- 1Zhejiang University—University of Edinburgh Institute, Zhejiang University, Haining, Zhejiang, China
- 2Deanery of Biomedical Sciences, University of Edinburgh, Edinburgh, United Kingdom
- 3The Second Affiliated Hospital of Zhejiang University, Hangzhou, Zhejiang, China
- 4Biomedical and Health Translational Centre of Zhejiang Province, Haining, Zhejiang, China
Unveiling how genetic variations lead to phenotypic variations is one of the key questions in evolutionary biology, genetics, and biomedical research. Deep mutational scanning (DMS) technology has allowed the mapping of tens of thousands of genetic variations to phenotypic variations efficiently and economically. Since its first systematic introduction about a decade ago, we have witnessed the use of deep mutational scanning in many research areas leading to scientific breakthroughs. Also, the methods in each step of deep mutational scanning have become much more versatile thanks to the oligo-synthesizing technology, high-throughput phenotyping methods and deep sequencing technology. However, each specific possible step of deep mutational scanning has its pros and cons, and some limitations still await further technological development. Here, we discuss recent scientific accomplishments achieved through the deep mutational scanning and describe widely used methods in each step of deep mutational scanning. We also compare these different methods and analyze their advantages and disadvantages, providing insight into how to design a deep mutational scanning study that best suits the aims of the readers’ projects.
Introduction
Since Mendel’s experiments with peas (Mendel, 1865) laid the foundation of modern genetics about 150 years ago, our ability to read, write, and rewrite genetic information has grown prominently. In comparison, our ability to understand genetic information—i.e., mapping genetic variations to phenotypic variations—is very limited. For instance, the effects of the vast majority of human genetic variations are unknown (Riesselman et al., 2018; Frazer et al., 2021; Lappalainen and MacArthur, 2021). In light of this challenge, deep mutational scanning (DMS) was developed to systematically quantify the effects of genetic variations on a large scale, with high efficiency and relatively low cost (Fowler et al., 2011; Hietpas et al., 2012). DMS, also known as massively parallel mutagenesis (Fowler et al., 2011; Fowler and Fields, 2014), involves making a comprehensive mutant library followed by high-throughput phenotyping and deep-sequencing of the mutant libraries before and after selection (Figure 1A).
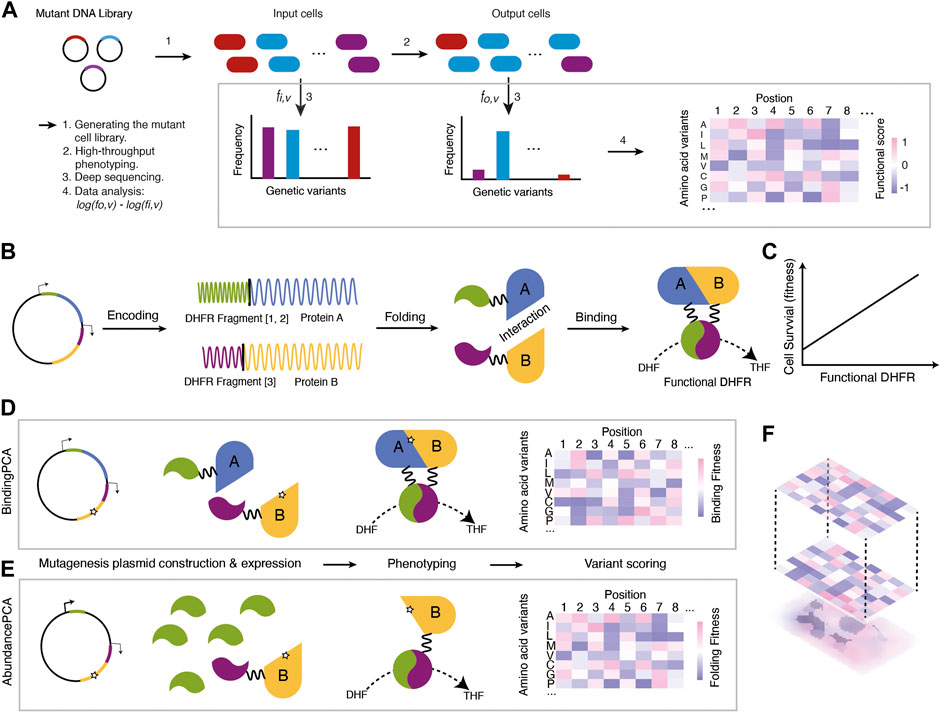
FIGURE 1. An overview of the DMS procedure (A) A mutant DNA library is transformed into cell types of interest to generate a mutant cell library. Then, the mutant cell library goes through high-throughput phenotyping where cells carrying functional variants are enriched (cells filled with blue) while those with detrimental variants are depleted (cells filled with red or purple). Genetic variants are extracted and sequenced to calculate the relative enrichment changes before and after selection. Finally, the enrichment scores are analysed as the functional scores of mutations (B) Protein-Fragment Complementation Assay (PCA) (C) The underlying assumption is that the concentrations of functional DHFR are linearly related to cell survival (fitness) (D) BindingPCA captures mutational effects on both stability and protein-protein interactions without distinguishing them (E) AbundancePCA captures mutational effects on stability (F) ddPCA combines BindingPCA and AbundancePCA and enables the inference of the bbphysical effects of mutations by quantifying and comparing phenotypic effects.
