- 1College of Animal Science and Technology, Sichuan Agricultural University, Chengdu, China
- 2Farm Animal Genetic Resources Exploration and Innovation Key Laboratory of Sichuan Province, Sichuan Agricultural University, Chengdu, China
As Drosophila is an extensively used genetic model system, understanding of its regulatory networks has great significance in revealing the genetic mechanisms of ageing and human diseases. Competing endogenous RNA (ceRNA)-mediated regulation is an important mechanism by which circular RNAs (circRNAs) and long non-coding RNAs (lncRNAs) regulate ageing and age-related diseases. However, extensive analyses of the multiomics (circRNA/miRNA/mRNA and lncRNA/miRNA/mRNA) characteristics of adult Drosophila during ageing have not been reported. Here, differentially expressed circRNAs and microRNAs (miRNAs) between 7 and 42-day-old flies were screened and identified. Then, the differentially expressed mRNAs, circRNAs, miRNAs, and lncRNAs between the 7- and 42-day old flies were analysed to identify age-related circRNA/miRNA/mRNA and lncRNA/miRNA/mRNA networks in ageing Drosophila. Several key ceRNA networks were identified, such as the dme_circ_0009500/dme_miR-289-5p/CG31064, dme_circ_0009500/dme_miR-289-5p/frizzled, dme_circ_0009500/dme_miR-985-3p/Abl, and XLOC_027736/dme_miR-985-3p/Abl XLOC_189909/dme_miR-985-3p/Abl networks. Furthermore, real-time quantitative PCR (qPCR) was used to verify the expression level of those genes. Those results suggest that the discovery of these ceRNA networks in ageing adult Drosophila provide new information for research on human ageing and age-related diseases.
1 Introduction
Drosophila melanogaster is an extensively used genetic model system that has been used for more than 100 years to study various aspects of the life sciences. In particular, fruit flies have been widely utilized to study ageing (Gubina et al., 2019) and human diseases, such as cancer (Enomoto et al., 2018), neurodegenerative disease (Cha et al., 2019), obesity and diabetes (Musselman et al., 2019), sterile inflammation (Nainu et al., 2019), and regeneration (Fox et al., 2020). D. melanogaster has also been utilized to study complex behavioural and developmental biology topics, including exercise (Watanabe and Riddle, 2019), courtship (Liu et al., 2019), and foraging (Khodaei and Long, 2019). Recently, mounting evidence has suggested that Drosophila is an outstanding model for studying ageing and age-related diseases (Surguchov et al., 2019; Brenman-Suttner et al., 2020). Ageing is a physiologic/pathologic process featuring declines in normal physiological functions and progressive impairment of cellular functions (Stallone et al., 2019). The ageing phenomenon has been conserved during biological evolution; even yeast other single-celled eukaryotes experience ageing (He et al., 2019). Thus, in-depth study of the regulatory mechanism of ageing in Drosophila can inform the study of human ageing and disease.
Current studies suggest that non-coding RNAs (ncRNAs) are involved in organismal ageing (Kim and Lee, 2019; Tower, 2019). With the development of sequencing technology, dynamic changes in the transcriptome [including changes in mRNA, long non-coding RNA (lncRNA), microRNA (miRNA), and circular RNA (circRNA) (Yang et al., 2016; Perry et al., 2017; Barter et al., 2019; Kinser and Pincus, 2020); the proteome (Brown et al., 2018); and the metabolome (Song et al., 2017)] have been described in the context of Drosophila ageing. Most miRNAs, mRNAs, and the proteins in fruit flies are evolutionarily conserved up to humans and regulate similar signalling pathways across organisms, such as the NF-κB, AMPK, mTOR, P53, PGC1α, and FoxO pathways (Zha et al., 2019; Kinser and Pincus, 2020; Wang et al., 2020). Large studies have demonstrated that the circRNA/miRNA/mRNA and axis the lncRNA/miRNA/mRNA axis play vital roles in ageing and age-related disease (Ruan et al., 2020; Wang et al., 2020).
As Drosophila is a workhorse model organism, thoroughly studying the characteristics of adult Drosophila from a multiomics perspective is necessary. Understanding how these conserved protein genes regulate ageing through ceRNA mechanisms in Drosophila is important. However, extensive analyses of the multiomics (circRNA/miRNA/mRNA lncRNA/miRNA/mRNA) characteristics of adult Drosophila ageing have not been reported. In the present study, we investigated the circRNA/miRNA/mRNA the lncRNA/miRNA/mRNA axis in adult Drosophila at two age points (day 7 and day 42) and determined the regulatory network of key differentially expressed (DE) genes in Drosophila ageing. The results provide knowledge on the gene regulation network of adult Drosophila ageing and a solid foundation for understanding the mechanisms of human ageing and age-related diseases.
2 Results
2.1 Overview of multiomics data
The DE genes and proteins in Drosophila between day 7 and day 42 were identified, and their networks were analysed (Table 1). A total of 537 DE mRNAs and 43 DE lncRNAs were obtained from a previous study in our laboratory (Supplementary Data Sheet S1). A total of 6,003 circRNAs and 226 miRNAs were identified at day 7 and day 42 (Supplementary Data Sheet S1). Ultimately, 29 DE circRNAs and 24 DE miRNAs were found (Supplementary Data Sheet S1). The merged sequences of novel circRNAs (Supplementary Data Presentation S1–S3) and lncRNAs (Supplementary Data Sheet S3) are shown in supplementary files.
2.2 DE circRNAs and miRNAs in Drosophila between day 7 day 42
The DE circRNAs and miRNAs between 7 and 42-day old flies were analysed. Between day 7 and day 42, 29 DE circRNAs in Drosophila were identified, including 21 upregulated and 8 downregulated circRNAs at day 42 (Table 2). The circRNAs were derived from different source genes. These source genes were found to be involved in multiple molecular functions (Table 2). Evidently, the biological processes of the short lifespan-related source genes arm and pan were involved in the Wnt signalling pathway and had similar molecular functions, such as binding, protein binding, and transcription factor binding, transcription regulator activity. In addition, different circRNAs were observed to originate from the same mRNA transcript. For example, and dme_circ_0008175 and dme_circ_0008173 originated from the Nlg1 gene, and dme_circ_0009514 dme_circ_0009500 were derived from the pan gene.
Furthermore, 24 DE miRNAs (11 upregulated and 13 downregulated) were identified at day 42 compared to day 7 (Supplementary Data Sheet S1). Dme_miR-9a-3p and dme_miR-985-3p were identified as canonical specific fruit fly miRNAs. Then, dme_miR-956-3p, dme_miR-284-3p, and dme_miR-289-5p were identified as non-canonical miRNAs. The remaining 19 DE miRNAs were canonically conserved miRNAs. Then, functional annotation was carried out for the DE miRNAs. The Gene Ontology (GO) annotations of age-related DE miRNAs based on their targets were investigated. 11 upregulated and 13 downregulated miRNAs targeted to 3,292 mRNAs and 2,951 mRNAs, respectively (Supplementary Data Sheet S1), which mainly enriched in biological process (1,314 terms and 1,233 terms, respectively) (Supplementary Data Sheet S1). The bar plot shows the top ten enrichment score value of the significant enrichment terms (Figures 1A, B), such as multicellular organism development, nervous system development, neurogenesis, development process, and cell differentiation. Specifically, 20 DE miRNAs were related to Drosophila aging based on previous reports, including 11 upregulated and 9 downregulated miRNAs in 42 days when compared to 7 days (Figure 1C). 13 DE miRNAs involved in the age-signalling pathways by target genes on post-transcriptional level (Figure 1C). Their tagets involved in regulation of ROS detoxification, autopage, circadian rhythm, apoptosis, and immunity biological process. Otherwise, three out of 24 total miRNAs were conserved in Drosophila, human and mouse, containing dme-miR-8-5p, dme-miR-133-3p, and dme-miR-10-5p.
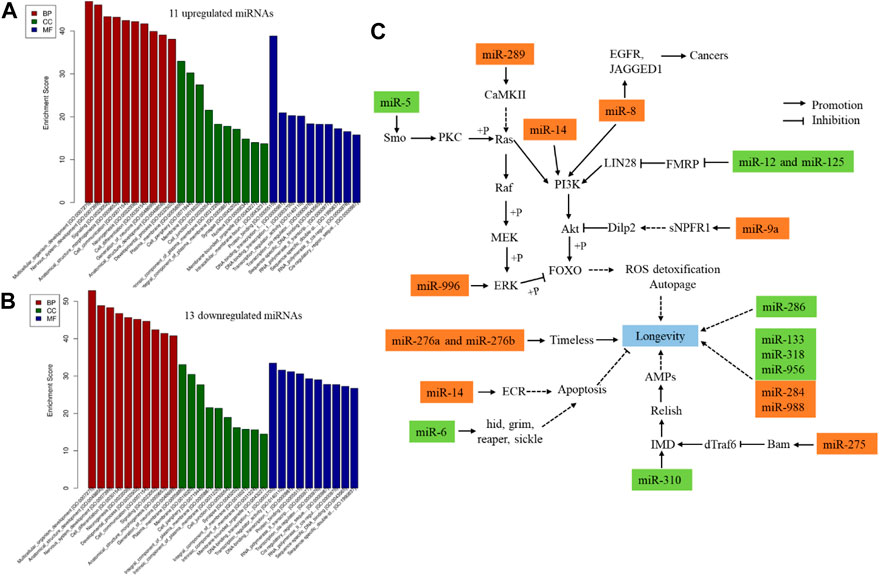
FIGURE 1. GO annotations age-related pathways of DE miRNAs in Drosophila between day 7 day 42. Twenty-four DE miRNAs were identified between 7 and 42-day old flies. (A), GO annotations of 11 upregulated miRNAs. (B), GO annotations of 13 downregulated miRNAs. (C), 20 DE miRNA affected the Drosophila agineg; rust colored frame, upregulated in 42 days when compared to 7 days (p < 0.05); green colored frame, downregulated in 42 days when compared to 7 days (p < 0.05). The references were followed as miR-289 (Chen et al., 2014; Nesler et al., 2016), miR-5 (Leaman et al., 2005; Chen et al., 2014), miR-8 (Soler Beatty et al., 2021; Chen et al., 2022), miR-12 (Yang et al., 2009), miR-125 (Bushey et al., 2009; Gendron and Pletcher, 2017; Luhur et al., 2017), miR-9a (Chen et al., 2014; Suh et al., 2015), miR-275 (Chen et al., 2014; Ji et al., 2019), miR-310 (Robins et al., 2005; Chen et al., 2014), miR-6 (Leaman et al., 2005; Chen et al., 2014), miR-14 (Xu et al., 2003; Varghese and Cohen, 2007), miR-276a (Chen et al., 2014; Zhang et al., 2021a), miR-276b (Chen et al., 2014; Ulgherait et al., 2020; Zhang et al., 2021b), miR-996 (Sun et al., 2015; Duan et al., 2018). BP, biological process; CC, celluar component; MF, molecular function.
