- 1Department of Laboratory Medicine, Shunde Hospital of Guangzhou University of Chinese Medicine, Foshan, China
- 2Department of Pathology, Shunde Hospital of Guangzhou University of Chinese Medicine, Foshan, China
- 3Department of Laboratory Science, The Second Affiliated Hospital of Guangzhou University of Chinese Medicine, Guangzhou, China
Background: The development of distant metastasis (DM) results in poor prognosis of breast cancer (BC) patients, however, it is difficult to predict the risk of distant metastasis.
Methods: Differentially expressed genes (DEGs) were screened out using GSE184717 and GSE183947. GSE20685 were randomly assigned to the training and the internal validation cohort. A signature was developed according to the results of univariate and multivariate Cox regression analysis, which was validated by using internal and external (GSE6532) validation cohort. Gene set enrichment analysis (GSEA) was used for functional analysis. Finally, a nomogram was constructed and calibration curves and concordance index (C-index) were compiled to determine predictive and discriminatory capacity. The clinical benefit of this nomogram was revealed by decision curve analysis (DCA). Finally, we explored the relationships between candidate genes and immune cell infiltration, and the possible mechanism.
Results: A signature containing CD74 and TSPAN7 was developed according to the results of univariate and multivariate Cox regression analysis, which was validated by using internal and external (GSE6532) validation cohort. Mechanistically, the signature reflect the overall level of immune infiltration in tissues, especially myeloid immune cells. The expression of CD74 and TSPAN7 is heterogeneous, and the overexpression is positively correlated with the infiltration of myeloid immune cells. CD74 is mainly derived from myeloid immune cells and do not affect the proportion of CD8+T cells. Low expression levels of TSPAN7 is mainly caused by methylation modification in BC cells. This signature could act as an independent predictive factor in patients with BC (p = 0.01, HR = 0.63), and it has been validated in internal (p = 0.023, HR = 0.58) and external (p = 0.0065, HR = 0.67) cohort. Finally, we constructed an individualized prediction nomogram based on our signature. The model showed good discrimination in training, internal and external cohort, with a C-index of 0.742, 0.801, 0.695 respectively, and good calibration. DCA demonstrated that the prediction nomogram was clinically useful.
Conclusion: A new immune infiltration related signature developed for predicting metastatic risk will improve the treatment and management of BC patients.
Introduction
Currently, breast cancer (BC) has been the highest cause of cancer death for women (Sung et al., 2021). Approximately 90% of patient death come from metastatic complications although only 20%–30% of patients suffer from metastatic recurrence, which makes the treatment schedules of metastatic patients different from those of non-metastatic patients (Chen et al., 2018; Medeiros and Allan, 2019). Therefore, accurate identification of distant metastasis (DM) in each individual patient with BC is crucial for determining individualized follow-up strategy and the optimal treatment regimen. The further exploration of new biomarkers is of great significance.
BC is a heterogeneous disease at molecular levels, which is associated with distinct patterns of metastatic spread (Liang et al., 2020). As a result of different molecular subtypes, accurate prediction of DM is still a great challenge. Notably, gene expression-based molecular subtyping appears to have clinical implications for the treatment of patients with BC (Masuda et al., 2013; Prat et al., 2015). Moreover, previous studies have shown that molecular subtypes of BC could be considered as a risk factor for distant recurrence (Gnant et al., 2014; Tobin et al., 2017), This suggests that gene expression profiling has a great value in predicting the probability of DM (Ellis et al., 2011; Filipits et al., 2014). However, what pattern of gene expression causes this difference still remains unclear. In this study, we aim to find gene biomarker associated with metastasis and construct a gene signature that could accurately predict distant metastasis–free survival (DMFS).
Nomogram, a simple devices for predicting the likelihood of disease, is widely used in the field of oncology (Balachandran et al., 2015). However, there is no literature that has applied gene signature to a nomogram for predicting DMFS in BC, although multiple predictive nomograms have been constructed for patients with BC (Rouzier et al., 2005; Wang et al., 2019; Huang et al., 2020). Therefore, the aim of this study was to provides a reliable nomogram to predict the risk of metastasis development based on gene signature in patients with BC.
Materials and methods
Data collection and pre-processing
Data of the cancer and adjacent normal tissues of samples with metastasis were downloaded from the Gene Expression Omnibus (GEO) database: GSE183947 and GSE184717. Meanwhile, GSE20685 and GSE6532 were singled out to construct a gene signature and a nomogram. Samples without complete clinical information or based on different platforms were regarded as substandard samples in the present study. Subsequently, batch effects were removed using Combat from R package SVA (Johnson et al., 2007).
Acquisition of differentially expressed genes (DEGs)
An R package limma (Ritchie et al., 2015) was applied to identify DEGs between cancer and adjacent normal tissues. The threshold was set to |logFC| >2 and the adjusted p < 0.05. Then, we use Venn diagrams to find the intersection of DEGs that simultaneously upregulated or downregulated in both metastatic tumor and primary tumor.
Screening of optimal predictive biomarkers and development of signature
GSE20685 was randomized 1:1 and split into a training cohort and an internal validation cohort. For reproducibility, the random seed was used and set to 3. To find optimal predictive biomarkers, univariate and multivariate Cox regression analysis was performed in the training cohort with a p-value cutoff of 0.05. Then, the regression coefficient was defined according to the multivariate Cox regression model and the formulas were described as follows:
Finally, results were visualized using the “forestplot” package in R.
Construction and assessment of the nomogram
In order to make better use of the signature, a nomogram was constructed using the “RMS” package. The Harrell’s Concordance index (C-index) values range from 0 to 1, which is positively correlated to the predictive performance of the nomogram (Harrell et al., 1996). The nomogram was subjected to bootstrapping validation (1,000 bootstrap resamples) to calculate a relatively corrected C-index. To assess the consistency of DMFS at 3-, 5-, and 10-year between the nomogram predicted probabilities and observed rates, calibration curves were plotted.
Evaluation of predictive value
Gene Expression Profiling Interactive Analysis (GEPIA) (http://gepia.cancer-pku.cn/) is an online tool, which contains massive RNA sequencing data from The Cancer Genome Atlas (TCGA) and Genotype-Tissue Expression (GETx) (Tang et al., 2017). We analyze the differential gene expression and correlation between BC tissues (n = 1,085) and normal tissues (n = 291) using GEPIA.
In terms of the signature, the optimal cutoff value was calculated according to the median value of the signature in the training cohort. Then we use it to divide patients into two groups and predict the DMFS in the training, internal validation, and external validation cohort by means of plotting survival curves based on the Kaplan-Meier method. To date fifty-five dataset have been included in Breast Cancer Gene-Expression Miner v4.7 (bc-GenExMiner) (http://bcgenex.centregauducheau.fr/), which can be used to improve gene prognostic analysis performance (Jézéquel et al., 2012). Candidate biomarkers were validated again using bc-GenExMiner.
Compared with ROC curves, decision curve analysis (DCA) can integrate patient and doctor preference into analysis, which is increasingly being utilized in clinical practice (Huang et al., 2016). To evaluate the clinical utility of models, DCA curves were developed using the “stdca.R” package in R.
Gene set enrichment analysis
The degree of differential gene expression was reordered by high- and low-score groups instead of definite differential gene thresholds, which was used to screen significantly enriched KEGG pathways. This method helps minimize losses of original gene expression data (Subramanian et al., 2005). We performed Gene set enrichment analysis (GSEA) to analyze the difference in anti-metastatic potential between two groups.
Immune infiltration analysis
The relationship between optimal predictive biomarkers and immune infiltrating levels was analyzed using Timer (https://cistrome.shinyapps.io/timer/) (Li et al., 2017). An algorithm named quanTIseq was used to estimate the fraction of immune cell subsets infiltrating the tissue from GSE20685 and GSE6532 (Finotello et al., 2019). Student’s t-test was used to test for significance between high-score group and low-score group.