DMS has been widely used in many biological systems, allowing breakthroughs in biological and biomedical research since its first introduction (Fowler et al., 2010; Fowler and Fields, 2014). For example, many human disease-related genetic variants with unknown significance have been classified as either benign or detrimental systematically (Majithia et al., 2016; Findlay et al., 2018; Matreyek et al., 2018; Mighell et al., 2018; Bridgford et al., 2020; Mighell et al., 2020; Hanna et al., 2021; Seuma et al., 2021). Genetic interaction patterns and the underlying biophysical mechanisms have been revealed for both between genes (Diss and Lehner, 2018; Lite et al., 2020; Faure et al., 2022) and within the same gene (Olson et al., 2014; Li et al., 2016; Puchta et al., 2016; Sarkisyan et al., 2016; Baeza-Centurion et al., 2019; Yoo et al., 2020; Faure et al., 2022). Also, using the positional genetic interaction scores generated from DMS experiments, protein structures can be accurately predicted (Rollins et al., 2019; Schmiedel and Lehner, 2019). The release of the DMS data on SARS-Cov2 spike protein RBD within a year of the SARS-Cov2 outbreak (Starr et al., 2020) demonstrates that DMS is a powerful technique to address pressing questions in a relatively short period. The data accurately captured some SARS-Cov2 mutations that became prevalent in the later stage of the pandemic (Starr et al., 2020; Starr et al., 2022). Furthermore, DMS data on immune-escape mutants of various SARS-Cov2 variants (Greaney et al., 2021a; Greaney et al., 2021b; Javanmardi et al., 2022) guides better vaccine design.
A typical DMS experiment involves three steps: 1) generating a genetic mutant library; 2) performing a high-throughput phenotyping assay; 3) and deep sequencing and data analysis. Several good reviews on designing DMS experiments were published (Fowler and Fields, 2014; Shin and Cho, 2015; Starita and Fields, 2015; Matuszewski et al., 2016; Cao et al., 2022) in the early days of DMS. However, many more technical options became available in DMS thanks to the fast-developing technology in gene synthesis, sequencing technologies and high-throughput phenotyping methods since the reviews. The recent reviews (Weile and Roth, 2018; Kemble et al., 2019; Kinney and McCandlish, 2019; Narayanan and Procko, 2021; Hanning et al., 2022) in light of the DMS boom mostly focus on specific biological insights—for example, how the technique enabled breakthroughs in human genetics (Weile and Roth, 2018), on transcriptional factors (TF) and cis-regulatory elements (CRE) (Kinney and McCandlish, 2019), on viral protein and receptors (Narayanan and Procko, 2021) or therapeutic antibody engineering (Hanning et al., 2022). Kemble et al. gave a comprehensive overview of genotype-phenotype mapping (Kemble et al., 2019) enabled by DMS technology. While the DMS strategy is straightforward, each step of the technique can be tricky and complicated to generate clean and meaningful data, as it involves various synthetic biology and massive parallel assays. In addition, genetic variants from DMS experiments are of low complexity but are of a big amount that needs special attention for statistical analysis. We notice a lack of such up-to-date reviews on the insights in technical aspects.
