- 1Biological Resource Centre, Precision Medicine Lab, Transfusion Medicine, Fondazione IRCCS Ca’ Granda Ospedale Maggiore Policlinico Milano, Milano, Italy
- 2Department of Pathophysiology and Transplantation, Università Degli Studi di Milano, Milano, Italy
Background: The cause of chronic liver diseases (CLD) remains undiagnosed in up to 30% of adult patients. Whole-Exome Sequencing (WES) can improve the diagnostic rate of genetic conditions, but it is not yet widely available, due to the costs and the difficulties in results interpretation. Targeted panel sequencing (TS) represents an alternative more focused diagnostic approach.
Aims: To validate a customized TS for hereditary CLD diagnosis.
Methods: We designed a customized panel including 82 CLD-associated genes (iron overload, lipid metabolism, cholestatic diseases, storage diseases, specific hereditary CLD and susceptibility to liver diseases). DNA samples from 19 unrelated adult patients with undiagnosed CLD were analyzed by both TS (HaloPlex) and WES (SureSelect Human All Exon kit v5) and the diagnostic performances were compared.
Results: The mean depth of coverage of TS-targeted regions was higher with TS than WES (300x vs. 102x; p < 0.0001). Moreover, TS yielded a higher average coverage per gene and lower fraction of exons with low coverage (p < 0.0001). Overall, 374 unique variants were identified across all samples, 98 of which were classified as “Pathogenic” or “Likely Pathogenic” with a high functional impact (HFI). The majority of HFI variants (91%) were detected by both methods; 6 were uniquely identified by TS and 3 by WES. Discrepancies in variant calling were mainly due to variability in read depth and insufficient coverage in the corresponding target regions. All variants were confirmed by Sanger sequencing except two uniquely detected by TS. Detection rate and specificity for variants in TS-targeted regions of TS were 96.9% and 97.9% respectively, whereas those of WES were 95.8% and 100%, respectively.
Conclusion: TS was confirmed to be a valid first-tier genetic test, with an average mean depth per gene higher than WES and a comparable detection rate and specificity.
Introduction
Chronic liver disease (CLD) is a major health concern whose prevalence and related morbidity and mortality continues to rise, accounting for over 2 million deaths annually worldwide (Karlsen et al., 2022). Despite advances in clinical and instrumental workup, the causes of CLD remain unknown in a large fraction of adults, ranging from 14% to 30% (Vilarinho and Mistry, 2019; Gao et al., 2021). In the last decade, advances in genetic technologies, using a next-generation sequencing (NGS) approach, have enhanced and broadened the comprehension of the causes of rare genetic disorders, increasing the understanding of CLD etiology and allowing to diagnose a large fraction of cases previously classified as “cryptogenic.” Whole-exome sequencing (WES) has been shown to yield a high diagnostic rate not only in pediatric population but also in adults with undiagnosed CLD (Pelusi et al., 2022; Valenti and Ronzoni, 2022; Zheng et al., 2022). Although WES is already available in clinical practice for some specific indications, there are some remaining challenges on the road to a more extensive implementation. These include the need of specific expertise related to both data analysis and interpretation, and the time and costs associated with analysis and diagnostic interpretation of genomic data. Furthermore, WES can detect incidental actionable inherited conditions, not related to primary indications for testing, which raises ethical issues (Hakim et al., 2019; Valenti et al., 2019).
Customized targeted gene panel sequencing (TS) focused on a limited set of genes specific for CLD is an alternative to WES. TS has the advantage of providing higher coverage that increases analytical sensitivity even in the detection of mosaicism, of being easier to interpret and of avoiding incidental secondary findings.
In this study, we optimized a customized TS for hereditary CLD diagnosis and compared its performance to that of WES in a cohort of 19 unrelated adult patients with undiagnosed CLD.
