- 1Department of Biostatistics, Fielding School of Public Health, University of California, Los Angeles, Los Angeles, CA, United States
- 2Cancer Epidemiology Program, University of Hawaii Cancer Center, Honolulu, HI, United States
- 3Department of Epidemiology, Fielding School of Public Health, University of California, Los Angeles, Los Angeles, CA, United States
- 4Department of Computational Medicine, University of California, Los Angeles, Los Angeles, CA, United States
- 5Translational Sciences Section, School of Nursing, University of California, Los Angeles, Los Angeles, CA, United States
- 6Jonsson Comprehensive Cancer Center, University of California, Los Angeles, Los Angeles, CA, United States
Tumor subtype and menopausal status are strong predictors of breast cancer (BC) prognosis. We aimed to find and validate subtype- or menopausal-status-specific changes in tumor DNA methylation (DNAm) associated with all-cause mortality or BC progression. Associations between site-specific tumor DNAm and BC prognosis were estimated among The Cancer Genome Atlas participants (n = 692) with Illumina Infinium HumanMethylation450 BeadChip array data. All-cause mortality and BC progression were modeled using Cox proportional hazards models stratified by tumor subtypes, adjusting for age, race, stage, menopausal status, tumor purity, and cell type proportion. Effect measure modification by subtype and menopausal status were evaluated by incorporating a product term with DNAm. Site-specific inference was used to identify subtype- or menopausal-status-specific differentially methylated regions (DMRs) and functional pathways. The validation of the results was carried out on an independent dataset (GSE72308; n = 180). We identified a total of fifteen unique CpG probes that were significantly associated (
Introduction
Breast cancer (BC) is the most frequently diagnosed cancer in women. In 2020, BC accounted for 6.9% (approximately 2.3 million cases) of new cancer deaths globally (Sung et al., 2021). Advancements in screening and treatment options have contributed to improvements in BC prognosis (Goldhirsch et al., 2011). Epigenetic dysregulation is a hallmark of cancer (Jones et al., 2016) and thus epigenetic patterns may serve as predictors of risk and prognosis to guide more personalized treatment plans to further improve patient outcomes. Both in vitro and population-based studies have implicated aberrant histone and DNA methylation (DNAm) in BC tumorigenesis and progression (Dumont et al., 2008; Elsheikh et al., 2009; Stone et al., 2015). Several population-based epigenomic studies of BC tissues have investigated the prognostic utility of DNAm at specific CpG loci as the potential candidate biomarker for BC survival (Hao et al., 2017; Xiao et al., 2018; de Almeida et al., 2019; Du et al., 2019; Liu et al., 2020; Tao et al., 2020). These studies have demonstrated a selected panel of CpG loci can potentially be used to distinguish high- and low-risk groups for all-cause mortality among BC patients, improving prediction of BC prognosis.
However, prior studies of the DNAm and BC survival have focused on identifying a panel of prognostic CpG probes that are agnostic to BC molecular subtypes or menopausal status (Hao et al., 2017; de Almeida et al., 2019; Du et al., 2019; Liu et al., 2020; Tao et al., 2020). BC is heterogeneous cancer with different intrinsic subtypes defined by female hormone-receptor status (Koboldt et al., 2012). Subtypes are distinguished using gene expression arrays or immunohistochemistry, and include luminal A, luminal B, HER-2, normal-like and basal-like. BC prognosis differs by the subtypes (Byler et al., 2014; Ohnstad et al., 2017; Yang and Polley, 2019), which have guided the use of subtype-specific therapeutic regimens (Goldhirsch et al., 2011). Indeed, Xiao et al. examined DNAm sites associated with all-cause mortality among subjects with luminal BC; however, they did not examine DNAm of subjects with basal-like subtype or based on menopausal status (Xiao et al., 2018). Pre-menopausal women have a higher proportion of tumor subtypes associated with poorer prognosis (e.g., basal-like and HER2), compared to post-menopausal women (Keegan et al., 2012). Moreover, the prognosis for pre-menopausal women with luminal A or luminal B subtype is worse than that of post-menopausal women with the same subtype (Lian et al., 2017). Thus, prognoses of patients with BC could differ by their menopausal status in conjunction with their molecular subtypes. DNAm may provide a means to capture these differences in biology that are linked to BC outcomes.
In this study, we aimed to identify DNAm sites associated with BC survival outcomes in breast tumor tissue, which are specific to different BC subtypes or menopause status. We evaluated these associations among The Cancer Genome Atlas (TCGA) BC participants with Illumina Infinium HumanMethylation450 BeadChip (450K) array data obtained from breast tumor tissue samples. This subset of the TCGA BC participants had a median overall follow-up time of 27.7 months, which is considered relatively a short follow-up time for survival analysis (Liu et al., 2018). Thus, in addition to analyzing overall survival (OS), we analyzed progression-free interval (PFI), which was defined as the period between the date of diagnosis and the date of first new tumor event. We conducted an epigenome-wide association study (EWAS) of these outcomes, accounting for potential effect measure modification by BC subtypes or menopausal status. We further validated these associations in an independent cohort of 180 subjects with breast tumor tissue samples. To the best of our knowledge, this is the first study that has investigated subtype- or menopausal-status-specific DNAm sites that are associated with all-cause mortality and BC progression. Identified DNAm sites will potentially enable clinicians to provide more precise prognosis for BC patients.
Materials and methods
Study population
The breast cancer data set of 862 samples and corresponding Infinium 450K DNAm data were obtained from TCGA Genomic Data Commons (GDC), which houses molecular profiles of different cancer types from the TCGA project. The data set included four different survival outcomes, including overall survival, progression-free interval, disease-specific survival, and disease-free interval. These outcomes were defined and curated using available clinical information by Liu et el. and were made available in GDC (Liu et al., 2018). BC subtypes of samples were classified based on Spearman distance between samples’ expression profiles and 50-gene centroids (representing different subtypes) constructed using the Prediction Analysis of Microarray (PAM) algorithm (Tibshirani et al., 2002). Inclusion criterion was availability of OS or PFI endpoints. Exclusion criteria were based on tumor types, stages and missingness of covariates which were included in the model. Specifically, we excluded subjects whose menopausal status (n = 80), age (n = 1), race (n = 16) and tumor stage (n = 7) information were missing. We also excluded subjects with stage four cancer (n = 16), and adjacent normal samples (n = 110) and HER2 subtype (n = 46). We randomly chose one sample from a subject if the subject had multiple samples. Subjects whose race was not white were categorized as other, and whose stage was not three was categorized as stage one or two. The menopausal status was categorized into two categories, post-menopause, or pre- or peri-menopause. For this study, we analyzed overall survival and progression-free interval outcomes.
In the end, our study included 692 breast tumor samples from female TCGA BC participants. A majority of these participants were post-menopausal (Table 1). Tumor subtypes of TCGA participants were previously classified using the 50-gene PAM50 model (Parker et al., 2009; Koboldt et al., 2012), with 415 samples classified as luminal A, 141 as luminal-B, and 136 as basal-like (Table 1). During follow-up, 87 participants died, and 94 progressed to a new tumor event. The median follow-up time was 42 months (IQR = 55) for OS endpoint and 27 months (IQR = 36) for PFI endpoint (Table 1).
Preparation of DNAm data
We used minfi (Version 1.34.0) in R (Aryee et al., 2014) to normalize, correct for background noise, and convert the raw signal intensities into beta-values. The raw DNAm data was preprocessed using Noob (normal-exponential out-of-band) background correction with dye-bias normalization in minfi. We included 485,512 CpG loci in our analysis of the TCGA and GEO datasets.
Estimation of cell purity and cell proportions
The purity of each tumor sample was estimated using the InfiniumPurify (Version 1.3.1) R package (Qin et al., 2018). First, the top 1000 informative, differentially methylated CpG sites (iDMCs) were selected using the rank-sum test to detect significant differences in methylation levels (beta-values) between tumor and normal samples. Additionally, the variances of methylation levels of tumor samples were required to be greater than 0.005 for iDMCs. Next, iDMCs were categorized as hypermethylated if the mean beta-values of the tumor samples were greater than the normal samples, and into the hypomethylated group, otherwise. Beta-values of hypomethylated iDMCs were transformed into
The cell-type proportions of each sample was estimated using the RefFreeEWAS (Version 2.2) R package (Houseman et al., 2014). The cell proportions for each sample were estimated by non-negative matrix factorization (NMF), which decomposes the beta matrix into a cell-type-specific methylation matrix and a cell proportion matrix. We used an existing cell-type-specific methylation matrix to initialize the NMF rather than estimating it de novo. This cell-type-specific methylation matrix was previously estimated using the informative probes selected based on reference DNA methylation profiles of known cell lines, and the TCGA breast cancer data set (Onuchic et al., 2016). Eight cell types were inherited from the initial cell-type specific methylation matrix, and thus no estimation of the number of cell types was conducted. The number of iterations was set to 100. The final cell-type-specific methylation matrix did not differ much from the initial cell-type-specific methylation matrix.
