figure 4, Table S1). The FST estimates corroborate the BSA results because they suggest a history of selection acting on loci that are associated with copper resistance.
Heritability of copper resistance was high in the DGRP (H2 = 84.9%). Using genome-wide association mapping, we identified two SNPs associated with copper resistance in the DGRP at a 5% FDR that were in introns of sls and CG31038 (Figure 5). Neither gene has previously been associated with copper resistance. Using the more relaxed significance threshold that is typically applied to the DGRP (p < 10−5) (Mackay et al., 2012; Huang et al., 2014; Morozova et al., 2014; Zhou et al., 2016), we found 35 SNPs associated with copper resistance. The SNPs were near or within 17 annotated genes, with some genes containing multiple SNPs (Supplementary Table S3). None of the 17 genes have been previously associated with copper, heavy metal resistance, or responses to stress, and there was no overlap at the SNP or gene level between the DGRP and wild-derived population results.
FIGURE 5. Genome wide association mapping of copper resistance in the DGRP identified multiple significantly associated SNPs. The red dashed line indicates p < 10−5 and variants surpassing this value are highlighted in red. Sites that were significantly associated with copper resistance at a 5% FDR are highlighted in blue.
We examined overlap between our BSA results and the SNPs associated with copper resistance in the DGRP at the gene level for all SNPs that fell within or near (within 3000bp of) genes and at the SNP level for all SNPs that could not be linked to genes. We found that GWA in the DGRP highlighted a set of genes and SNPs that were entirely distinct from the genes and SNPs associated with copper resistance in the wild-derived populations. The closest DGRP and BWA SNPs were >30,000 bp apart.
Overlap between our BSA results and regions associated with copper resistance in the DSPR was more extensive. Previous assessments of copper resistance carried out in the DSPR multi-parental mapping population indicated that allelic variation in several regions of the genome was linked to variation in copper resistance (Everman et al., 2021). This previous work highlighted several candidate genes involved in copper binding and metabolism and suggested that natural variation in genes involved in copper homeostasis may be under selection in natural populations. We identified one SNP upstream of the gene CG11825 that was highlighted by QTL mapping in the DSPR (Q5 in that study), but no other candidate genes identified in that work contained or were near SNPs associated with copper resistance in our wild-derived populations. Nine of the 12 QTL regions identified in our previous work (Everman et al., 2021) spanned regions that contained a total of 31 SNPs with significant LRT values. All but one of the 31 SNPs that fell within QTL was in or near an annotated gene, and several genes were identified as potential candidate genes in the present study including blw, ATPsynβL, CG7430, and LRR in addition to CG11825 (discussed below).
SNPs associated with copper resistance in the DGRP overlapped with two of the previously identified DSPR QTL. One DGRP SNP located on chromosome arm 2R fell within the Q5 DSPR QTL interval and six DGRP SNPs on chromosome arm 3L fell within the Q9 DSPR QTL interval. Combined, the DSPR Q5 and Q9 intervals contained several candidate copper resistance genes, which were followed up with RNAi in our previous work (Everman et al., 2021). However, none of the DGRP SNPs were near any of these functionally tested candidate genes.
Our assessment of overlap between the genetic architecture of copper resistance previously reported in the DSPR and the populations examined in this study may be influenced by differences in how the copper resistance traits were measured. In the present study, we measured copper resistance as adult female lifespan and in our previous work, DSPR copper resistance was assessed as survival after 48 h of exposure. However, for a subset of 194 DSPR strains, these two measures are highly correlated (F1,192 = 315.1, p << 0.0001, adj R2 = 62%, r = 80%; Supplementary Figure S4). This correlation suggests that it is unlikely that the overlap in genetic architectures of copper resistance between the DSPR and the other populations assessed in this study is solely due to subtle differences in the phenotype assayed.
We demonstrate a strong association between copper resistance within a population and its proximity to sources of pollution. Flies collected from smaller fruit farms (DBF and RFF) where copper-containing pesticide use is limited had lower levels of copper resistance, whereas flies collected from mining sites (AMM and BBM) had higher levels of copper resistance (Figure 3A). We note that GPO–an open-air fruit market specializing in peaches–yielded flies with copper resistance similar to that of flies from the BBM site (Figure 3A). Dispersal estimates for D. melanogaster suggest that flies can disperse up to 12 km and possibly further if assisted by wind (Leitch et al., 2021). Despite the proximity of these collection sites in the Copper Basin region of Tennessee (∼43 km apart; Figure 1A), pairwise population differentiation estimates suggest that the BBM and GPO populations are distinct (Table 3). Further, because produce sold at the GPO site is grown in Hogback, South Carolina, USA, it is likely that the high levels of copper resistance in the BBM and GPO wild-derived populations are influenced by distinct sources of selection. Copper-containing fungicides are often used to control infections caused by Taphrina deformans in peach trees (Pecknold, 2015; Reis et al., 2021), as well as fungi and bacteria that damage wine grapes, olives, and citrus (Schutte et al., 1997; Roca et al., 2007; Peña et al., 2018), increasing the copper exposure risk for flies collected from the large commercial orchards such as the GPO site. Use of copper-containing pesticides may contribute to elevated levels of copper resistance in the GPO flies, whereas elevated copper resistance in the BBM flies is more likely to be due to historical mining activities.
The high level of copper resistance in flies descended from the BBM population is striking because no mining activity has occurred at Burra Burra Mine for more than 60 years. Heavy metal pollution persists in contaminated soil and water sources for many years (Suman et al., 2018; Ali et al., 2019) and tends to accumulate through food chains, becoming increasingly concentrated at higher trophic levels (Heikens et al., 2001; Sánchez-Chardi and Nadal, 2007; Gall et al., 2015; Ali et al., 2019; Ali and Khan, 2019). We did not measure levels of copper or other heavy metals in soil or plant material at the collection site, but our results suggest that sources of selection leading to high copper resistance continue to persist at Burra Burra Mine.
Mining activity is also the most likely environmental contributor to high copper resistance in the flies collected from Anchutz Mine (AMM) (Figure 3). Although copper is not the primary product of the mine, exposure to toxic levels of other heavy metals may drive the evolution of increased copper resistance as a correlated response to selection. For example, experimental evolution of the least killifish Heterandria formosa in response to cadmium stress also increased resistance to copper toxicity (Xie and Klerks, 2003). Studies using laboratory populations have demonstrated overlap in the genetic control of resistance to cadmium and copper in Caenorhabditis elegans (Evans et al., 2018) and cadmium and lead in D. melanogaster (Zhou et al., 2017). More generally, many genes involved in the metabolism of copper and response to copper toxicity are known to interact with other heavy metals (Egli et al., 2006; Yepiskoposyan et al., 2006; Adams et al., 2015; Calap-Quintana et al., 2017). Our intriguing results suggest that a broader sampling of populations associated with mines is warranted and would deepen our understanding of the historical selection pressures exerted on D. melanogaster populations and of the potential evolutionary consequences metal pollution presents to other species.
