- 1Department of Animal Science, School of Agricultural and Veterinarian Sciences, São Paulo State University (Unesp), Jaboticabal, SP, Brazil
- 2Department of Animal Science, College of Agriculture and Natural Resources, Michigan State University, East Lansing, MI, United States
- 3Centre for Genetic Improvement of Livestock, Department of Animal Biosciences, University of Guelph, Guelph, ON, Canada
- 4Department of Animal Science, Viçosa Federal University, Viçosa, Brazil
- 5GenSys Associated Consultants, Porto Alegre, Brazil
- 6Researcher at National Council for Scientific and Technological Development (CNPq), Brasília, Brazil
Beef cattle affected by feet and legs malformations (FLM) cannot perform their productive and reproductive functions satisfactorily, resulting in significant economic losses. Accelerated weight gain in young animals due to increased fat deposition can lead to ligaments, tendon and joint strain and promote gene expression patterns that lead to changes in the normal architecture of the feet and legs. The possible correlated response in the FLM due to yearling weight (YW) selection suggest that this second trait could be used as an indirect selection criterion. Therefore, FLM breeding values and the genetic correlation between FLM and yearling weight (YW) were estimated for 295,031 Nellore animals by fitting a linear-threshold model in a Bayesian approach. A genome-wide association study was performed to identify genomic windows and positional candidate genes associated with FLM. The effects of single nucleotide polymorphisms (SNPs) on FLM phenotypes (affected or unaffected) were estimated using the weighted single-step genomic BLUP method, based on genotypes of 12,537 animals for 461,057 SNPs. Twelve non-overlapping windows of 20 adjacent SNPs explaining more than 1% of the additive genetic variance were selected for candidate gene annotation. Functional and gene prioritization analysis of candidate genes identified six genes (ATG7, EXT1, ITGA1, PPARD, SCUBE3, and SHOX) that may play a role in FLM expression due to their known role in skeletal muscle development, aberrant bone growth, lipid metabolism, intramuscular fat deposition and skeletogenesis. Identifying genes linked to foot and leg malformations enables selective breeding for healthier herds by reducing the occurrence of these conditions. Genetic markers can be used to develop tests that identify carriers of these mutations, assisting breeders in making informed breeding decisions to minimize the incidence of malformations in future generations, resulting in greater productivity and animal welfare.
1 Introduction
Brazil is the largest exporter of beef in the world in millions of tons (14.4% of the international market) and ranks third in value (US$ 7 billion) (Aragão and Contini, 2021). The Nelore is the main breed (80%) of the national herd and the Special Certificate of Identification and Production (CEIP) (MAPA, 2006) allows 20%–30% of the best bulls of each year to be certified as genetically superior. This guarantees a differentiated market value for the animals and their products as well as tax benefits when marketing them. CEIP is also a requirement to register animals as semen donors in the Breed Registration Service. However, animals of high genetic value and CEIP candidates are disqualified and slaughtered when affected by some morphological disorder, even though they have great genetic value for other traits of economic importance (e.g., reproductive traits, growth, and carcass quality). This leads to a decrease in efficiency in meat production systems due to the deficit of superior bulls from breeding programs to carry out natural mating in commercial herds (Rosa et al., 2021).
Animals affected by feet and legs malformations cannot perform their productive and reproductive functions satisfactorily, resulting in significant economic losses. The term “limb deformities” usually refers to defects in the legs and feet caused by the most varied etiological agents in different species of production animals (Rahe et al., 2022; Schwertz et al., 2023). Here we adopted the term feet and legs malformation (FLM) as a binary functional trait (having or not having defect) previously reported in the literature (Vargas et al., 2017; 2018), with a heritability of 0.18 (SE = 0.04) and an incidence of approximately 5% in Nellore cattle (Vargas et al., 2017). Efforts to reduce the incidence of FLM in Nellore cattle must begin by studying the degree of genetic variability in the population and possible genetic associations with other traits in the selection process. The genetic correlation between FLM and yearling weight (YW) (Vargas et al., 2017) suggests the possibility of using the second trait as an indirect selection criterion but needs to be recalculated due to the increased number of records available for FLM in this Nellore population.
Identification of significant regions in the bovine genome and candidate genes associated with FLM can also help to understand the biology behind the phenotype. In this way, Genome-wide Association Studies (GWAS), using phenotype, genotype, and pedigree data through the weighted single step genomic best linear unbiased predictor (WssGBLUP) method (Legarra et al., 2009) enables the identification of genomic regions that may be associated with the trait of interest. In a functional enrichment analysis, such as the one performed by Vargas et al. (2018), SNP windows that explain the highest proportion of additive variance are investigated in a database such as the Genome Data Viewer of the National Center for Biotechnology Information (NCBI, 2022) looking for genes located in these genomic regions. The presence of adjacent window genes of up to 1 Mb upstream and downstream of the analysed windows is also evaluated, as their effect can be captured by adjacent SNPs due to linkage disequilibrium (LD). Then articles published in indexed scientific journals are considered to support with biological evidence the potential role of genes located in these windows on the trait. However, the identification of functional candidate genes also can be performed using a systems biology approach, where genes shared between traits and studies are evaluated by a guilt by association gene prioritization (GUILDify and ToppGene software) in order to identify the best functional candidates (Fonseca et al., 2018).
This work aims to better understand the molecular mechanisms underlying FLM and provide subsidies that help to reduce the incidence of this problem in breeding programs for Nellore cattle, contributing to improve their production and reproductive efficiency. Vargas et al. (2017) defined the FLM genetic basis and Vargas et al. (2018) associated FLM with SNPs and performed a gene enrichment analysis. These previous analyses were updated using now a new genomic reference map (ARS-UCD1.2, Hu et al., 2022) and a larger dataset (295,031 phenotypes and 12,537 genotypes), in addition to apply a new methodology for the functional enrichment analysis of positional candidate genes (Fonseca et al., 2018). The objectives of this work were: 1) to re-estimate the genetic parameters of FLM, including genetic correlation between FLM and yearling weight (YW); 2) estimate genetic trends for these traits in Nellore cattle; 3) to find windows of adjacent SNPs significantly associated with FLM using the WssGBLUP methodology, and 4) to identify candidate genes supported by functional evidence with a potential role in the incidence of FLM.
2 Materials and methods
Approval from the Welfare and Animal Use Committee was not required for this study because the data was obtained from an existing database of phenotypic and genotypic records from Nellore cattle. Previous studies using these data also did not need this approval.
2.1 Population structure and phenotypic data
A final dataset of 295,031 Nellore cattle from the Nellore Alliance (GenSys, 2019), all with phenotypic information, collected between 2001 and 2017 was used in this study. Feet and legs malformation were evaluated by trained technicians which assigned binary scores (1 for affected animals and 0 for unaffected) to the overall structure of feet and legs at yearling (Vargas et al., 2018). Contemporary groups (CG) were defined considering the effects of herd, year and season of birth, sex, management group at weaning and yearling, date of measurement at yearling. Only data from CG with more than 10 records, variability in the trait and at least 10 genetic links to other CG were kept for the analyses. Connectedness among CG was checked using AMC software (Roso and Schenkel, 2006) with default parameters. As a result of the use of lots of multiple sires in the breeding season on part of the farms that make up the database, 31% (90,500) of the animals had the father information as unknown.