Materials and methods
Patients’ selection and study design
From January 2021 to December 2021, 19 unrelated adult patients with undiagnosed CLD with a suspected hereditary etiology, referred to the Hepatology outpatient service and Precision Medicine Lab of the Department of Pathophysiology and Transplantation, Transfusion Medicine Unit of the Fondazione IRCCS Ca’ Granda Ospedale Maggiore Policlinico of Milan, for clinical and genetic evaluation, were included into the study. All were of European ancestry. Viral and autoimmune hepatitis were ruled out using standard clinical and laboratory evaluation; CLD due to alcohol abuse were excluded.
For each patient, a peripheral blood DNA sample was analyzed by both TS and WES, filtered for the genes included in target panel design, and their performances were compared.
The study protocol was approved by the Ethical Committee of Fondazione IRCCS Ca’ Granda (CE 125_2018bis). Written informed consent for genetic analysis was obtained from each patient.
Custom targeted gene panel design
Based on data from the Human Genome Mutation Database (HGMD), Online Mendelian Inheritance in Man (OMIM) database (http://www.ncbi.nlm.nih.gov/omim), and an extensive literature review using PubMed, we selected 82 disease-causing genes associated to CLD, classified into six categories: iron overload, lipid metabolism, cholestatic diseases, storage diseases, specific hereditary CLD and genes associated to susceptibility to liver diseases (Supplementary Table S1). For each gene, we considered the coding exons with 25 flanking bp; selected 5'-/3′-UTRs and promoter regions known to harbor pathogenic variants were also included. Probes specific for the selected regions were designed with the HaloPlex online design tool (SureDesign, Agilent Technologies Inc.), using GRCh37-hg19 as reference genome.
DNA extraction
DNA was extracted from peripheral blood and quantified by a Qubit 2.0 analyzer using the Qubit dsDNA BR Assay Kit (Thermo-Fisher, Waltham, MA, United States). Sample purity was evaluated using a Nanodrop 1,000 spectrophotometer (Thermo-Fisher, Waltham, MA, United States) and integrity was assessed by gel electrophoresis and using the Agilent 2,200 TapeStation System and the Genomic DNA ScreenTape assay for DNA Integrity Number (DIN) assessment (Agilent Technologies, Santa Clara, CA, United States). Samples with a 260/280 ratio of absorbance ≥1.8 and a DIN ≥6.4 were used.
Targeted sequencing and analysis
Amplicon libraries were prepared from genomic DNA using the HaloPlex Target Enrichment System specific for the designed CLD panel (Agilent, Cernusco sul Naviglio, Milan, Italy), according to the manufacture’s protocol. Sequencing was performed on MiSeq platform (Illumina, San Diego, CA). FASTQ files were analyzed using SureCall version 4.2.2 (Agilent, Cernusco sul Naviglio, Milan, Italy). Briefly, adapter sequences and lower-quality bases were removed; reads were aligned to reference genome (GRCh37-hg19) using Burrows Wheeler Aligner (BWA)-MEM algorithm. Variant calling was performed using the algorithm SNPPET SNP of SureCall. A coverage depth cutoff of 10x was applied. The obtained Variant Call Format (vcf) files were analyzed and annotated in wANNOVAR server (http://wannovar.usc.edu).
Whole exome sequencing and analysis
Genomic DNA libraries were enriched for WES by the SureSelect Human All Exon v5 kit (Agilent, Cernusco sul Naviglio, Milan, Italy). Sequencing was performed on the NextSeq 550 platform (Illumina, San Diego, CA). Variants calling and analysis were performed using a validate pipeline previously described (Pelusi et al., 2019; Baselli et al., 2022).