Site-specific analysis
All statistical analyses were performed using R. The site-specific analysis was conducted using TCGA data. PH assumptions were assessed by examining log-log survival curves of OS and PFI for covariates including age, ethnicity, stage, menopausal status, and subtype. The log-log survival curves of PFI and OS for subtype overlapped noticeably across different subtypes. For this reason, Cox PH models was stratified by tumor subtype, allowing the baseline hazards functions to vary across the subtypes. The association between DNAm and our outcomes were assessed for each probe separately, with beta-values standardized across samples, such that the effect sizes corresponded to a one standard deviation increase in DNAm. All models were adjusted for age, race, stage, menopause status, tumor purity, and cell type proportion. For each model, the genome-wide significance level of
Functional pathway and differentially methylated region analysis
We used the functional class scoring (FCS) robust rank aggregation approach implemented in the methylGSA (Version 1.6.1) R package (Ren and Kuan, 2019) for the pathway analysis. This approach uses the site-specific EWAS inference to identify gene sets enriched among probes that are associated with survival outcomes. Two types of biases should be corrected for functional pathway analysis with DNAm data. One is the length bias arising from differing lengths of pre-defined gene sets based on molecular and cellular functions, which is addressed by FCS via Gene Set Enrichment Analysis (Subramanian et al., 2005). The other is the probe bias arising from different numbers of probes mapped to gene sets of equal size, which is addressed by the robust rank aggregation (RRA). RRA computes combined p-value based on the order statistic of p-values of probes (from site-specific analyses) mapped to a gene, assuming independent identical uniform distribution of p-values. We restricted our evaluation of enrichment to gene sets containing between 100 and 500 genes. The adjusted p-values of gene sets (enriched pathways) account for multiple hypothesis testing and the size of gene sets. We defer readers to (Subramanian et al., 2005) for details on calculation of adjusted p-values.
The ipDMR approach implemented in the Enmix (Version 1.25.1) R package (Xu et al., 2020) was used to identify differentially methylated regions (DMRs). The initial DMRs are identified as a region bound by a pair of adjacent probes across the methylome. These regions are filtered based on their sizes and combined
Validation analysis
We used the Illumina 450K array data of 295 BC tumor tissue samples available in GEO database (GSE72308) to validate site-specific analysis results. The samples were obtained from retrospectively selected frozen samples and prospective cohorts who were treated with adjuvant and neoadjuvant therapies at Jules Bordet Institute from 1995 to 2009 (Jeschke et al., 2017). IHC-based tumor subtypes available in the dataset were taken as the subtypes of the samples. To be consistent with site-specific analysis of TCGA data, we removed samples of HER2 subtype. OS endpoint was the only available outcome that was in common with survival outcomes available in TCGA data. Age and tumor subtype were the only available covariates in the data set. Tumor purity and cell type proportion were estimated using identical methods in the site-specific analysis. The beta-values of each probe were normalized across samples before conducting EWAS. Since menopausal status information was not available, Model C was not fitted. Model A and Model B were identical to the ones fitted using TCGA data except for the covariates such as race, stage, and menopausal status, which were not available in the GEO data set. EWAS results from each model were compared to the results from TCGA site-specific analyses. In particular, log hazards of the probes were compared to examine whether the directions of the association between beta-values to survival outcomes were similar between TCGA and GEO EWAS results.