In this review, we give an up-to-date overview of the DMS experiment (Figure 1A) with a specific focus on recently developed techniques in mutation library generation, high-throughput methods, and data analysis. Our motivation is to guide the readers on selecting the most appropriate techniques for a DMS project aim. Finally, we will discuss the ongoing efforts and challenges in improving DMS accuracy and scope.
Generating a genetic mutant library
A genetic mutant library is often first synthesized as a pool of oligos and amplified as a library of linear gene blocks. Then the amplified dsDNA is ligated to the expression vector backbones to substitute the wild-type region of the gene to be mutated. The ligation mix is introduced to the cloning cell lines to be amplified and extracted as a plasmid mutant library, which will be introduced to the destination cells (i.e., via transformation) for the next step—high throughput phenotyping assay. While most of the steps mentioned above follow regular molecular cloning procedures, mutagenesis in the very first step is not trivial and requires careful design. In this section, we will discuss the most widely used methods in designing and creating mutant libraries so that the readers can determine the optimal method to suit their research needs.
Error-prone PCR
Error-prone PCR is relatively cheap and easy to perform. It uses low-fidelity DNA polymerases to incorporate mistakes during the DNA amplification, and mutation rates can be modified by PCR conditions like different concentrations of manganese chloride and dNTP (Lin-Goerke et al., 1997; Shafikhani et al., 1997). The technique has been widely used in making random mutations for directed evolution experiments (Giver et al., 1998; Moore et al., 2000) and recently for DMS studies (Sarkisyan et al., 2016; Seuma et al., 2021; Faure et al., 2022). However, mutations generated via error-prone PCR are not completely random due to mutation biases of polymerases. For example, Taq polymerase-based mutation rates from A/T are much higher than from C/G (Shafikhani et al., 1997; Wan et al., 1998). Nowadays, error-prone PCR is made easier using commercial kits with mixes of engineered polymerases (Vanhercke et al., 2005), generating reduced biases. However, judging from the DMS data (Faure et al., 2022), mutation biases are only partially removable even with commercial kits. To be noted, error-prone PCR is suitable for generating comprehensive nucleotide-level mutations but not for all possible single amino acid substitutions for each codon. To achieve all possible 19 amino acid substitutions per codon, two consecutive nucleotides of a codon must often be mutated simultaneously. But such a mutation rate will likely hit two or more codons simultaneously, creating a mutation library mixed with single amino acid substitutions and multiple amino acid substitutions.
PCR with oligonucleotides containing mutations
Another commonly used method is a DMS library with a pool of oligos containing different mutations. Compared to the error-prone PCR, it is more costly but can generate a customized library with fewer biases. Oligonucleotides containing random mutations can be synthesized as a pool of doped oligos (Matteucci and Heyneker, 1983; Araya et al., 2012; Li et al., 2016; Puchta et al., 2016; Li et al., 2019; Wu et al., 2022) or oligos containing NNN triplets (sometimes NNS or NNK) (Hietpas et al., 2012; McLaughlin et al., 2012; Stiffler et al., 2015; Starr et al., 2017; Diss and Lehner, 2018; Hartman et al., 2018; Ahler et al., 2019; Starr et al., 2020; Park et al., 2022), where N represents any of the four nucleotide bases, S for G/C and K for G/T) targeting each codon. This strategy, combined with oligo pool synthesis technology like DropSynth (Plesa et al., 2018), allows construction of user-defined, scalable, and low-cost mutant libraries with comprehensive nucleotide or amino acid substitutions.
These oligos can be designed as doped oligos with each position incorporating a defined percentage of mutations (Starita and Fields, 2015) during the oligo synthesis. The pool of the long mutant oligos (up to 300 nt) can be used as DNA templates. These long oligos need to contain flanking wild-type sequences for primer binding, so they can be amplified and replace the wild-type sequences. On the other hand, short oligos with user-defined mutations or NNN triplets serve as primers. Mutations are introduced to the gene in a manner that is similar to site-directed mutagenesis. The oligos containing NNN triplets are more suited to create mutant libraries covering all possible single amino acid substitutions, while this doped oligo method also targets nucleotide-level mutations as error-prone PCR does. The disadvantage of using oligos with NNN triplets is that it often requires at least two consecutive PCR reactions to generate double amino acid substitutions.