The genetic control of shifts in resistance to heavy metals has been examined in several species, and instances of both single locus (e.g., Wright et al., 2013; 2015) and polygenic control of resistance have been characterized (e.g., Ehrenreich et al., 2012; Evans et al., 2018). We identified 288 SNPs distributed across the genome that contribute to copper resistance in the wild-derived populations (Figure 4, Table S1) and 35 SNPs associated with copper resistance in the DGRP laboratory population (Figure 5). Polygenic control of copper resistance is consistent with previous findings from the DSPR indicating that copper resistance has a complex genetic basis (Everman et al., 2021) and from work carried out in other laboratory populations that may be naïve to metal stress (Ehrenreich et al., 2012; Zhou et al., 2016; Zhou et al., 2017; Evans et al., 2018).
Gene ontology analysis of the 238 annotated genes with copper-associated SNPs detected in the wild-derived populations and the DGRP revealed enrichment of genes linked to many categories including those related to protein folding, mitochondrial function, and ATP synthesis (Supplementary Figure S2). The presence of 9 SNPs within three heat shock proteins belonging to the highly conserved Hsp70 family of chaperone proteins contributed to enrichment of these GO categories (Gupta et al., 2010): Hsc70-3, Hsc70-4, and Hsc70-5 (The UniProt Consortium et al., 2021) (Figure 6, Supplementary Table S1). Heat shock proteins respond to protein damage caused by oxidative stress following exposure to a diverse set of environmental stressors (Feder and Hofmann, 1999; reviewed in Gupta et al., 2010), and the related gene Hsp70 has been linked to response to heavy metal stress in bacteria, plants, nematodes, and fish (Williams et al., 1996; Arts et al., 2004; Roh et al., 2006; reviewed in Gupta et al., 2010; Cui et al., 2013; Cui et al., 2019; Zuily et al., 2022) prompting its assessment for use as a bioindicator of pollution, environmental contamination, and climate change (Pyza et al., 1997; Mukhopadhyay et al., 2003; Arts et al., 2004; Roh et al., 2006; Yusof et al., 2022). We recently reported an increase in expression of Hsc70-3 and Hsc70-5 in adult D. melanogaster females from the DSPR in response to copper stress (Everman et al., 2021). The SNPs that fell within the three Hsc70 chaperone genes had population-specific associations with copper resistance (LRT4, Figure 6). Overall, these patterns suggest that naturally occurring genetic variation in Hsc70 chaperone genes may be under selection for copper resistance to varying degrees across the four populations assessed.
FIGURE 6. Shift in allele frequency between copper-resistant and control pools of flies from the four wild-derived populations at SNPs that fell within or near genes related to protein folding and mitochondrial function. Positive dz values indicate that the reference base is more common in the copper-resistant pool relative to the control pool. Open symbols indicate populations with high copper exposure risk; closed symbols indicate populations with low exposure risk. Note that some symbols perfectly overlap.
As an essential micronutrient, copper ions act as cofactors for cytochrome c oxidase and super oxide dismutase, and they play a critical role in mitochondrial structure and function (Medeiros and Jennings, 2002; Belyaeva et al., 2012; Zischka and Einer, 2018; Ruiz et al., 2021). However, mitochondria are highly sensitive to excess copper accumulation in cells, and mitochondrial dysregulation of copper is associated with disease in human populations (Huster and Lutsenko, 2007; Zischka and Einer, 2018). Enrichment of GO categories related to mitochondrial function and ATP synthesis were the result of 36 SNPs in or near 16 genes: blw, Prx2540-1, CG11825, SdhAL, ATPsynbetaL, CG7430, CG7834, CG31644, ND-75, SdhA, Hsc70-4, Hsc70-5, Pink1, Gdap1, Sccpdh1, LRR, and CG7834 (The UniProt Consortium et al., 2021) (Figure 6). For all but three SNPs, allele frequency shift was population specific (LRT4). The remaining three SNPs shifted consistently in frequency in the copper-resistant pool across all populations (LRT1) (Figure 6).
Of the 16 genes linked to ATP synthesis, CG11825 is the only gene that has been previously directly associated with resistance to copper stress (Norgate et al., 2007) (Figure 6). Exposure of the D. melanogaster S2 cell line to high levels of copper resulted in decreased expression of CG11825, and RNAi knockdown increased copper resistance (Norgate et al., 2007). In a previous study, we found a QTL associated with copper resistance in the DSPR that included this gene, suggesting that allelic variation in CG11825 could influence copper resistance (Everman et al., 2021). Allele frequency shifts (dz values) for the variant 65bp upstream of CG11825 were positive in all four populations, indicating that the alleles present in each population near CG11825 contribute to copper resistance in a similar manner in the wild-derived populations we examined. Our data do not provide insight into the effect of the upstream SNP on CG11825 gene function, but regulatory SNPs have the potential to alter phenotype expression; for example, the upstream SNP may influence expression level of CG11825 in response to copper stress. In a recent study of copper resistance in European populations of D. melanogaster, regulatory variation was identified as an important contributor to variation in copper resistance that may be influenced by selection pressure resulting from pollution (Green et al., 2022).
In addition to CG11825, the gene blw also stands out among the potential candidates associated with mitochondrial function (Figure 6). Ten variants fell within the second and third exons of blw and had population-specific effects on copper resistance. blw is involved in ATP synthesis, encoding the alpha subunit of mitochondrial ATP synthase (Jacobs et al., 1998). Previously, blw mutant flies generated with a transposable element insertion were shown to have higher levels of reactive oxygen species (ROS) resulting in higher levels of oxidative stress (Di Cara et al., 2013). Allelic variation in the promoter region of blw has also been associated with increased lifespan in D. melanogaster (Carnes et al., 2015), and Garcia et al. (2017) suggested that increased expression of blw could increase metabolic activity and the production of ROS. blw has not been previously associated with copper metabolism or toxicity. However, copper ions may interact with ATP synthase (Medeiros and Jennings, 2002) and excess copper can lead to decreased energy production and disrupt mitochondrial protein structure and function (Borchard et al., 2018).
Of the remaining genes associated with mitochondrial function, CG7430, TrpRS-m, and SdhA were previously shown to shift in gene expression in response to copper stress in D. melanogaster females (Everman et al., 2021), and Prx2540-1 belongs to a family of peroxiredoxin genes that play a protective role in the response to oxidative stress (Wood et al., 2003). Our results suggest that genetic variation that influences mitochondrial function could be an important contributor to copper resistance even in populations with relatively low copper resistance.
In addition to genes related to mitochondrial function, we detected one SNP within the gene LRR, which has been previously linked to response to a neonicotinoid-containing insecticide and is involved in immune response in D. melanogaster and bees (Aphis mellifera) (Di Prisco et al., 2013). LRR is contains leucine-rich repeats, which is a broadly conserved protein domain that facilitates a wide range of responses to immune threats in plants and animals (Ng and Xavier, 2011). LRR has not been previously linked to copper resistance or heavy metal response in flies, but genes containing leucine-rich repeats have been linked to arsenic and lead resistance and copper toxicity response in Arabidopsis thaliana (Weber et al., 2006; Zhu et al., 2013; Fu et al., 2014). The SNP that fell within LRR had population-specific effects on copper resistance.