Immunohistochemistry
28 tissue samples of BC were collected in Shunde Hospital of Guangzhou University of Chinese Medicine to analyze the correlation between CD74 and myeloid cells. To reduce error, Immunohistochemistry (IHC) for CD74 (1:200 dilution; EPR4064, Abcam) and CD33 (1:200 dilution; EPR23051-101, Abcam) were performed by an autostrainer system (Lumatas Titan, LumatasBiosystem Inc.) on 3-μm-thick,formalin-fixed and paraffin-embedded (FFPE) Sections. Counterstaining was done with hematoxylin. The average optical density (AOD) was calculated with Image Pro Plus6.0 to determine the protein expression level.
Cell culture
BC cell line MCF-7,ZR-75-1, MDA-MB-231 and myeloid leukemia cell line K562 were all obtained from the Experimental Center, Shunde Hospital of Guangzhou University of Chinese Medicine (Foshan, China). Three breast cell lines were maintained in DMEM medium with 10% fetal bovine serum (FBS). Cells were seeded in 6-well plates and, 12 h after plating, demethylation was induced with 10 μM 5-aza for 24 h. Stable expression of TSPAN7 was confirmed by RT-qPCR and Western blot.
qRT-PCR
Total RNA was extracted using TRIzol (Invitrogen) and a two-step reverse transcription-quantitative PCR (RT-qPCR) protocol was performed using PrimeScript RT Master Mix (Takara) and TB Green Premix Ex Taq (Takara), following manufacturer’s instructions. GAPDH was used as a loading control. The sequences of TSPAN7 primers were as follows: 5′-CTGGCTGTTGGAGTCTGG-3′ (forward); 5′-CCGATGAGCACATAGGGA' (reverse). The sequences of GAPDH primers were:5′-CGGATTTGGTCGTATTGGG-3′ (forward); 5′-CTGGAAGATGGTGATGGGATT-3′ (reverse). The experiment was repeated three times biologically for statistical analysis. The relative expression was quantified using the 2−ΔΔCT method.
Western blot analysis
Cells were placed on ice and lysed with RIPA buffer (Beyotime, China) containing protease inhibitors Cocktail (MCE,China). Proteins were resolved on SDS-PAGE gels, transferred to PVDF membranes (Millipore, United States), and incubated at 4°C overnight with TSPAN7 primary antibodies diluted at 1:1,000 (ProteinTech, 18695-1-AP) and β-tubulin loading control antibodies diluted at 1:1,000 (Servicebio, GB11017) and the secondary antibody diluted at 1:10,000 (Abcam,ab6721). Bands were visualized using an ECL kit.
Results
Screening of DEGs
A flow chart of the study design is shown in Figure 1. The R package “limma” was used to screen DEGs between tumor and normal tissues in GSE184717 and GSE183947, where a total of 1967 (1,494 upregulated and 473 downregulated) and 351 (180 upregulated and 171downregulated) DEGs were obtained, respectively. The distribution of each gene was visualized by volcano plots (Figures 2A,B). The resulting list of DEGs was the intersection between the above datasets and a total of 62 overlapping DEGs were obtained (Figure 2C).
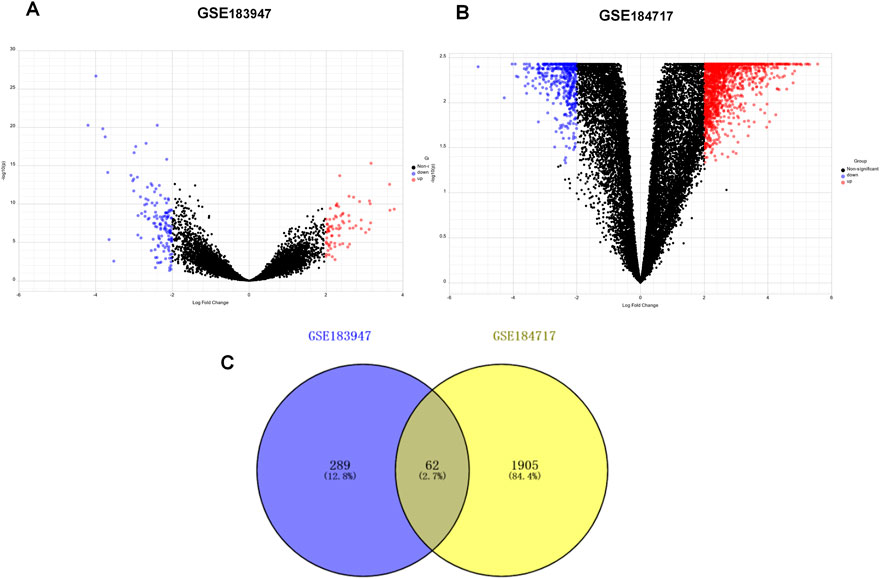
FIGURE 2. Identification of differentially expressed genes (DEGs). (A) Volcano plot of DEGs between primary tumor and paired normal tumor in GSE183947. (B) Volcano plot of DEGs between metastasis tumor and paired normal tumor in GSE184717. (C) Venn diagram showing the intersection of the DEGs in GSE 183947 and GSE 184717.
Two genes were screened out as potential predictive biomarkers
The clinicopathologic characteristics are summarized in Table 1. To identify DEGs that are associated with metastasis, univariate Cox regression analysis was performed. A total of nine candidate genes (CD74, TSPAN7, COL11A1, FLG, MMP11, CHRDL1, FNDC1, MELK, PITX1) with an adjusted p < 0.05 were screened out (Figure 3A). Detailed information for each gene was listed in Table 2.
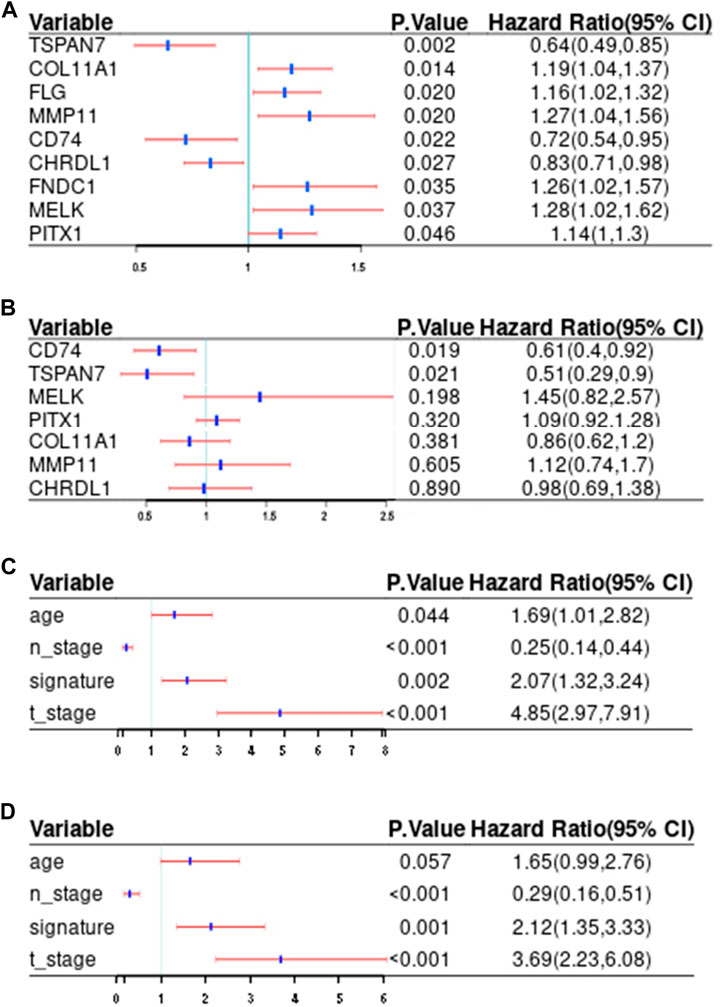
FIGURE 3. Screening of potential prediction biomarkers and risk factors for distant metastasis. (A, B) Univariate (A) and multivariate (B) Cox regression analysis of the metastasis-related genes. (C, D) Univariate (C) and multivariate (D) Cox regression analysis of correlations between the signature and clinical traits. Red and blue, respectively indicate 95% confidence interval and hazard ratio. T_stage, tumor size; N_stage, nodal status; Signature, the risk score.