2.2 Genetic parameter estimates
After checking consistency and editing data, estimates of variance components and estimated breeding values (EBV) were obtained for each animal by Bayesian inference using THRGIBBS1F90 software (Misztal et al., 2014). To evaluate a possible correlated selection response of YW in FLM, a linear-threshold animal model was fitted to the two-trait analysis. This statistical model was also used by Vargas et al. (2017) and can be written in matrix notation as:
where
The relationship between the score for the
in which:
2.3 Genotyping and quality control
Genotyping was completed from 6,624 animals using the Illumina BovineHD Genotyping BeadChip (HD; Illumina, Inc., San Diego, CA, United States), which included 612,174 SNP markers, and 28,155 animals genotyped with medium density panels (20–90 k SNP). All animals belong to the Nellore Alliance population (GenSys, 2019) and had their own performance record and/or progeny evaluated for FLM. The FImpute v3 (Sargolzaei et al., 2014) software was used for genotype imputation from the lower density panels to the HD SNP chip and marker coordinates were mapped according to the genomic positions provided by new bovine genome assembly ARS-UCD1.2 (Hu et al., 2022).
Quality control (QC) of the genotypic and pedigree datasets was performed using QCF90 software (Masuda et al., 2019) and the following criteria were used for the exclusion of SNP markers: p-value < 10–5 for the Hardy-Weinberg equilibrium test; minor allele frequency (MAF) < 0.02; and a call rate <90%.
2.4 Genome-wide association study
The GWAS results were reported as the proportion of the variance explained by non-overlapping genomic windows of 20 adjacent SNP (Zhang et al., 2016; Hay and Roberts, 2018; Oliveira et al., 2019; Zhuang et al., 2020). The phenotypes of FLM were used as dependent variables in a single-trait threshold animal model. SNP effects were estimated by the weighted single-step genomic BLUP (WssGBLUP) method with two iterations, as proposed by Wang et al. (2012), using the programs of BLUPF90 family (Misztal et al., 2014). As in Vargas et al. (2018) the solutions (
where
The POSTGSF90 software was used to estimate the proportion of variance explained by SNP effects and the criterion used to identify potentially important genomic regions was the sum of the variance in non-overlapping windows of 20 adjacent SNPs, approximately 130 Kb (average density of one SNP per 6.5 kb). Windows that explained greater than 1% of the genetic variance were selected for gene annotation, conducted using R Version 4.1.2 (R Development Core Team, 2017) and the R package GALLO (Fonseca et al., 2020). The .gtf file Bos_taurus.ARS-UCD1.2.104.gtf, corresponding to the bovine reference map ARS-UCD1.2 (Hu et al., 2022) was used for gene annotation.
As LD values (r2) > 0.08 are observed up to 400 Kb of distance between marker pairs for the Nellore breed, with higher linkage disequilibrium estimates (r2 > 0.15) observed in the first 100 Kb (Pérez O’Brien et al., 2014), we evaluated the presence of genes in adjacent 100 Kb windows upstream and downstream of the main windows (average annotation interval of 330 Kb). We also visually inspected the windows with the Genome Data Viewer (NCBI, 2022), in case candidate gene annotation failed to detect related genes located near the coordinates.
2.5 Gene prioritization analysis
The candidate gene prioritization was conducted using GUILDify and ToppGene (Chen, Bardes, et al., 2009; Guney et al., 2014). GUILDify relies on biological databases that predominantly focus on model organisms such as humans, mice and flies, which have been extensively studied and have well-characterized genetic and molecular interaction data. The limited availability of genomic and molecular data for Bos indicus restricts its suitability for interaction networks on GUILDify. Thus, a “trained list” of the top 100 genes associated with relevant trait keywords, including, “mobility aplomb,” “feet,” “foot angle,” “leg,” “rear legs,” “leg conformation,” “leg structure,” “conformational structure,” “feet quality,” “leg quality,” “articular cartilage,” “osteogenic differentiation,” “lameness resistance,” and “hoof health” was created using GUILDify and a species-specific (Homo sapiens) interaction network to identify orthologous genes between humans and cattle Then, the ToppGene Suite was used to perform a prioritization analysis comparing the functional information shared between the list of “trained” genes and the list of “test” genes (genes located within windows that explained more than 1% of the genetic variance). Gene Ontology terms (molecular function, biological process, and cellular component), human and mouse phenotypes, metabolic pathways, PubMed publications, coexpression patterns and diseases were used to retrieve the functional information from genes in training and testing lists (Fonseca et al., 2018). We selected, as prioritized, the genes on the “test” list with the same functional profile as the genes of “trained” list, based on a multiple correction false discovery rate of 5% (p-value ≤ 10–3) (Chen, Bardes, et al., 2009).
3 Results
The incidence value (approximately 5%) for FLM after consistency checks and preliminary editing described above should be highlighted (Table 1). The pedigree file contained 468,302 animals and the incidence of FLM has increased in the most recent years for both sexes (Figure 1) in the population studied. There was a statistically significant difference by Student’s t-test (p < 0.05) in the annual incidence averages between genders. Males (average incidence = 4.98%) were more affected than females (average incidence = 4.23%) by FLM in the period evaluated. After QC, 461,057 SNP remained. All genotyped samples had a call rate per individual greater than 0.9. Genotypes from 12,537 animals passed QC and were used in the GWAS analyses.
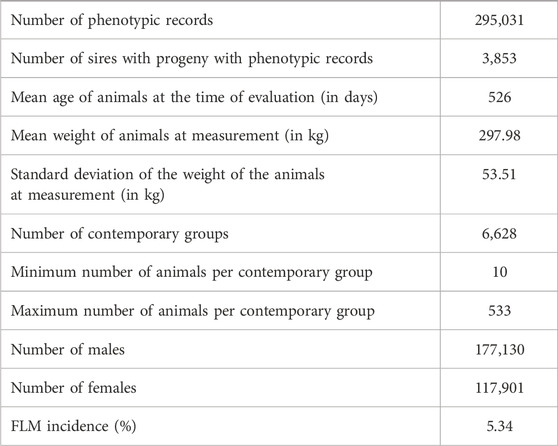
TABLE 1. Descriptive structure of Nellore data used in the yearling weight-feet and legs malformation (YW-FLM) two-trait analysis.