Results
Site-specific results
The association between site-specific DNAm and each clinical outcome was assessed across methylome. Time to event was modeled using Cox proportional hazards (PH) models, stratified by tumor subtype, and adjusted for age, race, stage, menopausal status, DNAm-estimated tumor purity and cell proportions (8 cell types). Based on this model, one probe was associated with OS at genome-wide significance (p <
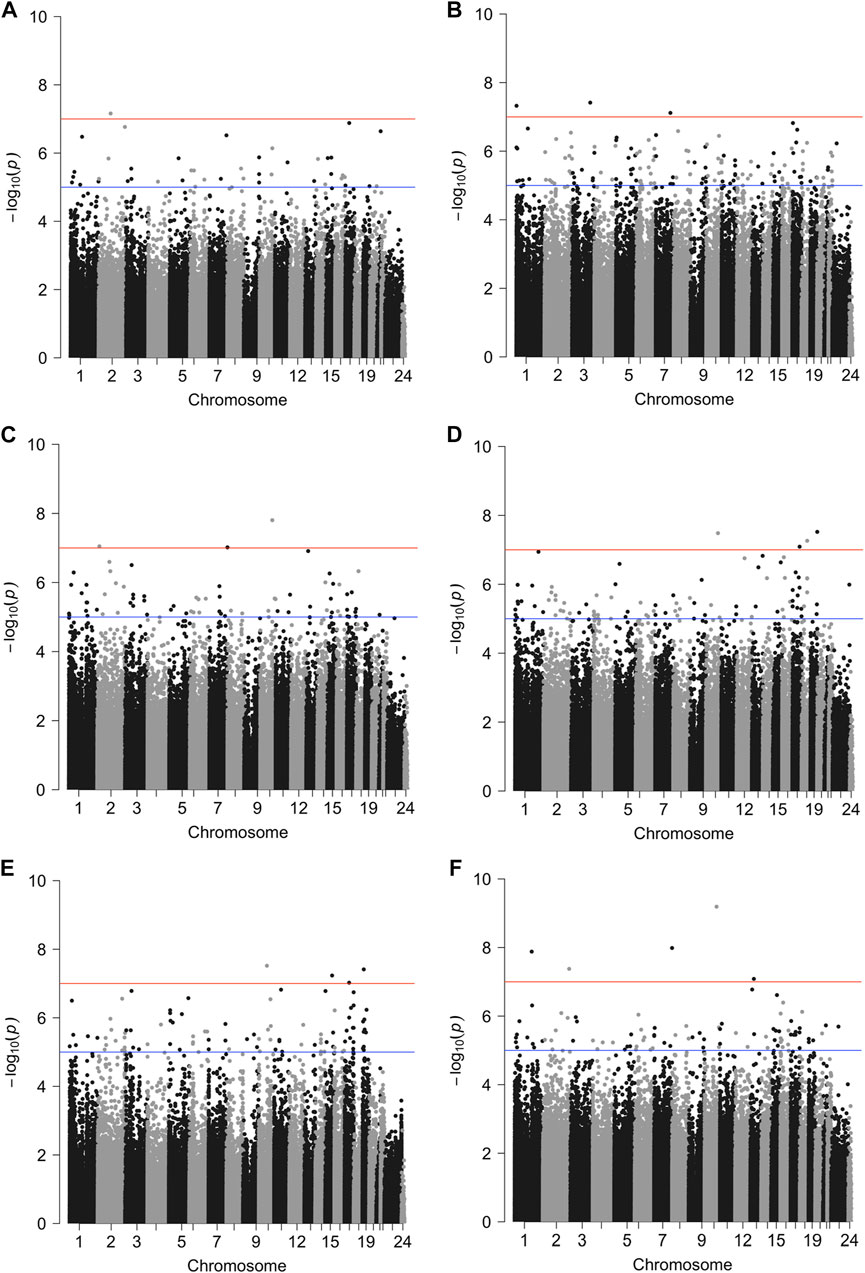
FIGURE 1. Manhattan plots of genome-wide associations between DNAm levels and survival outcomes. We employed Models A, B and C to investigate CpG probes associated with survival outcomes (overall survival and progression free interval) in a subtype or menopausal status specific manner. Model A tested the association of beta-values and the survival outcomes stratified by the tumor subtypes (no effect measure modifications); Model B tested the association between beta-values and the survival outcomes under the assumption that the association could be modified by tumor subtype; Model C tested the association between beta-values and the survival outcomes under the assumption that the association could depend on menopausal status (post-menopause vs. pre- and peri-menopause). All models adjusted for age, race, stage, menopausal status, tumor purity and cell type proportion. Here, we show Manhattan plots of genome-wide associations with at least one genome-wide significant CpG probe. The red line indicates a genome-wide significant level of
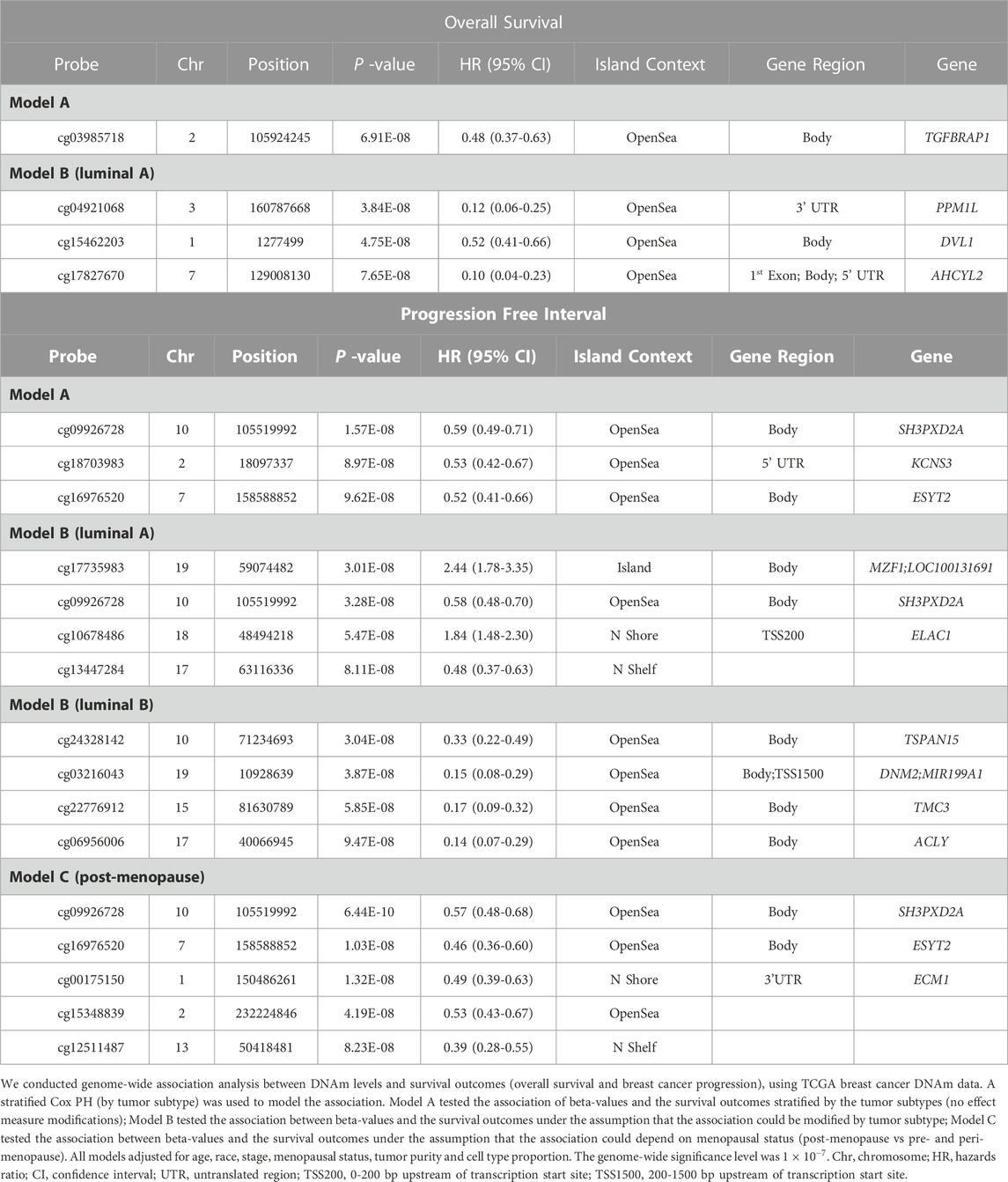
TABLE 2. Genome-wide significant CpG probes associated with survival outcomes specifically to tumor subtype or menopausal status of women.
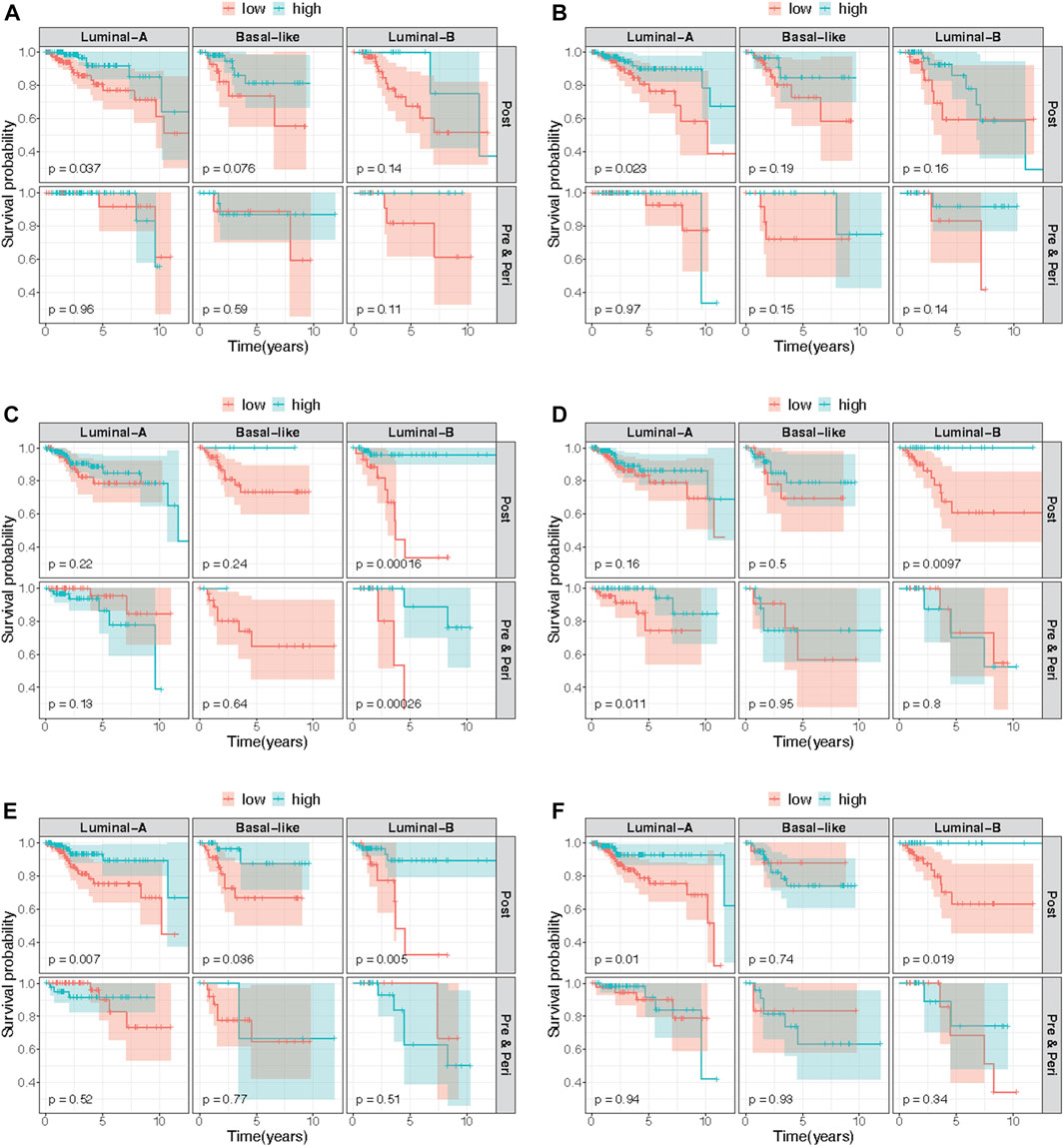
FIGURE 2. Kaplan-Meier curves comparing estimated survival probabilities between low and high methylation groups, stratified by both tumor subtype and menopausal status. We selected stratified Kaplan-Meir curves of top six probes based on p-values of stratified log-rank test (not shown) among the CpG probes that met genome-wide significance level of
Similar models were used to identify site-specific associations with PFI. A majority of genome-wide significant sites were found to be luminal A, luminal B and/or post-menopausal specific (Figure 1; Figure 2; Table 2, Supplementary Figure S1). There were 10 probes that were significantly associated with PFI only after accounting for effect measure modification by tumor subtype or menopausal status. Higher DNAm was associated with a reduced hazard of disease progression for all but two probes, cg17735983 and cg10678486, both of which were specific to the luminal A subtype (Table 2). While most of the probes were in open sea regions and gene bodies, cg17735983 was located in an CpG island, and cg10678486 was located within 200 bp of the transcription start site (TSS200) of ELAC1. Probe cg09926728 was associated with PFI overall, but this association was strongest among participants with the luminal A subtype and postmenopausal women (Supplementary Figure S5I). The estimated hazard ratios (HRs) for this probe were similar across different models ranging between 0.57–0.59. Similarly, higher methylation at cg16976520 was associated with a reduced hazard of progression overall, which was strongest among the post-menopausal women (Figure 2F; Supplementary Figure S5M). Among post-menopausal women, the rates of progression were higher among women with the luminal A and luminal B subtypes with lower methylation at cg16976520, relative to those with the basal-like subtype (Figure 2F).