We did not find an excess of copper-associated genes (based on FlyBase annotation, see Methods) implicated by SNPs that shifted in allele frequency between copper-resistant and control pools. The lack of significant SNPs was not due to a lack of genetic variability in or near copper-associated genes. SNP variants were present in many genes known to be involved in copper homeostasis or metabolism including the five metallothioneins, which are involved in the detoxification of copper, and the copper-responsive transcription factor MTF-1 (Zhang et al., 2001; Balamurugan et al., 2004) in all four wild-derived populations (Supplementary Table S4). This genetic variation may influence copper resistance in our wild-derived populations with effects that are too small to detect with our experimental design. It is possible that with a larger number of natural replicates of high and low exposure risk populations, SNPs that contribute more moderate improvements to copper resistance could be detected.
Overall, many potential candidate genes were highlighted by our BSA analysis. Several of these potential candidates are especially promising given previous studies in D. melanogaster and other species that have associated the genes we identified with responses related to metal or oxidative stress resistance. In particular Everman et al. (2021) functionally tested the gene CG11825 and demonstrated that RNAi knockdown of this gene reduced copper resistance. Similar additional follow up of our novel candidates would be necessary to verify and fully characterize the role they may play in copper resistance.
Most significant SNPs identified with BSA had population-specific effects on copper resistance (consistent with the LRT4 model), suggesting that the effect of resistance-associated alleles is likely modified by a combination of genetic background and selection regime unique to each of the populations we sampled. The LRT4 model highlights SNPs with alleles that, for example, contribute to increased copper resistance in one population that may not be associated with resistance in another population, or indeed may have an opposite effect on resistance (Figure 2). Many of the candidates identified in the present study are components of larger gene families or pathways (e.g., heat shock proteins (Gupta et al., 2010)) and they interact with other components of these pathways. Genetic variation in interacting genes has the potential to modify the effects of trait-associated alleles through multiple forms of epistasis, a phenomenon that is estimated to be common and itself polygenic (Chandler et al., 2014; Chandler et al., 2017; Goldstein and Ehrenreich, 2021). In yeast, for example, allelic variation in the heat shock chaperone protein Hsp90 was estimated to modify the phenotypic expression of up to 20% of variable loci in the genome (Jarosz and Lindquist, 2010; Goldstein and Ehrenreich, 2021). Gene by environmental interactions have the potential to further modify these epistatic interactions (Price et al., 2003; Goldstein and Ehrenreich, 2021), and it is likely that epistasis influences copper resistance in our study. However, to assess epistatic interactions, we would need individual-level phenotypes and genotypes, which are not offered in a BSA design. It is also possible that our results are impacted by power; including additional populations with variable copper exposure risk may demonstrate that the predominance of population-specific patterns are characteristic of our focal populations. In the present study, the association between phenotypic variation in copper resistance and proximity to copper pollution combined with population differentiation strongly suggests that the effects of selection for copper resistance are often population-specific and subject to epistatic and environmental interactions.
In addition to the SNPs with significant population-specific effects on copper resistance, we identified 93 significant SNPs with consistent effects on copper resistance in multiple populations, whether the effects were consistent in all populations (LRT1–70 SNPs) or were consistent in the high copper resistance vs. low copper resistance populations (LRT2–23 SNPs) (Table 2). In contrast to SNPs with effects that may be differently modified by selection or background effects, SNPs with consistent effects across populations or classes of populations have the potential to provide insight into evolutionarily strategies for adapting to copper stress. Parallel adaption to copper stress has been previously observed through experimental evolution of yeast (Gerstein et al., 2015). S. cerevisiae exposed to elevated copper levels repeatedly evolved mutations in genes related to mitochondrial function as well as by increasing the number of copies of CUP1-1, a copper chelator (Gerstein et al., 2015; Hull et al., 2017). Genetic signatures of parallel adaptation in a costal plant species Silene uniflora in response to heavy metal pollution from mining activities also point to instances of shared adaptive responses across multiple natural populations (Papadopulos et al., 2021). In each of these cases and in our own study, unique adaptations were predominant; however, the potential for parallel adaptation to heavy metal stress in natural populations based on SNPs with parallel effects warrants further investigation.
The original contributions presented in the study are publicly available. All sequence data can be found here: https://www.ncbi.nlm.nih.gov/, PRJNA923720 and remaining data is available on FigShare: https://doi.org/10.6084/m9.figshare.22332775.v1.
EE, SM, and JK designed the experiments. EE performed the experiments. EE and JK analysed the data and JK wrote the BSA code. EE wrote the paper. EE, SM, and JK edited and revised the paper.
EE and the research was supported by Postdoctoral Fellowships from the NIH (National Institutes of Health) (F32 GM133111 and K99 ES033257) and the Kansas INBRE (Kansas Idea Network of Biomedical Research Excellence) project (P20 GM103418). Additional support for experiments was provided by NIH R01 ES029922 to SJM. Sequencing was performed by the KU Genome Sequencing Core which is supported by the CMADP (Center for Molecular Analysis of Disease Pathways), and NIH COBRE (Centers of Biomedical Research Excellence) award (P20 GM103638). Open access publishing of this article was supported by the David Henry Wenrich Memorial endowment fund at the University of Kansas.
We thank Dave Mosby (USDA, Anshutz Mine), Ken Rush (Burra Burra Mine), Rex Rees (Ree’s Fruit Farm), Dan Hoerz (Duncan’s Berry Farm), and the Gilliland Family (Gilliland Peach Orchard) for permission and assistance with the collection of flies used in this study. We also thank Paul Klawinski for assistance with field work.
The authors declare that the research was conducted in the absence of any commercial or financial relationships that could be construed as a potential conflict of interest.
All claims expressed in this article are solely those of the authors and do not necessarily represent those of their affiliated organizations, or those of the publisher, the editors and the reviewers. Any product that may be evaluated in this article, or claim that may be made by its manufacturer, is not guaranteed or endorsed by the publisher.