Briefly, raw reads quality control was performed using FastQC software (Brabaham bioinformatics, Cambridge, United Kingdom). Reads mapping on human GRCh37 genome was performed using MEM algorithm of Burrows Wheeler Aligner (BWA) version 0.7.10. Reads with low quality alignments and duplicate reads have been filtered out using SAMtools to generate high quality bam (HQ-BAM) files and mapping quality control was performed using Picard-tools and Bedtools softwares. Variants calling was performed following Genome Analysis Toolkit (GATK) best practices. Variants quality score log-odds (VQSLOD) above 99% tranche were considered true positives and variants present in <20% of total reads discarded; indel left-normalization was performed using BCFtools software and variants annotation using both variant effect predictor (VEP) and ANNOVAR tools. Only the variants included in the regions covered by TS were considered for the analysis. A coverage depth cutoff of 10x was applied. Pathogenic variants analysis was performed using ClinVar database. Variants pathogenicity was predicted using public algorithms such as CADD (Combined Annotation Dependent Depletion) and a score >20 was selected in order to classify the variants with a potential high functional impact (HFI) (Baselli et al., 2022).
Sanger sequencing
Selected variants, uniquely detected by TS or WES, were confirmed by Sanger sequencing. The specific PCR primers were designed using both Primer Design Tool-NCBI (https://www.ncbi.nlm.nih.gov/tools/primer-blast/) and Primer3 software (https://primer3.ut.ee/); primers sequences are available upon request. Amplicons were sequenced using the Big Terminator v3.1 cycle sequencing kit (Applied Biosystems™, Thermo-Fisher, Waltham, MA, United