Functional pathway analysis results
We evaluated gene ontology (GO) enrichment among the site-specific associations with BC outcomes, taking into account the length bias of a gene and the number of probes per gene (Supplementary Figures S2 and Supplementary Figure S3). For each endpoint, GO enrichment was assessed based on the overall model, as well as models incorporating effect modification by either subtype or menopausal status. The number of significantly (adjusted p-value
Differentially methylated region analysis
To identify DMRs associated with our outcomes, we applied an approach that integrates site-specific inference between proximal loci. Significant DMRs differed by tumor subtype and menopausal status (Table3; Table 4; Figure 3, Supplementary Table S1, Supplementary Figure S4). These included a DMR within the promoter of ELAC1 that was associated with PFI among the luminal A tumor samples, but not among the luminal B and basal-like samples (Figure 3A). In particular, this DMR was the most significant among all DMRs containing at least four probes, and included a genome-wide significant CpG probe, cg10678486, which was identified in the site-specific analysis to be associated with PFI for women with luminal A tumor subtype. We identified 271 luminal A specific DMRs that were associated with OS (Table 3). Only one out of three genome-wide significant luminal A subtype-specific probes associated with OS appeared within these significant DMRs. Similarly, only one of the three probes significantly associated with PFI was within one of the 42 DMRs associated with PFI (Table 3). These results indicated a majority of DMRs were not driven by one strong site-specific association, but several weaker associations in the same direction among proximal loci (Table 4). Several DMRs were not close to known genes. For example, an identified DMR specific to luminal A subtype did not reside near any known gene, but in a region indicated by chromatin state of promoter upstream TSS (Supplementary Table S1, Supplementary Figure S4F).
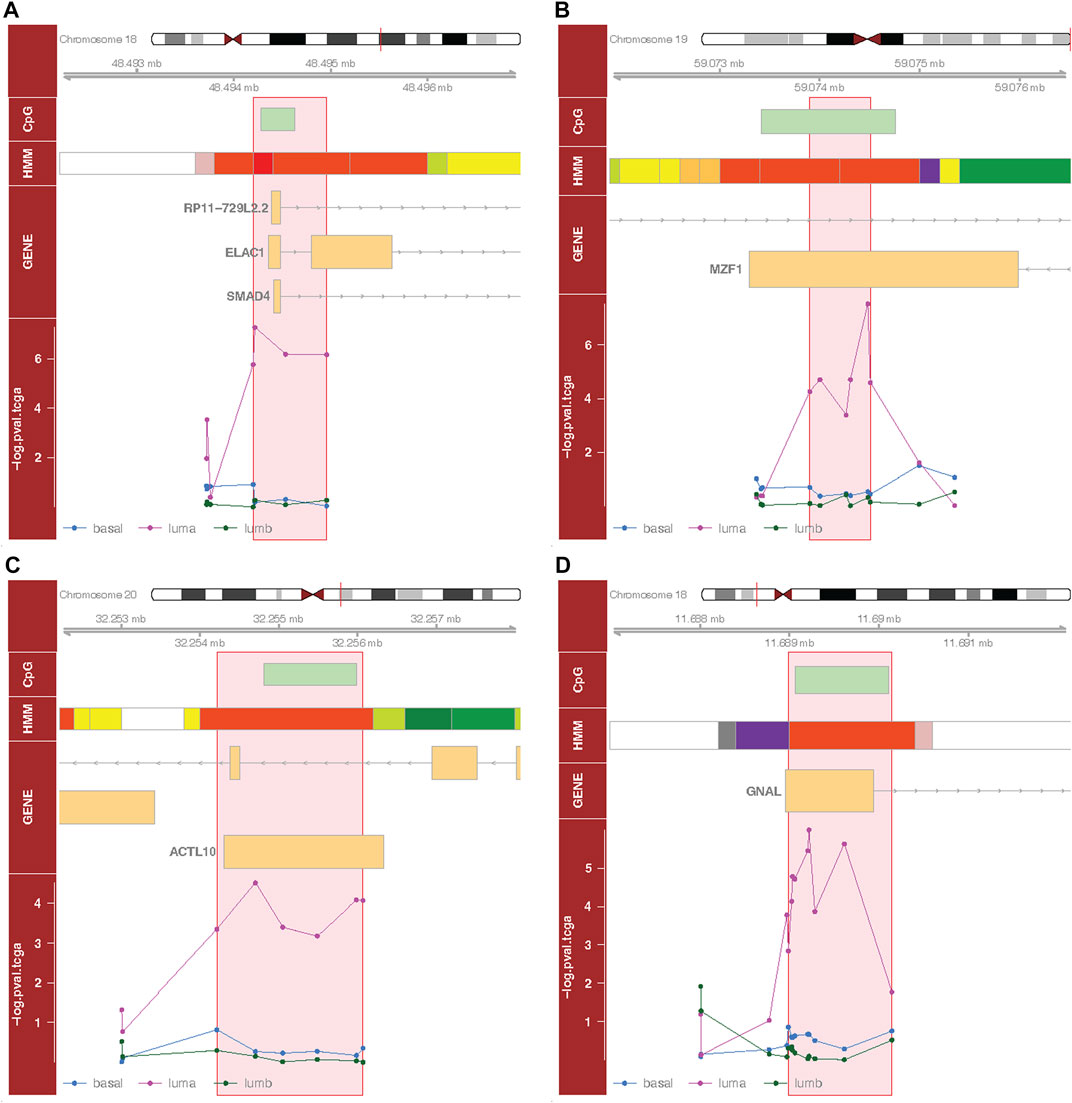
FIGURE 3. Selected differentially methylated regions (DMRs). Each subplot depicts a region in the genome around identified DMR (flanking 2000 bp). Here, we show the top four DMRs based on adjusted p-values (See Materials and Methods), containing at least four CpG probes. (A) Luminal A-specific DMR (chr18:48494200–48494958) associated with progression free interval; (B) luminal A-specific DMR (chr19:59073901–59074507) associated with progression free interval; (C) luminal A-specific DMR (chr20:32254215–32256071) associated with progression free interval; (D) luminal A-specific DMR (chr18:11688987–11690145) associated with overall survival. The first track shows the CpG island context; the second track shows the chromatin state; the third track shows the gene context (Ensembl); the fourth track shows the p-values of the probes (from the site-specific analyses) for each subtype. Red boxes represent DMRs. Vertical bars on the chromosome schematic locate plotted regions. Chromatin state color scheme: electric lime, transcribed 3′ preferential and enhancer (Enh) or transcribed 5′ preferential and Enh or transcribed and weak Enh; red, active transcription start site (TSS); orange red, promoter (Prom) upstream/downstream TSS; yellow, weak Enh or primary H3K27ac possible Enh; white, quiescent; orange, active Enh; pink, poised Prom; dark purple, bivalent Prom; light green, weak transcription; green, transcribed or strong transcription; gray, repressed polycomb.
Validation results
Validation of results of TCGA site-specific analyses was carried out on Illumina 450K array data profiled from BC tissue of 180 patients available in GEO database (GSE72308) (Jeschke et al., 2017). We were only able to analyze associations with OS since GEO dataset did not include any other common type of survival outcome. These validation models did not adjust for race and stage, or evaluate effect modification by menopausal status as these characteristics were missing from the GEO data. Tumor purity and cell proportion of samples were estimated and included in the models. The age of a majority of subjects was less than 60 years old in both TCGA and GEO. At alpha level of 0.05, there were no significant age differences between the two datasets (p-value = 0.148) based on binary categorization of the age variable. More than 50 percent of tumor subtypes of TCGA samples was luminal A, whereas the subtypes of GEO samples were relatively balanced across luminal A, luminal B and basal-like subtypes (Table 1). The median follow-up times for all-cause mortality were not significantly different (p-value = 0.789) between the two datasets at alpha level of 0.05. We compared the top 20 most significant (arranged by increasing order of p-values) associations with OS identified from TCGA site-specific analyses to those estimated among the GEO dataset. While the directions of associations were similar, most did not reach statistical significance, potentially due to the smaller sample size of the GEO dataset. Associations with similar magnitudes between the two datasets include the overall association with DNAm at cg05497253 (Figure 4A), as well as the luminal A subtype-specific associations at cg05860556 and cg13328771 (Figure 4B), and the basal-like-subtype specific associations at cg04706201 and cg14253517 (Figure 4D).