We further analyzed the differential gene expression between BC tissues (n = 1,085) and normal tissues (n = 291) using GEPIA. As shown in Figure 4, eight genes were found to be differentially expressed in BC tissues, including six upregulated genes (CD74,MMP11,MELK,COL11A1,PITX1,FNDC1) and two downregulated genes (TSPAN7, CHRDL1).
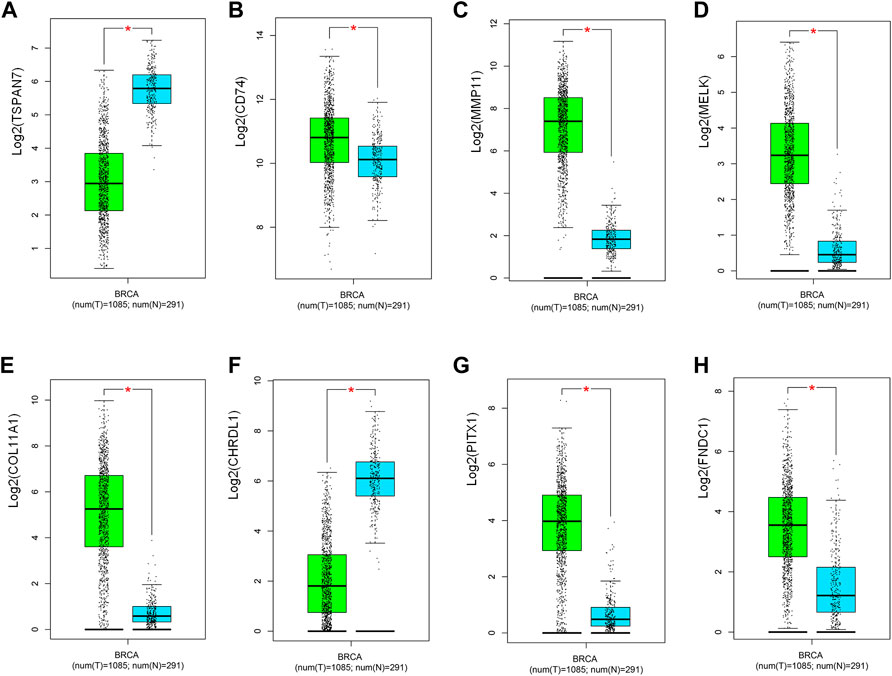
FIGURE 4. Validation of the expression of potential predictive biomarker in TCGA and GETx (n = 1,376). (A) TSPAN7, (B) CD74, (C) MMP11, (D) MELK, (E) COL11A1, (F) CHRDL1, (G) PITX1, (H) FNDC1. T: tumor; N: normal. Green and blue, respectively indicate tumor and normal groups. *p < 0.05.
Interestingly, breast tissues with low CD74 expression were related to poorer DMFS although CD74 commonly upregulated in BC patients.
Additionally, bc-GenExMiner was utilized to validate the relationship between predictive biomarkers and DMFS. The expression levels of seven genes, including TSPAN7(HR:0.75; 95%CI:0.69-0.83; p < 0.0001), CD74(HR:0.88; 95%CI:0.81-0.97; p = 0.0094), MMP11 (HR:1.33; 95%CI:1.21-1.46; p < 0.0001), MELK(HR:1.87; 95%CI:1.70-2.06; p < 0.0001), COL11A1(HR:1.13; 95%CI:1.03-1.24; p = 0.0108), CHRDL1(HR:0.81; 95%CI:0.73-0.89; p < 0.0001), PITX1(HR:1.17; 95%CI:1.07-1.29; p = 0.0010), were found able to estimate DMFS (Figure 5).
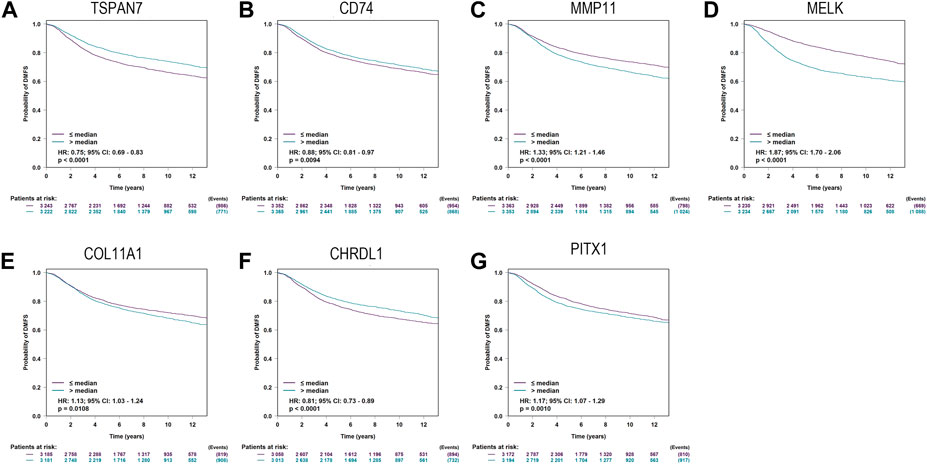
FIGURE 5. Kaplan-Meier curves for Distant metastasis-free survival (DMFS) of TSPAN7 (A), CD74 (B), MMP11 (C), MELK (D), COL11A1 (E), CHRDL1 (F), and PITX1 (G)in breast cancer patients using bc-GenExMiner.
Finally, CD74 (HR:0.61; 95%CI:0.4-0.92; p = 0.019) and TSPAN7(HR:0.51; 95%CI:0.29-0.9; p = 0.021) were screened out to develop a signature according to the results of multivariate Cox analysis (Figure 3B).
Development and validation of the signature
We calculated the signature based on the expression of CD74 and TSPAN7 as follows:
Signature = (0.7,697,433 X expression of CD74) + (0.6633031 X expression of TSPAN7).
Of note, the expression of CD74 and TSPAN7 was negatively correlated with DMFS, suggesting that patients with low scores have a higher probability of distant metastasis.
To be better applied in clinical diagnosis, a constant cutoff value was determined by the median of the training cohort (15.488). Survival analysis, using the Kaplan-Meier method, indicated that the low score group portends a worse DMFS (HR:0.63; 95%CI:0.49-0.82; p = 0.01; Figure 6A). Subsequently, survival analysis was performed twice with the same results in the internal validation cohort (HR:0.58; 95%CI:0.36-0.96; p = 0.023; Figure 6B), and the external validation cohort (HR:0.67; 95%CI:0.47-0.95; p = 0.0065; Figure 6C), respectively. To individually show the differences between low score and high score groups, we visualized the scores, distant metastasis, and gene expression profiles in the three cohorts (Figures 6D–F). The above results indicated that the signature could predict the risk of metastasis development as an independent risk feature.
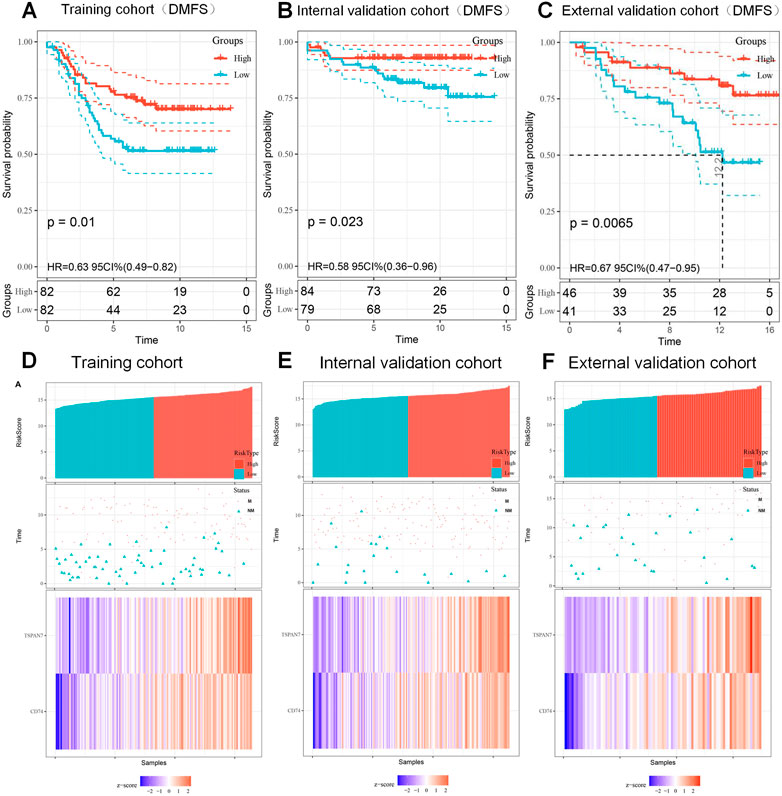
FIGURE 6. Kaplan-Meier curves of DMFS according to signature and the prognosis of patients with BC. (A–C) Kaplan-Meier curves of DMFS based on the signature (15.488) in training cohort (A), internal validation cohort (B) and external validation cohort (C). (D–F) The distribution of risk score (top), metastatic status (middle) and expression heatmap (bottom) of the two biomarkers in training cohort (D), internal validation cohort (E) and external validation cohort (F). M, metastasized; NM, unmetastasized.