2.3 DE circRNA/miRNA/mRNA networks in Drosophila ageing
In this section, the DE circRNA/miRNA/mRNA networks are analysed. Through the ceRNA mechanism, miRNAs can negatively regulate mRNA expression. Overall, 12 DE circRNAs, 21 DE miRNAs, and 30 DE mRNAs had interactions (Figure 2A, Supplementary Data Sheet S4). The binding sites between circRNA vs. miRNA (Supplementary Data Sheet S5) and miRNA vs. mRNA (Supplementary Data Sheet S6) are shown. According to the trends in the expression quantity changes, five DE circRNAs (dme_circ_0006913, dme_circ_0008173, dme_circ_0009500, dme_circ_0009667, and dme_circ_0010536) targeted the DE miRNAs with opposite expression trends (Figure 2B). Based on qPCR results, the expression patterns of four circRNAs (dme_circ_0008173, dme_circ_0009500, dme_circ_0009667, and dme_circ_0010536), and four miRNAs (dme_miR-289-5p, dme_miR-985-3p, dme_miR-286-3p, dme_miR-14-5p), four mRNAs (frizzled, CG31064, Abl and SERCA) were consistent with the RNA-seq data (Figure 2C). Importantly, the expression trends of dme_circ_0009500/dme_miR-289-5p/CG31064, dme_circ_0009500/dme_miR-289-5p/frizzled, and dme_circ_0009500/dme_miR-985-3p/Abl conformed to the ceRNA mechanism.
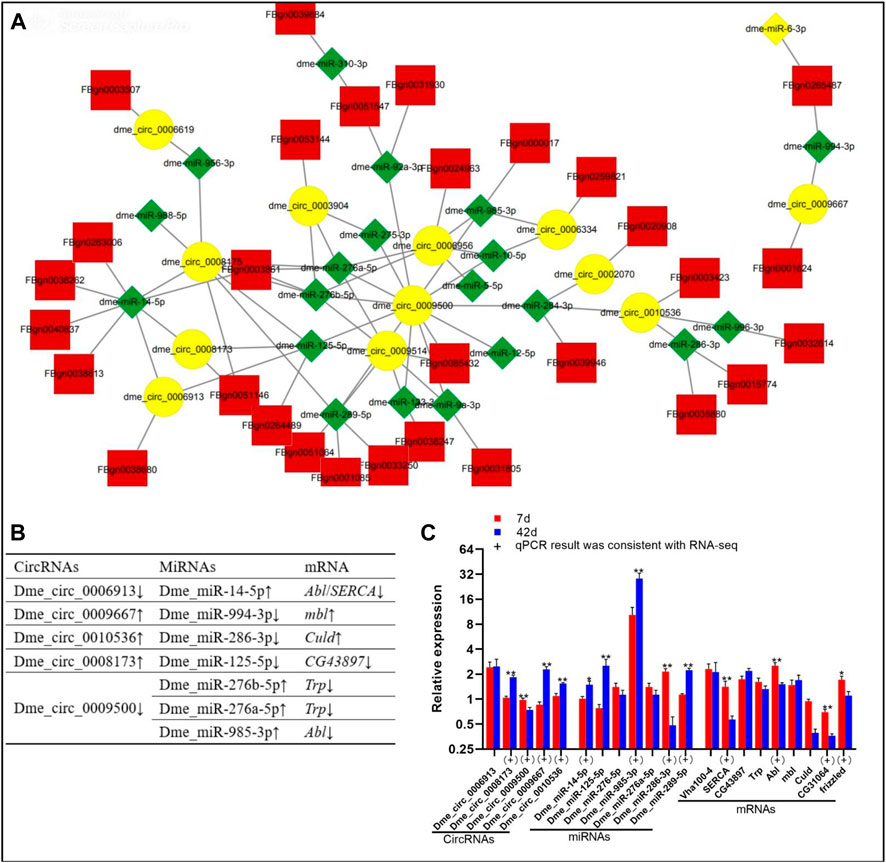
FIGURE 2. DE circRNA/miRNA/mRNA networks of Drosophila at day 7 day 42. (A), circRNA/miRNA/mRNA interaction networks; yellow, circRNAs; red, mRNAs; green, miRNAs. (B), Specific circRNA/miRNA/mRNA networks; “↑” “↓” indicate upregulation downregulation on day 42 compared to day 7 in RNA-seq data, respectively. (C), Data on the relative expression of circRNA, miRNA, mRNA detected by qPCR analysis. The relative gene expression levels were calculated by the cycle threshold values that were identified as 2−ΔΔCT. The ribosomal protein L32 (rp 49) gene was used as the reference gene to calculate the relative mRNA, miRNA, circRNA levels. “*” above the bars indicates a significant difference at the 0.05 level, “**” indicates a significant difference at the 0.01 level. (+) represents the qPCR results were consistent with the RNA-seq data.
2.4 DE lncRNA/miRNA/mRNA networks in Drosophila ageing
According to the functional patterns of lncRNAs competing with mRNAs for binding to miRNAs, the interaction networks of DE lncRNAs/miRNAs/mRNAs were identified (Supplementary Data Sheet S7). Then, the binding sites between lncRNAs and miRNAs were included in Supplementary Data Sheet S8. In addition, DE miRNAs targeted DE mRNAs with opposite expression trends. Based on the DE genes in our database, 15 lncRNAs, 15 miRNAs, and 32 mRNAs had interactions (Figure 3A). Based on qPCR results, the expression patterns of two lncRNAs (XLOC_027736 and XLOC_189909), three miRNAs (dme_miR-289-5p, dme_miR-985-3p, and dme_miR-14-5p), and three mRNAs (frizzled, CG31064, and Abl) were consistent with the RNA-seq data (Figure 3A). Several specific lncRNA/miRNA/mRNA networks, including XLOC_027736/dme_miR-985-3p/Abl, XLOC_073604/dme_miR-994-3p-3p/mbl, XLOC_189909/dme_miR-985-3p/Abl (Figure 3B), were found. In the XLOC_027736/dme_miR-985-3p/Abl and XLOC_189909/dme_miR-985-3p/Abl networks, the expression trend of these genes was determined by qPCR analysis to be consistent with the RNA-seq data from our study (Figure 3C).
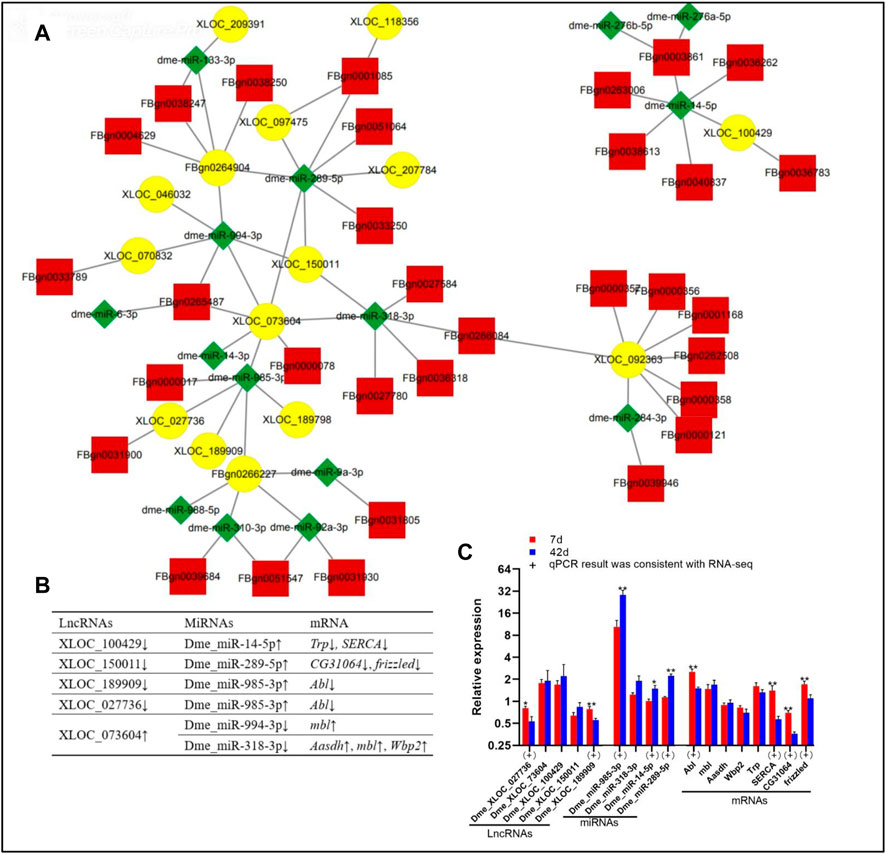
FIGURE 3. DE lncRNA/miRNA/mRNA networks in Drosophila at day 7 day 42. (A), lncRNA/miRNA/mRNA interaction networks; yellow, lncRNAs; red, mRNAs; green, miRNAs. (B), Specific lncRNA/miRNA/mRNA networks; “↑” “↓” indicate upregulation downregulation on day 42 compared to day 7, respectively. (C), Data on the relative expression of lncRNAs, miRNAs, mRNAs by qPCR analysis. 7 days, 7 days; 42 days, 42 days. The relative gene expression levels were calculated using the cycle threshold values that were identified as 2−ΔΔCT. The ribosomal protein L32 (rp 49) gene was used as the reference gene to calculate the relative mRNA, miRNA, lncRNA levels. “*” above the bars indicates a significant difference at the 0.05 level, “**” indicates a significant difference at the 0.01 level. (+) represents the qPCR results were consistent with the RNA-seq data.