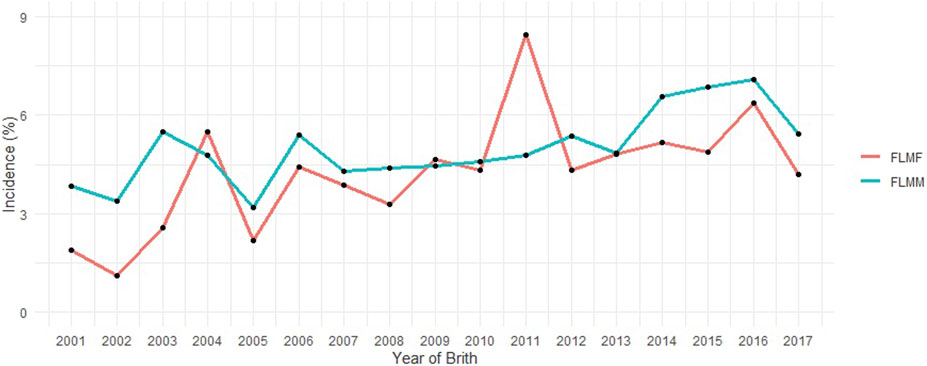
FIGURE 1. Incidence of feet and legs malformation (FLM) at yearling in males (FLMM) and females (FLMF) by year of birth.
The posterior mean of the heritability estimates (L95%; U95%) for FLM was of moderate magnitude (0.1856). In single-trait analyses for comparison purposes, the mean heritability estimated for FLM (L95%; U95%) was 0.17 (0.13; 0.20). The linear trait (YW) had a high heritability value (0.4718) (Table 2), while the posterior mean estimate of the genetic correlation between FLM and YW was −0.2311 (±0.0076). The annual genetic trends for YW and FLM were 0.25 and −0.25 units of genetic standard deviation, respectively (Figure 2).
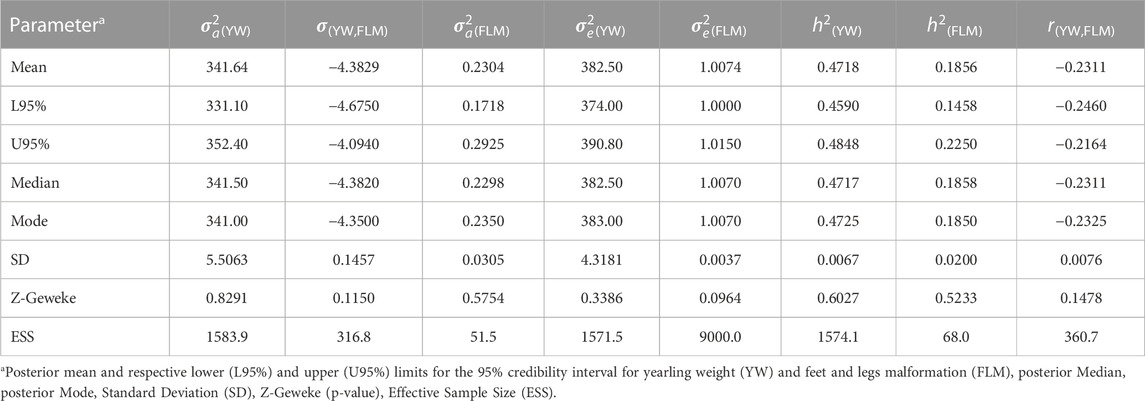
TABLE 2. Estimates of additive (
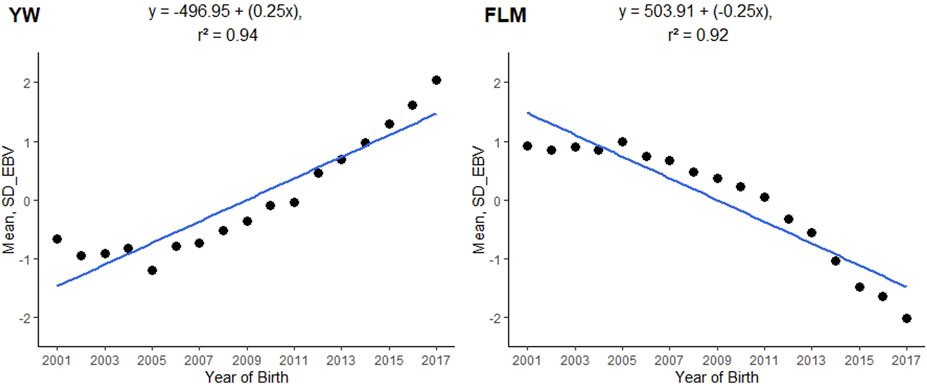
FIGURE 2. Genetic trends of posterior means of breeding values by year of birth for yearling weight (YW) and feet and legs malformation (FLM) evaluated at yearling in Nellore cattle; SD_EBV = a posterior mean of the estimated breeding value expressed in units of genetic standard deviation.
Twelve windows, two of these located on chromosome BTA23 and one on each of chromosomes BTA2, BTA3, BTA5, BTA6, BTA14, BTA18, BTA20, BTA22, BTA27, and BTA29, explained >1% of the genetic variance of FLM. Together, these windows explained 16.37% of the total genetic variance of FLM (Figure 3). Considering 100 Kb upstream and downstream non-overlapping windows of 20 consecutive SNPs, 61 positional candidate genes for FLM were identified. The window containing the most FLM candidate genes (17 genes) was in BTA3, and the window explaining the highest proportion of variance (1.7%) was in BTA27. The four candidate genes (EXT1, ITGA1, ATG7, and PPARD) prioritized by GUILDify and ToppGene analysis were in four windows, which together explained 5.43% of the total genetic variance. Additionally, skeletal development-related genes SCUBE3 and SHOX that were not prioritized in the ToppGene analysis were identified by visual inspection in the Genome Data Viewer (NCBI, 2022). We also identified 24 genes (starting from LOC) for which no associated sign gene was present (Table 3). There were not any orthologous genes for these LOC that could be interesting to discuss in the FLM context.
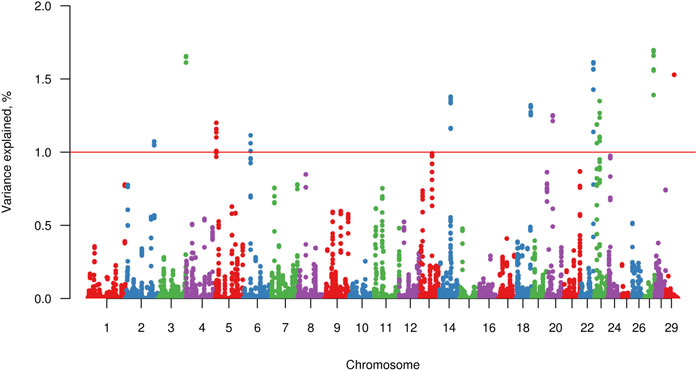
FIGURE 3. Manhattan plot for percentage of variance explained by non-overlapping windows of 20 adjacent SNP for feet and legs malformation obtained by WssGBLUP method. The red line indicates the 1% variance threshold explained by the windows.