States) and a Sanger Sequencing 3,500 Dx Series Genetic Analyzer (Applied Biosystems™, Thermo-Fisher, Waltham, MA, United States). Sequences were first analyzed using the Sequencing Analysis Software v7.0 (Applied Biosystems™, Thermo-Fisher, Waltham, MA, United States) and then compared to reference genome using BLAST software (https://blast.ncbi.nlm.nih.gov/Blast.cgi).
Statistical analysis
For statistical analysis, non-parametric Wilcoxon Signed Ranked test or Chi-squared test were used, as appropriate. p values < 0.05 (two tailed) were considered statistically significant.
Results
TS and WES coverage
The mean depth of coverage of the TS-targeted regions obtained with TS was higher, although more variable, than that obtained with WES in all the samples, with an average depth of 300x for TS (range 122-738x) and 102x for WES (range 94-119x), and with 97% and 87% of the targeted regions covered at 10x sequence depth in TS and WES respectively (Table 1).
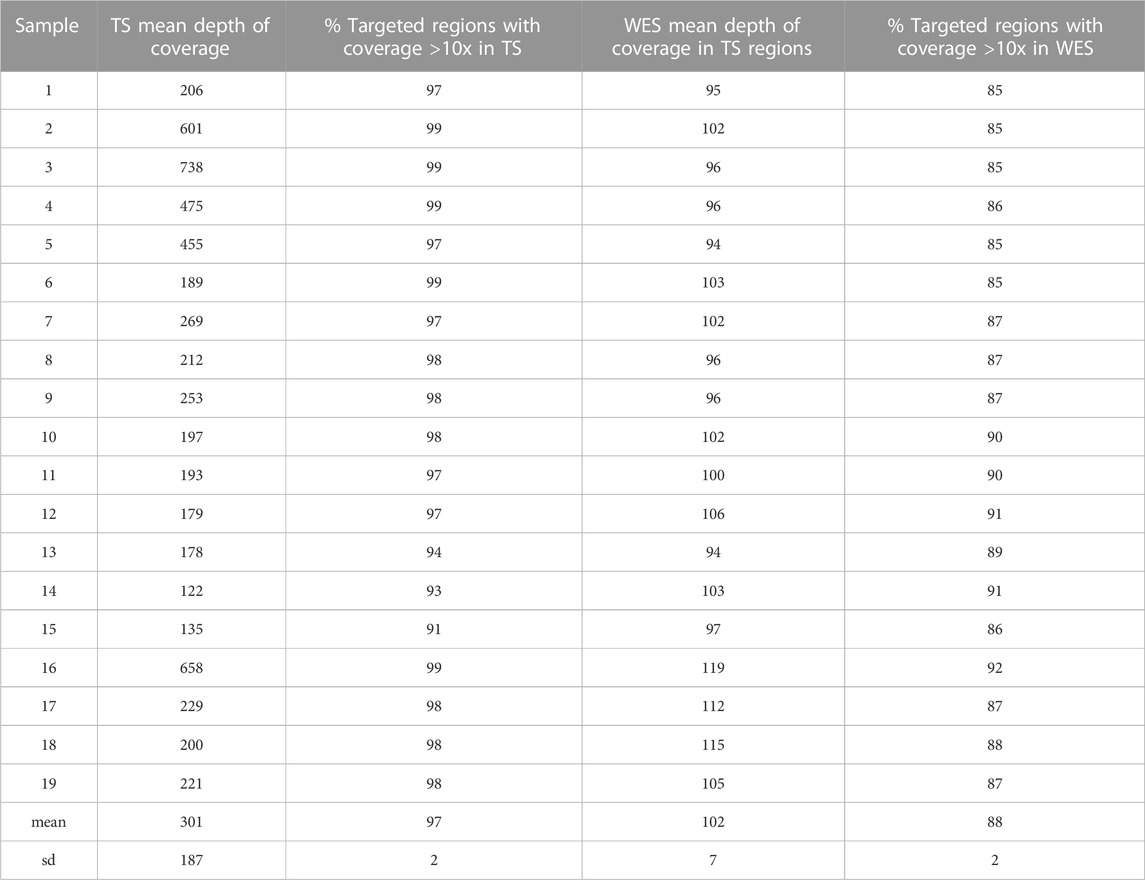
TABLE 1. Mean depth of coverage and percentage of targeted regions with coverage >10x in TS and WES.
We next examined the coverage on a gene-by-gene basis by pooling data from all samples. TS yielded higher average mean depth per gene than WES (p = 3.7e-15) (Figure 1A), and had substantially fewer exons with less than tenfold mean depth (0.2% vs 2.8%; p = 2.1e-06) (Figure 1B)
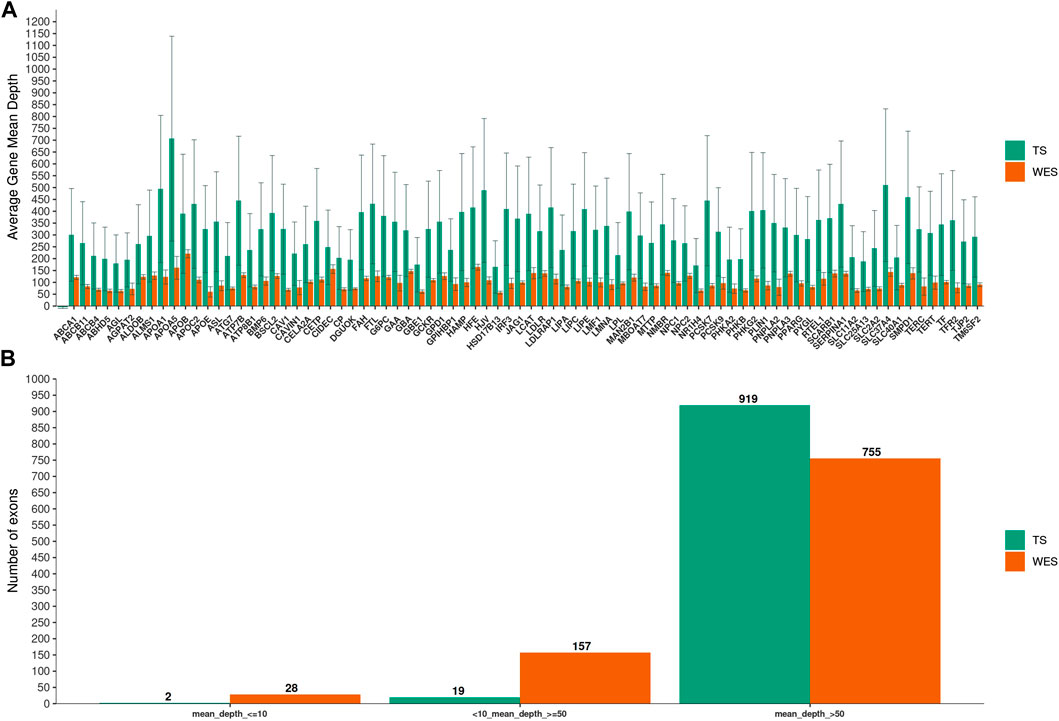
FIGURE 1. Mean depth of coverage per chronic liver diseases genes. In (A), Targeted Sequencing (TS) yielded significantly higher average mean depth per gene compared to Whole-Exome Sequencing (WES). Bars indicate standard deviation. In (B), the fraction of exons covered to the given depth for each methods is shown.