2.5 Functional annotation of DE circRNA/lncRNA-associated networks
GO functional annotation of DE circRNAs/lncRNAs/mRNAs was carried out based on 74 target mRNA genes (Supplementary Data Sheet S9). The first 30 GO terms based on the lowest p values are listed (Figure 4). In circRNA/mRNA GO terms, there were just two major GO categories in the circRNA-associated networks, including biological processes (29 GO terms) and cellular components (1 GO term, perinuclear region of cytoplasm). Similarly, the GO annotations of the lncRNA-associated networks consisted of 25 GO terms in biological processes and 5 GO terms in the cellular component.
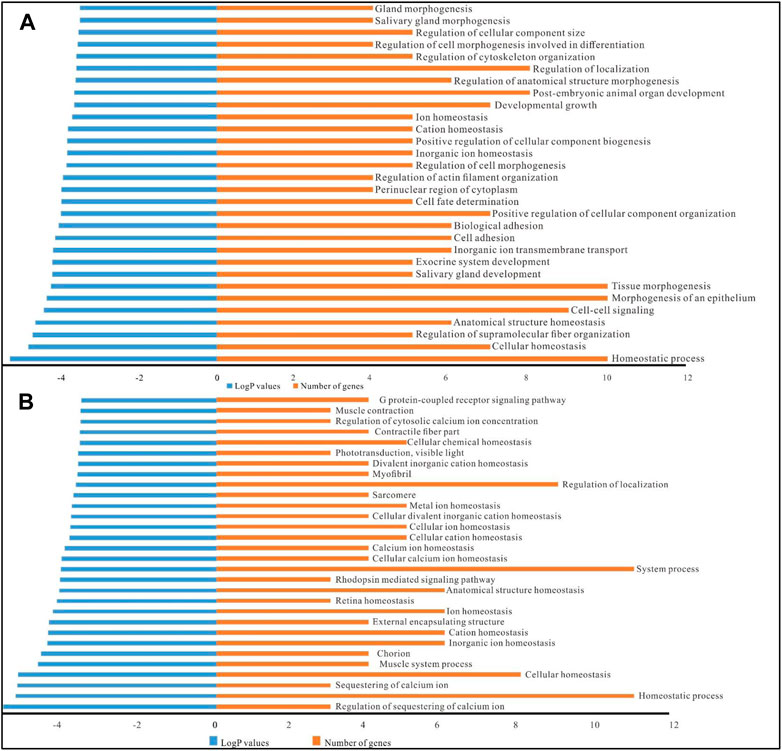
FIGURE 4. GO annotations of the DE circRNA-/lncRNA-associated networks. (A), GO annotations of the DE circRNA/miRNA/mRNA networks. (B), GO annotations of the DE lncRNA/miRNA/mRNA networks. LogP values indicate the enrichment degree of targets in the corresponding GO term, a smaller value represents a higher enrichment degree. The number of genes represents the number of target genes enriched in GO terms.
In the GO annotations of the circRNA-associated networks, there were several ageing-related biological processes in GO terms, such as homeostatic process (10 DE genes), cellular homeostasis (7 DE genes), cation homeostasis (5 DE genes), ion homeostasis (5 DE genes), cell-cell signalling (9 DE genes), cell fate determination (5 DE genes), developmental growth (7 DE genes) (Figure 5A). Furthermore, 13 out of the first 30 GO terms of the lncRNA-associated networks were related to homeostatic processs, including cellular homeostasis, retinal homeostasis, ion homeostasis, calcium ion homeostasis, and cellular cation homeostasis (Figure 5B). In the ageing-related ceRNA networks dme_circ_0009500/dme_miR-985-3p/Abl, XLOC_027736/dme_miR-985-3p/Abl and XLOC_189909/dme_miR-985-3p/Abl in Drosophila, Abl was involved in multiple GO terms, such as regulation of cell morphogenesis, developmental growth, regulation of cell differentiation, and regulation of neuron differentiation. Furthermore, frizzled was involved in the positive regulation of developmental growth, developmental growth involved in morphogenesis, cell-cell signalling, cell fate determination, and the Wnt signalling pathway in the dme_circ_0009500/dme_miR-289-5p/frizzled network.
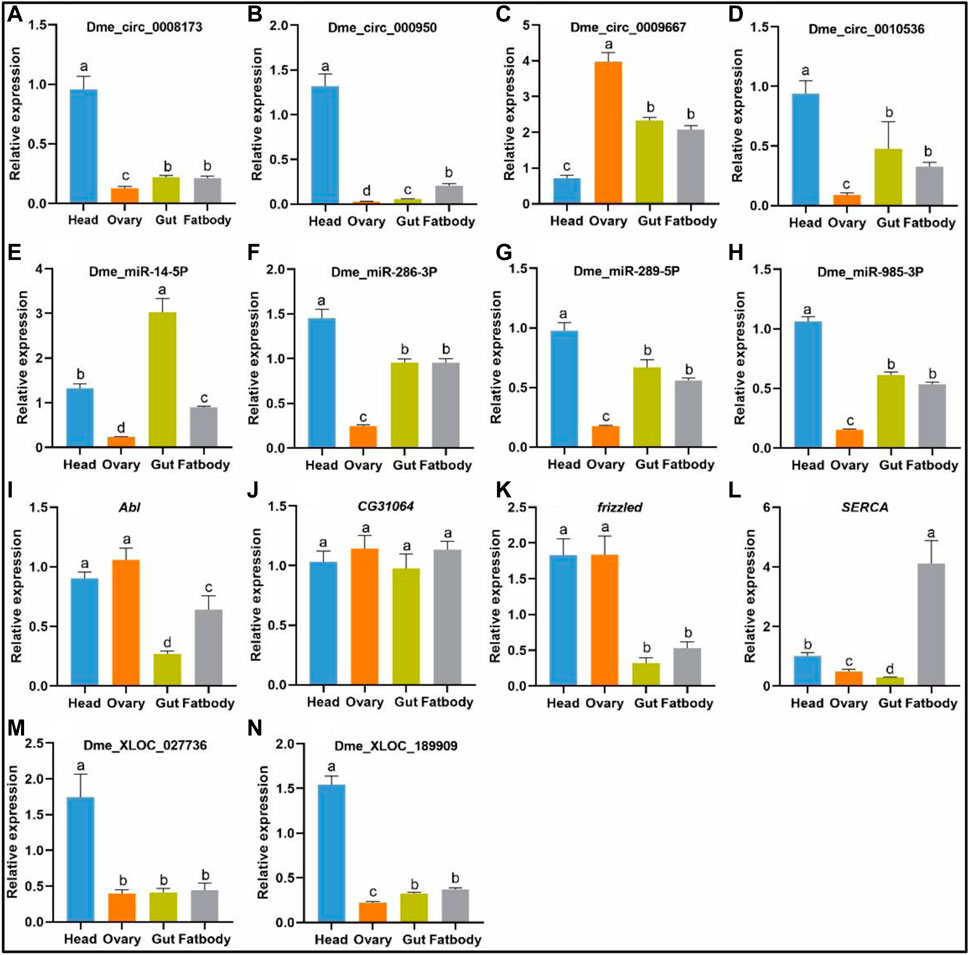
FIGURE 5. Tissue-specific expression patterns of the specific DE lncRNAs, circRNAs, miRNAs, mRNAs in the head, ovary, gut, fat body of flies. The expression levels of the DE genes in the circRNA/miRNA/mRNA lncRNA/miRNA/mRNA networks, as determined through qPCR, were consistent with the RNA-seq data. (A–D), The relative expression level of four DE circRNAs; (E–H), the relative expression level of four DE miRNAs; (I–L), the relative expression level of four DE mRNAs; (M,N), the relative expression level of two DE lncRNAs. The relative gene expression levels were calculated by the cycle threshold values that were identified as 2−ΔΔCT. The ribosomal protein L32 (rp 49) gene was used as the reference gene to calculate the relative mRNA, miRNA, lncRNA levels. The different letters above the bars indicate significant differences at the 0.05 level. (+) represents the qPCR results were consistent with the RNA-seq data.
2.6 Tissue expression pattern analysis
The tissue-specific expression patterns of the DE lncRNAs, circRNAs, miRNAs, and mRNAs were analysed in the head, ovary, gut, and fat body, the qPCR results of which were consistent with the RNA-seq data in the DE circRNA/miRNA/mRNA networks and lncRNA/miRNA/mRNA networks (Figure 5). The results showed that the tissue-specific expression patterns of dme_circ_0009500/dme_miR-289-5p/CG31064, dme_circ_0009500/dme_miR-289-5p/frizzled, and dme_circ_0009500/dme_miR-985-3p/Abl were mainly expressed in the head. Specifically, dme_circ_0009667 was mainly located in the ovary, and dme_miR-14-5p was mainly expressed in the gut. Similar results were found in the lncRNA/miRNA/mRNA networks. The tissue patterns of the XLOC_027736/dme_miR-985-3p/Abl and XLOC_189909/dme_miR-985-3p/Abl networks were also mainly expressed in the head.
2.7 Binding sites of specific ceRNAs
The binding sites of dme_circ_0009500/dme_miR-289-5p/CG31064, dme_circ_0009500/dme_miR-289-5p/frizzled, dme_circ_0009500/dme_miR-985-3p/Abl, XLOC_027736/dme_miR-985-3p/Abl, and XLOC_189909/dme_miR-985-3p/Abl were analyzed (Figure 6). Specificly, there were five binding sites between dme_circ_0009500 and dme_miR-289-5p with the higher binding free energy from -15.23 to -20.30 (Figure 6A; and Supplementary Data Sheet S5). Furthermore, 3′UTR sequence of frizzled had the two binding site with miR-289-5p, and also had the higher binding free energy -23.31 (Figure 6E and Supplementary Data Sheet S6).