The Supplementary Material for this article can be found online at: https://www.frontiersin.org/articles/10.3389/fgene.2023.1144221/full#supplementary-material
Abnoos, H., Fereidoni, M., Mahdavi-shahri, N., Haddad, F., and Jalal, R. (2013). Developmental study of mercury effects on the fruit fly (Drosophila melanogaster). Interdiscip. Toxicol. 6, 34–40. doi:10.2478/intox-2013-0007
Adams, S. V., Barrick, B., Christopher, E. P., Shafer, M. M., Makar, K. W., Song, X., et al. (2015). Genetic variation in metallothionein and metal-regulatory transcription factor 1 in relation to urinary cadmium, copper, and zinc. Toxicol. Appl. Pharmacol. 289, 381–388. doi:10.1016/j.taap.2015.10.024
Ali, H., and Khan, E. (2019). Trophic transfer, bioaccumulation, and biomagnification of non-essential hazardous heavy metals and metalloids in food chains/webs—concepts and implications for wildlife and human health. Hum. Ecol. Risk Assess. Int. J. 25, 1353–1376. doi:10.1080/10807039.2018.1469398
Ali, H., Khan, E., and Ilahi, I. (2019). Environmental chemistry and ecotoxicology of hazardous heavy metals: Environmental persistence, toxicity, and bioaccumulation. J. Chem. 2019, 1–14. doi:10.1155/2019/6730305
Arts, M.-J. S. J., Schill, R. O., Knigge, T., Eckwert, H., Kammenga, J. E., and Kohler, H. R. (2004). Stress proteins (hsp70, hsp60) induced in isopods and nematodes by field exposure to metals in a gradient near Avonmouth, UK. Ecotoxicology 13, 739–755. doi:10.1007/s10646-003-4473-5
Ashida, J. (1965). Adaptation of fungi to metal toxicants. Annu. Rev. Phytopathol. 3, 153–174. doi:10.1146/annurev.py.03.090165.001101
Balamurugan, K., Egli, D., Selvaraj, A., Zhang, B., Georgiev, O., and Schaffner, W. (2004). Metal-responsive transcription factor (MTF-1) and heavy metal stress response in Drosophila and mammalian cells: A functional comparison. Biol. Chem. 385, 597–603. doi:10.1515/BC.2004.074
Belyaeva, E. A., Sokolova, T. V., Emelyanova, L. V., and Zakharova, I. O. (2012). Mitochondrial electron transport chain in heavy metal-induced neurotoxicity: Effects of cadmium, mercury, and copper. Sci. World J. 2012, 136063–136114. doi:10.1100/2012/136063
Borchard, S., Bork, F., Rieder, T., Eberhagen, C., Popper, B., Lichtmannegger, J., et al. (2018). The exceptional sensitivity of brain mitochondria to copper. Toxicol. Vitro 51, 11–22. doi:10.1016/j.tiv.2018.04.012
Calap-Quintana, P., González-Fernández, J., Sebastiá-Ortega, N., Llorens, J., and Moltó, M. (2017). Drosophila melanogaster models of metal-related human diseases and metal toxicity. Int. J. Mol. Sci. 18, 1456. doi:10.3390/ijms18071456
Cao, X., Bi, R., and Song, Y. (2017). Toxic responses of cytochrome P450 sub-enzyme activities to heavy metals exposure in soil and correlation with their bioaccumulation in Eisenia fetida. Ecotoxicol. Environ. Saf. 144, 158–165. doi:10.1016/j.ecoenv.2017.06.023
Carlson, M. (2019). Genome wide annotation for fly. R Package Version 382. org.Dm.eg.db. doi:10.18129/B9.bioc.org.Dm.eg.db
Carnes, M. U., Campbell, T., Huang, W., Butler, D. G., Carbone, M. A., Duncan, L. H., et al. (2015). The genomic basis of postponed senescence in Drosophila melanogaster. PLOS ONE 10, e0138569. doi:10.1371/journal.pone.0138569
Catalán, A., Glaser-Schmitt, A., Argyridou, E., Duchen, P., and Parsch, J. (2016). An indel polymorphism in the MtnA 3’ untranslated region is associated with gene expression variation and local adaptation in Drosophila melanogaster. PLOS Genet. 12, e1005987. doi:10.1371/journal.pgen.1005987
Chain, F. J. J., Finlayson, S., Crease, T., and Cristescu, M. (2019). Variation in transcriptional responses to copper exposure across Daphnia pulex lineages. Aquat. Toxicol. 210, 85–97. doi:10.1016/j.aquatox.2019.02.016
Chandler, C. H., Chari, S., Tack, D., and Dworkin, I. (2014). Causes and consequences of genetic background effects illuminated by integrative genomic analysis. Genetics 196, 1321–1336. doi:10.1534/genetics.113.159426
Chandler, C. H., Chari, S., Kowalski, A., Choi, L., Tack, D., DeNieu, M., et al. (2017). How well do you know your mutation? Complex effects of genetic background on expressivity, complementation, and ordering of allelic effects. PLOS Genet. 13, e1007075. doi:10.1371/journal.pgen.1007075
Chen, S., Zhou, Y., Chen, Y., and Gu, J. (2018). fastp: an ultra-fast all-in-one FASTQ preprocessor. Bioinformatics 34, i884–i890. doi:10.1093/bioinformatics/bty560
Cui, Y., Xu, G., Wang, M., Yu, Y., Li, M., da Rocha, P. S. C. F., et al. (2013). Expression of OsMSR3 in Arabidopsis enhances tolerance to cadmium stress. Plant cell tissue organ cult. PCTOC 113, 331–340. doi:10.1007/s11240-012-0275-x
Cui, Y., Wang, M., Yin, X., Xu, G., Song, S., Li, M., et al. (2019). OsMSR3, a small heat shock protein, confers enhanced tolerance to copper stress in Arabidopsis thaliana. Int. J. Mol. Sci. 20, 6096. doi:10.3390/ijms20236096
Cunningham, F., Allen, J. E., Allen, J., Alvarez-Jarreta, J., Amode, M. R., Armean, I. M., et al. (2022). Ensembl 2022. Nucleic Acids Res. 50, D988–D995. doi:10.1093/nar/gkab1049
Danecek, P., Bonfield, J. K., Liddle, J., Marshall, J., Ohan, V., Pollard, M. O., et al. (2021). Twelve years of SAMtools and BCFtools. GigaScience 10, giab008. doi:10.1093/gigascience/giab008
Davis, E. M., Sun, Y., Liu, Y., Kolekar, P., Shao, Y., Szlachta, K., et al. (2021). SequencErr: Measuring and suppressing sequencer errors in next-generation sequencing data. Genome Biol. 22, 37. doi:10.1186/s13059-020-02254-2
Di Cara, F., Duca, E., Dunbar, D. R., Cagney, G., and Heck, M. M. S. (2013). Invadolysin, a conserved lipid droplet-associated metalloprotease, is required for mitochondrial function in Drosophila. J. Cell Sci. Jcs. 133306, 4769–4781. doi:10.1242/jcs.133306
Di Prisco, G., Cavaliere, V., Annoscia, D., Varricchio, P., Caprio, E., Nazzi, F., et al. (2013). Neonicotinoid clothianidin adversely affects insect immunity and promotes replication of a viral pathogen in honey bees. Proc. Natl. Acad. Sci. 110, 18466–18471. doi:10.1073/pnas.1314923110
Durinck, S., Moreau, Y., Kasprzyk, A., Davis, S., De Moor, B., Brazma, A., et al. (2005). BioMart and bioconductor: A powerful link between biological databases and microarray data analysis. Bioinformatics 21, 3439–3440. doi:10.1093/bioinformatics/bti525
Egli, D., Selvaraj, A., Yepiskoposyan, H., Zhang, B., Hafen, E., Georgiev, O., et al. (2003). Knockout of ‘metal-responsive transcription factor’ MTF-1 in Drosophila by homologous recombination reveals its central role in heavy metal homeostasis. EMBO J. 22, 100–108. doi:10.1093/emboj/cdg012
Egli, D., Domènech, J., Selvaraj, A., Balamurugan, K., Hua, H., Capdevila, M., et al. (2006). The four members of the Drosophila metallothionein family exhibit distinct yet overlapping roles in heavy metal homeostasis and detoxification. Genes Cells. 11, 647–658. doi:10.1111/j.1365-2443.2006.00971.x
Ehrenreich, I. M., Bloom, J., Torabi, N., Wang, X., Jia, Y., Kruglyak, L., et al. (2012). Genetic architecture of highly complex chemical resistance traits across four yeast strains. PLoS Genet. 8, e1002570. doi:10.1371/journal.pgen.1002570
Enayati, A. A., Ranson, H., and Hemingway, J. (2005). Insect glutathione transferases and insecticide resistance. Insect Mol. Biol. 14, 3–8. doi:10.1111/j.1365-2583.2004.00529.x
Evans, K. S., Brady, S. C., Bloom, J. S., Tanny, R. E., Cook, D. E., Giuliani, S. E., et al. (2018). Shared genomic regions underlie natural variation in diverse toxin responses. Genetics 210, 1509–1525. doi:10.1534/genetics.118.301311
Everman, E. R., McNeil, C. L., Hackett, J. L., Bain, C. L., and Macdonald, S. J. (2019). Dissection of complex, fitness-related traits in multiple Drosophila mapping populations offers insight into the genetic control of stress resistance. Genetics 211, 1449–1467. doi:10.1534/genetics.119.301930
Everman, E. R., Cloud-Richardson, K. M., and Macdonald, S. J. (2021). Characterizing the genetic basis of copper toxicity in Drosophila reveals a complex pattern of allelic, regulatory, and behavioral variation. Genetics 217, 1–20. doi:10.1093/genetics/iyaa020
Falcon, S., and Gentleman, R. (2007). Using GOstats to test gene lists for GO term association. Bioinformatics 23, 257–258. doi:10.1093/bioinformatics/btl567
Feder, M. E., and Hofmann, G. E. (1999). Heat-shock proteins, molecular chaperones, and the stress response: Evolutionary and ecological physiology. Annu. Rev. Physiol. 61, 243–282. doi:10.1146/annurev.physiol.61.1.243
Fogel, S., and Welch, J. W. (1982). Tandem gene amplification mediates copper resistance in yeast. Proc. Natl. Acad. Sci. 79, 5342–5346. doi:10.1073/pnas.79.17.5342
Frankham, R., Ballou, J. D., and McInnes, K. H. (2002). Introduction to conservation genetics. Cambridge: Cambridge University Press.