Gene set enrichment analysis
GSEA was performed to analyze the causes of BC metastasis risk difference between high and low score groups. Figure 7A showed that upregulated genes in the high score group were significantly enriched in immune related signal pathways, such as T cell receptor signaling and chemokine signaling pathway, suggesting that our signature may be an immune infection related signature (IIRS).
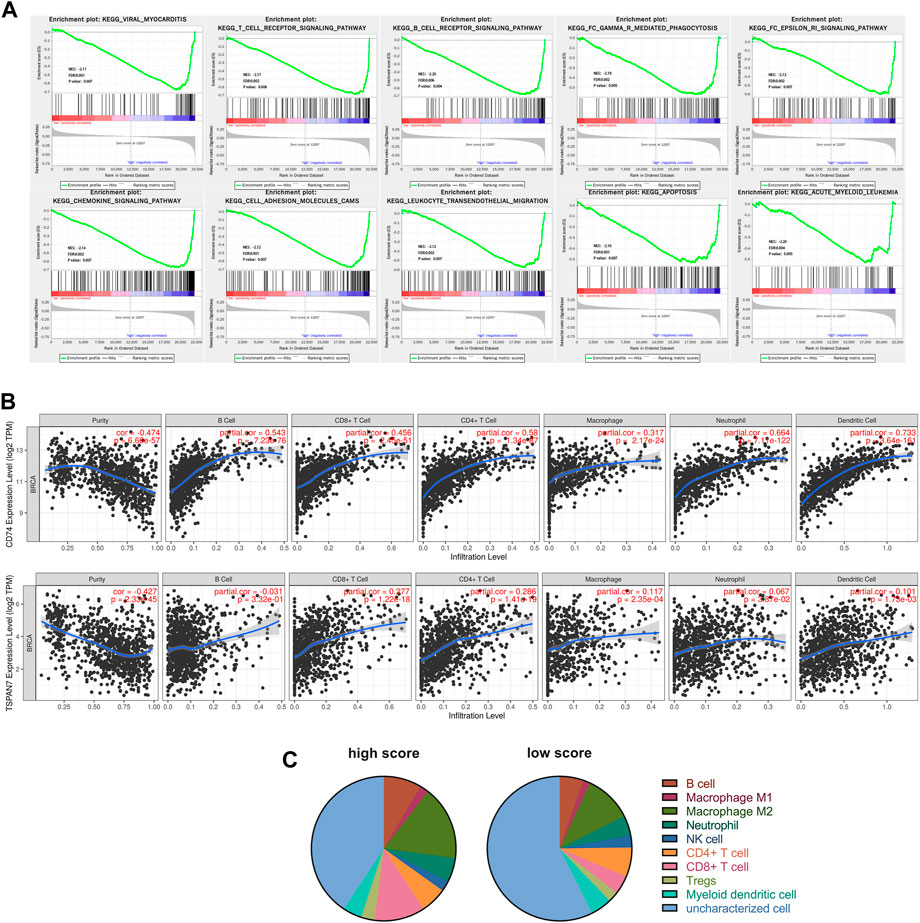
FIGURE 7. Functional analysis of the CD74 and TSPAN7. (A) GSEA identified gene sets significantly enriched in the phenotype of high score patients based on the IIRS. (B) Correlations between CD74 or TSPAN7 expression and immune infiltration levels. (C) Different levels of immune infiltration were observed between the high score (n = 212) and the low score (n = 202) groups.
Immune infiltration analysis
We further utilized TIMER to analyze the possible correlation between CD74, TSPAN7 expression and levels of immune infiltration in BC (Figure 7B). Both CD74 and TSPAN7 are associated with multiple immune cell infiltration, especially T cells. Therefore, we want to know whether the correlation between the signature and immune infiltration is applicable to all our cohorts. We integrated three cohorts and calculated the fraction of immune cell subsets using quanTIseq. As shown in Figure 7C, the high score group had a higher fraction of B cells (p < 0.001), M2 Macrophage (p < 0.001), Neutrophil (p = 0.009), CD8+T cell (p < 0.001), Tregs (p < 0.001). On the contrary, the fraction of NK cell (p = 0.012) and uncharacterized cells were found to increase significantly in the low score group. It is worth noting that the analysis showed that there was the most significant difference in CD8+T cells between groups.
In view of myeloid cells playing an important role in the recruitment and concentration of CD8+ T cells (Jiang et al., 2022), we attempted to evaluate the correlation between myeloid cell levels and the IIRS in BC patients. CD33 is widely used as a marker for tumor infiltrating myeloid cells (Choi et al., 2020; Toor et al., 2021), so we collected FFPEs from 28 consecutive pairs of BC patients and performed IHC for CD74 and CD33, respectively. Interestingly, CD74 protein mainly existed in some CD33+ cells (Figure 8A), and was positively correlated with CD33 levels in three cohorts (Figure 8B), IHC (Figure 8C) and TCGA (Figure 8D), respectively. In addition, there is a significant positive correlation between CD74 and CD8 in BC (Figure 8E), which means that CD74+ myeloid cells will not affect the recruitment of CD8+T cells.
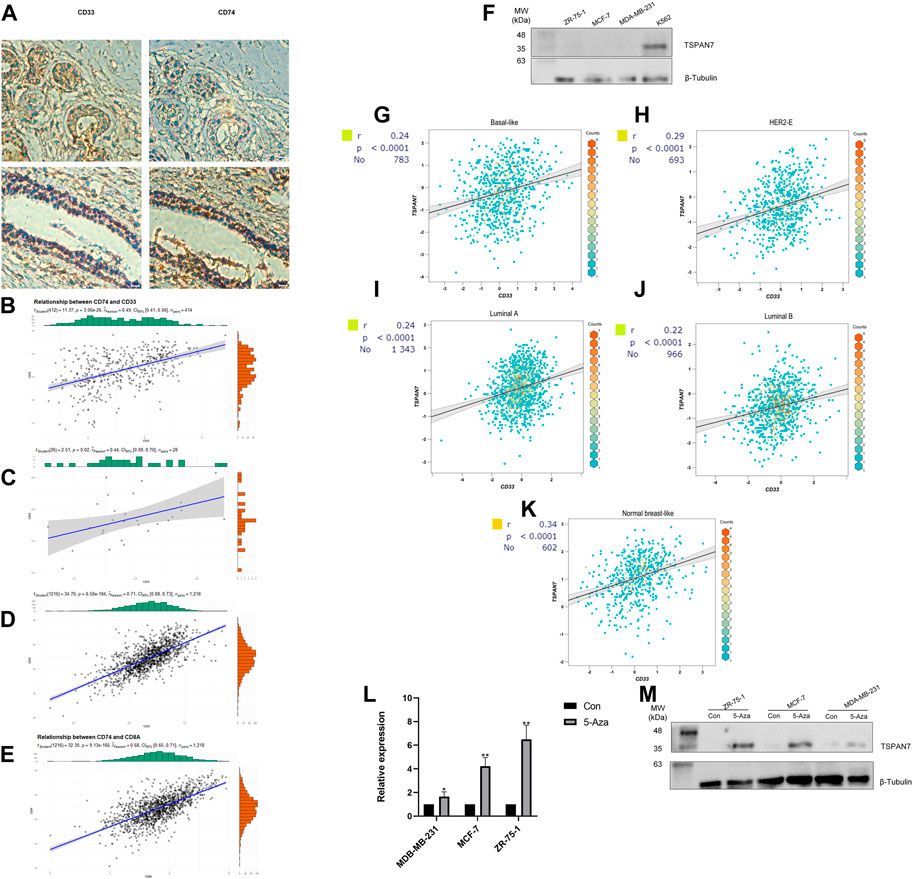
FIGURE 8. Gene expression and correlation. (A) IHC for CD74 and myeloid marker CD33. (B–D) Correlation between the expression of CD74 and CD33 in cohorts (Panel B), IHC (Panel C) and TCGA (Panel D) (Pearson correlation coefficient). (E) Correlation between the expression of CD74 and CD8A in TCGA. (F) Western blot analysis of TSPAN7 in cell lysates of myeloid cells and breast cancer cells. (G–K) Correlation between CD33 and TSPAN7 expression of different PAM50 subtypes in bc-GenExMiner. Basal-like subtype (G); HER2-enriched subtype (H); Luminal A subtype (I); Luminal B subtype (J); Nromal-like subtype (K). (L, M) TSPAN7 mRNA (L) and protein (M) expression was upregulated after treatment with the demethylation reagent 5-Aza. *p < 0.05,**p < 0.01, by Student’s t-test.