Variants detection
In order to compare TS and WES performance in variants detection, we first considered the total number of variants identified across all samples in the regions covered by TS design (exonic, UTR and splice-site variants).
Overall, 374 unique variants were identified across all samples, 288 of which (77%) were identified by both methods, 66 (18%) uniquely by TS and 20 (5%) by WES only. We then restricted the analysis to variants with a potential HFI. Specifically, we considered exonic non-synonymous (missense, frameshift, nonsense), UTR or splice-site variants. Among these, we next selected variants with at least one of the following characteristics: a) reported as “Pathogenic” or “Likely Pathogenic” in the ClinVar database; b) rare variants of unknown significance (VUS) with a minor allele frequency (MAF) < 0.005 according to public database (ExAC_NFE); or c) VUS with a MAF between 0.005 and 0.05 and a CADD score >20.
We identified a total of 98 HFI variants, 89 of which were detected by both methods. We next focused on the 9 HFI variants uniquely detected by TS or WES to analyze the possible reasons underlying these discrepancies. All these variants were also analyzed by Sanger sequencing.
(i) Variants uniquely detected by TS
All the six variants uniquely detected by TS were exonic non-synonymous ones (Table 2). Two variants (rs201365106 in RTEL1 and rs78250081 in SMPD1 gene) were not confirmed by Sanger sequencing. One variant (rs201365106) was a Single Nucleotide Variant (SNV) in a GC-rich region of RTEL1 gene, while the other one (rs78250081) was a SNV affecting a repetitive hexanucleotide GCTGGC sequence in a polymorphic region of SMPD1 gene (Supplementary Figure S1). In both cases, the mis-alignment of some TS sequencing reads led to incorrect base identification with a false positive variant calling. The other four variants uniquely detected by TS were confirmed by Sanger sequencing. The causes for missing call by WES for these variants were different. In two cases, both involving the PCSK7 gene, the corresponding regions in WES had insufficient mean depth (>10x) to make an accurate base call (Supplementary Figures S2A, S2B); this could be related to the WES capture design that did not contain enough baits for these PCSK7 regions. In the other two cases, analysis of WES raw data showed that discrepancies were due to low SNV quality score estimated by the SAMtools variant identification software.
(ii) Variants uniquely detected by WES
All the three variants uniquely detected by WES were exonic and were confirmed by Sanger sequencing (Table 3). The main cause of missing call by TS was the low mean depth of the corresponding region in Target Panel design.
Overall, we identified a total of 96 true HFI variants, 89 of which (93%) were detected by both methods, 4 (4%) were uniquely identified by TS and 3 (3%) by WES only (Figure 2).
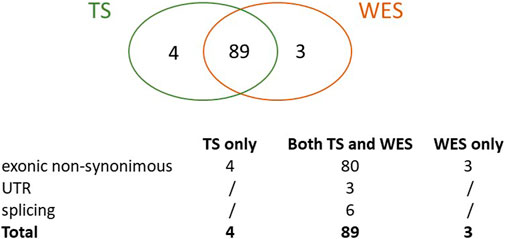
FIGURE 2. True variants with high functional impact detected by TS, WES or both. The Venn graph and the type of variants detected are shown.