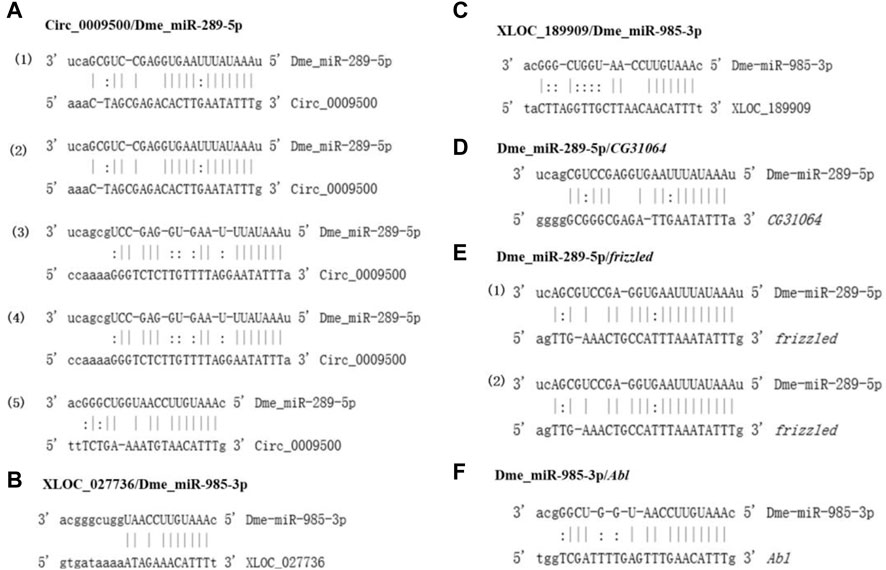
FIGURE 6. The binding sites of specific ceRNAs. (A), the binding sites between circ_0009500/Dme_miR-289-5p; (B), the binding sites between XLOC_027736 dme_miR-985-3p; (C), the binding sites between XLOC_189909 dme_miR-985-3p; (D), the binding sites between dme_miR-289-5p CG31064; (E), the binding sites between dme_miR-289-5p frizzled; (F), the binding sites between dme_miR-985-3p Abl.
3 Discussion
Drosophila is an ideal model for genetics, and the multiple age-related researches were carried out by Dahomey strain (Mołoń et al., 2020; De Groef et al., 2021). Previous study has reported the trend of wild-type female lifespan (Dahomey, Canton S, Oregon R) changes were similar (Sanz et al., 2010). Furthermore, several studies just used one wildtype strain in multiple-omics research (Shi et al., 2020; Wang et al., 2022). Thus, the wild-type female of Dahomey strain was utilized in our manuscript. Increasing evidence has suggested that ceRNA networks play key roles in a variety of biological processes, such as cancer (Wang et al., 2018; Abdollahzadeh et al., 2019), Alzheimer’s disease (AD) (Zhang et al., 2019), skeletal muscle myogenesis (Yue et al., 2019), and ageing (Zhao et al., 2019; Chen et al., 2020). miRNAs are the core molecules of the ceRNA regulatory system (Yue et al., 2019; Chen et al., 2020). Thus, screening DE miRNAs is essential for research on ceRNA networks related to Drosophila ageing. miRNAs generally induce mRNA degradation or repress translation of target transcripts through sequence-specific binding to the transcript 3′UTR (Bushati and Cohen, 2007; Chen et al., 2019). Each transcript can be targeted by multiple miRNAs, and each miRNA can target hundreds of different transcripts (mRNA, circRNA, and lncRNA transcripts) (Dori and Bicciato, 2019; Zhou et al., 2019; Kinser and Pincus, 2020). Thus, the miRNA regulatory network is far-reaching (Kinser and Pincus, 2020). Previous studies have verified that miRNAs are important small regulatory ncRNA molecules that control a fairly large number of biological processes; their important functions have generated interest in their use as biomarkers and their roles as regulators of ageing (Lai et al., 2019; Kinser and Pincus, 2020) and a number of cancer types (Liang et al., 2020; Mishan et al., 2020; Wang et al., 2020). Recent studies have also suggested that miRNAs are involved in the regulation of age-associated processes and pathologies in multiple mammalian tissues, including the brain, heart, bones, and muscles (Chen et al., 2020; John et al., 2020; Kinser and Pincus, 2020; Ullah et al., 2020).
In our study, more than 80% (20 out of 24 total miRNAs) of DE miRNAs between day 7 and day 42 could affect fruit fly ageing. Among these miRNAs, miR-14 as a cell death suppressor, regulates fat metabolism, insulin production and metabolism through its targets (Xu et al., 2003; Nelson et al., 2014). In addition, knockout of five DE miRNAs (miR-133, miR-284, miR-286, miR-318, miR-956, and miR-988) decreased the Drosophila lifespan, and miR-286 KO increased female lifespan (Chen et al., 2014). The consreved miR-8 was the homolog of vertebrate miR-200 family. It is worth noting that miR-8 acted through U-shaped to activate PI3K and thereby promoted fat cell growth cell-autonomously and the Insulin-like Receptor signaling pathway (Soler Beatty et al., 2021; Chen et al., 2022). Then, two targets of miR-200 (dme-miR-8 homolog) in human were JAGGED1 (JAG1) (Xue et al., 2021) and epidermal growth factor receptor (EGFR) (Xue et al., 2019). In a lung cancer metastasis, overexpression of the miR-200 decreased JAG1 protein levels and impeded cell growth (Xue et al., 2021). Furthermore, Dmel\ EGFR is orthologous to human gene ERBB2, which has also been implicated in multiple cancers (Xue et al., 2019). The results indicate that these miRNAs may act as sponges through the circRNA/miRNA/mRNA and lncRNA/miRNA/mRNA networks involved in the regulation of Drosophila ageing. In a previous study, global identification of functional miRNA-mRNA interactions in Drosophila was performed (Wessels et al., 2019). However, circRNA/miRNA/mRNA and lncRNA/miRNA/mRNA interactions in fly ageing have remained unclear.
Most of the DE circRNAs accumulated in 42-day-old Drosophila, which has been reported in a previous study (Westholm et al., 2014) in flies and other model organisms (Knupp and Miura, 2018; Kim and Lee, 2019). circRNAs are highly stable molecules that play important roles in ageing (Weigelt et al., 2020) and age-related diseases (Ren et al., 2020; Wang P. et al., 2020). Additionally, six source genes of circRNAs were related to fruit fly ageing, including asator (short-lived, AsatorGD7323) (Neely et al., 2010), dad (short-lived, DadGD1335) (Kamiya et al., 2008), gish (short-lived, gishGD10588) (Neely et al., 2010; Fulford et al., 2019), pan (short-lived, panΔN.UAS) (Buchon et al., 2013; Franz et al., 2017), psq (long-lived, psqBG01031) (Magwire et al., 2010; Bonchuk et al., 2011), and arm (short-lived, armS10. UAS. Tag:MYC) (Dupont et al., 2012). In the present study, multiple networks of DE circRNAs/miRNAs/mRNAs and DE lncRNAs/miRNAs/mRNAs in Drosophila between day 7 and day 42 were identified. GO annotations of the DE circRNA/miRNA/mRNA and DE lncRNA/miRNA/mRNA networks demonstrated that the DE circRNAs and lncRNAs may take part in Drosophila ageing via various biological processes. Multiple GO terms enriched in the DE circRNA/miRNA/mRNA networks, such as cellular homeostasis (Hartl, 2016) and developmental growth (Clarke et al., 2020), are clearly related to ageing. Specifically, multiple GO terms, including inorganic ion homeostasis, cation homeostasis, and cellular calcium ion homeostasis, are involved in regulating the sequestration of calcium ions. Ca2+ dyshomeostasis is associated with several ageing-related neurodegenerative diseases, such as AD, Huntington’s disease (HD), Parkinson’s disease (PD), and amyotrophic lateral sclerosis (ALS), with an altered Ca2+ buffering capacity, an altered regulation of Ca2+ channels and pumps, and an altered neuronal excitability (Bezprozvanny, 2010). A previous study demonstrated that ageing was closely linked to the dysregulation of Ca2+ homeostasis, resulting in a chronically elevated level of cytosolic Ca2+ in experimental models of neuronal ageing (Thibault et al., 2007; Duncan et al., 2010). The results suggest that the DE circRNA/miRNA/mRNA and DE lncRNA/miRNA/mRNA networks may play a significant role in Drosophila ageing.
Based on the same expression trend between the RNA-seq and qPCR detection results in our study, some specific ceRNA networks, including the dme_circ_0009500/dme_miR-289-5p/CG31064, dme_circ_0009500/dme_miR-289-5p/frizzled, dme_circ_0009500/dme_miR-985-3p/Abl, XLOC_027736/dme_miR-985-3p/Abl and XLOC_189909/dme_miR-985-3p/Abl networks, were identified. Thus, we determined that these networks merited further investigation. In our study, miR-289-5p was mainly expressed in the Drosophila head and upregulated in 42-day-old flies compared to 7-day-old flies. In previous studies, miR-289-5p, which may be responsible for silencing the expression of candidate genes during the diapause of Sarcophaga bullata, was overexpressed in diapausing pupae (Reynolds et al., 2017). Then, miR-289 participates in the control of a diverse array of pleiotropic cellular processes during Drosophila development (Nesler et al., 2013). It has been reported that miR-289 is downregulated in adult-onset AD Drosophila brains (Kong et al., 2014). Neuronal misexpression of miR-289 suppresses activity-dependent synaptic growth (Nesler et al., 2013). The target CG31064 of miR-289-5p was located in the adult head in our study in previous research (Aradska et al., 2015), which enabled a small GTPase binding activity (Gillingham et al., 2014). Another target, frizzled of miR-289-5p, has been reported to be involved in the regulation of the mTOR and signalling pathway the Wnt signalling pathway (Zeng et al., 2018). The GO frizzled terms were related to positive regulation of developmental growth, developmental growth involved in morphogenesis, cell-cell signalling, cell fate determination, and the Wnt signalling pathway. Previous study has reported that frizzled in involved in regulation of pro-survival processes in human PD through Wnt1/Fzd-1/β-catenin astrocyte-dopamine autoprotective loop (L'Episcopo et al., 2011). Therefore, dme_circ_0009500/dme_miR-289-5p/CG31064 and dme_circ_0009500/dme_miR-289-5p/frizzled may play roles in brain ageing in fruit flies.