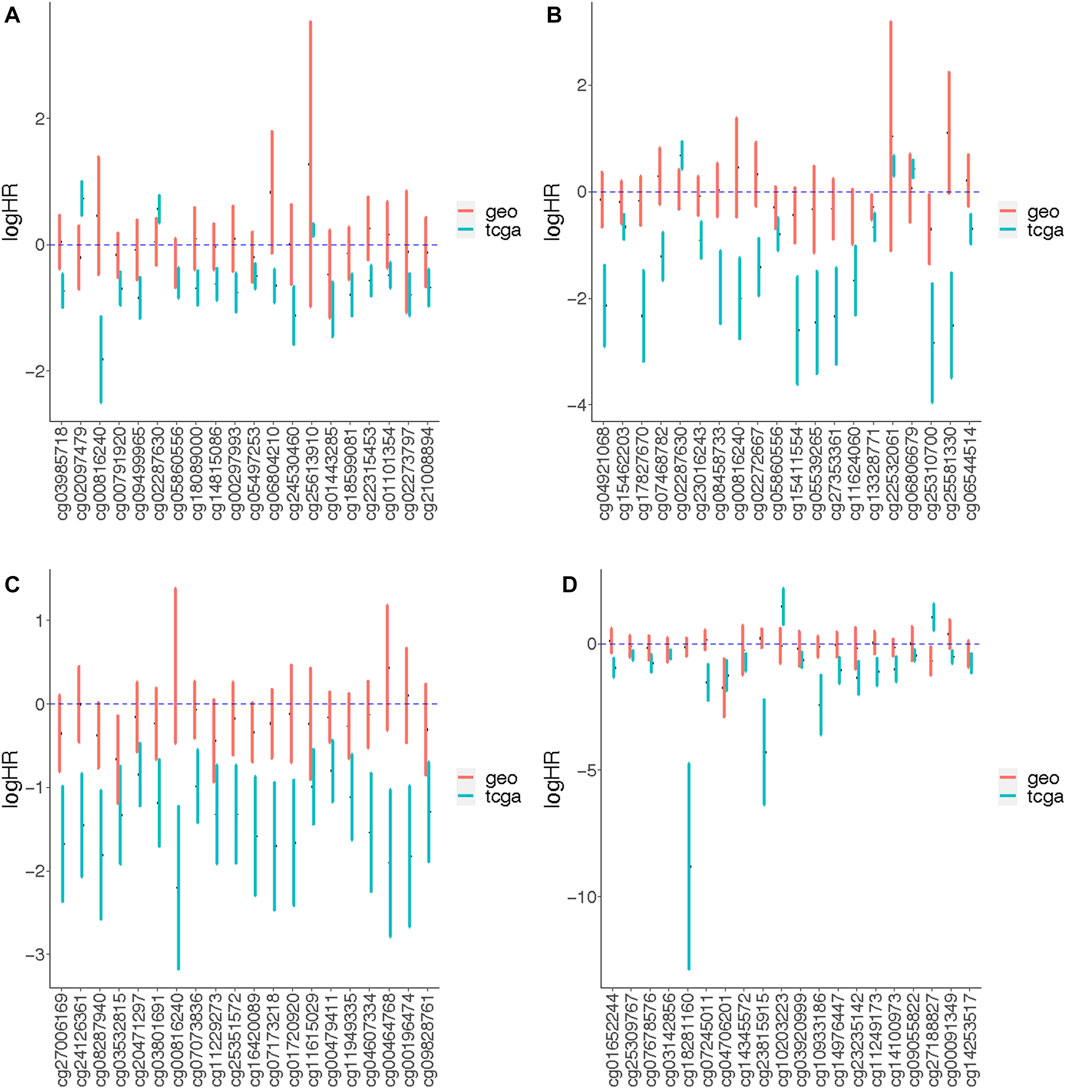
FIGURE 4. The effect measures of association between DNAm and overall survival outcomes quantified from genome-wide association analyses of TCGA and GEO data. The CpG probes labeled on the x-axis are top 20 significant probes from the TCGA site-specific analyses, which are arranged from left to right in an increasing order by p-values. We compare effect measures of association between DNAm and overall survival quantified from site-specific analyses of TCGA and GEO data using (A) Model A, (B) Model B (luminal A-specific), (C) Model B (luminal B-specific) and (D) Model B (basal-like-specific). Model A tested the association of beta-values and the survival outcomes stratified by the tumor subtypes (no effect measure modifications); Model B tested the association between beta-values and the survival outcomes under the assumption that the association could be modified by tumor subtype. The models for TCGA data adjusted for age, race, stage, menopausal status, tumor purity and cell type proportion. The models for GEO data adjusted for age, tumor purity and cell type proportion.
Further, we compared p-values for the 20 most significantly enriched GOs identified from the TCGA site-specific analysis results to the p-values of matching GOs identified from the GEO site-specific analysis results (Figure 5). Several GOs seemed to be consistent across datasets. Specifically, for the luminal A subtype-specific associations, GO:0031012 (extracellular matrix) and GO:0042383 (sarcolemma) showed consistent results between TCGA and GEO (Figure 5B). For the luminal B subtype-specific associations, GO:0007389 (pattern specification process), GO:0001216 (DNA-binding transcription activator activity), and GO:0001228 (DNA-binding transcription activator activity) showed consistent results between TCGA and GEO (Figure 5C). For the basal-like subtype-specific associations, GO:0007269 (neurotransmitter secretion) and GO:0099643 (signal release from synapse) showed consistent results across datasets (Figure 5D).
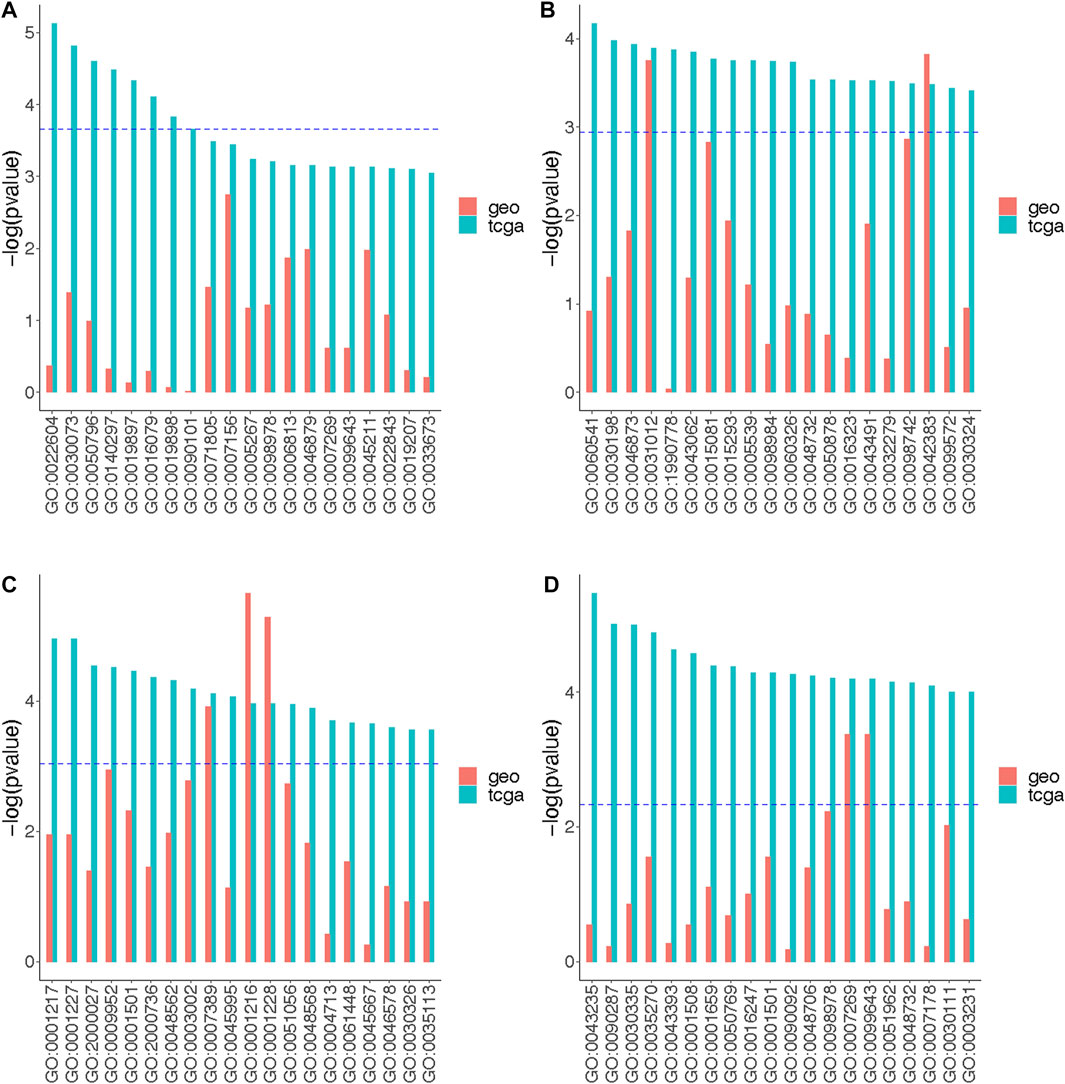
FIGURE 5. The strengths of the functional pathway enrichment by the association signals between DNAm and overall survival outcomes. The top 20 significantly enriched GO terms representing functional pathways are labeled on the x-axis. The GO terms are arranged from left to right in an increasing order by adjusted p-values obtained from the gene set enrichment analysis. The enriched pathways were identified using the strengths of the signals indicated by p-values obtained from the site-specific analyses of TCGA and GEO data using (A) Model A, (B) Model B (luminal A-specific), (C) Model B (luminal B-specific) and (D) Model B (basal-like-specific). Model A tested the association of beta-values and the survival outcomes stratified by the tumor subtypes (no effect measure modifications); Model B tested the association between beta-values and the survival outcomes under the assumption that the association could be modified by tumor subtype. The models for TCGA data adjusted for age, race, stage, menopausal status, tumor purity and cell type proportion. The models for GEO data adjusted for age, tumor purity and cell type proportion. The blue dotted lines indicate the maximum unadjusted enrichment p-value of GOs whose adjusted enrichment p-values were at most 0.05.