TS and WES detection rate
TS and WES detection rate was calculated as the ability of TS or WES to identify a SNV over the total of detected variants. Variants detected by either one of the two methods and confirmed by Sanger sequencing were considered true positive. Detection rate of TS was 96.9% while that of WES was 95.8%. TS specificity was 97.9% and that of WES was 100%.
Discussion
TS customized for specific subsets of candidate genes has been proposed as a first-tier diagnostic approach for several hereditary diseases (Consugar et al., 2015; Butz et al., 2021; Oh et al., 2021). Compared to WES, TS has the advantage to be more focused on disease-causing genes, allowing a higher depth of coverage in targeted regions and producing a lower volume of data, thereby reducing costs and turnaround time. However, the majority of the studies supporting the higher diagnostic yields of TS did not include any cross comparisons with the diagnostic yield of WES for the same disease, or TS performance was compared to that of WES performed on different samples, or only theoretically estimated by bioinformatics approaches (LaDuca et al., 2017).
In this study, we designed a customized TS panel including 82 liver-related genes, selected on the basis of patient phenotypes and through an extensive literature review. Both targeted panel and exome sequencing were successfully performed concurrently on 19 consecutive patients.
We confirmed that TS had a higher mean depth of coverage compared to WES in the targeted regions. Particularly, TS had an average mean depth per gene significantly higher than WES and substantially fewer exons with less than tenfold mean depth (p-value<0.0001), allowing a deeper evaluation of specific target genes and regions.
Although the majority of variants with a functional or likely functional impact (89 over 98: 91%) were recognized by both methods, some variants (9 over 98) were identified by TS only (6) or WES only (3). The main reason of these discrepancies, accounting for 5 inconsistencies over a total of 9 (55%), was related to the insufficient read depth leading to missing variants call (2 missed by WES and 3 by TS). Two variants recognized by TS only were false positives, not confirmed by Sanger sequencing. These false positive calls were most likely due to alignment ambiguity in repetitive or CG-rich regions, leading to incorrect base identification by the software used for the analysis. Two other variants identified by TS only and confirmed by Sanger sequencing were missed by WES due to the analytical pipeline used for variant calling and filtering. Overall, 4 over 9 discrepancies in variant calling (45%) were accounted for by the analytical pathway rather than by the NGS approach.
Some limitations of our study should be noted. First, NGS analyses (TS and WES) were performed once for each sample, so that intra-sample reproducibility of the results could not be evaluated. This did not allow to quantify the possible role of technical variability, mainly in libraries preparation and capturing steps, that may account for insufficient coverage of specific exons in individual samples and consequently for missing variant calling. However, the strength of the study was that both TS and WES were performed on each sample, allowing a direct comparison between the two methods.
Overall, we confirmed the possible advantage of TS over WES in identifying high functional impact variants in specific cases. In fact, although in the present limited cohort of patients analyzed the detection rate and specificity between the two NGS approach were not significantly different, TS was a less laborious, less expensive and with a reduced turnaround time method. Moreover, based that the clinical utility of NGS methods mainly relied on the ability to accurately isolate or amplify the genes of interest, TS allowed a deeper evaluation of the regions of interest. Despite this advantage, one key drawback of TS was that they may become outdated rather quickly as new disease-related genes are discovered and they did not allow to identify variants in genes or regions not comprised in panel design. As a result, extending to WES as a second-tier genetic test in cases remained undiagnosed after TS, using a hierarchical strategy, would greatly increase the costs of the overall diagnostic workflow. On the other hand, WES had the important advantage of providing evidence about the putative variants related to the disease without previous biased knowledge. This feature can be useful in heterogeneous conditions such as CLD, characterized by variable expressivity and incomplete penetrance.
In conclusion, when the genetic background is well-defined and a specific hereditary CLD is suspected, TS can be used as a first-tier genetic test, supporting clinical and instrumental evaluation. However, when no suspect gene stands behind the clinical phenotype, as in cryptogenic CLD, WES can provide a wider screening option, especially in association with familial segregation studies.