Fu, S.-F., Chen, P.-Y., Nguyen, Q. T. T., Huang, L.-Y., Zeng, G.-R., Huang, T. L., et al. (2014). Transcriptome profiling of genes and pathways associated with arsenic toxicity and tolerance in Arabidopsis. BMC Plant Biol. 14, 94. doi:10.1186/1471-2229-14-94
Gall, J. E., Boyd, R. S., and Rajakaruna, N. (2015). Transfer of heavy metals through terrestrial food webs: A review. Environ. Monit. Assess. 187, 201–221. doi:10.1007/s10661-015-4436-3
Garcia, J. F., Carbone, M. A., Mackay, T. F. C., and Anholt, R. R. H. (2017). Regulation of Drosophila lifespan by bellwether promoter alleles. Sci. Rep. 7, 4109. doi:10.1038/s41598-017-04530-x
Gerstein, A. C., Ono, J., Lo, D. S., Campbell, M. L., Kuzmin, A., and Otto, S. P. (2015). Too much of a good thing: The unique and repeated paths toward copper adaptation. Genetics 199, 555–571. doi:10.1534/genetics.114.171124
Goldstein, I., and Ehrenreich, I. M. (2021). The complex role of genetic background in shaping the effects of spontaneous and induced mutations. Yeast 38, 187–196. doi:10.1002/yea.3530
González-Guerrero, M., Benabdellah, K., Valderas, A., Azcón-Aguilar, C., and Ferrol, N. (2010). GintABC1 encodes a putative ABC transporter of the MRP subfamily induced by Cu, Cd, and oxidative stress in Glomus intraradices. Mycorrhiza 20, 137–146. doi:10.1007/s00572-009-0273-y
Green, L., Coronado-Zamora, M., Radío, S., Rech, G. E., Salces-Ortiz, J., and Gonzalez, J. (2022). The genomic basis of copper tolerance in Drosophila is shaped by a complex interplay of regulatory and environmental factors. BMC Biol. 20, 275. doi:10.1186/s12915-022-01479-w
Gupta, S. C., Sharma, A., Mishra, M., Mishra, R. K., and Chowdhuri, D. K. (2010). Heat shock proteins in toxicology: How close and how far? Life Sci. 86, 377–384. doi:10.1016/j.lfs.2009.12.015
Hartl, D. L., and Clark, G. C. (1997). Principles of population genetics. Sunderland: Sinauer Associates.
He, Z., Shentu, J., Yang, X., Baligar, V. C., Zhang, T., and Stoffella, P. J. (2015). Heavy metal contamination of soils: Sources, indicators, and assessment. J. Environ. Indic. 9, 17–18.
Heikens, A., Peijnenburg, W. J. G. M., and Hendriks, A. J. (2001). Bioaccumulation of heavy metals in terrestrial invertebrates. Environ. Pollut. 113, 385–393. doi:10.1016/S0269-7491(00)00179-2
Hivert, V., Leblois, R., Petit, E. J., Gautier, M., and Vitalis, R. (2018). Measuring genetic differentiation from pool-seq data. Genetics 210, 315–330. doi:10.1534/genetics.118.300900
Hua, H., Günther, V., Georgiev, O., and Schaffner, W. (2011). Distorted copper homeostasis with decreased sensitivity to cisplatin upon chaperone Atox1 deletion in Drosophila. BioMetals 24, 445–453. doi:10.1007/s10534-011-9438-1
Huang, W., Massouras, A., Inoue, Y., Peiffer, J., Ràmia, M., Tarone, A. M., et al. (2014). Natural variation in genome architecture among 205 Drosophila melanogaster Genetic Reference Panel lines. Genome Res. 24, 1193–1208. doi:10.1101/gr.171546.113
Huang, W., Campbell, T., Carbone, M. A., Jones, W. E., Unselt, D., Anholt, R. R. H., et al. (2020). Context-dependent genetic architecture of Drosophila life span. PLOS Biol. 18, e3000645. doi:10.1371/journal.pbio.3000645
Hull, R. M., Cruz, C., Jack, C. V., and Houseley, J. (2017). Environmental change drives accelerated adaptation through stimulated copy number variation. PLOS Biol. 15, e2001333. doi:10.1371/journal.pbio.2001333
Huster, D., and Lutsenko, S. (2007). Wilson disease: Not just a copper disorder. Analysis of a wilson disease model demonstrates the link between copper and lipid metabolism. Mol. Biosyst. 3, 816–824. doi:10.1039/b711118p
Illumina (2020). Data concordance between the NextSeq 1000, NextSeq 2000, and NextSeq 550 seqyencing systems. Illumina.