Discussion
In this study, we detected subtype- or menopausal-status-specific changes in DNAm associated with OS and PFI for BC across the genome. We also investigated functional pathways enriched among the site-specific associations with these BC survival outcomes. Several previous studies that aimed to identify shifts in DNAm associated with BC prognosis acknowledged these relationships may differ across tumor subtypes (Hao et al., 2017; de Almeida et al., 2019; Du et al., 2019; Liu et al., 2020; Tao et al., 2020). Unfortunately, these prior studies did not directly evaluate effect modification by subtype or menopausal status. Xiao et al. examined associations between subtype-specific DNAm and OS (Xiao et al., 2018). However, their analyses were limited to subjects with luminal BC and did not examine effect modification based on menopausal status and associations between DNAm and BC progression. Tumor subtype and menopausal status inform patient treatment, and are associated with different prognoses and treatment responses. In fact, alterations in DNAm can induce resistance to chemotherapy or hormone therapy for BC patients, and recent developments in epigenetic therapies combined with conventional therapies seem promising (Vietri et al., 2021; Schröder et al., 2022). Thus, studying the subtype- and menopausal-status-specific DNAm sites in association with BC prognosis could potentially improve the precision of BC prognoses and help to identify potential drug targets. To the best of our knowledge, our study is the first to report subtype- or menopausal-status-specific genome-wide significant probes that can potentially be utilized as prognostic BC biomarkers. These CpG probes have not been reported in the previous tissue-based BC studies that aimed to find DNAm biomarkers for BC prognosis prediction, indicating potential existence of lurking DNAm sites for BC prognosis that can be detected only after accounting for effect modification by subtype or menopausal status. This was illustrated in our site-specific results where we have found multiple CpG probes whose DNAm levels were significantly associated with BC progression or all-cause mortality, only in models that incorporated effect modification by subtype or menopausal status.
A study by de Almeida et al, (2019) examined genome-wide differential methylation between BC tissue and matched normal tissue using TCGA’s 450K array data. They reported that all of CpG loci associated with poor prognosis (with respect to overall survival) were hypermethylated. In the current study, two CpG loci were associated with increased risk of BC progression with higher methylation levels. In addition, they have found adjusting for ER status of samples rendered some of identified prognostic CpG probes no longer significantly associated with survival outcomes. This indicates an evidence of effect measure modification by subtype on methylation levels on survival outcomes, which consistent with the current study’s findings.
Some of the genes mapped to the significant probes identified in this study have previously been associated with BC initiation and progression. Higher DNAm at cg00175150, a probe within the 3’ untranslated region (UTR) of ECM1, was associated with a reduced hazard of PFI among post-menopausal women. The ECM1 protein is secreted by HER2-overexpressing cancer cells, leading to positive downstream effects on tumor migration and invasion, facilitating tumor progression (Steinhaeuser et al., 2020). Among women with the luminal A subtype, higher DNAm at cg15462203, a probe within the gene body of disheveled segment polarity protein 1 (DVL1), was associated with a reduced hazard of all-cause mortality. The DVL1 gene plays a role in activating Wnt transcriptional pathways (Lee et al., 2008; Paclíková et al., 2017), which regulate cellular functions such as cell migration, proliferation and stem cell renewal. DVL1 has been shown to be overexpressed in primary breast tumors compared to non-cancerous breast tissues, and DVL1 protein has been found to be more present in the cytoplasm of cancer cells compared to that of normal epithelial cells in breast tumors (Nagahata et al., 2003). Also, DVL1 is involved in regulation of CYP9A1 transcripts in a promoter specific and cell-type specific manner. Encoded by CYP9A1, aromatase enzyme converts androgen into estrogen; hence, aromatase enzyme is considered a main driver of hormone-dependent breast tumors (Castro-Piedras et al., 2018). Knockout of DVL1 in hormone receptor positive BC cell lines increased the total aromatase transcript levels (Castro-Piedras et al., 2018). Moreover, in the same type of cell lines, the knockout of DVL1 showed a trend of increased estradiol levels compared to non-target controls, although it was not statistically significant. These findings suggest the tumor suppressive role of DVL1 by reducing estrogen production via regulation of CYP9A1 for hormone receptor positive BC cells. Higher DNAm at cg03216043, a probe within the gene body of Dynamin 2 (DNM2), was found to decrease risk of BC progression among luminal B tumors. DNM2 plays a role in driving cell migration and invasion in cancer cells (Eppinga et al., 2012; Chernikova et al., 2018). Knockdown of DNM2 impairs DNA repair mechanisms of tumor cells in mice (Wang et al., 2017). In retrospective analysis, lower expression of DNM2 was associated with favorable response to chemotherapy for hormonal receptor negative and triple-negative BC patients. In our study, cg06956006 was mapped to the ACLY gene, and was related to a lower risk of BC progression given higher levels of methylation. Upregulation of ACLY gene, which plays an important role in synthesis of fatty acids in cancer proliferation, is associated with BC and its recurrence (Yancy et al., 2007; Wang et al., 2017; Chen et al., 2020). The hazard of BC progression was greater among patients with higher DNAm at cg17735983, a probe mapping to MZF1. MZF1 is involved in the signaling pathways of HER2+ BC and implicated in development of more aggressive BC (Brix et al., 2020).
We detected several enriched GOs among our site-specific associations with OS and PFI, which differed by tumor subtype and menopause status. Of note, a gene set associated with GO:0050796, tied to insulin secretion, included KCNS3 gene, which was found in our site-specific analysis and mapped by cg18703983. This particular probe was significantly associated with PFI, suggesting that the hypermethylation of this probe is associated with BC prognosis, in part via the regulation of insulin secretion.
Our DMR analysis identified several regional changes in DNAm that were associated with different subtype or menopausal status for both OS and PFI endpoints. The top four significant DMRs intersected with ELAC1, MZF1, NECAB3, and GNAL, respectively. Both ELAC1 and MZF1 were associated with PFI among the luminal A tumor samples in site-specific analyses. ELAC1 and GNAL have not been reported to be linked with BC. However, NECAB3 was identified to be tumorigenic by promoting normoxic glycolysis in non-breast cancer cell lines (Nakaoka et al., 2016).