Data availability statement
The genetic data has been summitted to https://www.ebi.ac.uk/eva/. Project: PRJEB62846. Analyses: ERZ18542841.
Ethics statement
The studies involving human participants were reviewed and approved by Fondazione IRCCS Ca’ Granda, Milan, Italy Ethical Committee 125_2018bis. The patients/participants provided their written informed consent to participate in this study.
Author contributions
LR concepted and designed the study, analyzed and interpreted NGS data, drafted the initial manuscript, and revised the initial and final manuscript; IM performed NGS analysis, drafted the initial manuscript; GP, FM, DM performed bioinformatic analysis, drafted the initial manuscript; CB and SP recruited patients; DP overviewed the study and revised the final manuscript; LV concepted and designed the study, overviewed the study and revised the initial and final manuscript. All authors contributed to the article and approved the submitted version.
Funding
Italian Ministry of Health (Ministero della Salute), Ricerca Finalizzata 2016, RF-2016-02364358 (“Impact of whole exome sequencing on the clinical management of patients with advanced nonalcoholic fatty liver and cryptogenic liver disease”) (LV); Italian Ministry of Health (Ministero della Salute), Fondazione IRCCS Ca’ Granda Ospedale Maggiore Policlinico, Ricerca Corrente (LV, DP); Fondazione Patrimonio Ca’ Granda, “Liver BIBLE” (PR-0361) (LV); Italian Ministry of Health (Ministero della Salute, Direzione Generale della Ricerca e dell’Innovazione in Sanità), Fondazione IRCCS Ca’ Granda, 5 × 1000 2020 funds (RC5100020G) (LV).
Conflict of interest
The authors declare that the research was conducted in the absence of any commercial or financial relationships that could be construed as a potential conflict of interest.
Publisher’s note
All claims expressed in this article are solely those of the authors and do not necessarily represent those of their affiliated organizations, or those of the publisher, the editors and the reviewers. Any product that may be evaluated in this article, or claim that may be made by its manufacturer, is not guaranteed or endorsed by the publisher.
Supplementary material
The Supplementary Material for this article can be found online at: https://www.frontiersin.org/articles/10.3389/fgene.2023.1137016/full#supplementary-material
Abbreviations
CLD, Chronic Liver Disease; NGS, Next-Generation Sequencing; WES, Whole-exome sequencing; TS, Targeted gene panel Sequencing; HGMD, Human Genome Mutation Database; OMIM, Online Mendelian Inheritance in Man; CADD, Combined Annotation Dependent Depletion; HFI, High Functional Impact; VUS, Variants of Unknown Significance; MAF, Minor Allele Frequency; SNV, Single Nucleotide Variant.
References
Baselli, G. A., Jamialahmadi, O., Pelusi, S., Ciociola, E., Malvestiti, F., Saracino, M., et al. (2022). Rare ATG7 genetic variants predispose patients to severe fatty liver disease. J. Hepatol. 77 (3), 596–606. doi:10.1016/j.jhep.2022.03.031
Butz, H., Nyírő, G., Kurucz, P. A., Likó, I., and Patócs, A. (2021). Molecular genetic diagnostics of hypogonadotropic hypogonadism: From panel design towards result interpretation in clinical practice. Hum. Genet. 140 (1), 113–134. doi:10.1007/s00439-020-02148-0
Consugar, M. B., Navarro-Gomez, D., Place, E. M., Bujakowska, K. M., Sousa, M. E., Fonseca-Kelly, Z. D., et al. (2015). Panel-based genetic diagnostic testing for inherited eye diseases is highly accurate and reproducible, and more sensitive for variant detection, than exome sequencing. Genet. Med. 17 (4), 253–261. doi:10.1038/gim.2014.172