Furthermore, the lncRNAs XLOC_027736 and XLOC_189909, the dme_circ_0009500 and the mRNA Abl were predicted to competitively bind miR-985-3p, which was mainly expressed in the Drosophila head. Abl phosphorylates cell adhesion and cytoskeletal proteins and acts as a scaffold in a signalling complex to regulate both epithelial and nervous system morphogenesis (Zhu and Bhat, 2011; Liu and Wu, 2014). The age-related phenotype associated with the Abl mutant led to a shorter lifespan for flies with three specific alleles (Abll2, Abll3, and AblGD1344) (Belote et al., 1990; Huang et al., 2007; Neely et al., 2010). Furthermore, human ABL1 (Drosophila Abl homolog) protein kinases play many important roles in neuron development, maintenance, and signalling (Manley et al., 2022). In future studies, it will be necessary to determine the biological role of the lncRNAs XLOC_027736 XLOC_189909, the circRNA dme_circ_0009500, and miR-985-3p in the fly ageing process.
In our study, DESeq2 and EdgeR were used to analyse the DE circRNAs and miRNAs, respectively. DESeq2 and EdgeR are efficient tools for differential analysis of RNA-seq data with the more than 80% overlapping, both of which use the negative binomial distribution (Liu et al., 2021). Then, DESeq2 can more accurately identify DE genes for small samples to reduce false positives (Love et al., 2014). Then, two biological replicates were utilized to analysis DE circRNAs. Thus, DESeq2 was chosen to identify the DE circRNAs. The above results suggest that our data are also available. To date, DE circRNAs and miRNAs cannot be reanalysed only by DESeq2 or EdgeR in the present study. This would bring some questions to our subsequent analysis. For example, some DE circRNAs and miRNAs may be lost, which may result that part ceRNA networks could not be recognized. More experiments will be needed to verify these networks in our future study.
In this study, several DE miRNAs were identified between day 7 and day 42, such as the dme_miR-289, dme_miRNA-14, and the conserved miR-8. Then, the potential ceRNA networks may play a role in Drosophila aging, for example, dme_circ_0009500/dme_miR-289-5p/frizzled and XLOC_027736/dme_miR-985-3p/Abl networks. Therefore, the result of DE miRNAs, circRNA/miRNA/mRNA, and lncRNA/miRNA/mRNA interactions provides an important foundation to parse the genetic process of Drosophila ageing.
4 Materials and methods
4.1 Sample collection and preparation
Female flies (DahomeyWT) that had mated with male flies for 48 h after hatching were bred under a 12 h on/off light cycle at 25°C in 50% humidity. Sample collection of adult flies at day 7 and day 42 was performed as described by Yang et al. (2016). RNA-seq (mRNA, lncRNA, and circRNA) was conducted on two biological replicates, while miRNA sequencing was conducted on five biological replicates. All samples were stored at −80°C until use.
4.2 LncRNA, mRNA, circRNA data from Drosophila at days 7 and 42
RNA-seq data for the lncRNAs and mRNAs of Drosophila at day 7 and day 42 were obtained by Yang et al. (2016). The circRNA analysis was based on the RNA-seq data for Drosophila at day 7 and day 42 from Yang et al. (2016). Overall, 95.79% clean reads were obtained from 26.7 GB of raw sequence data (SRP073695) then aligned to the D. melanogaster genome from FlyBase (Dmel_Release_6, http://FlyBase.org/). The find_circ (Memczak et al., 2013) and CIRI2 (Gao et al., 2015) software tools were utilized to identify circRNAs. Then, the overlapping Drosophila circRNAs identified by both software programs were selected. The input data for the circRNA differential expression analysis were readCount data obtained from the circRNA expression level analysis. Then, paired differential expression analysis of circRNAs between day 7 and day 42 was conducted with DESeq2 (Varet et al., 2016) based on a negative binomial distribution. The p-value was adjusted using Hochberg and Benjamini’s methods (Hinkley et al., 2018) to control the error discovery rate. A p-value <0.05 was considered to indicate a DE circRNA. These original data were from our laboratory.
4.3 MiRNAs in Drosophila at day 7 and day 42
TRIzol Reagent (Invitrogen, CA) was used to extract the total RNA from fruit flies at day 7 and day 42. Agarose gel electrophoresis was performed to verify the integrity of the total RNA samples. A NanoDrop ND-1000 instrument was used to accurately measure the concentrations and protein contamination of the total RNA samples. miRNA sequencing libraries were generated using rRNA-depleted RNA with a NEBNext® Ultra™ Multiplex Small RNA Library Prep Set Kit for Illumina® (New EnglBiolabs, United States) following the manufacturer’s recommendations. Subsequently, an Agilent 2,100 Bioanalyser and an Agilent DNA 1000 chip kit (Agilent, part #5067-1504) were utilized to accurately assess the quality and concentration of the sequencing libraries. The libraries were sequenced using an Illumina NextSeq 500. MiRNA fragment sequencing was performed by the Aksomics company.
Clean reads were generated from the raw sequence data from the Illumina NextSeq instrument through real-time base calling and quality filtering. The clean reads were recorded in FASTQ format and contained read information, sequences and quality encoding. Subsequently, the 5′- and 3′-adapter sequences were trimmed from the clean reads by Cutadapt, and reads with lengths shorter than 14 nt or longer than 40 nt were discarded. The trimmed reads were collapsed into FASTA format. The raw data has been uploaded to NCBI database (PRJNA716466). The trimmed reads that did not map to mature or precursor tRNA sequences were aligned with an allowance of only one mismatch to miRNA reference sequences with miRDeep2 (Friedlander et al., 2012). The expression profiles of miRNAs were determined based on the counts of the reads mapped. The DE miRNAs were identified with the R package EdgeR based on the count values (Robinson et al., 2010). A fold change cut-off of 1.5 and a p-value cut-off of 0.05 were applied only when replicates were used for screening DE miRNAs. The gene prediction of DE miRNA integrates two algorithms, miRanda (Enright et al., 2003) and TargetScan (Garcia et al., 2011). GO enrichment analysis of the targets of DE miRNAs was implemented with the GOseq R package (Young et al., 2010).
4.4 CeRNA analysis of lncRNA/circRNA-miRNA-mRNA
The lncRNAs, circRNAs, and miRNAs showed significantly different expression levels between day 7 and day 42 and were thus analysed. The potential ceRNAs were searched based on the sequences of the lncRNAs, circRNAs, and mRNAs. The offline software MiRanda (Enright et al., 2003) was utilized to predict miRNA binding seed sequence sites, and overlap of the same miRNA binding sites on lncRNAs/circRNAs-miRNAs and miRNAs-mRNAs was taken to indicate a lncRNA/circRNA-miRNA-mRNA interaction. Then, the “clusterProfiler” R package was utilized to perform Gene Ontology (GO) enrichment of ceRNA networks based on the mRNAs.
The DE mRNAs associated with ageing and the corresponding ceRNA networks (including the circRNAs, lncRNAs, miRNAs, and mRNAs) were selected to detect the expression level by qPCR. In total, 9 miRNAs, 10 mRNAs, 5 lncRNAs, and 5 circRNAs were selected to qualify the expression level. Samples (three biological duplicates) from 7- and 42-day-old fruit flies were used to isolate the total RNA using TRIzol™ LS reagent (Thermo Fisher) according to the manufacturer’s instructions. The total RNA (1 μg) from each sample was reverse transcribed with random primers using a RevertAid First-strand cDNA Synthesis Kit (Thermo Fisher) according to the manufacturer’s protocol, which was utilized to detect the expression levels of mRNA, lncRNA, and circRNA. In addition, the total RNA (1 μg) used for miRNA expression detection in each sample was reverse transcribed using a TaqMan™ MicroRNA Reverse Transcription Kit (Thermo Fisher) according to the kit instructions. All gene primers (Supplementary Data Sheet S10) were designed using the Primer 5.0 software and purchased from Sangon Biotech. The SYBR green method was used for qRT-PCR with TransStart® Green qPCR SuperMix (TransGen Biotech) following the manufacturer’s instructions. All tested samples were three biological replicates. The relative gene expression levels were calculated using cycle threshold values and the 2-ΔΔCT method. Ribosomal protein L32 (rp 49) was used as the reference gene to calculate the relative mRNA, miRNA, lncRNA, and circRNA levels. Differential expression levels were compared by independent-samples t-tests between groups.
4.5 Tissue-specific expression pattern
Based on the same expression pattern between day 7- and day 42-day-old flies, as determined by the RNA-seq data and qPCR tests on 7-day and 42-day-old flies, the tissue-specific expression model of four circRNAs (dme_circ_0008173, dme_circ_000950, dme_circ_0009667, and dme_circ_0010536), four miRNAs (dme_miR-289P, dme_miR-985-3P, dme_miR-286-3P, and dme_miR-14-5P), four mRNA (SERCA, frizzled, Abl, and CG31064), and two lncRNAs (XLOC_027736 and XLOC_189909) were selected to analyse the tissue specificity of the head, ovary, gut and fat body of female fruit flies at 7 days. Each sample included three biological replicates. The expression levels of these genes were detected by qRT-PCR as described above. Differential expression levels were compared by independent-samples t-tests between groups.
Data availability statement
The original contributions presented in the study are publicly available. This data can be found here: https://www.ncbi.nlm.nih.gov/ (Accession number PRJNA716466).
Author contributions
DY and FX: writing data analysis; JL: data analysis; XF: quality control data verification; QN, YL, MZ, and TY: data visualization and quality control; DY: revision and typesetting; ZH and MY: design of the study revision.
Funding
The National Natural Science Foundation of China (grant numbers 31972777, 2019; 31402286, 2015) and China Scholarship Council (202106915017).
Conflict of interest
The authors declare that the research was conducted in the absence of any commercial or financial relationships that could be construed as a potential conflict of interest.
Publisher’s note
All claims expressed in this article are solely those of the authors and do not necessarily represent those of their affiliated organizations, or those of the publisher, the editors and the reviewers. Any product that may be evaluated in this article, or claim that may be made by its manufacturer, is not guaranteed or endorsed by the publisher.