We detected TSPAN7 protein expression in three common BC cell lines (MCF-7, ZR-75-1, MDA-MB-231) and a myeloid cell line K562 (Figure 8F). TSPAN7 was almost undetectable in BC cell lines, contrary to the myeloid cell line. Importantly, we found that the expression of TSPAN7 was significantly correlated with CD33 in a variety of molecular subtypes, which has been proven to exist mainly in myeloid immune cells (Figures 8G–K). It suggested that the differential expression of TSPAN7 may affect the prognosis by reflecting the infiltration of myeloid cells. However, TSPAN7 expression in BC tissues is lower than that in normal tissues without immune invasion. To explain this paradoxical phenomenon, we hypothesized that TSPAN7 methylation occured and treated three TSPAN7-silenced cells with demethylation agent 5-Aza, respectively. TSPAN7 expression was subsequently restored in all treated BC cells (Figures 8L,M). TSPAN7 methylation in BC cells and myeloid cells infiltration together result in the difference of TSPAN7 expression.
Construction and assessment of predictive nomogram
In order to increase clinical utility, a predictive nomogram was constructed (Figure 9) based on the risk feature verified by univariate and multivariate Cox analysis, including the IIRS, age, T stage, and N stage (Figures 3C,D). Interestingly, we found that younger patients are at higher risk of metastasis, which was consistent with previous findings (Purushotham et al., 2014).
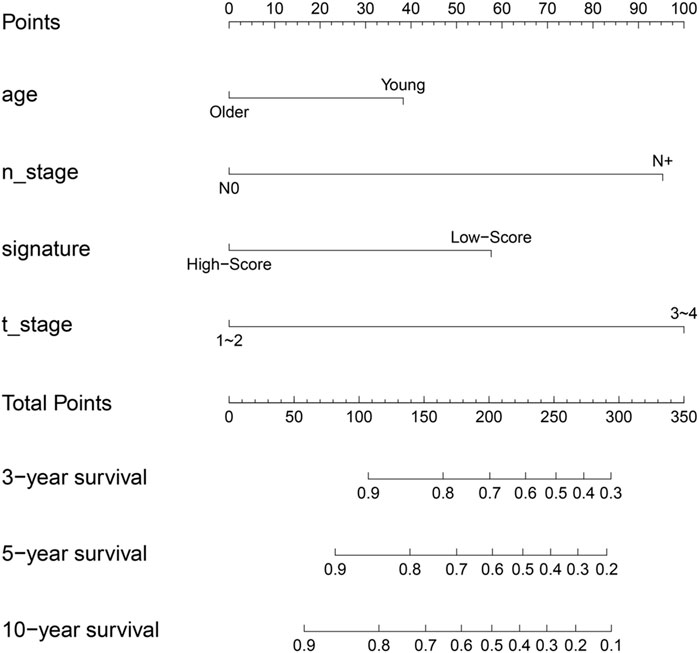
FIGURE 9. Nomogram to predict for 3-year,5-year and 10-year probabilities of DMFS for breast cancer patients. Age: older: ≥60,young: <60; T_stage: 0: T0; 1: T1; 2: T2; 3: T3; 4: T4; N_stage: 0: N0; N+: N1, N2, N3; signature, IIRS.
Subsequently, calibrate curves were plotted to identify the consistency between ideal outcome and actual observation in prediction of 3-,5- 10-year distant metastasis-free survival times. The calibration curves showed good performance in training cohort (Figure 10A), internal validation cohort (Figure 10B), and external validation cohort (Figure 10C), especially for long term survival rate (10-year DMFS).
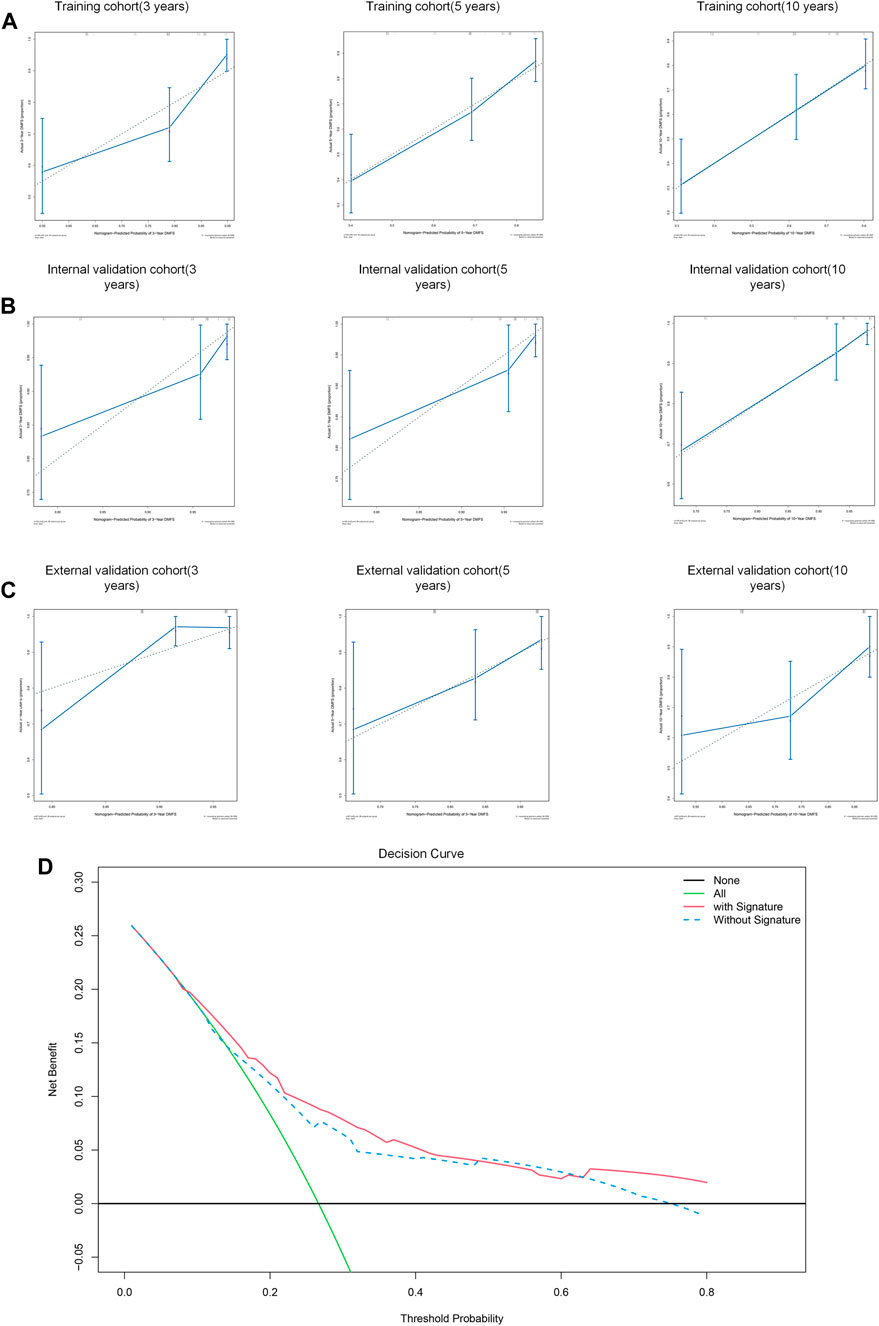
FIGURE 10. Performance of the nomogram to predict DMFS. (A–C) Calibration curves of 3-year, 5-year and 10-year DMFS in training cohort (A), internal validation cohort (B), and external validation cohort (C). (D) Decision curve analysis for the nomogram with/without signature.