We note several limitations of our study. Our validation analysis was limited to 180 individuals, for whom key characteristics including race, tumor stage and menopausal status were missing. Thus, we were unable to adjust for these characteristics, which may have contributed to the lack of significant associations with OS. Moreover, while BC samples of TCGA subjects were collected before any adjuvant and neoadjuvant therapies, BC samples from the validation dataset were collected after patients have undergone the therapies, possibly leading to perturbed DNAm levels. Non-etheless, we were able to identify probes with consistent associations between two the two datasets based on magnitudes and directions of estimated coefficients. Moreover, we found several cellular functions associated with OS for luminal A, luminal B, and basal-like subtypes across the two studies. Another limitation is that our study included relatively fewer luminal B or basal-like tumors, and a majority of patients was post-menopausal. We might be able detect more genome-wide significant associations specific to luminal B and basal-like subtypes and pre- and peri-menopausal women with larger sample size. Due to these smaller strata, we were not able to simultaneously evaluate effect modification by tumor subtype and menopausal status. Since pre-menopausal patients have different BC prognoses compared to post-menopausal patients (Goldhirsch et al., 2011; Keegan et al., 2012; Lian et al., 2017), stratified analysis by both tumor subtype and menopause status could improve the precision of BC prognosis. We also did not have information on patient treatment status or types of treatments. These can be an important factor affecting survival outcomes of patients. Lastly, different clinical sites where BC patients were treated and recruited could potentially affect the survival outcomes, but this information was not available to us.
Despite those limitations, our study had several strengths. These include adjustment for estimated tumor purity and cell proportions of each sample, which may confound the associations. In addition, we fitted models stratified by subtypes and menopause status, yielding probes associated with two survival outcomes, OS and PFI, in subtype- or menopausal-status-specific manner.
Overall, our study found that specific patterns of DNAm were associated with BC prognosis, namely, OS and PFI, and that these associations differed by molecular subtype or menopausal status. We were also able to identify genomic regions and functional pathways that were specific to molecular subtype or menopausal status. These findings warrant additional replication studies in larger, independent datasets as well as further investigations of the functional implications of these patterns of DNAm. Our detected genome-wide-associated-CpG loci could improve prognosis prediction for BC patients and contribute to more tailored therapeutic regimens.
Data availability statement
Publicly available datasets were analyzed in this study. This data can be found here: TCGA DNA methylation data (https://portal.gdc.cancer.gov), TCGA survival data for breast cancer (https://gdc.cancer.gov/about-data/publications/PanCan-Clinical-2018), DNA methylation data for validation analysis (https://www.ncbi.nlm.nih.gov/geo/query/acc.cgi?accandequals; GSE72308), Cell-type-specific methylation matrix for TCGA breast cancer (https://genboree.org/theCommons/projects/edec/documents).
Author contributions
Conceptualized the study: SJ, AB. Performed the data QC: AB, DK. Performed the statistical analysis: DK. Participated in the study coordination and interpreted the data: DK, AB, SJ, HZ. Oversaw with inputs on analysis results: AB, SJ, HZ. Provided statistical guidance throughout the study: AB. Secured funding: SJ. All participated in the paper writing and editing. All authors have read and approved the submission of the manuscript.
Funding
SJ was supported by the National Institute of Nursing Research of the National Institutes of Health under Award Number K01NR017852. HZ was supported by the grants from National Institutes of Health (R35GM141798, R01HG006139) and National Science Foundation (DMS-2054253, IIS-2205441). DK was supported by the National Institutes of Health under T32-HG002536 training grant. AB was supported by the National Cancer Institute of the National Institutes of Health under Award Number K07CA225856.
Conflict of interest
The authors declare that the research was conducted in the absence of any commercial or financial relationships that could be construed as a potential conflict of interest.
Publisher’s note
All claims expressed in this article are solely those of the authors and do not necessarily represent those of their affiliated organizations, or those of the publisher, the editors and the reviewers. Any product that may be evaluated in this article, or claim that may be made by its manufacturer, is not guaranteed or endorsed by the publisher.
Supplementary material
The Supplementary Material for this article can be found online at: https://www.frontiersin.org/articles/10.3389/fgene.2023.1133443/full#supplementary-material
References
Aryee, M. J., Jaffe, A. E., Corrada-Bravo, H., Ladd-Acosta, C., Feinberg, A. P., Hansen, K. D., et al. (2014). Minfi: a flexible and comprehensive bioconductor package for the analysis of Infinium DNA methylation microarrays. Bioinformatics 30, 1363–1369. doi:10.1093/bioinformatics/btu049
Brix, D. M., Bundgaard Clemmensen, K. K., and Kallunki, T. (2020). Zinc finger transcription factor MZF1—a specific regulator of cancer invasion. Cells 9, 223. doi:10.3390/cells9010223
Byler, S., Goldgar, S., Heerboth, S., Leary, M., Housman, G., Moulton, K., et al. (2014). Genetic and epigenetic aspects of breast cancer progression and therapy. Anticancer Res. 34, 1071–1077. Available at: https://ar.iiarjournals.org/content/34/3/1071 (Accessed March 17, 2021).
Castro-Piedras, I., Sharma, M., den Bakker, M., Molehin, D., Martinez, E. G., Vartak, D., et al. (2018). DVL1 and DVL3 differentially localize to CYP19A1 promoters and regulate aromatase mRNA in breast cancer cells. Oncotarget 9, 35639–35654. doi:10.18632/oncotarget.26257
Chen, Y., Li, K., Gong, D., Zhang, J., Li, Q., Zhao, G., et al. (2020). ACLY: A biomarker of recurrence in breast cancer. Pathol. Res. Pract. 216, 153076. doi:10.1016/j.prp.2020.153076
Chernikova, S. B., Nguyen, R. B., Truong, J. T., Mello, S. S., Stafford, J. H., Hay, M. P., et al. (2018). Dynamin impacts homology-directed repair and breast cancer response to chemotherapy. J. Clin. Invest. 128, 5307–5321. doi:10.1172/JCI87191
de Almeida, B. P., Apolónio, J. D., Binnie, A., and Castelo-Branco, P. (2019). Roadmap of DNA methylation in breast cancer identifies novel prognostic biomarkers. BMC Cancer 19, 219. doi:10.1186/s12885-019-5403-0
Du, T., Liu, B., Wang, Z., Wan, X., and Wu, Y. (2019). CpG methylation signature predicts prognosis in breast cancer. Breast Cancer Res. Treat. 178, 565–572. doi:10.1007/s10549-019-05417-3
Dumont, N., Wilson, M. B., Crawford, Y. G., Reynolds, P. A., Sigaroudinia, M., and Tlsty, T. D. (2008). Sustained induction of epithelial to mesenchymal transition activates DNA methylation of genes silenced in basal-like breast cancers. PNAS 105, 14867–14872. doi:10.1073/pnas.0807146105
Elsheikh, S. E., Green, A. R., Rakha, E. A., Powe, D. G., Ahmed, R. A., Collins, H. M., et al. (2009). Global histone modifications in breast cancer correlate with tumor phenotypes, prognostic factors, and patient outcome. Cancer Res. 69, 3802–3809. doi:10.1158/0008-5472.CAN-08-3907
Eppinga, R. D., Krueger, E. W., Weller, S. G., Zhang, L., Cao, H., and McNiven, M. A. (2012). Increased expression of the large GTPase dynamin 2 potentiates metastatic migration and invasion of pancreatic ductal carcinoma. Oncogene 31, 1228–1241. doi:10.1038/onc.2011.329
Goldhirsch, A., Wood, W. C., Coates, A. S., Gelber, R. D., Thürlimann, B., Senn, H.-J., et al. (2011). Strategies for subtypes—dealing with the diversity of breast cancer: highlights of the St gallen international expert consensus on the primary therapy of early breast cancer 2011. Ann. Oncol. 22, 1736–1747. doi:10.1093/annonc/mdr304