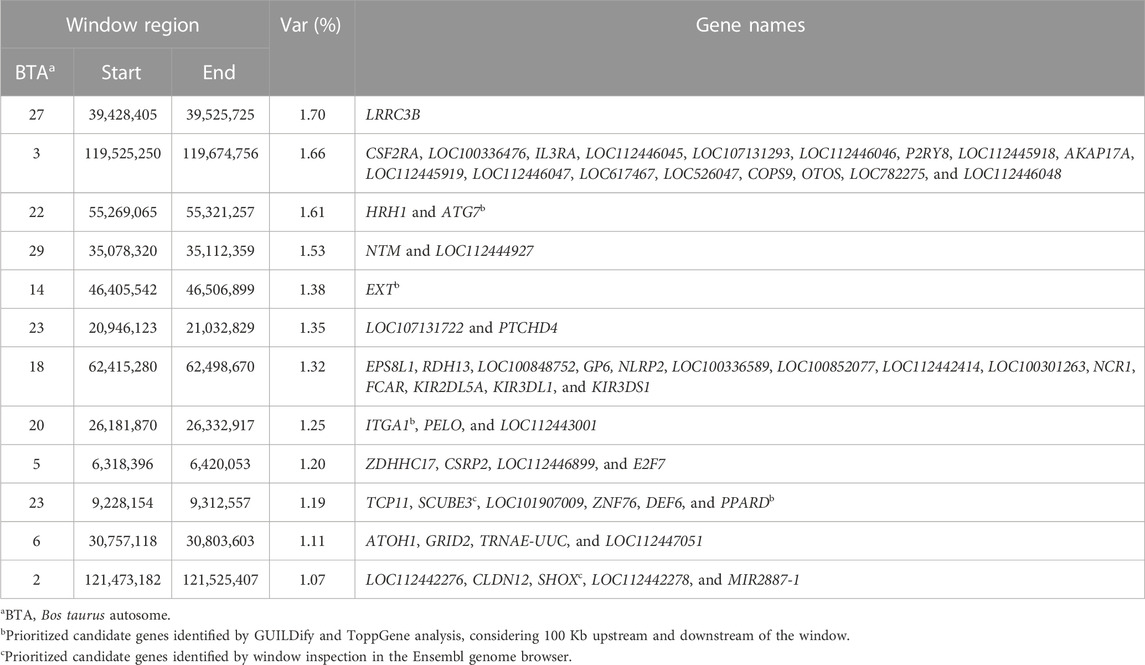
TABLE 3. Annotated genes within the windows of 20 adjacent SNP that explained more than 1% of genetic variance for FLM in Nellore cattle.
4 Discussion
New-born animals must participate in their own nutrition and locomotion to survive. Thus, they are endowed with a set of muscles that grew during prenatal life in such a way that they play their part in ensuring survival. The new-born animal is able to walk and to stuck because “early developing” muscles of the distal parts of the limbs are well developed at birth (Berg and Butterfield, 1974). During their development, however, the musculoskeletal system is influenced by genetic factors that, if expressed, alter the animal’s posture. In addition, environmental factors such as overfeeding, the availability and distribution of food and water, and the topography of grazing environments can also contribute to persistent postural issues (Vargas et al., 2018).
Differences in growth curves and body weight composition between the sexes can be explained by hormonal differences such as androgenic effects (e.g., testosterone) (Young and Bass, 1984; Mangwiro et al., 2013). In the Nellore breed, there is little difference between male growth and female growth before weaning, but this difference becomes more pronounced with age (Malhado et al., 2009; Arruda et al., 2018). This could explain the observed differences in the incidence of FLM in males and females (Figure 1). The observed overall incidence rate (5.34%) was higher than that reported by Vargas et al. (2017) (4.5%), who used a subsample from a single Nellore Alliance program with approximately one-third of the observations used in this study. The increase in the incidence of FLM in recent years in the same population may be influenced by the subjectivity of assessment and should be observed with caution. As field technicians gain experience over the years, they tend to place higher discerning demands on the evaluation. “Knock-kneed” (when the knee joints lie inside this line), “bow-legged” (when the knee joints lie outside this line) and “straight-legged” (high angulation of the tibiotarsal joint) (Vargas et al., 2018) are examples of malformation patterns that can be best rated by an experienced technician.
The magnitude of heritability estimated for the FLM depends on the rating assigned to the animal at the time of assessment and may be directly related to the quality of the recording set measured for the classification of the trait (Vargas et al., 2017). Furthermore, using YW as an “anchor,” in a two-trait analysis, may help capture a greater proportion of additional variability. Studies in dairy cows have shown heritability estimates for leg and foot conformation traits to range from 0.03 to 0.22 (Berry et al., 2004; Chapinal et al., 2013; Häggman and Juga, 2013). In the Nellore breed, Passafaro et al. (2013) and Vargas et al. (2018), Vargas et al. (2017) obtained moderate to high heritability estimates of the FLM, as estimated in this study, which indicating the potential for improvement through selection.
The assessment of the genetic correlation between YW and FLM (−0.2311) was considered favourable, since in FLM, the lowest taxonomic rank (score 0) indicated that animals did not have feet and legs defects, whereas in YW animals with stronger expression of the trait are required. For example, in the population studied, animals that had difficulty walking due to congenital deformities of the legs and feet (score 1) tended to gain less weight. Consistent with the results of this study, Vargas et al. (2017) estimated the genetic correlation between YW and FLM to be 0.39, on a scale of 1–5 (ranging from least ideal to most ideal). This suggests that animals with better legs and feet tend to be heavier. In addition to YW-related responses, which are part of the selection index, estimated genetic trends from FLM suggest that strategies cull problem animals promote favorable genetic changes and facilitate the genetic progression of the trait in the study population.
A literature search, such as that carried out by Vargas et al. (2018), is an important step in the process of identifying functional candidate genes mapped around candidate markers. However, if a greater number of positional candidate genes are identified, the literature review may become unfeasible (Fonseca et al., 2018). The strategy of automating gene prioritization analysis (Martins de Carvalho et al., 2020; Sweett et al., 2020) speeds up the identification of genes related to the regulation of biological processes associated with the phenotype. Combining GUILDify and ToppGene in a single analysis is a novel approach in the functional prioritization literature (Kominakis et al., 2017). Fonseca et al. (2018) highlight the importance of combining tools and functional information from multiple sources (and species) to perform a better selection of functional candidate genes and further explore GWAS results. ToppGene does not use keywords to select genes, instead, the software uses the similarities between the functional patterns of the genes presented in the candidate gene list and the trained gene list. Therefore, the prioritized genes presented can be interpreted as a statistical measure of how much the functional profile of each candidate gene is like the entire functional profile of the trained list (GUILDify) (Martins de Carvalho et al., 2020).
With the aim of finding genes that are closely associated with the phenotype and are critical for its development and maintenance, the present GWAS allowed to find genes reported in the literature with traits related to the feet and legs conformation in dairy and beef cattle populations. For example, the specific deletion in the muscle tissue of the ATG7 gene (BTA22) in mice that resulted in profound muscle atrophy and age-dependent decrease in strength (Masiero et al., 2009). The ATG7 gene (BTA22) encodes an E1-like essential activating enzyme for autophagy and cytoplasmic transport to the vacuole (Gao et al., 2013). The autophagy flow is important to preserve muscle mass and maintain the integrity of the myofiber. In beef cattle, increased ATG7 gene expression was correlated with skeletal muscle growth and body weight during the fattening period, regardless of the muscle evaluated (Nakanishi et al., 2019).