To assess the predictive performance of this model, the nomogram was internally validated by 1,000 bootstrap resamples in three cohorts. Pleasantly, and surprisingly, the nomogram yielded a C-index of 0.742 (95% CI, 0.715–0.769) for training cohort, 0.801 (95% CI, 0.754 to 0.0.848) for internal validation cohort and 0.695 (95% CI, 0.643 to 0.0.747) for external validation cohort. Therefore, we demonstrated that the nomogram could predict the DMFS of BC patients effectively.
We subsequently performed a decision curve analysis to evaluate the clinical net benefit in predicting the probability of 10- year DMFS. As shown in Figure 10D, if the threshold probability of a patient or doctor is <48% or >63%, using the nomogram with IIRS to predict DMFS adds more benefit than the other without IIRS.
Discussion
It is well known that almost all metastatic BC has poor overall survival and incurable nature (Gobbini et al., 2018). In recent years, it is gratifying to note that the median survival time after diagnosis of metastatic BC has been increasing due to improved treatment (Mariotto et al., 2017). Therefore, early assessment and diagnosis of distant metastasis are still meaningful strategies for improving the prognosis of BC patients.
Nowadays multiple biomarkers, such as circulating miRNA (Baldasici et al., 2022), circulating tumor DNA (Page et al., 2021) and circulating tumor cell (Ring et al., 2022), can be used to detect metastasis of BC. What’s more, several serum biomarkers related to metastasis, including soluble POSTN (Jia et al., 2022), PTHrP (Washam et al., 2013), S100P (Peng et al., 2016), have been found. Non-etheless, when these biomarkers increase, it is very likely that BC has undergone distant metastasis (Al-Mahmood et al., 2018). Therefore, improved methods based on gene expression to predict the risk of BC metastasis in advance are needed.
Unfortunately, there is no effective way to achieve this goal. Although PAM50 signature has proven to be able to provide prognostic information from the lymph node metastasis of BC patients, only a few subtypes are associated with metastasis (Tobin et al., 2017; Wang et al., 2019), suggesting that individualized accurate prediction according to PAM50 subtypes is still difficult.
In this study, we sought to identify metastasis-related genes to predict the probability of distant metastasis in BC patients. A total of 62 genes were screened out in GSE184717 and GSE183947.7 genes (CD74,TSPAN7,COL11A1, MMP11, CHRDL1, MELK, PITX1) proved to be associated with DMFS in BC patients, and seven of them (TSPAN7, CD74, MMP11, MELK, COL11A1, CHRDL1, PITX1) were verified by bc-GenExMiner. Two potential biomarkers (CD74, TSPAN7) of metastasis development were used to construct a gene signature by multivariate analysis. The signature proved to be associated with distant metastasis–free survival in three cohort.
The CD74 gene, responsible for producing a protein associate with class II major histocompatibility complex (MHC) is implicated in an effective intratumor immune response (Wang et al., 2017). It also serves as a cell surface receptor for the cytokine macrophage migration inhibitory factor, which may play a pro-oncogenic role in promoting BC cell-stroma interactions (Verjans et al., 2009). Of note, CD74 was observed to be related to triple-negative breast cancer, which is the most aggressive subtype of breast cancer (Tian et al., 2012). The TSPAN7 is a cell-surface protein coding gene, and the coding protein of which plays a role in the regulation of cell development, activation, growth and motility (Perot and Ménager, 2020). For this reason, TSPAN7 is also known as CD231. Our study is the first to link CD74 and TSPAN7 expression with distant metastasis–free survival in breast cancer, highlighting gene signature based on CD74 and TSPAN7 as a predictor of metastasis development in BC, with a strong effect on patients’ distant metastasis–free survival.
In past studies, multiple prognostic models have been constructed because of the differential expression of CD74 (Wang et al., 2020) or TSPAN7 (Wu et al., 2020) between tumor and normal tissues. However, the reason for this difference is still unclear. As a result, much effort has been expended in trying to explore differential expression of CD74 or TSPAN7 in cancer cell strains, ignoring the influence of immune infiltration (Greenwood et al., 2012; Wang et al., 2018; Qi et al., 2020; Yu et al., 2021). Notably, heterogeneity of CD74 expression has been confirmed in tumor tissues (Richard et al., 2014), indicating that characterization of immune landscape cannot be discounted. In this study, GSEA revealed a statistical enrichment of immune-related signaling pathways in the high score group. Thus we speculated that the signature we constructed may reveal the levels of immune infiltration. The results of TIMER database confirmed this conjecture. CD74 and TSPAN7 expression was negatively correlated with tumor purity while positively correlated with the level of multiple immune cells. Furthermore, the fraction of immune cells in high score group was significantly higher than that in low score group, especially CT8+ T cells. According to the results presented above, we identified a novel cause of differential expression of CD74 and TSPAN7. The levels of CD74 and TSPAN7 reflected the ability of immune cells to infiltrate tumors. To the best of our knowledge, this is the first study to show that high CD74 and TSPAN7 expression is associated with tumor-infiltrating immune cells. Further studies on Intra-tumoral heterogeneity are warranted, in order to analyze the relationship between survival time and the level of immune cell infiltration.
Our study has some potential limitations. First, pathologic features were unavailable, so the correlation between our signature and pathological features could not be evaluated. Second, quanTIseq is a prediction tool of immune cell fraction based on deconvolution algorithm, as a result, it will predict a minimal amount of immune cells even though they are absent (Sturm et al., 2019).
In conclusion, we identified seven genes related to distant metastasis–free survival, namely, TSPAN7, CD74, MMP11, MELK, COL11A1, CHRDL1, PITX1, and a signature based on CD74 and TSPAN7 expression that may have potential of predicting DMFS. We found that methylation of TSPAN7 in BC cells inhibits the recruitment of CD74 positive immune cells, which may be associated with a lower risk of metastasis. The present study was the first to propose that high CD74 expression may be derived from tumor-infiltrating immune cells. Given the favorable discrimination of the signature, we developed a nomogram for clinical applications. This is the first nomogram based on gene signature that can be used to facilitate the individualized prediction of DMFS in BC patients.
Data availability statement
The original contributions presented in the study are included in the article/supplementary material, further inquiries can be directed to the corresponding authors.
Ethics statement
The studies involving human participants were reviewed and approved by the Ethnic Committee of Shunde Hospital of Guangzhou University of Chinese Medicine. Written informed consent for participation was not required for this study in accordance with the national legislation and the institutional requirements.
Author contributions
All authors listed have made direct intellectual contributions to the work, and approved it for its publication. CR made a substantial contribution when drafting the manuscript.
Funding
This work was supported by the basic and applied basic research program of Guangdong province (2019A1515011960); . This research was also supported by the Guangzhou Science and Technology Plan Project (202102010178).
Acknowledgments
Special thanks to the researchers that collected, curated, and maintain the publicly database GEPIA and bc-GenExMiner.
Conflict of interest
The reviewer MY declared a shared parent affiliation with the author(s) DL to the handling editor at the time of review.
The authors declare that the research was conducted in the absence of any commercial or financial relationships that could be construed as a potential conflict of interest.
Publisher’s note
All claims expressed in this article are solely those of the authors and do not necessarily represent those of their affiliated organizations, or those of the publisher, the editors and the reviewers. Any product that may be evaluated in this article, or claim that may be made by its manufacturer, is not guaranteed or endorsed by the publisher.