Hao, X., Luo, H., Krawczyk, M., Wei, W., Wang, W., Wang, J., et al. (2017). DNA methylation markers for diagnosis and prognosis of common cancers. Proc. Natl. Acad. Sci. U. S. A. 114, 7414–7419. doi:10.1073/pnas.1703577114
Houseman, E. A., Molitor, J., and Marsit, C. J. (2014). Reference-free cell mixture adjustments in analysis of DNA methylation data. Bioinformatics 30, 1431–1439. doi:10.1093/bioinformatics/btu029
Jeschke, J., Bizet, M., Desmedt, C., Calonne, E., Dedeurwaerder, S., Garaud, S., et al. (2017). DNA methylation–based immune response signature improves patient diagnosis in multiple cancers. J. Clin. Invest. 127, 3090–3102. doi:10.1172/JCI91095
Jones, P. A., Issa, J.-P. J., and Baylin, S. (2016). Targeting the cancer epigenome for therapy. Nat. Rev. Genet. 17, 630–641. doi:10.1038/nrg.2016.93
Keegan, T. H., DeRouen, M. C., Press, D. J., Kurian, A. W., and Clarke, C. A. (2012). Occurrence of breast cancer subtypes in adolescent and young adult women. Breast Cancer Res. 14, R55. doi:10.1186/bcr3156
Koboldt, D. C., Fulton, R. S., McLellan, M. D., Schmidt, H., Kalicki-Veizer, J., McMichael, J. F., et al. (2012). Comprehensive molecular portraits of human breast tumours. Nature 490, 61–70. doi:10.1038/nature11412
Lee, Y.-N., Gao, Y., and Wang, H. (2008). Differential mediation of the Wnt canonical pathway by mammalian Dishevelleds-1, -2, and -3. Cell. Signal. 20, 443–452. doi:10.1016/j.cellsig.2007.11.005
Lian, W., Fu, F., Lin, Y., Lu, M., Chen, B., Yang, P., et al. (2017). The impact of young age for prognosis by subtype in women with early breast cancer. Sci. Rep. 7, 11625. doi:10.1038/s41598-017-10414-x
Liu, J., Lichtenberg, T., Hoadley, K. A., Poisson, L. M., Lazar, A. J., Cherniack, A. D., et al. (2018). An integrated TCGA pan-cancer clinical data resource to drive high-quality survival outcome analytics. Cell 173, 400–416.e11. doi:10.1016/j.cell.2018.02.052
Liu, X.-P., Hou, J., Chen, C., Guan, L., Hu, H.-K., and Li, S. (2020). A DNA methylation-based panel for the prognosis and dagnosis of patients with breast cancer and its mechanisms. Front. Mol. Biosci. 7, 118. doi:10.3389/fmolb.2020.00118
Nagahata, T., Shimada, T., Harada, A., Nagai, H., Onda, M., Yokoyama, S., et al. (2003). Amplification, up-regulation and over-expression of DVL-1, the human counterpart of the Drosophila disheveled gene, in primary breast cancers. Cancer Sci. 94, 515–518. doi:10.1111/j.1349-7006.2003.tb01475.x
Nakaoka, H. J., Hara, T., Yoshino, S., Kanamori, A., Matsui, Y., Shimamura, T., et al. (2016). NECAB3 promotes activation of hypoxia-inducible factor-1 during normoxia and enhances tumourigenicity of cancer cells. Sci. Rep. 6, 22784. doi:10.1038/srep22784
Ohnstad, H. O., Borgen, E., Falk, R. S., Lien, T. G., Aaserud, M., Sveli, M. A. T., et al. (2017). Prognostic value of PAM50 and risk of recurrence score in patients with early-stage breast cancer with long-term follow-up. Breast Cancer Res. 19, 120. doi:10.1186/s13058-017-0911-9
Onuchic, V., Hartmaier, R. J., Boone, D. N., Samuels, M. L., Patel, R. Y., White, W. M., et al. (2016). Epigenomic deconvolution of breast tumors reveals metabolic coupling between constituent cell types. Cell Rep. 17, 2075–2086. doi:10.1016/j.celrep.2016.10.057
Paclíková, P., Bernatík, O., Radaszkiewicz, T. W., and Bryja, V. (2017). The N-terminal part of the dishevelled DEP domain is required for wnt/β-catenin signaling in mammalian cells. Mol. Cell. Biol. 37, 001455–e217. doi:10.1128/MCB.00145-17
Parker, J. S., Mullins, M., Cheang, M. C. U., Leung, S., Voduc, D., Vickery, T., et al. (2009). Supervised risk predictor of breast cancer based on intrinsic subtypes. JCO 27, 1160–1167. doi:10.1200/JCO.2008.18.1370
Qin, Y., Feng, H., Chen, M., Wu, H., and Zheng, X. (2018). InfiniumPurify: An R package for estimating and accounting for tumor purity in cancer methylation research. Genes Dis. 5, 43–45. doi:10.1016/j.gendis.2018.02.003
Ren, X., and Kuan, P. F. (2019). methylGSA: a Bioconductor package and Shiny app for DNA methylation data length bias adjustment in gene set testing. Bioinformatics 35, 1958–1959. doi:10.1093/bioinformatics/bty892
Schröder, R., Illert, A.-L., Erbes, T., Flotho, C., Lübbert, M., and Duque-Afonso, J. (2022). The epigenetics of breast cancer – opportunities for diagnostics, risk stratification and therapy. Epigenetics 17, 612–624. doi:10.1080/15592294.2021.1940644
Steinhaeuser, S. S., Morera, E., Budkova, Z., Schepsky, A., Wang, Q., Rolfsson, O., et al. (2020). ECM1 secreted by HER2-overexpressing breast cancer cells promotes formation of a vascular niche accelerating cancer cell migration and invasion. Lab. Investig. 100, 928–944. doi:10.1038/s41374-020-0415-6
Stone, A., Zotenko, E., Locke, W. J., Korbie, D., Millar, E. K. A., Pidsley, R., et al. (2015). DNA methylation of oestrogen-regulated enhancers defines endocrine sensitivity in breast cancer. Nat. Commun. 6, 7758. doi:10.1038/ncomms8758
Subramanian, A., Tamayo, P., Mootha, V. K., Mukherjee, S., Ebert, B. L., Gillette, M. A., et al. (2005). Gene set enrichment analysis: A knowledge-based approach for interpreting genome-wide expression profiles. PNAS 102, 15545–15550. doi:10.1073/pnas.0506580102
Sung, H., Ferlay, J., Siegel, R. L., Laversanne, M., Soerjomataram, I., Jemal, A., et al. (2021). Global cancer statistics 2020: GLOBOCAN estimates of incidence and mortality worldwide for 36 cancers in 185 countries. CA Cancer J. Clin. 71, 209–249. doi:10.3322/caac.21660
Tao, C., Luo, R., Song, J., Zhang, W., and Ran, L. (2020). A seven-DNA methylation signature as a novel prognostic biomarker in breast cancer. J. Cell. Biochem. 121, 2385–2393. doi:10.1002/jcb.29461
Tibshirani, R., Hastie, T., Narasimhan, B., and Chu, G. (2002). Diagnosis of multiple cancer types by shrunken centroids of gene expression. PNAS 99, 6567–6572. doi:10.1073/pnas.082099299
Vietri, M. T., D’Elia, G., Benincasa, G., Ferraro, G., Caliendo, G., Nicoletti, G. F., et al. (2021). DNA methylation and breast cancer: A way forward (Review). Int. J. Oncol. 59, 98–12. doi:10.3892/ijo.2021.5278
Wang, D., Yin, L., Wei, J., Yang, Z., and Jiang, G. (2017). ATP citrate lyase is increased in human breast cancer, depletion of which promotes apoptosis. Tumor Biol. 39, 1010428317698338. doi:10.1177/1010428317698338
Xiao, B., Chen, L., Ke, Y., Hang, J., Cao, L., Zhang, R., et al. (2018). Identification of methylation sites and signature genes with prognostic value for luminal breast cancer. BMC Cancer 18, 405. doi:10.1186/s12885-018-4314-9
Xu, Z., Xie, C., Taylor, J. A., and Niu, L. (2020). ipDMR: identification of differentially methylated regions with interval P-values. Bioinformatics 37, 711–713. doi:10.1093/bioinformatics/btaa732
Yancy, H. F., Mason, J. A., Peters, S., Thompson, C. E., Littleton, G. K., Jett, M., et al. (2007). Metastatic progression and gene expression between breast cancer cell lines from African American and Caucasian women. J. Carcinog. 6, 8. doi:10.1186/1477-3163-6-8
Keywords: DNA methylation signatures, breast cancer, subtypes, menopause, survival and prognosis
Citation: Kim DH, Binder AM, Zhou H and Jung SY (2023) DNA methylation patterns associated with breast cancer prognosis that are specific to tumor subtype and menopausal status. Front. Genet. 14:1133443. doi: 10.3389/fgene.2023.1133443
Received: 29 December 2022; Accepted: 20 February 2023;
Published: 02 March 2023.
Edited by:
Miljana Tanic, Institute of Oncology and Radiology of Serbia, SerbiaReviewed by:
Barbara Ruszkowska-Ciastek, Nicolaus Copernicus University in Toruń, PolandMaria Teresa Vietri, Seconda Università Degli Studi Di Napoli, Italy
Copyright © 2023 Kim, Binder, Zhou and Jung. This is an open-access article distributed under the terms of the Creative Commons Attribution License (CC BY). The use, distribution or reproduction in other forums is permitted, provided the original author(s) and the copyright owner(s) are credited and that the original publication in this journal is cited, in accordance with accepted academic practice. No use, distribution or reproduction is permitted which does not comply with these terms.
*Correspondence: Alexandra M. Binder, YW1iaW5kZXJAaGF3YWlpLmVkdQ==