Ives, P. T. (1945). The genetic structure of American populations of Drosophila melanogaster. Genetics 30, 167–196. doi:10.1093/genetics/30.2.167
Jacobs, H., Stratmann, R., and Lehner, C. F. (1998). A screen for lethal mutations in the chromosomal region 59AB suggests that bellwether encodes the alpha subunit of the mitochondrial ATP synthase in Drosophila melanogaster. Mol. Gen. Genet. MGG 259, 383–387. doi:10.1007/s004380050826
Janssens, T. K. S., Roelofs, D., and van Straalen, N. M. (2009). Molecular mechanisms of heavy metal tolerance and evolution in invertebrates. Insect Sci. 16, 3–18. doi:10.1111/j.1744-7917.2009.00249.x
Jarosz, D. F., and Lindquist, S. (2010). Hsp90 and environmental stress transform the adaptive value of natural genetic variation. Science 330, 1820–1824. doi:10.1126/science.1195487
Kang, W., Zheng, J., Bao, J., Wang, Z., Zheng, Y., He, J. Z., et al. (2020). Characterization of the copper resistance mechanism and bioremediation potential of an Acinetobacter calcoaceticus strain isolated from copper mine sludge. Environ. Sci. Pollut. Res. 27, 7922–7933. doi:10.1007/s11356-019-07303-3
Karin, M., Najarian, R., Haslinger, A., Valenzuela, P., Welch, J., and Fogel, S. (1984). Primary structure and transcription of an amplified genetic locus: The CUP1 locus of yeast. Proc. Natl. Acad. Sci. 81, 337–341. doi:10.1073/pnas.81.2.337
Karnchanawong, S., and Limpiteeprakan, P. (2009). Evaluation of heavy metal leaching from spent household batteries disposed in municipal solid waste. Waste Manag. 29, 550–558. doi:10.1016/j.wasman.2008.03.018
Kelly, J. K., and Hughes, K. A. (2019). Pervasive linked selection and intermediate-frequency alleles are implicated in an evolve-and-resequencing experiment of Drosophila simulans. Genetics 211, 943–961. doi:10.1534/genetics.118.301824
King, E. G., Macdonald, S. J., and Long, A. D. (2012). Properties and power of the Drosophila Synthetic Population Resource for the routine dissection of complex traits. Genetics 191, 935–949. doi:10.1534/genetics.112.138537
Lam, H. Y. K., Clark, M. J., Chen, R., Chen, R., Natsoulis, G., O'Huallachain, M., et al. (2012). Performance comparison of whole-genome sequencing platforms. Nat. Biotechnol. 30, 78–82. doi:10.1038/nbt.2065
Lee, G., Bigham, J. M., and Faure, G. (2002). Removal of trace metals by coprecipitation with Fe, Al and Mn from natural waters contaminated with acid mine drainage in the Ducktown Mining District, Tennessee. Appl. Geochem. 17, 569–581. doi:10.1016/S0883-2927(01)00125-1
Leitch, K. J., Ponce, F. V., Dickson, W. B., van Breugel, F., and Dickinson, M. H. (2021). The long-distance flight behavior of Drosophila supports an agent-based model for wind-assisted dispersal in insects. Proc. Natl. Acad. Sci. 118, e2013342118. doi:10.1073/pnas.2013342118
Li, H., and Durbin, R. (2009). Fast and accurate short read alignment with Burrows-Wheeler transform. Bioinformatics 25, 1754–1760. doi:10.1093/bioinformatics/btp324
Li, H., Handsaker, B., Wysoker, A., Fennell, T., Ruan, J., Homer, N., et al. (2009). The sequence alignment/map format and SAMtools. Bioinformatics 25, 2078–2079. doi:10.1093/bioinformatics/btp352
Li, J., Liu, Y.-R., Cui, L.-J., Hu, H.-W., Wang, J.-T., and He, J. Z. (2017). Copper pollution increases the resistance of soil archaeal community to changes in water regime. Microb. Ecol. 74, 877–887. doi:10.1007/s00248-017-0992-0
Li, C., Zhou, K., Qin, W., Tian, C., Qi, M., Yan, X., et al. (2019). A review on heavy metals contamination in soil: Effects, sources, and remediation techniques. Soil Sediment. Contam. Int. J. 28, 380–394. doi:10.1080/15320383.2019.1592108
Li, X.-D., Xin, L., Rong, W.-T., Liu, X.-Y., Deng, W.-A., Qin, Y. C., et al. (2021). Effect of heavy metals pollution on the composition and diversity of the intestinal microbial community of a pygmy grasshopper (Eucriotettix oculatus). Ecotoxicol. Environ. Saf. 223, 112582. doi:10.1016/j.ecoenv.2021.112582
Liu, L., Li, W., Song, W., and Guo, M. (2018). Remediation techniques for heavy metal-contaminated soils: Principles and applicability. Sci. Total Environ. 633, 206–219. doi:10.1016/j.scitotenv.2018.03.161
Lu, K., Song, Y., and Zeng, R. (2021). The role of cytochrome P450-mediated detoxification in insect adaptation to xenobiotics. Curr. Opin. Insect Sci. 43, 103–107. doi:10.1016/j.cois.2020.11.004
Luo, M., Finet, C., Cong, H., Wei, H., and Chung, H. (2020). The evolution of insect metallothioneins. Proc. R. Soc. B Biol. Sci. 287, 20202189–20202210. doi:10.1098/rspb.2020.2189
Lynch, M., and Walsh, B. (1998). Genetic and analysis of quantitative traits. Sunderland, MA: Sinauer.
Mackay, T. F. C., Richards, S., Stone, E. A., Barbadilla, A., Ayroles, J. F., Zhu, D., et al. (2012). The Drosophila melanogaster genetic reference panel. Nature 482, 173–178. doi:10.1038/nature10811
MacNair, M. R., Smith, S. E., and Cumbes, Q. J. (1993). Heritability and distribution of variation in degree of copper tolerance in Mimulus guttatus at Copperopolis, California. Heredity 71, 445–455. doi:10.1038/hdy.1993.162
MacNair, M. R. (1983). The genetic control of copper tolerance in the yellow monkey flower, Mimulus guttatus. Heredity 53, 283–293. doi:10.1038/hdy.1983.30
Mahapatra, C. T., Bond, J., Rand, D. M., and Rand, M. D. (2010). Identification of methylmercury tolerance gene candidates in Drosophila. Toxicol. Sci. 116, 225–238. doi:10.1093/toxsci/kfq097
Maroni, G., and Watson, D. (1985). Uptake and binding of cadmium, copper and zinc by Drosophila melanogaster larvae. Insect Biochem. 15, 55–63. doi:10.1016/0020-1790(85)90044-7
Martins, N., Lopes, I., Harper, R. M., Ross, P., and Ribeiro, R. (2007). Differential resistance to copper and mine drainage in Daphnia longispina: Relationship with allozyme genotypes. Environ. Toxicol. Chem. 26, 1904–1909. doi:10.1897/06-111R.1
Mateo, L., Rech, G. E., and González, J. (2018). Genome-wide patterns of local adaptation in Western European Drosophila melanogaster natural populations. Sci. Rep. 8, 16143. doi:10.1038/s41598-018-34267-0
McNeilly, T. (1968). Evolution in closely adjacent plant populations III. Agrostis tenuis on a small copper mine. Heredity 23, 99–108. doi:10.1038/hdy.1968.8
Medeiros, D. M., and Jennings, D. (2002). Role of copper in mitochondrial biogenesis via interaction with ATP synthase and cytochrome c oxidase. J. Bioenerg. Biomembr. 34, 389–395. doi:10.1023/A:1021206220851
Merritt, T. J. S., and Bewick, A. J. (2017). Genetic diversity in insect metal tolerance. Front. Genet. 8, 172. doi:10.3389/fgene.2017.00172
Michelmore, R. W., Paran, I., and Kesseli, R. V. (1991). Identification of markers linked to disease-resistance genes by bulked segregant analysis: A rapid method to detect markers in specific genomic regions by using segregating populations. Proc. Natl. Acad. Sci. 88, 9828–9832. doi:10.1073/pnas.88.21.9828
Moleirinho, A., Carneiro, J., Matthiesen, R., Silva, R. M., Amorim, A., Azevedo, L., et al. (2011). Gains, losses and changes of function after gene duplication: Study of the metallothionein family. PLoS ONE 6, e18487. doi:10.1371/journal.pone.0018487