Supplementary material
The Supplementary Material for this article can be found online at: https://www.frontiersin.org/articles/10.3389/fgene.2023.1096902/full#supplementary-material
References
Abdollahzadeh, R., Daraei, A., Mansoori, Y., Sepahvand, M., Amoli, M. M., and Tavakkoly-Bazzaz, J. (2019). Competing endogenous RNA (ceRNA) cross talk language in ceRNA regulatory networks: A new look at hallmarks of breast cancer. J. Cell Physiol. 234 (7), 10080–10100. doi:10.1002/jcp.27941
Aradska, J., Bulat, T., Sialana, F. J., Birner-Gruenberger, R., Erich, B., and Lubec, G. (2015). Gel-free mass spectrometry analysis of Drosophila melanogaster heads. Proteomics 15 (19), 3356–3360. doi:10.1002/pmic.201500092
Barter, T. T., Greenspan, Z. S., Phillips, M. A., Mueller, L. D., Rose, M. R., and Ranz, J. M. (2019). Drosophila transcriptomics with and without ageing. Biogerontology 20 (5), 699–710. doi:10.1007/s10522-019-09823-4
Belote, J. M., Hoffmann, F. M., McKeown, M., Chorsky, R. L., and andBaker, B. S. (1990). Cytogenetic analysis of chromosome region 73AD of Drosophila melanogaster. Genetics 125 (4), 783–793. doi:10.1093/genetics/125.4.783
Bezprozvanny, I. B. (2010). Calcium signaling and neurodegeneration. Acta Naturae 2 (1), 72–80. doi:10.32607/actanaturae.10761
Bonchuk, A., Denisov, S., Georgiev, P., and Maksimenko, O. (2011). Drosophila BTB/POZ domains of "ttk group" can form multimers and selectively interact with each other. J. Mol. Biol. 412 (3), 423–436. doi:10.1016/j.jmb.2011.07.052
Brenman-Suttner, D. B., Yost, R. T., Frame, A. K., Robinson, J. W., Moehring, A. J., and Simon, A. F. (2020). Social behavior and aging: A fly model. Genes Brain Behav. 19 (2), e12598. doi:10.1111/gbb.12598
Brown, C. J., Kaufman, T., Trinidad, J. C., and Clemmer, D. E. (2018). Proteome changes in the aging Drosophila melanogaster head. Int. J. Mass Spectrom. 425, 36–46. doi:10.1016/j.ijms.2018.01.003
Buchon, N., Osman, D., David, F. P., Fang, H. Y., Boquete, J. P., Deplancke, B., et al. (2013). Morphological and molecular characterization of adult midgut compartmentalization in Drosophila. Cell Rep. 3 (5), 1725–1738. doi:10.1016/j.celrep.2013.04.001
Bushati, N., and Cohen, S. M. (2007). microRNA functions. Annu. Rev. Cell Dev. Biol. 23, 175–205. doi:10.1146/annurev.cellbio.23.090506.123406
Bushey, D., Tononi, G., and Cirelli, C. (2009). The Drosophila fragile X mental retardation gene regulates sleep need. J. Neurosci. 29 (7), 1948–1961. doi:10.1523/JNEUROSCI.4830-08.2009
Cha, S. J., Do, H. A., Choi, H. J., Lee, M., and Kim, K. (2019). The Drosophila model: Exploring novel therapeutic compounds against neurodegenerative diseases. Antioxidants (Basel) 8 (12), 623. doi:10.3390/antiox8120623
Chen, D., Liu, J., Meng, J., Li, D., Zhao, P., Duan, Y., et al. (2020). Integrative analysis of long non-coding RNAs (lncRNAs), miRNAs, and mRNA-associated ceRNA network in lung tissue of aging mice and changes after treatment with codonopsis pilosula. Med. Sci. Monit. 26, e921580. doi:10.12659/MSM.921580
Chen, J., Huang, Y., and Qi, G. (2022). LncRNA-IRAR-mediated regulation of insulin receptor transcripts in Drosophila melanogaster during nutritional stress. Insect Mol. Biol. 31 (3), 261–272. doi:10.1111/imb.12756
Chen, P., Chen, F., Lei, J., Li, Q., and Zhou, B. (2019). Activation of the miR-34a-mediated SIRT1/mTOR signaling pathway by urolithin A attenuates D-galactose-induced brain aging in mice. Neurotherapeutics 16 (4), 1269–1282. doi:10.1007/s13311-019-00753-0
Chen, Y. W., Song, S., Weng, R., Verma, P., Kugler, J. M., Buescher, M., et al. (2014). Systematic study of Drosophila microRNA functions using a collection of targeted knockout mutations. Dev. Cell 31 (6), 784–800. doi:10.1016/j.devcel.2014.11.029
Clarke, G. D., Li, J., Kuo, A. H., Moody, A. J., and Nathanielsz, P. W. (2020). Cardiac magnetic resonance imaging: Insights into developmental programming its consequences for aging. J. Dev. Orig. Health Dis. 12, 203. doi:10.1017/S2040174420001233
De Groef, S., Wilms, T., Balmand, S., Calevro, F., and Callaerts, P. (2021). Sexual dimorphism in metabolic responses to western diet in Drosophila melanogaster. Biomolecules 12 (1), 33. doi:10.3390/biom12010033
Dori, M., and Bicciato, S. (2019). Integration of bioinformatic predictions and experimental data to identify circRNA-miRNA associations. Genes (Basel) 10 (9), 642. doi:10.3390/genes10090642
Duan, H., de Navas, L. F., Hu, F., Sun, K., Mavromatakis, Y. E., Viets, K., et al. (2018). The mir-279/996 cluster represses receptor tyrosine kinase signaling to determine cell fates in the Drosophila eye. Development 145 (7), dev159053. doi:10.1242/dev.159053
Duncan, R. S., Goad, D. L., Grillo, M. A., Kaja, S., Payne, A. J., and Koulen, P. (2010). Control of intracellular calcium signaling as a neuroprotective strategy. Molecules 15 (3), 1168–1195. doi:10.3390/molecules15031168
Dupont, P., Besson, M. T., Devaux, J., and Lievens, J. C. (2012). Reducing canonical Wingless/Wnt signaling pathway confers protection against mutant Huntingtin toxicity in Drosophila. Neurobiol. Dis. 47 (2), 237–247. doi:10.1016/j.nbd.2012.04.007
Enomoto, M., Siow, C., and Igaki, T. (2018). Drosophila as a cancer model. Adv. Exp. Med. Biol. 1076, 173–194. doi:10.1007/978-981-13-0529-0_10
Enright, A. J., John, B., Gaul, U., Tuschl, T., Sander, C., and Marks, D. S. (2003). MicroRNA targets in Drosophila. Genome Biol. 5 (1), R1. doi:10.1186/gb-2003-5-1-r1
Fox, D. T., Cohen, E., and Smith-Bolton, R. (2020). Model systems for regeneration: Drosophila. Development 147 (7), dev173781. doi:10.1242/dev.173781
Franz, A., Shlyueva, D., Brunner, E., Stark, A., and Basler, K. (2017). Probing the canonicity of the Wnt/Wingless signaling pathway. PLoS Genet. 13 (4), e1006700. doi:10.1371/journal.pgen.1006700
Friedlander, M. R., Mackowiak, S. D., Li, N., Chen, W., and Rajewsky, N. (2012). miRDeep2 accurately identifies known and hundreds of novel microRNA genes in seven animal clades. Nucleic Acids Res. 40 (1), 37–52. doi:10.1093/nar/gkr688
Fulford, A. D., Holder, M. V., Frith, D., Snijders, A. P., Tapon, N., and Ribeiro, P. S. (2019). Casein kinase 1 family proteins promote Slimb-dependent Expanded degradation. Elife 8, e46592. doi:10.7554/eLife.46592
Gao, Y., Wang, J., and Zhao, F. (2015). Ciri: An efficient and unbiased algorithm for de novo circular RNA identification. Genome Biol. 16, 4. doi:10.1186/s13059-014-0571-3
Garcia, D. M., Baek, D., Shin, C., Bell, G. W., Grimson, A., and Bartel, D. P. (2011). Weak seed-pairing stability and high target-site abundance decrease the proficiency of lsy-6 and other microRNAs. Nat. Struct. Mol. Biol. 18, 1139–1146. doi:10.1038/nsmb.2115
Gendron, C. M., and Pletcher, S. D. (2017). MicroRNAs mir-184 and let-7 alter Drosophila metabolism and longevity. Aging Cell 16 (6), 1434–1438. doi:10.1111/acel.12673
Gillingham, A. K., Sinka, R., Torres, I. L., Lilley, K. S., and Munro, S. (2014). Toward a comprehensive map of the effectors of rab GTPases. Dev. Cell 31 (3), 358–373. doi:10.1016/j.devcel.2014.10.007
Gubina, N., Naudi, A., Stefanatos, R., Jove, M., Scialo, F., Fernandez-Ayala, D. J., et al. (2019). Essential physiological differences characterize short- and long-lived strains of Drosophila melanogaster. J. Gerontol. A Biol. Sci. Med. Sci. 74 (13), 1835–1843. doi:10.1093/gerona/gly143
Hartl, F. U. (2016). Cellular homeostasis and aging. Annu. Rev. Biochem. 85, 1–4. doi:10.1146/annurev-biochem-011116-110806
He, Q. Y., Liu, G. H., Bao, Z. J., Kong, Q. P., Tao, J., Yu, Y. Q., et al. (2019). Chinese aging anti-aging experts agree. Geriatr. Health Care 25 (5), 551–553.