References
Al-Mahmood, S., Sapiezynski, J., Garbuzenko, O. B., and Minko, T. (2018). Metastatic and triple-negative breast cancer: Challenges and treatment options. Drug Deliv. Transl. Res. 8 (5), 1483–1507. doi:10.1007/s13346-018-0551-3
Balachandran, V. P., Gonen, M., Smith, J. J., and DeMatteo, R. P. (2015). Nomograms in oncology: More than meets the eye. Lancet Oncol. 16 (4), e173–e180. doi:10.1016/S1470-2045(14)71116-7
Baldasici, O., Pileczki, V., Cruceriu, D., Gavrilas, L. I., Tudoran, O., Balacescu, L., et al. (2022). Breast cancer-delivered exosomal miRNA as liquid biopsy biomarkers for metastasis prediction: A focus on translational research with clinical applicability. Int. J. Mol. Sci. 23 (16), 9371. doi:10.3390/ijms23169371
Chen, W., Hoffmann, A. D., Liu, H., and Liu, X. (2018). Organotropism: New insights into molecular mechanisms of breast cancer metastasis. NPJ Precis. Oncol. 2 (1), 4. doi:10.1038/s41698-018-0047-0
Choi, J. W., Kim, Y. J., Yun, K. A., Won, C. H., Lee, M. W., Choi, J. H., et al. (2020). The prognostic significance of VISTA and CD33-positive myeloid cells in cutaneous melanoma and their relationship with PD-1 expression. Sci. Rep. 10 (1), 14372. doi:10.1038/s41598-020-71216-2
Ellis, M. J., Suman, V. J., Hoog, J., Lin, L., Snider, J., Prat, A., et al. (2011). Randomized phase II neoadjuvant comparison between letrozole, anastrozole, and exemestane for postmenopausal women with estrogen receptor-rich stage 2 to 3 breast cancer: Clinical and biomarker outcomes and predictive value of the baseline PAM50-based intrinsic subtype--ACOSOG Z1031. J. Clin. Oncol. 29 (17), 2342–2349. doi:10.1200/JCO.2010.31.6950
Filipits, M., Nielsen, T. O., Rudas, M., Greil, R., Stöger, H., Jakesz, R., et al. (2014). The PAM50 risk-of-recurrence score predicts risk for late distant recurrence after endocrine therapy in postmenopausal women with endocrine-responsive early breast cancer. Clin. Cancer Res. 20 (5), 1298–1305. doi:10.1158/1078-0432.CCR-13-1845
Finotello, F., Mayer, C., Plattner, C., Laschober, G., Rieder, D., Hackl, H., et al. (2019). Molecular and pharmacological modulators of the tumor immune contexture revealed by deconvolution of RNA-seq data. Genome Med. 11 (1), 34. doi:10.1186/s13073-019-0638-6
Gnant, M., Filipits, M., Greil, R., Stoeger, H., Rudas, M., Bago-Horvath, Z., et al. (2014). Predicting distant recurrence in receptor-positive breast cancer patients with limited clinicopathological risk: Using the PAM50 risk of recurrence score in 1478 postmenopausal patients of the ABCSG-8 trial treated with adjuvant endocrine therapy alone. Ann. Oncol. 25 (2), 339–345. doi:10.1093/annonc/mdt494
Gobbini, E., Ezzalfani, M., Dieras, V., Bachelot, T., Brain, E., Debled, M., et al. (2018). Time trends of overall survival among metastatic breast cancer patients in the real-life ESME cohort. Eur. J. Cancer 96, 17–24. doi:10.1016/j.ejca.2018.03.015
Greenwood, C., Metodieva, G., Al-Janabi, K., Lausen, B., Alldridge, L., Leng, L., et al. (2012). Stat1 and CD74 overexpression is co-dependent and linked to increased invasion and lymph node metastasis in triple-negative breast cancer. J. Proteomics 75 (10), 3031–3040. doi:10.1016/j.jprot.2011.11.033
Harrell, F. E., Lee, K. L., and Mark, D. B. (1996). Multivariable prognostic models: Issues in developing models, evaluating assumptions and adequacy, and measuring and reducing errors. Stat. Med. 15 (4), 361–387. doi:10.1002/(SICI)1097-0258(19960229)15:4<361::AID-SIM168>3.0.CO;2-4
Huang, Y. Q., Liang, C. H., He, L., Tian, J., Liang, C. S., Chen, X., et al. (2016). Development and validation of a radiomics nomogram for preoperative prediction of lymph node metastasis in colorectal cancer. J. Clin. Oncol. 34 (18), 2157–2164. doi:10.1200/JCO.2015.65.9128
Huang, Z. Z., Hua, X., Song, C. G., Xia, W., Bi, X. W., Yuan, Z. Y., et al. (2020). The prognostic prediction value of systemic inflammation score and the development of a nomogram for patients with surgically treated breast cancer. Front. Oncol. 10, 563731. doi:10.3389/fonc.2020.563731
Jézéquel, P., Campone, M., Gouraud, W., Guérin-Charbonnel, C., Leux, C., Ricolleau, G., et al. (2012). bc-GenExMiner: an easy-to-use online platform for gene prognostic analyses in breast cancer. Breast Cancer Res. Treat. 131 (3), 765–775. doi:10.1007/s10549-011-1457-7
Jia, L., Li, G., Ma, N., Zhang, A., Zhou, Y., Ren, L., et al. (2022). Soluble POSTN is a novel biomarker complementing CA153 and CEA for breast cancer diagnosis and metastasis prediction. BMC Cancer 22 (1), 760. doi:10.1186/s12885-022-09864-y
Jiang, X., Wang, J., Zheng, X., Liu, Z., Zhang, X., Li, Y., et al. (2022). Intratumoral administration of STING-activating nanovaccine enhances T cell immunotherapy. J. Immunother. Cancer 10 (5), e003960. doi:10.1136/jitc-2021-003960
Johnson, W. E., Li, C., and Rabinovic, A. (2007). Adjusting batch effects in microarray expression data using empirical Bayes methods. Biostatistics 8 (1), 118–127. doi:10.1093/biostatistics/kxj037
Li, T., Fan, J., Wang, B., Traugh, N., Chen, Q., Liu, J. S., et al. (2017). Timer: A web server for comprehensive analysis of tumor-infiltrating immune cells. Cancer Res. 77 (21), e108–e110. doi:10.1158/0008-5472.CAN-17-0307
Liang, Y., Zhang, H., Song, X., and Yang, Q. (2020). Metastatic heterogeneity of breast cancer: Molecular mechanism and potential therapeutic targets. Semin. Cancer Biol. 60, 14–27. doi:10.1016/j.semcancer.2019.08.012
Mariotto, A. B., Etzioni, R., Hurlbert, M., Penberthy, L., and Mayer, M. (2017). Estimation of the number of women living with metastatic breast cancer in the United States. Cancer Epidemiol. Biomarkers Prev. 26 (6), 809–815. doi:10.1158/1055-9965.EPI-16-0889
Masuda, H., Baggerly, K. A., Wang, Y., Zhang, Y., Gonzalez-Angulo, A. M., Meric-Bernstam, F., et al. (2013). Differential response to neoadjuvant chemotherapy among 7 triple-negative breast cancer molecular subtypes. Clin. Cancer Res. 19 (19), 5533–5540. doi:10.1158/1078-0432.CCR-13-0799
Medeiros, B., and Allan, A. L. (2019). Molecular mechanisms of breast cancer metastasis to the lung: Clinical and experimental perspectives. Int. J. Mol. Sci. 20 (9), 2272. doi:10.3390/ijms20092272
Page, K., Martinson, L. J., Fernandez-Garcia, D., Hills, A., Gleason, K. L. T., Gray, M. C., et al. (2021). Circulating tumor DNA profiling from breast cancer screening through to metastatic disease. JCO Precis. Oncol. 5, 1768–1776. doi:10.1200/PO.20.00522
Peng, C., Chen, H., Wallwiener, M., Modugno, C., Cuk, K., Madhavan, D., et al. (2016). Plasma S100P level as a novel prognostic marker of metastatic breast cancer. Breast Cancer Res. Treat. 157 (2), 329–338. doi:10.1007/s10549-016-3776-1