Another popular primer-based method is the nicking mutagenesis (Wrenbeck et al., 2016; Faure et al., 2022), which is developed from a method called Pfunkle (Firnberg and Ostermeier, 2012). Both methods use the circular dsDNA as the template and incorporate mutations using a mix of phosphorylated primers. To remove excessive wild-type template, thymidine in the template is replaced with uracil and degraded after the mutagenesis using the uracil DNA glycosylase and exonuclease III (Exo III) (Firnberg and Ostermeier, 2012). For the same purpose, nicking mutagenesis uses a pair of endonucleases (NtBbvCl and NbBbvCl) that nick one strand of the template dsDNA at a time.
Firstly, a 5’ phosphorylated mutant oligo pool as primers is applied to the NtBbvCI-treated ssDNA template to generate the second-strand DNA with mutations. Then, a second phosphorylated primer without mutations will synthesize the complementary strand for each mutated genetic variant, using the PCR-derived strand with mutations as templates. While other primer-based mutagenesis methods require a pair of primers per mutant, both Pfunkle and nicking mutagenesis requires only one primer per mutant, greatly reducing the cost of oligo synthesis. But to perform Pfunkle or nicking mutagenesis, one needs to ensure high-quality circular DNA and careful design of the primer libraries with a freshly phosphorylated state. Nevertheless, nicking mutagenesis has been rising in popularity for achieving codon-level saturation mutagenesis recently.
Generating a library with mutations at the endogenous genetic loci
For DMS studies aimed at endogenous genetic loci, CRISPR-based technologies (Doudna and Charpentier, 2014; Rees and Liu, 2018) are used. The mutant library can be designed as a sgRNA library targeting intended genetic loci (Wang et al., 2014; Hart et al., 2015; Sadhu et al., 2018; Hanna et al., 2021) or as a donor DNA mutant library for homology-directed repair (HDR) template (Findlay et al., 2014; Sharon et al., 2018; Choudhury et al., 2020; Shen et al., 2022). The donor DNA mutant library can be generated using the methods mentioned above and combined into the backbone flanked by the recombination arms and necessary components. Simultaneous use of two gRNAs also enables multiplexed mutagenesis (Campa et al., 2019). Yet, compared to the ectopic expression of a mutation library, there are much fewer DMS studies performed at the endogenous loci due to additional technical limitations—including sgRNA-dependent uneven editing efficiencies (Wang et al., 2014; Bassalo et al., 2018; Choudhury et al., 2020), low HDR efficiency (Findlay et al., 2014), and high incidences of undetected off-target mutations and editing biases (Cui and Bikard, 2016; Zerbini et al., 2017). The challenges are even more prominent when the mammalian cell genome is the target of saturation mutagenesis (Rees and Liu, 2018).
To sum up, different methods of generating mutation libraries have their own pros and cons (Table 1). The choice of the method should be determined primarily by the purpose. For instance, should the experiment target nucleotide or codon level, single or combinations of mutations? How extensive the mutant library should be, and should the mutations be ectopically expressed or integrated into the genome?
High-throughput phenotyping
After obtaining the mutant plasmid library or gene blocks via various molecular cloning steps, including amplification, ligation, etc., the library is delivered (via transformation, transfection, or transduction) to the cell types of interest for high-throughput quantification of the phenotypes coupled by the deep sequencing. These phenotyping assays are usually designed to enrich functional genetic variants while depleting the detrimental variants in a bulk experiment (Fowler and Fields, 2014; Olson et al., 2014; Bandyopadhyay et al., 2020) or via reporter-based cell sorting (Starr et al., 2017; Matreyek et al., 2018; Li et al., 2019; Park et al., 2022).
Measured phenotypes can be divided into two main categories: 1) Fitness based on the reproduction rate of cells (Li et al., 2016; Puchta et al., 2016; Domingo et al., 2018) or 2) measurement of the molecular function (abundance, binding, or activity) (Araya et al., 2012; Olson et al., 2014; Sarkisyan et al., 2016; Matreyek et al., 2018; Li et al., 2019; Tack et al., 2021; Faure et al., 2022). In this section, we will describe and compare techniques used in these two categories and another recently developed method that can decompose molecular functions via the fitness-based assay.