Gao, E., Hercun, J., Heller, T., and Vilarinho, S. (2021). Undiagnosed liver diseases. Transl. Gastroenterol. Hepatol. 6, 28. doi:10.21037/tgh.2020.04.04
Hakim, A., Zhang, X., DeLisle, A., Oral, E. A., Dikas, D., Drzewiecki, K., et al. (2019). Clinical utility of genomic analysis in adults with idiopathic liver disease. J. Hepatol. 70 (6), 1214–1221. doi:10.1016/j.jhep.2019.01.036
Karlsen, T. H., Sheron, N., Zelber-Sagi, S., Carrieri, P., Dusheiko, G., Bugianesi, E., et al. (2022). The EASL-lancet liver commission: Protecting the next generation of Europeans against liver disease complications and premature mortality. Lancet 399 (10319), 61–116. doi:10.1016/S0140-6736(21)01701-3
LaDuca, H., Farwell, K. D., Vuong, H., Lu, H-M., Mu, W., Shahmirzadi, L., et al. (2017). Exome sequencing covers >98% of mutations identified on targeted next generation sequencing panels. PLoS One 12 (2), e0170843. doi:10.1371/journal.pone.0170843
Oh, J., Shin, J. I., Lee, K., Lee, C., Ko, Y., and Lee, J. S. (2021). Clinical application of a phenotype-based NGS panel for differential diagnosis of inherited kidney disease and beyond. Clin. Genet. 99 (2), 236–249. doi:10.1111/cge.13869
Pelusi, S., Baselli, G., Pietrelli, A., Dongiovanni, P., Donati, B., McCain, M. V., et al. (2019). Rare pathogenic variants predispose to hepatocellular carcinoma in nonalcoholic fatty liver disease. Sci. Rep. 9 (1), 3682. doi:10.1038/s41598-019-39998-2
Pelusi, S., Ronzoni, L., Malvestiti, F., Bianco, C., Marini, I., D'Ambrosio, R., et al. (2022). Clinical exome sequencing for diagnosing severe cryptogenic liver disease in adults: A case series. Liver Int. 42 (4), 864–870. doi:10.1111/liv.15185
Valenti, L., Pelusi, S., and Baselli, G. (2019). Whole exome sequencing for personalized hepatology: Expanding applications in adults and challenges. J. Hepatol. 71 (4), 849–850. doi:10.1016/j.jhep.2019.06.008
Valenti, L., and Ronzoni, L. (2022). Genetics: A new clinical tool for the hepatologist. Liver Int. 42 (4), 724–726. doi:10.1111/liv.15205
Vilarinho, S., and Mistry, P. K. (2019). Exome sequencing in clinical hepatology. Hepatology 70 (6), 2185–2192. doi:10.1002/hep.30826
Keywords: hereditary chronic liver diseases, targeted sequencing, gene panel design, whole exome sequencing (WES), next-generation sequencing, NGS
Citation: Ronzoni L, Marini I, Passignani G, Malvestiti F, Marchelli D, Bianco C, Pelusi S, Prati D and Valenti L (2023) Validation of a targeted gene panel sequencing for the diagnosis of hereditary chronic liver diseases. Front. Genet. 14:1137016. doi: 10.3389/fgene.2023.1137016
Received: 03 January 2023; Accepted: 30 May 2023;
Published: 14 June 2023.
Edited by:
Mingjie Wang, Shanghai Jiao Tong University, ChinaReviewed by:
Cristina Skrypnyk, Arabian Gulf University, BahrainMiki Scaravaglio, University of Milano-Bicocca, Italy
Carlos Jose Pirola, Universidad Abierta Interamericana, Argentina
Copyright © 2023 Ronzoni, Marini, Passignani, Malvestiti, Marchelli, Bianco, Pelusi, Prati and Valenti. This is an open-access article distributed under the terms of the Creative Commons Attribution License (CC BY). The use, distribution or reproduction in other forums is permitted, provided the original author(s) and the copyright owner(s) are credited and that the original publication in this journal is cited, in accordance with accepted academic practice. No use, distribution or reproduction is permitted which does not comply with these terms.
*Correspondence: Luisa Ronzoni, bHVpc2Eucm9uem9uaUBwb2xpY2xpbmljby5taS5pdA==