Hinkley, T., Brown, H., Carson, V., and Teychenne, M. (2018). Cross sectional associations of screen time and outdoor play with social skills in preschool children. PLoS One 13 (4), e0193700. doi:10.1371/journal.pone.0193700
Huang, C. H., Lin, T. Y., Pan, R. L., and Juang, J. L. (2007). The involvement of Abl and PTP61F in the regulation of Abi protein localization and stability and lamella formation in Drosophila S2 cells. J. Biol. Chem. 282 (44), 32442–32452. doi:10.1074/jbc.M702583200
Ji, S., Luo, Y., Cai, Q., Cao, Z., Zhao, Y., Mei, J., et al. (2019). LC domain-mediated coalescence is essential for otu enzymatic activity to extend Drosophila lifespan. Mol. Cell 74 (2), 363–377.e5. doi:10.1016/j.molcel.2019.02.004
John, A., Kubosumi, A., and Reddy, P. H. (2020). Mitochondrial MicroRNAs in aging and neurodegenerative diseases. Cells 9 (6), 1345. doi:10.3390/cells9061345
Kamiya, Y., Miyazono, K., and Miyazawa, K. (2008). Specificity of the inhibitory effects of dad on TGF-beta family type I receptors, thickveins, saxophone, and baboon in Drosophila. FEBS Lett. 582 (17), 2496–2500. doi:10.1016/j.febslet.2008.05.052
Khodaei, L., and Long, T. A. F. (2019). Kin recognition and co-operative foraging in Drosophila melanogaster larvae. J. Evol. Biol. 32 (12), 1352–1361. doi:10.1111/jeb.13531
Kim, S. S., and Lee, S. V. (2019). Non-Coding RNAs in Caenorhabditis elegans aging. Mol. Cells 42 (5), 379–385. doi:10.14348/molcells.2019.0077
Kinser, H. E., and Pincus, Z. (2020). MicroRNAs as modulators of longevity and the aging process. Hum. Genet. 139 (3), 291–308. doi:10.1007/s00439-019-02046-0
Knupp, D., and Miura, P. (2018). CircRNA accumulation: A new hallmark of aging? Mech. Ageing Dev. 173, 71–79. doi:10.1016/j.mad.2018.05.001
Kong, Y., Wu, J., and Yuan, L. (2014). MicroRNA expression analysis of adult-onset Drosophila Alzheimer's disease model. Curr. Alzheimer Res. 11 (9), 1–891. doi:10.2174/1567205011666141001121416
L'Episcopo, F., Serapide, M. F., Tirolo, C., Testa, N., Caniglia, S., Morale, M. C., et al. (2011). A Wnt1 regulated Frizzled-1/β-Catenin signaling pathway as a candidate regulatory circuit controlling mesencephalic dopaminergic neuron-astrocyte crosstalk: Therapeutical relevance for neuron survival and neuroprotection. Mol. Neurodegener. 6, 49. doi:10.1186/1750-1326-6-49
Lai, W. F., Lin, M., and Wong, W. T. (2019). Tackling aging by using miRNA as a target and a tool. Trends Mol. Med. 25 (8), 673–684. doi:10.1016/j.molmed.2019.04.007
Leaman, D., Chen, P. Y., Fak, J., Yalcin, A., Pearce, M., Unnerstall, U., et al. (2005). Antisense-mediated depletion reveals essential and specific functions of microRNAs in Drosophila development. Cell 121 (7), 1097–1108. doi:10.1016/j.cell.2005.04.016
Liang, Y., Liang, Q., Qiao, L., and Xiao, F. (2020). MicroRNAs modulate drug resistance-related mechanisms in hepatocellular carcinoma. Front. Oncol. 10, 920. doi:10.3389/fonc.2020.00920
Liu, L., and Wu, C. F. (2014). Distinct effects of abelson kinase mutations on myocytes and neurons in dissociated Drosophila embryonic cultures: Mimicking of high temperature. PLoS One 9 (1), e86438. doi:10.1371/journal.pone.0086438
Liu, S., Wang, Z., Zhu, R., Wang, F., Cheng, Y., and Liu, Y. (2021). Three differential expression analysis methods for RNA sequencing: Limma, EdgeR, DESeq2. J. Vis. Exp. 175. doi:10.3791/62528
Liu, W., Ganguly, A., Huang, J., Wang, Y., Ni, J. D., Gurav, A. S., et al. (2019). Neuropeptide F regulates courtship in Drosophila through a male-specific neuronal circuit. Elife 8, e49574. doi:10.7554/eLife.49574
Love, M. I., Huber, W., and Anders, S. (2014). Moderated estimation of fold change and dispersion for RNA-seq data with DESeq2. Genome Biol. 15 (12), 550. doi:10.1186/s13059-014-0550-8
Luhur, A., Buddika, K., Ariyapala, I. S., Chen, S., and Sokol, N. S. (2017). Opposing post-transcriptional control of InR by FMRP and LIN-28 adjusts stem cell-based tissue growth. Cell Rep. 21 (10), 2671–2677. doi:10.1016/j.celrep.2017.11.039
Magwire, M. M., Yamamoto, A., Carbone, M. A., Roshina, N. V., Symonenko, A. V., Pasyukova, E. G., et al. (2010). Quantitative and molecular genetic analyses of mutations increasing Drosophila life span. PLoS Genet. 6 (7), e1001037. doi:10.1371/journal.pgen.1001037
Manley, P. W., Huth, F., Moussaoui, S., and Schoepfer, J. (2022). A kinase inhibitor which specifically targets the ABL myristate pocket (STAMP), but unlike asciminib crosses the blood–brain barrier. Bioorg. Med. Chem. Lett. 59, 128577. doi:10.1016/j.bmcl.2022.128577
Memczak, S., Jens, M., Elefsinioti, A., Torti, F., Krueger, J., Rybak, A., et al. (2013). Circular RNAs are a large class of animal RNAs with regulatory potency. Nature 495 (7441), 333–338. doi:10.1038/nature11928
Mishan, M. A., Khazeei Tabari, M. A., Parnian, J., Fallahi, J., Mahrooz, A., and Bagheri, A. (2020). Functional mechanisms of miR-192 family in cancer. Genes Chromosom. Cancer 12, 722–735. doi:10.1002/gcc.22889
Mołoń, M., Dampc, J., Kula-Maximenko, M., Zebrowski, J., Mołoń, A., Dobler, R., et al. (2020). Effects of temperature on lifespan of Drosophila melanogaster from different genetic backgrounds: Links between metabolic rate and longevity. Insects 11 (8), 470. doi:10.3390/insects11080470
Musselman, L. P., Fink, J. L., and Baranski, T. J. (2019). Similar effects of high-fructose and high-glucose feeding in a Drosophila model of obesity and diabetes. PLoS One 14 (5), e0217096. doi:10.1371/journal.pone.0217096
Nainu, F., Salim, E., Asri, R. M., Hori, A., and Kuraishi, T. (2019). Neurodegenerative disorders and sterile inflammation: Lessons from a Drosophila model. J. Biochem. 166 (3), 213–221. doi:10.1093/jb/mvz053
Neely, G. G., Kuba, K., Cammarato, A., Isobe, K., Amann, S., Zhang, L., et al. (2010). A global in vivo Drosophila RNAi screen identifies NOT3 as a conserved regulator of heart function. Cell 141 (1), 142–153. doi:10.1016/j.cell.2010.02.023
Nelson, C., Ambros, V., and Baehrecke, E. H. (2014). miR-14 regulates autophagy during developmental cell death by targeting ip3-kinase. Mol. Cell 256 (3), 376–388. doi:10.1016/j.molcel.2014.09.011
Nesler, K. R., Sand, R. I., Symmes, B. A., Pradhan, S. J., Boin, N. G., Laun, A. E., et al. (2013). The miRNA pathway controls rapid changes in activity-dependent synaptic structure at the Drosophila melanogaster neuromuscular junction. PLoS One 8 (7), e68385. doi:10.1371/journal.pone.0068385
Nesler, K. R., Starke, E. L., Boin, N. G., Ritz, M., and Barbee, S. A. (2016). Presynaptic CamKII regulates activity-dependent axon terminal growth. Mol. Cell Neurosci. 76, 33–41. doi:10.1016/j.mcn.2016.08.007
Perry, N., Volin, M., and Toledano, H. (2017). microRNAs in Drosophila regulate cell fate by repressing single mRNA targets. Int. J. Dev. Biol. 61 (3-4-5), 165–170. doi:10.1387/ijdb.160271ht
Ren, S., Lin, P., Wang, J., Yu, H., Lv, T., Sun, L., et al. (2020). Circular RNAs: Promising molecular biomarkers of human aging-related diseases via functioning as an miRNA sponge. Mol. Ther. Methods Clin. Dev. 18, 215–229. doi:10.1016/j.omtm.2020.05.027
Reynolds, J. A., Peyton, J. T., and Denlinger, D. L. (2017). Changes in microRNA abundance may regulate diapause in the flesh fly, Sarcophaga bullata. Insect Biochem. Mol. Biol. 84, 1–14. doi:10.1016/j.ibmb.2017.03.002
Robins, H., Li, Y., and Padgett, R. W. (2005). Incorporating structure to predict microRNA targets. Proc. Natl. Acad. Sci. U.S.A. 102 (11), 4006–4009. doi:10.1073/pnas.0500775102
Robinson, M. D., McCarthy, D. J., and Smyth, G. K. (2010). edgeR: a Bioconductor package for differential expression analysis of digital gene expression data. Bioinformatics 26 (1), 139–140. doi:10.1093/bioinformatics/btp616
Ruan, Y., Li, Z., Shen, Y., Li, T., Zhang, H., and Guo, J. (2020). Functions of circular RNAs and their potential applications in gastric cancer. Expert Rev. Gastroenterol. Hepatol. 14 (2), 85–92. doi:10.1080/17474124.2020.1715211
Sanz, A., Fernández-Ayala, D. J., Stefanatos, R. K., and Jacobs, H. T. (2010). Mitochondrial ROS production correlates with, but does not directly regulate lifespan in Drosophila. Aging (Albany NY) 2 (4), 200–223. doi:10.18632/aging.100137
Shi, Z., Lim, C., Tran, V., Cui, K., Zhao, K., and Chen, X. (2020). Single-cyst transcriptome analysis of Drosophila male germline stem cell lineage. Development 147 (8), dev184259. doi:10.1242/dev.184259
Soler Beatty, J., Molnar, C., Luque, C. M., de Celis, J. F., and Martín-Bermudo, M. D. (2021). EGFRAP encodes a new negative regulator of the EGFR acting in both normal and oncogenic EGFR/Ras-driven tissue morphogenesis. PLoS Genet. 17 (8), e1009738. doi:10.1371/journal.pgen.1009738
Song, C., Zhu, C., Wu, Q., Qi, J., Gao, Y., Zhang, Z., et al. (2017). Metabolome analysis of effect of aspirin on Drosophila lifespan extension. Exp. Gerontol. 95, 54–62. doi:10.1016/j.exger.2017.04.010