The EXT1 gene (BTA14) encodes a glycosyltransferase responsible for heparan sulfate (HS) polymerization, related to fibroblast growth factor (Nadanaka et al., 2008). The interaction between growth factors and their receptors is regulated by the amount of HS. A defect in HS biosynthesis due to somatic mutations in EXT1 can cause a localized break in the negative feedback loop that regulates chondrocyte proliferation and maturation, allowing premature differentiation and therefore aberrant bone growth (Duncan et al., 2001).
Another prioritized candidate gene, ITGA1 (BTA20), encodes a subunit of the cell-surface receptor integrin α1β1, which acts in the regulation of hepatic glucose and lipid metabolism under conditions of overnutrition in vivo. On a high fat diet, mice with the ITGA1 gene inactivated show severe hepatic insulin resistance and decreased hepatic fat accumulation (Williams et al., 2015). In Korean Hanwoo cattle, the ITGA1 gene was significantly associated with intramuscular fat deposition (Lee et al., 2013; de las Heras-Saldana et al., 2020). Weight gain accelerated by increased fat deposition in young animals can cause stress on ligaments, tendons and joints, promoting gene expression patterns that predispose to alterations in the normal conformation of feet and legs.
The same association can be made for the PPARD gene (BTA23), the most abundant form of Peroxisome Proliferator-Activated Receptor (PPAR) family in skeletal muscle (Brennan et al., 2009). A key role for the PPARD gene in controlling the biological processes that drive ruminal epithelial cell development in new-born calves was suggested by Naeem et al. (2012). Its increased expression after improved diet suggests an important role in promoting the use of long-chain fatty acids as substrates for oxidation in cell membranes during differentiation in ruminal development. In dairy cows, the PPARD gene is involved in muscle fatty acid transport and oxidation during early lactation, and muscle fat replacement from days 3 to 30 of lactation (Schäff et al., 2013). Studies have shown that the gene is highly expressed in the intestinal epithelium, keratinocytes, and liver, consistent with an important biological role in these tissues and influenced by the onset lactation and the type of lipids that are supplied (Girroir et al., 2008; Akbar et al., 2013).
The SCUBE3 (BTA23) and SHOX (BTA2) genes, identified by visual inspection of significant windows of the FLM, have relevant roles in bone formation. The SCUBE3 gene acts as a BMP2/BMP4 (bone morphogenetic protein) coreceptor and positively regulates signalling possibly enhancing the specific interaction between BMP and BMP type I receptors (Lin et al., 2021). Malfunction of the SCUBE3 gene has been linked to problems such as craniofacial and dental defects, reduced body size, and defective endochondral bone growth in mice, and osteosarcoma in humans (Liang et al., 2015). The SCUBE3 and PPARD genes are in the same window of BTA23, 79 kb apart. The presence of antagonistic causal mutations in these two genes, that is, an increased expression in PPARD influencing accelerated growth and a malfunction in SCUBE3 promoting bone defects suggests a possible biological mechanism for the genetic correlations observed between FLM and YW. However, it is important to emphasize that this information is specific to the mentioned genes and their relation to specific traits. Additional studies and deeper analyses would be needed to confirm and fully understand the role of these genes and their interactions in relation to the mentioned traits.
Rafati et al. (2016) found a complete association between the SHOX gene deletion and the skeletal atavism of the Shetland pony. It is a genetic disorder characterized by abnormal growth of the ulna and fibula, which elongate the carpal and tarsal joints, respectively. This can lead to abnormal bone structure and restricted movement. The SHOX gene is also associated with skeletal defects such as Léri-Weill dyschondrosteosis (Benito-Sanz et al., 2012), short stature, and limb deformities in humans (Jorge et al., 2007; Chen, Wildhardt, et al., 2009; Raudsepp et al., 2012).
5 Conclusion
Overall, this study provides valuable information on the genetic basis of feet and leg malformations in Nellore cattle, with important implications for both animal welfare and agricultural productivity. Estimates of additive genetic variability and heritability for this trait suggest that selection may reduce the incidence of this problem. The phenotypic incidence shows the importance of reinforcing this selection, although the estimated trend curve shows a relatively favorable genetic gain over the study period. Furthermore, the genetic association of yearling weight with feet and legs malformations may have implications for selection criteria used in breeding programs. The current GWAS was able to identify chromosomal regions associated with feet and legs malformation in Nellore cattle. The roles of prioritized candidate genes in skeletal muscle development (ATG7), aberrant bone growth (EXT1), lipid metabolism (ITGA1, PPARD), intramuscular fat deposition (ITGA1), and skeletogenesis (SCUBE3, SHOX) underscore the importance of these genes in malformation incidence. The identification of genes associated with foot and leg malformations allows selective breeding of animals that are less likely to develop these conditions, leading to healthier and more productive herds. The identified genetic markers could facilitate the development of genetic tests that can identify carriers of these mutations, allowing breeders to make more informed breeding decisions that reduce the incidence of malformations in future generations.
Data availability statement
The data analyzed in this study was obtained from GenSys Associated Consultants, the following licenses/restrictions apply: access is restricted to protect confidential or proprietary information. Requests to access these datasets should be directed to Haroldo Neves, gensys.haroldo@gmail.com.
Author contributions
RC, LA, CG, PF, and TS contributed to conception and design of the study; HN and RC organized the database; RC, LA, and CG provided computational resources to perform the analyzes; TS performed the statistical analysis; PF, DS, GV, IC, CT, and RC validated the results; TS wrote the first draft of the manuscript; RC, CG, PF, DS, GV, HN, IC, CT, and TS wrote sections of the manuscript; LA, RC, and TS contributed to funding acquisition. All authors contributed to the article and approved the submitted version.
Funding
This research was financially supported by São Paulo Research Foundation (FAPESP—Grant Nos 2017/10630–2 and 2018/20026–8), National Council for Science and Technological Development (CNPq), and “Coordenação de Aperfeiçoamento de Pessoal de Nível Superior—Brasil” (CAPES)—Finance Code 001.
Acknowledgments
The authors would like to thank the GenSys for providing the data.
Conflict of interest
Author HN was employed by GenSys Associated Consultants.
The remaining authors declare that the research was conducted in the absence of any commercial or financial relationships that could be construed as a potential conflict of interest.
Publisher’s note
All claims expressed in this article are solely those of the authors and do not necessarily represent those of their affiliated organizations, or those of the publisher, the editors and the reviewers. Any product that may be evaluated in this article, or claim that may be made by its manufacturer, is not guaranteed or endorsed by the publisher.
References
Akbar, H., Schmitt, E., Ballou, M. A., Corrêa, M. N., Depeters, E. J., and Loor, J. J. (2013). Dietary lipid during late-pregnancy and early-lactation to manipulate metabolic and inflammatory gene network expression in dairy cattle liver with a focus on PPARs. Gene Regul. Syst. Biol. 2013 (7), 103–123. doi:10.4137/GRSB.S12005
Aragão, A., and Contini, E. (2021). O agro no Brasil e no mundo: uma síntese do período de 2000 a 2020. Embrapa SIRE.