Morozova, T. V., Mackay, T. F. C., and Anholt, R. R. H. (2014). Genetics and genomics of alcohol sensitivity. Mol. Genet. Genomics 289, 253–269. doi:10.1007/s00438-013-0808-y
Mukhopadhyay, I., Nazir, A., Saxena, D. K., and Chowdhuri, D. K. (2003). Heat shock response: hsp70 in environmental monitoring. J. Biochem. Mol. Toxicol. 17, 249–254. doi:10.1002/jbt.10086
Ng, A., and Xavier, R. J. (2011). Leucine-rich repeat (LRR) proteins: Integrators of pattern recognition and signaling in immunity. Autophagy 7, 1082–1084. doi:10.4161/auto.7.9.16464
Norgate, M., Southon, A., Zou, S., Zhan, M., Sun, Y., Batterham, P., et al. (2007). Copper homeostasis gene discovery in Drosophila melanogaster. BioMetals 20, 683–697. doi:10.1007/s10534-006-9075-2
Olaniran, A., Balgobind, A., and Pillay, B. (2013). Bioavailability of heavy metals in soil: Impact on microbial biodegradation of organic compounds and possible improvement strategies. Int. J. Mol. Sci. 14, 10197–10228. doi:10.3390/ijms140510197
Pan, B., Ren, L., Onuchic, V., Guan, M., Kusko, R., Bruinsma, S., et al. (2022). Assessing reproducibility of inherited variants detected with short-read whole genome sequencing. Genome Biol. 23, 2. doi:10.1186/s13059-021-02569-8
Papadopulos, A. S. T., Helmstetter, A. J., Osborne, O. G., Comeault, A. A., Wood, D. P., Straw, E. A., et al. (2021). Rapid parallel adaptation to anthropogenic heavy metal pollution. Mol. Biol. Evol. 38, 3724–3736. doi:10.1093/molbev/msab141
Paradis, E., Claude, J., and Strimmer, K. (2004). APE: Analyses of phylogenetics and evolution in R language. Bioinformatics 20, 289–290. doi:10.1093/bioinformatics/btg412
Peña, N., Antón, A., Kamilaris, A., and Fantke, P. (2018). Modeling ecotoxicity impacts in vineyard production: Addressing spatial differentiation for copper fungicides. Sci. Total Environ. 616–617, 796–804. doi:10.1016/j.scitotenv.2017.10.243
Pinheiro, J., Bates, D., DebRoy, S., and Sarkar, D.R Core Team (2017). nlme: linear and nonlinear mixed effects models. R Package Version, 31–131.
Pool, J. E. (2016). Genetic mapping by bulk segregant analysis in Drosophila: Experimental design and simulation-based inference. Genetics 204, 1295–1306. doi:10.1534/genetics.116.192484
Poste, E. P. (1932). The Tennessee Copper Basin. Ind. Eng. Chem. 24, 690–694. doi:10.1021/ie50270a030
Poupardin, R., Reynaud, S., Strode, C., Ranson, H., Vontas, J., and David, J. P. (2008). Cross-induction of detoxification genes by environmental xenobiotics and insecticides in the mosquito Aedes aegypti: Impact on larval tolerance to chemical insecticides. Insect biochem. Mol. Biol. 38, 540–551. doi:10.1016/j.ibmb.2008.01.004
Price, T. D., Qvarnström, A., and Irwin, D. E. (2003). The role of phenotypic plasticity in driving genetic evolution. Proc. R. Soc. Lond. B Biol. Sci. 270, 1433–1440. doi:10.1098/rspb.2003.2372
Pyza, E., Mak, P., Kramarz, P., and Laskowski, R. (1997). Heat shock proteins (HSP70) as biomarkers in ecotoxicological studies. Ecotoxicol. Environ. Saf. 38, 244–251. doi:10.1006/eesa.1997.1595
Rangen, K., and Mosby, D. E. (2015). Addendum damage assessment plan for southeast Missouri lead mining district: Madison County mines site. State of Missouri Department of Natural Resources.
Reis, E. M., Guerra, W. D., Reis, A. C., Zanatta, M., Carmona, M., and Sautura, F. (2021). Fungi resistance to multissite fungicides. J. Agric. Sci. 13, 141. doi:10.5539/jas.v13n11p141
Riaz, M. A., Poupardin, R., Reynaud, S., Strode, C., Ranson, H., and David, J. P. (2009). Impact of glyphosate and benzo[a]pyrene on the tolerance of mosquito larvae to chemical insecticides. Role of detoxification genes in response to xenobiotics. Aquat. Toxicol. 93, 61–69. doi:10.1016/j.aquatox.2009.03.005
Roca, L. F., Moral, J., Viruega, J. R., Ávila, A., Oliveira, R., and Trapero-Casas, A. (2007). Copper fungicides in the control of olive diseases. Inf. Bull. FAO 26, 48–50.
Roelofs, D., Overhein, L., de Boer, M. E., Janssens, T. K. S., and van Straalen, N. M. (2006). Additive genetic variation of transcriptional regulation: Metallothionein expression in the soil insect Orchesella cincta. Heredity 96, 85–92. doi:10.1038/sj.hdy.6800756
Roh, J.-Y., Lee, J., and Choi, J. (2006). Assessment of stress-related gene expression in the heavy metal-exposed nematode Caenorhabditis elegans: A potential biomarker for metal-induced toxicity monitoring and environmental risk assessment. Environ. Toxicol. Chem. 25, 2946–2956. doi:10.1897/05-676R.1
Ruiz, L. M., Libedinsky, A., and Elorza, A. A. (2021). Role of copper on mitochondrial function and metabolism. Front. Mol. Biosci. 8, 711227. doi:10.3389/fmolb.2021.711227
Saad, A. A., El-Sikaily, A., and Kassem, H. (2016). Metallothionein and glutathione content as biomarkers of metal pollution in mussels and local fishermen in Abu Qir Bay, Egypt. J. Health Pollut. 6, 50–60. doi:10.5696/2156-9614-6-12.50
Sánchez-Chardi, A., and Nadal, J. (2007). Bioaccumulation of metals and effects of landfill pollution in small mammals. Part I. The greater white-toothed shrew, Crocidura russula. Chemosphere 68, 703–711. doi:10.1016/j.chemosphere.2007.01.042
Schutte, G. C., Beeton, K. V., and Kotzé, J. M. (1997). Rind stippling on Valencia oranges by copper fungicides used for control of citrus black spot in South Africa. Plant Dis. 81, 851–854. doi:10.1094/PDIS.1997.81.8.851
Suman, J., Uhlik, O., Viktorova, J., and Macek, T. (2018). Phytoextraction of heavy metals: A promising tool for clean-up of polluted environment? Front. Plant Sci. 9, 1476. doi:10.3389/fpls.2018.01476
Tabrez, S., and Ahmad, M. (2013). Cytochrome P450 system as potential biomarkers of certain toxicants: Comparison between plant and animal models. Environ. Monit. Assess. 185, 2977–2987. doi:10.1007/s10661-012-2765-z
The UniProt Consortium Bateman, A., Martin, M.-J. S. O., and Magrane, M. (2021). UniProt: The universal protein knowledgebase in 2021. Nucleic Acids Res. 49, D480–D489. doi:10.1093/nar/gkaa1100
Thurmond, J., Goodman, J. L., Strelets, V. B., Attrill, H., Gramates, L. S., Marygold, S. J., et al. (2019). FlyBase 2.0: The next generation. Nucleic Acids Res. 47, D759–D765. doi:10.1093/nar/gky1003
Vaisnav, M., Xing, C., Ku, H.-C., Hwang, D., Stojadinovic, S., Pertsemlidis, A., et al. (2014). Genome-wide association analysis of radiation resistance in Drosophila melanogaster. PLoS ONE 9, e104858. doi:10.1371/journal.pone.0104858
Vareda, J. P., Valente, A. J. M., and Durães, L. (2019). Assessment of heavy metal pollution from anthropogenic activities and remediation strategies: A review. J. Environ. Manage. 246, 101–118. doi:10.1016/j.jenvman.2019.05.126
Verspoor, R. L., and Haddrill, P. R. (2011). Genetic diversity, population structure and Wolbachia infection status in a worldwide sample of Drosophila melanogaster and D. simulans populations. PLoS ONE 6, e26318. doi:10.1371/journal.pone.0026318
Walsh, B., and Lynch, M. (2018). Evolution and selection of quantitative traits. Oxford: Oxford University Press.