Fitness assays
Fitness competition is the most straightforward and economical approach for a high-throughput functional selection. Its logic is that if the gene product is required for cell survival or reproduction, cells carrying functional genetic variants will enrich. In contrast, detrimental variants will deplete over time in a culture medium. As a result, frequency changes of genetic variants can be calculated as fitness scores (Domingo et al., 2018). This strategy does not require special equipment, making it easy to conduct. However, the obtained fitness scores may not necessarily be linearly related to the molecular mechanisms of the mutations, making it complicated to acquire mechanistic insight into the mutational effects on the molecular level (Stein et al., 2019). Besides, marginally detrimental effects on molecular functions may be masked due to the non-linear relationship between fitness and molecular function (Soskine and Tawfik, 2010; Stiffler et al., 2015). It also needs to be noted that mutational effects often alter in different environments (Stiffler et al., 2015; Domingo et al., 2018; Chen et al., 2022).
Therefore, it is essential to select an optimal condition that either reflects the physiological situation best (Starita et al., 2015; Braun et al., 2018; Cantor et al., 2018; Hartman et al., 2018; Staller et al., 2018; Ahler et al., 2019; Matreyek et al., 2020; Mighell et al., 2020) to unveil disease-causing mutations or evolutionary paths of mutations. On the other hand, to easily infer biophysical effects, the fitness assay conditions should be selected to be linearly related to the molecular function (Domingo et al., 2018; Li et al., 2019; Leander et al., 2020; Starr et al., 2020; Faure et al., 2022).
Functional assays
Using the protein stability or binding affinity as a phenotype (Araya et al., 2012; Olson et al., 2014; Starr et al., 2020; Faure et al., 2022) is another widely used method to evaluate mutational effects for a protein-coding gene. This approach can capture essential biophysical effects of mutations and give more mechanistic insights into mutations.
Stability assays often involve tagging the target protein to a reporter, like the green fluorescent protein (GFP) as an indicator of the protein stability (Li et al., 2019; Leander et al., 2020; Matreyek et al., 2020; Park et al., 2022). Cells can be sorted based on the fluorescence levels into several bins, followed by deep sequencing of each sorted subpopulation (Peterman and Levine, 2016; Matreyek et al., 2018). Then, each mutant’s mean fluorescence level is calculated based on the frequencies of each genetic variant in each sorted bin.
In vitro display methods, such as phage display (Araya et al., 2012), yeast display (Klesmith et al., 2017; Starr et al., 2017; Cao et al., 2022), and mRNA display (Olson et al., 2014), detect frequency changes of genetic variants based on the binding affinity of the protein to its ligands. Although the experimental results from such an approach reveal the functional effects of mutations, it does not immediately indicate whether mutations affect the function by changing the protein stability or binding affinity, which is termed biophysical ambiguity hereafter. Nevertheless, it is crucial to resolve the biophysical ambiguity of mutations if we want to predict the combined effects of mutations (Otwinowski et al., 2018; Li and Lehner, 2020) accurately. To overcome this, approaches like combining the binding affinity-based functional assay and the stability-based assay (Starr et al., 2020), or predicting mutants’ biophysical effects by analyzing how mutations combine based on a single assay (Otwinowski et al., 2018) have been shown.
Performing two sets of different experiments (Starr et al., 2020) are often troublesome while predicting folding and binding energy changes based on the protein structures (Capriotti et al., 2005; Schymkowitz et al., 2005; Zhang et al., 2020) is not as accurate as experiment results. Recently, a method called ddPCA (Faure et al., 2022) that uses a relatively simple experimental approach to solve the biophysical ambiguity has been developed, which we will discuss in the following part.
ddPCA: Untangling biophysical parameters with the fitness assays
The method called Double Deep Protein-Fragment Complementation Assay (ddPCA) (Faure et al., 2022) is based on the protein-fragment complementation (PCA) assay (Tarassov et al., 2008). In ddPCA, the expression ratios of dihydrofolate reductase (DHFR) fragments are tweaked into two sets so that one assay can detect mutational effects on the stability of the protein (AbundancePCA) while the other detects both stability and protein-protein interactions (BindingPCA) (Figures 1B–F).