Arruda, R. M. d. S. de, Souza, J. C. d., Jardim, R. J. D., Ferraz Filho, P. B., and Silva, L. O. C. (2018). Growth curves and nutritional requirements for maintenance of asymptotic weight of Nellore cattle. Rev. Ciência Agronômica 49 (4), 692–698. doi:10.5935/1806-6690.20180078
Benito-Sanz, S., Royo, J. L., Barroso, E., Paumard-Hernández, B., Barreda-Bonis, A. C., Liu, P., et al. (2012). Identification of the first recurrent PAR1 deletion in Léri-Weill dyschondrosteosis and idiopathic short stature reveals the presence of a novel SHOX enhancer. J. Med. Genet. 49 (7), 442–450. doi:10.1136/jmedgenet-2011-100678
Berg, R. T., and Butterfield, R. M. (1974). “Growth of meat animals,” in Meat. Butterwort. Editors D. J. A. Cole, and R. A. Lawrie (London: Elsevier Science), 22–29. Available at: https://books.google.com.br/books?id=TAbLBAAAQBAJ.
Berry, D. P., Buckley, F., Dillon, P., Evans, R. D., and Veerkamp, R. F. (2004). Genetic relationships among linear type traits, milk yield, body weight, fertility and somatic cell count in primiparous dairy cows. Ir. J. Agric. Food Res. 43 (2), 161–176.
Brennan, K. M., Michal, J. J., Ramsey, J. J., and Johnson, K. A. (2009). Body weight loss in beef cows: i. The effect of increased β-oxidation on messenger ribonucleic acid levels of uncoupling proteins two and three and peroxisome proliferator-activated receptor in skeletal muscle. J. Animal Sci. 87 (9), 2860–2866. doi:10.2527/jas.2008-1302
Chapinal, N., Koeck, A., Sewalem, A., Kelton, D. F., Mason, S., Cramer, G., et al. (2013). Genetic parameters for hoof lesions and their relationship with feet and leg traits in Canadian Holstein cows. J. Dairy Sci. 96, 2596–2604. doi:10.3168/jds.2012-6071
Chen, J., Bardes, E. E., Aronow, B. J., and Jegga, A. G. (2009). ToppGene Suite for gene list enrichment analysis and candidate gene prioritization. Nucleic Acids Res. 37 (2), 305–311. doi:10.1093/nar/gkp427
Chen, J., Wildhardt, G., Zhong, Z., Röth, R., Weiss, B., Steinberger, D., et al. (2009). Enhancer deletions of the SHOX gene as a frequent cause of short stature: the essential role of a 250 kb downstream regulatory domain. J. Med. Genet. 46 (12), 834–839. doi:10.1136/jmg.2009.067785
de las Heras-Saldana, S., Chung, K. Y., Kim, H., Lim, D., Gondro, C., and van der Werf, J. H. J. (2020). Differential gene expression in longissimus dorsi muscle of hanwoo steers—New insight in genes involved in marbling development at younger ages. Genes. 11 (11), 1–17. doi:10.3390/genes11111381
Duncan, G., McCormick, C., and Tufaro, F. (2001). The link between heparan sulfate and hereditary bone disease: finding a function for the EXT family of putative tumor suppressor proteins. J. Clin. Investigation 108 (4), 511–516. doi:10.1172/JCI13737
Fonseca, P. A. de S., Dos Santos, F. C., Lam, S., Suárez-Vega, A., Miglior, F., Schenkel, F. S., et al. (2018). Genetic mechanisms underlying spermatic and testicular traits within and among cattle breeds: systematic review and prioritization of GWAS results. J. Animal Sci. 96 (12), 4978–4999. doi:10.1093/jas/sky382
Fonseca, P. A. S., Suárez-Vega, A., Marras, G., and Cánovas, Á. (2020). Gallo: an R package for genomic annotation and integration of multiple data sources in livestock for positional candidate loci. GigaScience 9 (12), giaa149. doi:10.1093/gigascience/giaa149
Gao, W., Chen, Z., Wang, W., and Stang, M. T. (2013). E1-Like activating enzyme Atg7 is preferentially sequestered into p62 aggregates via its interaction with LC3-I. PLoS ONE 8 (9), e73229. doi:10.1371/journal.pone.0073229
GenSys, C. A. S. L. (2019). Aliança Nelore: sumário de avaliação de reprodutores. Available at: https://gensys.com.br/sumario/sumario-alianca-nelore-2019-2020/ (Accessed October 18, 2019).
Geweke, J. (1992). Evaluating the accuracy of sampling-based approaches to the calculations of posterior moments. Bayesian Stat. 4, 641–649.
Gianola, D., and Foulley, J. L. (1983). Sire evaluation for ordered categorical data with a threshold model. Genet. Sel. Evol. 15 (2), 201–224. doi:10.1186/1297-9686-15-2-201
Girroir, E. E., Hollingshead, H. E., He, P., Zhu, B., Perdew, G. H., and Peters, J. M. (2008). Quantitative expression patterns of peroxisome proliferator-activated receptor-beta/delta (PPARbeta/delta) protein in mice. Biochem. Biophysical Res. Commun. 371 (3), 456–461. doi:10.1016/j.bbrc.2008.04.086
Guney, E., Garcia-Garcia, J., and Oliva, B. (2014). GUILDify: a web server for phenotypic characterization of genes through biological data integration and network-based prioritization algorithms. Bioinformatics 30 (12), 1789–1790. doi:10.1093/bioinformatics/btu092
Häggman, J., and Juga, J. (2013). Genetic parameters for hoof disorders and feet and leg conformation traits in Finnish Holstein cows. J. Dairy Sci. 96, 3319–3325. doi:10.3168/jds.2012-6334
Hay, E. H., and Roberts, A. (2018). Genome-wide association study for carcass traits in a composite beef cattle breed. Livest. Sci. 213, 35–43. doi:10.1016/j.livsci.2018.04.018
Heidelberger, P., and Welch, P. D. (1983). Simulation run length control in the presence of an initial transient. Operations Res. 31 (6), 1109–1144. doi:10.1287/opre.31.6.1109
Hu, Z.-L., Park, C. A., and Reecy, J. M. (2022). Bringing the animal QTLdb and CorrDB into the future: meeting new challenges and providing updated services. Nucleic Acids Res. 50 (D1), D956–D961. doi:10.1093/nar/gkab1116
Irano, N., de Camargo, G. M. F., Costa, R. B., Terakado, A. P. N., Magalhães, A. F. B., Silva, R. M. d. O., et al. (2016). Genome-wide association study for indicator traits of sexual precocity in Nellore cattle. PLoS ONE 11 (8), e0159502. doi:10.1371/journal.pone.0159502
Jorge, A. A. L., Souza, S. C., Nishi, M. Y., Billerbeck, A. E., Libório, D. C. C., Kim, C. A., et al. (2007). SHOX mutations in idiopathic short stature and Leri-Weill dyschondrosteosis: frequency and phenotypic variability. Clin. Endocrinol. 66 (1), 130–135. doi:10.1111/j.1365-2265.2006.02698.x