Weber, M., Trampczynska, A., and Clemens, S. (2006). Comparative transcriptome analysis of toxic metal responses in Arabidopsis thaliana and the Cd2+-hypertolerant facultative metallophyte Arabidopsis halleri. Plant Cell Environ. 29, 950–963. doi:10.1111/j.1365-3040.2005.01479.x
Williams, J. H., Petersen, N. S., Young, P. A., Stansbury, M. A., Farag, A. M., and Bergman, H. L. (1996). Accumulation of hsp70 in juvenile and adult rainbow trout gill exposed to metal-contaminated water and/or diet. Environ. Toxicol. Chem. 15, 1324–1328. doi:10.1002/etc.5620150810
Wood, Z. A., Schröder, E., Robin Harris, J., and Poole, L. B. (2003). Structure, mechanism and regulation of peroxiredoxins. Trends biochem. Sci. 28, 32–40. doi:10.1016/S0968-0004(02)00003-8
Wright, K. M., Lloyd, D., Lowry, D. B., Macnair, M. R., and Willis, J. H. (2013). Indirect evolution of hybrid lethality due to linkage with selected locus in Mimulus guttatus. PLoS Biol. 11, e1001497. doi:10.1371/journal.pbio.1001497
Wright, K. M., Hellsten, U., Xu, C., Jeong, A. L., Sreedasyam, A., Chapman, J. A., et al. (2015). Adaptation to heavy-metal contaminated environments proceeds via selection on pre-existing genetic variation. bioRxiv. doi:10.1101/029900
Xie, L., and Klerks, P. L. (2003). Responses to selection for cadmium resistance in the least killifish, Heterandria formosa. Environ. Toxicol. Chem. 22, 313–320. doi:10.1002/etc.5620220211
Yepiskoposyan, H., Egli, D., Fergestad, T., Selvaraj, A., Treiber, C., Multhaup, G., et al. (2006). Transcriptome response to heavy metal stress in Drosophila reveals a new zinc transporter that confers resistance to zinc. Nucleic Acids Res. 34, 4866–4877. doi:10.1093/nar/gkl606
Yusof, N. A., Masnoddin, M., Charles, J., Thien, Y. Q., Nasib, F. N., Wong, C. M. V. L., et al. (2022). Can heat shock protein 70 (HSP70) serve as biomarkers in Antarctica for future ocean acidification, warming and salinity stress? Polar Biol. 45, 371–394. doi:10.1007/s00300-022-03006-7
Zhang, B., Egli, D., Georgiev, O., and Schaffner, W. (2001). The Drosophila homolog of mammalian zinc finger factor MTF-1 activates transcription in response to heavy metals. Mol. Cell. Biol. 21, 4505–4514. doi:10.1128/MCB.21.14.4505-4514.2001
Zhou, S., Morozova, T. V., Hussain, Y. N., Luoma, S. E., McCoy, L., Yamamoto, A., et al. (2016). The genetic basis for variation in sensitivity to lead toxicity in Drosophila melanogaster. Environ. Health Perspect. 124, 1062–1070. doi:10.1289/ehp.1510513
Zhou, S., Luoma, S. E., Armour, G. E. St., Thakkar, E., Mackay, T. F. C., Anholt, R. R. H., et al. (2017). A Drosophila model for toxicogenomics: Genetic variation in susceptibility to heavy metal exposure. PLOS Genet. 13, e1006907. doi:10.1371/journal.pgen.1006907
Zhu, F.-Y., Li, L., Lam, P. Y., Chen, M.-X., Chye, M.-L., and Lo, C. (2013). Sorghum extracellular Leucine-Rich Repeat protein SbLRR2 mediates lead tolerance in transgenic Arabidopsis. Plant Cell Physiol. 54, 1549–1559. doi:10.1093/pcp/pct101
Zischka, H., and Einer, C. (2018). Mitochondrial copper homeostasis and its derailment in Wilson disease. Int. J. Biochem. Cell Biol. 102, 71–75. doi:10.1016/j.biocel.2018.07.001
Keywords: heavy metals, toxicity, stress resistance, genome wide association, Drosophila
Citation: Everman ER, Macdonald SJ and Kelly JK (2023) The genetic basis of adaptation to copper pollution in Drosophila melanogaster. Front. Genet. 14:1144221. doi: 10.3389/fgene.2023.1144221
Received: 14 January 2023; Accepted: 21 March 2023;
Published: 04 April 2023.
Edited by:
Chao Tong, University of Pennsylvania, United StatesReviewed by:
Palle Duun Rohde, Aalborg University, DenmarkCopyright © 2023 Everman, Macdonald and Kelly. This is an open-access article distributed under the terms of the Creative Commons Attribution License (CC BY). The use, distribution or reproduction in other forums is permitted, provided the original author(s) and the copyright owner(s) are credited and that the original publication in this journal is cited, in accordance with accepted academic practice. No use, distribution or reproduction is permitted which does not comply with these terms.
*Correspondence: Elizabeth R. Everman, ZS5ldmVybWFuQGt1LmVkdQ==
Disclaimer: All claims expressed in this article are solely those of the authors and do not necessarily represent those of their affiliated organizations, or those of the publisher, the editors and the reviewers. Any product that may be evaluated in this article or claim that may be made by its manufacturer is not guaranteed or endorsed by the publisher.
Research integrity at Frontiers
Learn more about the work of our research integrity team to safeguard the quality of each article we publish.