BindingPCA uses the traditional PCA method in which two interacting partners are each tagged with interacting partners are each tagged with DHFR[1,2] and DHFR[3] fragments (Figure 1D). Mutations that affect the binding affinity to the ligand and/or the protein stability will reduce the functional DHFR concentration inside the cells and therefore minimize cell survival (fitness) (Figures 1B, C). AbundancePCA, on the other hand, only has one protein-coding gene tagged to one fragment of DHFR (DHFR[3]) while overexpressing the other fragment DHFR[1,2]. This allows the cellular fitness to be solely determined by the limiting concentration of the protein tagged with DHFR fragment (i.e., DHFR [1,2], which reflects the protein stability (Figure 1E). The combination of the BindingPCA and the AbundancePCA serves to determine biophysical effects and resolve biophysical ambiguities (Figure 1F). Compared to other experimental approaches, ddPCA is a much simpler approach to unveil stability and binding affinity of mutations because both AbundancePCA and BindingPCA use the same fitness selection system. ddPCA has been applied to several allosteric proteins and resolves the ‘biophysical ambiguities’, as well as pinpointing allosteric sites systematically (Faure et al., 2022; Weng et al., 2022).
Besides the methods mentioned above, enzyme kinetics can be measured in a dynamic system using microfluidics technology. For instance, the High-Throughput Microfluidic Enzyme Kinetics (HT-MEK) in a DMS experiment allows the systematic investigation of enzymes in an automatically valved microfluidics expression system (Markin et al., 2021).
Deep sequencing and data analysis
Deep-sequencing of the genetic variants for both the input (before phenotyping) and the output (after phenotyping) follows the high-throughput phenotyping. Samples from a DMS experiment are special in that there are up to tens of thousands of genetic variants. Yet, they are with a low frequency of mutations at each position (sometimes as low as 0.1%) in an overall very homogenous sequence. Considering that genotype-phenotype mapping depends on frequencies of each genetic variant that are often only one or two hamming distances away from each other, choosing a high-throughput sequencing platform with high accuracy is especially important for a DMS study.
Sequencing platforms
The most widely used platform in DMS studies has been the Illumina HiSeq platforms for their relatively lower error rates compared to the third-generation sequencing platforms (PacBio or Nanopore sequencing) and the higher cost-effectiveness (cost/base pair) compared to Illumina MiSeq (Shendure et al., 2017; Pfeiffer et al., 2018). However, the HiSeq platforms have a sequencing read length limitation to 300 nt. This makes the identification of long-range epistatic interactions challenging if the mutated region exceeds the length limit. To overcome this, barcoding genetic variants can be applied, first to associate genetic variants with barcodes using Miseq or PacBio sequencing (Puchta et al., 2016; Starr et al., 2020; Wu et al., 2022) and then to perform deep sequencing of the barcodes using HiSeq.
Recently, UMI-based Nanopore sequencing (Zurek et al., 2020) and new circular consensus sequencing (CCS) method using PacBio (Wenger et al., 2019) were developed to increase the accuracies of long-read sequencing platforms to >99.5% (Zurek et al., 2020; Karst et al., 2021). This suggests that Nanopore or PacBio may completely substitute HiSeq for DMS studies in the future.
Experiment design and library preparation for sequencing are utterly important to obtain high-quality data, regardless of the sequencing platforms used. One should always 1) start with a sufficient number of molecules per variant in each mutant library; 2) have multiple independent biological replicates; and 3) avoid experimental bottlenecks, over-sequencing or under-sequencing. Especially, it is essential not to over-sequence as that would impactfully hinder accurate prediction of the variant frequencies (Faure et al., 2020). Read-depths should not be more than that of total expected molecule numbers before generating sequencing libraries but also need to be sufficiently bigger than the expected unique counts of genetic variants.
Data analysis
The phenotype of each genetic variant is often quantified as the normalized relative enrichment scores from the aggregated count data (i.e., after versus before selection) compared to that of the wild type, as shown in Eq. 1 below.
Fv,output and Fv,input are the frequencies of a given variant v after selection (output) and before selection (input) respectively, and Ev is the normalized enrichment score of the variant to the wild type. When the phenotype is based on the reporter fluorescence intensity and cell sorting (Starr et al., 2020), functional scores as the mean fluorescence signals are estimated based on the variant counts in each sorted bin and the bin fluorescence parameters (Peterman and Levine, 2016).