Kominakis, A., Hager-Theodorides, A. L., Zoidis, E., Saridaki, A., Antonakos, G., and Tsiamis, G. (2017). Combined GWAS and ‘guilt by association’-based prioritization analysis identifies functional candidate genes for body size in sheep. Genet. Sel. Evol. 49 (1), 41–16. doi:10.1186/s12711-017-0316-3
Lee, H. J., Jang, M., Kim, H., Kwak, W., Park, W., Hwang, J. Y., et al. (2013). Comparative transcriptome analysis of adipose tissues reveals that ECM-receptor interaction is involved in the depot-specific adipogenesis in cattle. PLoS ONE 8 (6), e66267. doi:10.1371/journal.pone.0066267
Legarra, A., Aguilar, I., and Misztal, I. (2009). A relationship matrix including full pedigree and genomic information. J. Dairy Sci. 92 (9), 4656–4663. doi:10.3168/jds.2009-2061
Liang, W., Yang, C., Peng, J., Qian, Y., and Wang, Z. (2015). The expression of HSPD1, SCUBE3, CXCL14 and its relations with the prognosis in osteosarcoma. Cell. Biochem. Biophysics 73 (3), 763–768. doi:10.1007/s12013-015-0579-7
Lin, Y. C., Niceta, M., Muto, V., Vona, B., Pagnamenta, A. T., Maroofian, R., et al. (2021). SCUBE3 loss-of-function causes a recognizable recessive developmental disorder due to defective bone morphogenetic protein signaling. Am. J. Hum. Genet. 108 (1), 115–133. doi:10.1016/j.ajhg.2020.11.015
Lourenco, D., Legarra, A., Tsuruta, S., Masuda, Y., Aguilar, I., and Misztal, I. (2020). Single-step genomic evaluations from theory to practice: using SNP chips and sequence data in BLUPF90. Genes. 11 (7), 790. doi:10.3390/genes11070790
Malhado, C. H. M., Ramos, A. A., Carneiro, P. L. S., Azevedo, D. M. M. R., de Mello, P. R. A., Martins Filho, R., et al. (2009). Nonlinear models used to describe the growth of Nellore cattle in the state of bahia: 1. Environmental effect. Braz. J. Animal Health Prod. 10 (4), 821–829.
Mangwiro, T. N., Dhliwayo, M., and Tayengwa, T. (2013). Non genetic factors affecting calf growth traits in mashona cattle. Sci. J. Animal Sci. 2 (2), 222–227. doi:10.14196/sjas.v2i8.854
MAPA (2006). Instrução Normativa no53, de 27 de setembro de 2006, Secretaria de Defesa Agropecuária. Available at: http://sistemasweb.agricultura.gov.br/sislegis/action/detalhaAto.do?method=visualizarAtoPortalMapa&chave=735332411 (Accessed October 29, 2019).
Martins de Carvalho, L., Fonseca, P. A. S., Paiva, I. M., Damasceno, S., Pedersen, A. S. B., da Silva E Silva, D., et al. (2020). Identifying functionally relevant candidate genes for inflexible ethanol intake in mice and humans using a guilt-by-association approach. Brain Behav. 10 (12), 018799–e1915. doi:10.1002/brb3.1879
Masiero, E., Agatea, L., Mammucari, C., Blaauw, B., Loro, E., Komatsu, M., et al. (2009). Autophagy is required to maintain muscle mass. Cell. Metab. 10 (6), 507–515. doi:10.1016/j.cmet.2009.10.008
Masuda, Y., Legarra, A., Aguilar, I., and Misztal, I. (2019). 331 Efficient quality control methods for genomic and pedigree data used in routine genomic evaluation. J. Animal Sci. 97 (3), 50–51. doi:10.1093/jas/skz258.101
Melo, T. P., Takada, L., Baldi, F., Oliveira, H. N., Dias, M. M., Neves, H. H. R., et al. (2016). Assessing the value of phenotypic information from non-genotyped animals for QTL mapping of complex traits in real and simulated populations. BMC Genet. 17 (1), 89–9. doi:10.1186/s12863-016-0394-1
Misztal, I., Tsuruta, S., Lourenco, D. A. L., Aguilar, I., Legarra, A., Vitezica, Z., et al. (2014). Manual for BLUPF90 family of programs. Athens, GA: Animal and Dairy Science, University of Georgia. Available at: http://nce.ads.uga.edu/wiki/doku.php?id=start.
Nadanaka, S., Ishida, M., Ikegami, M., and Kitagawa, H. (2008). Chondroitin 4-O-sulfotransferase-1 modulates Wnt-3a signaling through control of E disaccharide expression of chondroitin sulfate. J. Biol. Chem. 283 (40), 27333–27343. doi:10.1074/jbc.M802997200
Naeem, A., Drackley, J. K., Stamey, J., and Loor, J. J. (2012). Role of metabolic and cellular proliferation genes in ruminal development in response to enhanced plane of nutrition in neonatal Holstein calves. J. Dairy Sci. 95 (4), 1807–1820. doi:10.3168/jds.2011-4709
Nakanishi, T., Tokunaga, T., Ishida, T., Kobayashi, I., Katahama, Y., Yano, A., et al. (2019). Changes in expression of the autophagy-related genes microtubule-associated protein 1 light chain 3β and autophagy related 7 in skeletal muscle of fattening Japanese black cattle: a pilot study. Asian-Australasian J. Animal Sci. 32 (4), 592–598. doi:10.5713/ajas.18.0370
Oliveira, H. R., Cant, J. P., Brito, L. F., Feitosa, F. L. B., Chud, T. C. S., Fonseca, P. A. S., et al. (2019). Genome-wide association for milk production traits and somatic cell score in different lactation stages of Ayrshire, Holstein, and Jersey dairy cattle. J. Dairy Sci. 102 (9), 8159–8174. doi:10.3168/jds.2019-16451
Passafaro, T. L., Raidan, F. S. S., Aquino, M. P., Eescarce, T. C., Josahkian, L. A., Toral, F. L. B., et al. (2013). “Parâmetros genéticos para escores visuais de tourinhos Nelore com modelos lineares e de limiar,” in X simpósio brasileiro de Melhoramento animal uberaba, MG – 2013. Available at: http://sbmaonline.org.br/anais/x/trabalhos/pdf/6NRQ.pdf.
Pérez O’Brien, A. M., Mészáros, G., Utsunomiya, Y. T., Sonstegard, T. S., Garcia, J. F., Van Tassell, C. P., et al. (2014). Linkage disequilibrium levels in Bos indicus and Bos taurus cattle using medium and high density SNP chip data and different minor allele frequency distributions. Livest. Sci. 166 (1), 121–132. doi:10.1016/j.livsci.2014.05.007
Plummer, M., Best, N., Cowles, K., and Vines, K. (2006). Coda: convergence diagnosis and output analysis for MCMC. R. News 6 (1), 7–11.
R Development Core Team, (2017). R: A language and environment for statistical computing. ’ Vienna, Austria: R Foundation for Statistical Computing. Available at: https://www.r-project.org/.