Perot, B. P., and Ménager, M. M. (2020). Tetraspanin 7 and its closest paralog tetraspanin 6: Membrane organizers with key functions in brain development, viral infection, innate immunity, diabetes and cancer. Med. Microbiol. Immunol. 209 (4), 427–436. doi:10.1007/s00430-020-00681-3
Prat, A., Pineda, E., Adamo, B., Galván, P., Fernández, A., Gaba, L., et al. (2015). Clinical implications of the intrinsic molecular subtypes of breast cancer. Breast 24 (2), S26–S35. doi:10.1016/j.breast.2015.07.008
Purushotham, A., Shamil, E., Cariati, M., Agbaje, O., Muhidin, A., Gillett, C., et al. (2014). Age at diagnosis and distant metastasis in breast cancer--a surprising inverse relationship. Eur. J. Cancer 50 (10), 1697–1705. doi:10.1016/j.ejca.2014.04.002
Qi, Y., Li, H., Lv, J., Qi, W., Shen, L., Liu, S., et al. (2020). Expression and function of transmembrane 4 superfamily proteins in digestive system cancers. Cancer Cell Int. 20, 314. doi:10.1186/s12935-020-01353-1
Richard, V., Kindt, N., Decaestecker, C., Gabius, H. J., Laurent, G., Noel, J. C., et al. (2014). Involvement of macrophage migration inhibitory factor and its receptor (CD74) in human breast cancer. Oncol. Rep. 32 (2), 523–529. doi:10.3892/or.2014.3272
Ring, A., Campo, D., Porras, T. B., Kaur, P., Forte, V. A., Tripathy, D., et al. (2022). Circulating tumor cell transcriptomics as biopsy surrogates in metastatic breast cancer. Ann. Surg. Oncol. 29 (5), 2882–2894. doi:10.1245/s10434-021-11135-2
Ritchie, M. E., Phipson, B., Wu, D., Hu, Y., Law, C. W., Shi, W., et al. (2015). Limma powers differential expression analyses for RNA-sequencing and microarray studies. Nucleic Acids Res. 43 (7), e47. doi:10.1093/nar/gkv007
Rouzier, R., Pusztai, L., Delaloge, S., Gonzalez-Angulo, A. M., Andre, F., Hess, K. R., et al. (2005). Nomograms to predict pathologic complete response and metastasis-free survival after preoperative chemotherapy for breast cancer. J. Clin. Oncol. 23 (33), 8331–8339. doi:10.1200/JCO.2005.01.2898
Sturm, G., Finotello, F., Petitprez, F., Zhang, J. D., Baumbach, J., Fridman, W. H., et al. (2019). Comprehensive evaluation of transcriptome-based cell-type quantification methods for immuno-oncology. Bioinformatics 35 (14), i436–i445. doi:10.1093/bioinformatics/btz363
Subramanian, A., Tamayo, P., Mootha, V. K., Mukherjee, S., Ebert, B. L., Gillette, M. A., et al. (2005). Gene set enrichment analysis: A knowledge-based approach for interpreting genome-wide expression profiles. Proc. Natl. Acad. Sci. U. S. A. 102 (43), 15545–15550. doi:10.1073/pnas.0506580102
Sung, H., Ferlay, J., Siegel, R. L., Laversanne, M., Soerjomataram, I., Jemal, A., et al. (2021). Global cancer statistics 2020: GLOBOCAN estimates of incidence and mortality worldwide for 36 cancers in 185 countries. CA Cancer J. Clin. 71 (3), 209–249. doi:10.3322/caac.21660
Tang, Z., Li, C., Kang, B., Gao, G., Li, C., and Zhang, Z. (2017). GEPIA: A web server for cancer and normal gene expression profiling and interactive analyses. Nucleic Acids Res. 45 (W1), W98–W102. doi:10.1093/nar/gkx247
Tian, B., Zhang, Y., Li, N., Liu, X., and Dong, J. (2012). CD74: A potential novel target for triple-negative breast cancer. Tumour Biol. 33 (6), 2273–2277. doi:10.1007/s13277-012-0489-x
Tobin, N. P., Lundberg, A., Lindström, L. S., Harrell, J. C., Foukakis, T., Carlsson, L., et al. (2017). PAM50 provides prognostic information when applied to the lymph node metastases of advanced breast cancer patients. Clin. Cancer Res. 23 (23), 7225–7231. doi:10.1158/1078-0432.ccr-17-2301
Toor, S. M., Taha, R. Z., Sasidharan Nair, V., Saleh, R., Murshed, K., Abu Nada, M., et al. (2021). Differential gene expression of tumor-infiltrating CD33+ myeloid cells in advanced-versus early-stage colorectal cancer. Cancer Immunol. Immunother. 70 (3), 803–815. doi:10.1007/s00262-020-02727-0
Verjans, E., Noetzel, E., Bektas, N., Schütz, A. K., Lue, H., Lennartz, B., et al. (2009). Dual role of macrophage migration inhibitory factor (MIF) in human breast cancer. BMC Cancer 9, 230. doi:10.1186/1471-2407-9-230
Wang, J., Yang, Z., Zhang, C., Ouyang, J., Zhang, G., and Wu, C. (2020). A four-gene signature in the tumor microenvironment that significantly associates with the prognosis of patients with breast cancer. Gene 761, 145049. doi:10.1016/j.gene.2020.145049
Wang, X., Lin, M., Zhao, J., Zhu, S., Xu, M., and Zhou, X. (2018). TSPAN7 promotes the migration and proliferation of lung cancer cells via epithelial-to-mesenchymal transition. Onco Targets Ther. 11, 8815–8822. doi:10.2147/OTT.S167902
Wang, Y., Yang, Y., Chen, Z., Zhu, T., Wu, J., Su, F., et al. (2019). Development and validation of a novel nomogram for predicting distant metastasis-free survival among breast cancer patients. Ann. Transl. Med. 7 (20), 537. doi:10.21037/atm.2019.10.10
Wang, Z. Q., Milne, K., Webb, J. R., and Watson, P. H. (2017). CD74 and intratumoral immune response in breast cancer. Oncotarget 8 (8), 12664–12674. doi:10.18632/oncotarget.8610
Washam, C. L., Byrum, S. D., Leitzel, K., Ali, S. M., Tackett, A. J., Gaddy, D., et al. (2013). Identification of PTHrP(12-48) as a plasma biomarker associated with breast cancer bone metastasis. Cancer Epidemiol. Biomarkers Prev. 22 (5), 972–983. doi:10.1158/1055-9965.EPI-12-1318-T
Wu, M., Li, X., Liu, R., Yuan, H., Liu, W., and Liu, Z. (2020). Development and validation of a metastasis-related gene signature for predicting the overall survival in patients with pancreatic ductal adenocarcinoma. J. Cancer 11 (21), 6299–6318. doi:10.7150/jca.47629
Keywords: distant metastasis, gene signature, nomogram, immune infiltration, myeloid cells
Citation: Ren C, Gao A, Fu C, Teng X, Wang J, Lu S, Gao J, Huang J, Liu D and Xu J (2023) The biomarkers related to immune infiltration to predict distant metastasis in breast cancer patients. Front. Genet. 14:1105689. doi: 10.3389/fgene.2023.1105689
Received: 23 November 2022; Accepted: 10 February 2023;
Published: 22 February 2023.
Edited by:
Bin Fang, The First Affiliated Hospital of Guangzhou University of Chinese Medicine, ChinaReviewed by:
Jieqiong Tan, Central South University, ChinaMaojin Yao, First Affiliated Hospital of Guangzhou Medical University, China
Copyright © 2023 Ren, Gao, Fu, Teng, Wang, Lu, Gao, Huang, Liu and Xu. This is an open-access article distributed under the terms of the Creative Commons Attribution License (CC BY). The use, distribution or reproduction in other forums is permitted, provided the original author(s) and the copyright owner(s) are credited and that the original publication in this journal is cited, in accordance with accepted academic practice. No use, distribution or reproduction is permitted which does not comply with these terms.
*Correspondence: Dongdong Liu, MTM3MTAzMTIwMjRAMTYzLmNvbQ==; Jianhua Xu, amh4dTE5NzZAZ3p1Y20uZWR1LmNu
†These authors have contributed equally to this work