Stallone, G., Infante, B., Prisciandaro, C., and Grandaliano, G. (2019). mTOR and aging: An old fashioned dress. Int. J. Mol. Sci. 20 (11), 2774. doi:10.3390/ijms20112774
Suh, Y. S., Bhat, S., Hong, S. H., Shin, M., Bahk, S., Cho, K. S., et al. (2015). Genome-wide microRNA screening reveals that the evolutionary conserved miR-9a regulates body growth by targeting sNPFR1/NPYR. Nat. Commun. 6, 7693. doi:10.1038/ncomms8693
Sun, K., Jee, D., de Navas, L. F., Duan, H., and Lai, E. C. (2015). Multiple in vivo biological processes are mediated by functionally redundant activities of Drosophila mir-279 and mir-996. PLoS Genet. 11 (6), e1005245. doi:10.1371/journal.pgen.1005245
Surguchov, A., Emamzadeh, F. N., and Surguchev, A. A. (2019). Amyloidosis and longevity: A lesson from plants. Biol. (Basel) 8 (2), 43. doi:10.3390/biology8020043
Thibault, O., Gant, J. C., and Landfield, P. W. (2007). Expansion of the calcium hypothesis of brain aging and Alzheimer's disease: Minding the store. Aging Cell 6 (3), 307–317. doi:10.1111/j.1474-9726.2007.00295.x
Tower, J. (2019). Drosophila flies in the face of aging. J. Gerontol. A Biol. Sci. Med. Sci. 74 (10), 1539–1541. doi:10.1093/gerona/glz159
Ulgherait, M., Chen, A., McAllister, S. F., Kim, H. X., Delventhal, R., Wayne, C. R., et al. (2020). Circadian regulation of mitochondrial uncoupling and lifespan. Nat. Commun. 11 (1), 1927. doi:10.1038/s41467-020-15617-x
Ullah, M., Ng, N. N., Concepcion, W., and Thakor, A. S. (2020). Emerging role of stem cell-derived extracellular microRNAs in age-associated human diseases and in different therapies of longevity. Ageing Res. Rev. 57, 100979. doi:10.1016/j.arr.2019.100979
Varet, H., Brillet-Gueguen, L., Coppee, J. Y., and Dillies, M. A. (2016). SARTools: A DESeq2-and EdgeR-based R pipeline for comprehensive differential analysis of RNA-seq data. PLoS One 11 (6), e0157022. doi:10.1371/journal.pone.0157022
Varghese, J., and Cohen, S. M. (2007). microRNA miR-14 acts to modulate a positive autoregulatory loop controlling steroid hormone signaling in Drosophila. Genes Dev. 21 (18), 2277–2282. doi:10.1101/gad.439807
Wang, J. Y., Yang, Y., Ma, Y., Wang, F., Xue, A., Zhu, J., et al. (2020). Potential regulatory role of lncRNA-miRNA-mRNA axis in osteosarcoma. Biomed. Pharmacother. 121, 109627. doi:10.1016/j.biopha.2019.109627
Wang, L., Chen, S., Liu, Y., Zhang, H., Ren, N., Ma, R., et al. (2020). The biological and diagnostic roles of MicroRNAs in meningiomas. Rev. Neurosci. 31 (7), 771–778. doi:10.1515/revneuro-2020-0023
Wang, M., Hu, Q., Lv, T., Wang, Y., Lan, Q., Xiang, R., et al. (2022). High-resolution 3D spatiotemporal transcriptomic maps of developing Drosophila embryos and larvae. Dev. Cell 57 (10), 1271–1283.e4. doi:10.1016/j.devcel.2022.04.006
Wang, P., Xu, L. L., Zheng, X. B., Hu, Y. T., Zhang, J. F., Ren, S. S., et al. (2020). Correlation between the expressions of circular RNAs in peripheral venous blood and clinicopathological features in hepatocellular carcinoma. Ann. Transl. Med. 8 (6), 338. doi:10.21037/atm.2020.02.134
Wang, Q., Cai, J., Fang, C., Yang, C., Zhou, J., Tan, Y., et al. (2018). Mesenchymal glioblastoma constitutes a major ceRNA signature in the TGF-beta pathway. Theranostics 8 (17), 4733–4749. doi:10.7150/thno.26550
Watanabe, L. P., and Riddle, N. C. (2019). New opportunities: Drosophila as a model system for exercise research. J. Appl. Physiol. 127 (2), 482–490. doi:10.1152/japplphysiol.00394.2019
Weigelt, C. M., Sehgal, R., Tain, L. S., Cheng, J., Esser, J., Pahl, A., et al. (2020). An insulin-sensitive circular RNA that regulates lifespan in Drosophila. Mol. Cell 79 (2), 268–279.e5. doi:10.1016/j.molcel.2020.06.011
Wessels, H. H., Lebedeva, S., Hirsekorn, A., Wurmus, R., Akalin, A., Mukherjee, N., et al. (2019). Global identification of functional microRNA-mRNA interactions in Drosophila. Nat. Commun. 10 (1), 1626. doi:10.1038/s41467-019-09586-z
Westholm, J. O., Miura, P., Olson, S., Shenker, S., Joseph, B., Sanfilippo, P., et al. (2014). Genome-wide analysis of drosophila circular RNAs reveals their structural and sequence properties and age-dependent neural accumulation. Cell Rep. 9 (5), 1966–1980. doi:10.1016/j.celrep.2014.10.062
Xu, P., Vernooy, S. Y., Guo, M., and Hay, B. A. (2003). The Drosophila microRNA Mir-14 suppresses cell death and is required for normal fat metabolism. Curr. Biol. 13 (9), 790–795. doi:10.1016/s0960-9822(03)00250-1
Xue, B., Chuang, C. H., Prosser, H. M., Fuziwara, C. S., Chan, C., Sahasrabudhe, N., et al. (2021). miR-200 deficiency promotes lung cancer metastasis by activating Notch signaling in cancer-associated fibroblasts. Genes Dev. 35 (15-16), 1109–1122. doi:10.1101/gad.347344.120
Xue, L., Su, D., Li, D., Gao, W., Yuan, R., and Pang, W. (2019). Retraction note to: miR-200 regulates epithelial-mesenchymal transition in anaplastic thyroid cancer via EGF/EGFR signaling. Cell Biochem. Biophys. 77 (3), 277. doi:10.1007/s12013-019-00879-0
Yang, D., Lian, T., Tu, J., Gaur, U., Mao, X., Fan, X., et al. (2016). LncRNA mediated regulation of aging pathways in Drosophila melanogaster during dietary restriction. Aging (Albany NY) 8 (9), 2182–2203. doi:10.18632/aging.101062
Yang, Y., Xu, S., Xia, L., Wang, J., Wen, S., Jin, P., et al. (2009). The bantam microRNA is associated with drosophila fragile X mental retardation protein and regulates the fate of germline stem cells. PLoS Genet. 5 (4), e1000444. doi:10.1371/journal.pgen.1000444
Young, M. D., Wakefield, M. J., Smyth, G. K., and Oshlack, A. (2010). Gene ontology analysis for RNA-seq: Accounting for selection bias. Genome Biol. 11 (2), R14. doi:10.1186/gb-2010-11-2-r14
Yue, B., Wang, J., Song, C., Wu, J., Cao, X., Huang, Y., et al. (2019). Biogenesis and ceRNA role of circular RNAs in skeletal muscle myogenesis. Int. J. Biochem. Cell Biol. 117, 105621. doi:10.1016/j.biocel.2019.105621
Zeng, H., Lu, B., Zamponi, R., Yang, Z., Wetzel, K., Loureiro, J., et al. (2018). mTORC1 signaling suppresses Wnt/β-catenin signaling through DVL-dependent regulation of Wnt receptor FZD level. Proc. Natl. Acad. Sci. U. S. A. 115 (44), E10362–E10369. doi:10.1073/pnas.1808575115
Zha, F., Qu, X., Tang, B., Li, J., Wang, Y., Zheng, P., et al. (2019). Long non-coding RNA MEG3 promotes fibrosis and inflammatory response in diabetic nephropathy via miR-181a/Egr-1/TLR4 axis. Aging (Albany NY) 11 (11), 3716–3730. doi:10.18632/aging.102011
Zhang, R., Du, J., Zhao, X., Wei, L., and Zhao, Z. (2021a). Regulation of circadian behavioural output via clock-responsive miR-276b. Insect Mol. Biol. 30 (1), 81–89. doi:10.1111/imb.12679
Zhang, R., Zhao, X., Du, J., Wei, L., and Zhao, Z. (2021b). Regulatory mechanism of daily sleep by miR-276a. FASEB J. 35 (1), e21222. doi:10.1096/fj.202001220R
Zhang, Y., Yu, F., Bao, S., and Sun, J. (2019). Systematic characterization of circular RNA-associated CeRNA network identified novel circRNA biomarkers in Alzheimer's disease. Front. Bioeng. Biotechnol. 7, 222. doi:10.3389/fbioe.2019.00222
Zhao, L., Hu, K., Cao, J., Wang, P., Li, J., Zeng, K., et al. (2019). lncRNA miat functions as a ceRNA to upregulate sirt1 by sponging miR-22-3p in HCC cellular senescence. Aging (Albany NY) 11 (17), 7098–7122. doi:10.18632/aging.102240
Zhou, R. S., Zhang, E. X., Sun, Q. F., Ye, Z. J., Liu, J. W., Zhou, D. H., et al. (2019). Integrated analysis of lncRNA-miRNA-mRNA ceRNA network in squamous cell carcinoma of tongue. BMC Cancer 19 (1), 779. doi:10.1186/s12885-019-5983-8
Keywords: Drosophila, ageing, ceRNA network, correlation of mRNA/protein, circRNA, lncRNA
Citation: Yang D, Xiao F, Li J, Wang S, Fan X, Ni Q, Li Y, Zhang M, Yan T, Yang M and He Z (2023) Age-related ceRNA networks in adult Drosophila ageing. Front. Genet. 14:1096902. doi: 10.3389/fgene.2023.1096902
Received: 26 November 2022; Accepted: 10 February 2023;
Published: 28 February 2023.
Edited by:
Elena G. Pasyukova, Institute of Molecular Genetics (RAS), RussiaReviewed by:
Hua Bai, Iowa State University, United StatesAaron Michael Tarone, Texas A&M University, United States
Copyright © 2023 Yang, Xiao, Li, Wang, Fan, Ni, Li, Zhang, Yan, Yang and He. This is an open-access article distributed under the terms of the Creative Commons Attribution License (CC BY). The use, distribution or reproduction in other forums is permitted, provided the original author(s) and the copyright owner(s) are credited and that the original publication in this journal is cited, in accordance with accepted academic practice. No use, distribution or reproduction is permitted which does not comply with these terms.
*Correspondence: Mingyao Yang, eWFuZ21pbmd5YW9Ac2ljYXUuZWR1LmNu; Zhi He, emhpaGVAc2ljYXUuZWR1LmNu
†These authors have contributed equally to this work