Rafati, N., Andersson, L. S., Mikko, S., Feng, C., Raudsepp, T., Pettersson, J., et al. (2016). Large deletions at the SHOX locus in the pseudoautosomal region are associated with skeletal atavism in shetland ponies. G3 Genes., Genomes, Genet. 6 (7), 2213–2223. doi:10.1534/g3.116.029645
Rahe, M. C., Evrard, L., and Holmes, N. B. (2022). Congenital limb deformities in a neonatal crossbred pig. Case Rep. Veterinary Med. 2022, 5516633. doi:10.1155/2022/5516633
Raudsepp, T., Das, P. J., Avila, F., and Chowdhary, B. P. (2012). The pseudoautosomal region and sex chromosome aneuploidies in domestic species. Sex. Dev. 6 (1-3), 72–83. doi:10.1159/000330627
Rosa, A. N., Menezes, G. R. O., Egito, A. A., and Nogueira, E. (2021). Genética aplicada para pequenos e médios produtores de gado de corte. Available at: http://www.infoteca.cnptia.embrapa.br/handle/doc/1130820.
Roso, V. M., and Schenkel, F. S. (2006). “AMC-A computer program to assess the degree of connectedness among contemporary groups,” in Proceedings of the 8th World Congress on Genetics Applied to Livestock Production, Belo Horizonte, Minas Gerais, Brazil, August, 2006, 13–18.
Sargolzaei, M., Chesnais, J. P., and Schenkel, F. S. (2014). A new approach for efficient genotype imputation using information from relatives. BMC Genomics 15 (1), 478. doi:10.1186/1471-2164-15-478
Schäff, C., Börner, S., Hacke, S., Kautzsch, U., Sauerwein, H., Spachmann, S. K., et al. (2013). Increased muscle fatty acid oxidation in dairy cows with intensive body fat mobilization during early lactation. J. Dairy Sci. 96 (10), 6449–6460. doi:10.3168/jds.2013-6812
Schwertz, C. I., Bianchi, R. M., Vielmo, A., Piva, M. M., Gris, A. H., Pavarini, S. P., et al. (2023). Nutritional chondrodysplasia in cattle in Brazil. Trop. Animal Health Prod. 55 (1), 26–28. doi:10.1007/s11250-022-03438-7
Smith, B. J. (2007). boa: an R package for MCMC output convergence assessment and posterior inference. J. Stat. Softw. 21 (11), 1–37. doi:10.18637/jss.v021.i11
Sorensen, D., and Gianola, D. (2002). “Likelihood, Bayesian and MCMC methods in quantitative genetics,” in Statistics for biology and health. Editor K. Dietzet al. (New York, NY: Springer), 740.
Sweett, H., Fonseca, P. A. S., Suárez-Vega, A., Livernois, A., Miglior, F., and Cánovas, A. (2020). Genome-wide association study to identify genomic regions and positional candidate genes associated with male fertility in beef cattle. Sci. Rep. 10 (1), 20102–20114. doi:10.1038/s41598-020-75758-3
Vallejo, R. L., Leeds, T. D., Fragomeni, B. O., Gao, G., Hernandez, A. G., Misztal, I., et al. (2016). Evaluation of genome-enabled selection for bacterial cold water disease resistance using progeny performance data in rainbow trout: insights on genotyping methods and genomic prediction models. Front. Genet. 7, 96. doi:10.3389/fgene.2016.00096
Vargas, G., Neves, H. H. R., Camargo, G. M. F., Cardoso, V., Munari, D. P., and Carvalheiro, R. (2018). Genome-wide association study and functional analysis of feet and leg conformation traits in Nellore cattle. J. Animal Sci. 96 (5), 1617–1627. doi:10.1093/jas/sky079
Vargas, G., Neves, H. H. R., Cardoso, V., Munari, D. P., and Carvalheiro, R. (2017). Genetic analysis of feet and leg conformation traits in Nelore cattle. J. Animal Sci. 95 (6), 2379–2384. doi:10.2527/jas2016.1327
Wang, H., Misztal, I., Aguilar, I., Legarra, A., and Muir, W. M. (2012). Genome-wide association mapping including phenotypes from relatives without genotypes. Genet. Res. 94 (2), 73–83. doi:10.1017/S0016672312000274
Williams, A. S., Kang, L., Zheng, J., Grueter, C., Bracy, D. P., James, F. D., et al. (2015). Integrin α1-null mice exhibit improved fatty liver when fed a high fat diet despite severe hepatic insulin resistance. J. Biol. Chem. 290 (10), 6546–6557. doi:10.1074/jbc.M114.615716
Young, O. A., and Bass, J. J. (1984). Effect of castration on bovine muscle composition. Meat Sci. 11 (2), 139–156. doi:10.1016/0309-1740(84)90011-1
Zhang, X., Lourenco, D., Aguilar, I., Legarra, A., and Misztal, I. (2016). Weighting strategies for single-step genomic BLUP: an iterative approach for accurate calculation of GEBV and GWAS. Front. Genet. 7, 151. doi:10.3389/fgene.2016.00151
Zhuang, Z., Xu, L., Yang, J., Gao, H., Zhang, L., Gao, X., et al. (2020). Weighted single-step genome-wide association study for growth traits in Chinese simmental beef cattle. Genes. 11 (2), 189. doi:10.3390/genes11020189
NCBI (2022) Cattle (Bos taurus) - NCBI Genome Data Viewer, . Bethesda, Maryland: National Library of Medicine, Available at: https://www.ncbi.nlm.nih.gov/genome/gdv/?org=bos-taurus (Accessed: 4 May 2022).
Keywords: Bos taurus indicus, candidate genes, functional trait, locomotion, welfare
Citation: Silva TdL, Gondro C, Fonseca PAdS, Silva DAd, Vargas G, Neves HHdR, Carvalho Filho I, Teixeira CdS, Albuquerque LGd and Carvalheiro R (2023) Feet and legs malformation in Nellore cattle: genetic analysis and prioritization of GWAS results. Front. Genet. 14:1118308. doi: 10.3389/fgene.2023.1118308
Received: 07 December 2022; Accepted: 01 August 2023;
Published: 16 August 2023.
Edited by:
Luciana Regitano, Brazilian Agricultural Research Corporation (EMBRAPA), BrazilReviewed by:
Vinicius Henrique Da Silva, University of São Paulo (ESALQ-USP), BrazilBárbara Silva-Vignato, University of São Paulo, Brazil
Juliana Petrini, Instituto Clínica do Leite, Brazil
Copyright © 2023 Silva, Gondro, Fonseca, Silva, Vargas, Neves, Carvalho Filho, Teixeira, Albuquerque and Carvalheiro. This is an open-access article distributed under the terms of the Creative Commons Attribution License (CC BY). The use, distribution or reproduction in other forums is permitted, provided the original author(s) and the copyright owner(s) are credited and that the original publication in this journal is cited, in accordance with accepted academic practice. No use, distribution or reproduction is permitted which does not comply with these terms.
*Correspondence: Thales de Lima Silva, tl.silva@unesp.br