To estimate mutants’ phenotypes from the sequencing data accurately and to obtain enough statistical power, correct error detection and propagation are essential. However, it is not a simple task as there are many sources of errors in the typical DMS dataset, including sequencing error, Poisson error, errors from the replicates, and stochastic error (Rubin et al., 2017). Enrich2 (Rubin et al., 2017) and a more recent software DiMSum (Faure et al., 2020) are two good statistical frameworks developed for DMS sequencing data to help users reliably quantify the data and perform error estimation. Enrich2 and DiMSum are both based on the Poisson-based sequence count distribution, and they both integrate the empirical variance into account to estimate the errors. However, the way they handle the empirical variance is different. For instance, Enrich2 takes the mix-effects from the empirical variance, while DiMSum introduced replicate-specific additive and multiplicative modifier terms from empirical variance. Thus, the error estimated from the two models differs (Faure et al., 2020). The only available and direct comparisons between the two statistical software are from Faure and others who developed DiMSum. Based on the 12 datasets examined, Enrich2 and DiMSum performed similarly well on the datasets with little overdispersion, but Enrich2 underestimates errors on the dataset with a lot of overdispersion (Faure et al., 2020). Still, DiMSum is not as widely used as Enrich2 in DMS data analysis, likely because it is still a relatively newly developed pipeline. Either error models from Enrich2 or DiMSum cannot capture systematic errors arising from the experiments, which need to be identified and judged by the researchers using diagnostic plots. After this step, one could select only reliable data based on the error thresholds for further analysis.
Relative mutational effects are often presented in a 2D map, with each x- and y-axis representing mutation position and substitution respectively, and the phenotype in a gradient of filled color as a heatmap (Figure 1). With such a descriptive figure giving an overview of the data, one can quickly judge which positions are more sensitive to mutations and whether certain types of substitutes are more acceptable than others. There are also tools developed to visualize both published and own DMS data. MaveVis, developed as part of the MaveDB (Esposito et al., 2019), allows users to generate heatmaps integrating the protein structural information for each position. It is available for both web-based interfaces and as an R package. Another web-based tool called dms-view (Hilton et al., 2020) can provide a quick exploration of the DMS data to look for specific mutations per site and in the context of protein 3D structure in an interactive manner. Compared to MaveVis, the advantage of dms-view is the integration of the protein 3D structure and logo generation based on mutational effects. However, local users cannot use the software as it is only web-based.
To obtain mechanistic insights into genotype-phenotype maps, machine-learning algorithms (Wang et al., 2014; Hart et al., 2015; Majithia et al., 2016; Klesmith et al., 2017; Rocklin et al., 2017; Weile et al., 2017; Gray et al., 2018; Staller et al., 2018; Song et al., 2021; Faure et al., 2022; Hsu et al., 2022; Leander et al., 2022) and deep learning algorithms (Sarkisyan et al., 2016; Gelman et al., 2021; Faure et al., 2022; Tareen et al., 2022; Vaishnav et al., 2022) are frequently used. Especially, a recently developed python package called MAVE-NN (Tareen et al., 2022) can unveil the one-dimensional latent phenotypes (i.e., a hidden type of biophysical parameter values) that are non-linearly linking genotypes to phenotypes, based on the neural-network algorithm. MAVE-NN has its limitations. For example, it cannot analyse DMS data with only single mutations or mutations affecting more than one type of expected biophysical parameters to reveal the latent phenotypes.
Discussion
Recent years have witnessed a boom in DMS applied to various coding and non-coding genes from many organisms, including viruses, bacteria, yeast, and mammalian cells. In this review, we presented an overview of DMS that combines synthetic biology, high-throughput phenotyping methods, and deep sequencing technology. We also showed the main steps in the DMS technique and compared different choices of designing mutation libraries, phenotyping assays, and sequencing platforms. Finally, by comparing different techniques, we gave brief guidance on selecting the most appropriate strategy according to different scientific questions and experimental models.
A high-quality DMS dataset not only provides important information on genotype-phenotype mapping for biomedical research, but also guides other research fields including structural biology, biophysics, and protein engineering. For instance, the very comprehensive single and double-mutant GB1 DMS dataset (Olson et al., 2014) enabled accurate prediction of the protein 3D structure (Schmiedel and Lehner, 2019) and biophysical effects of each mutation without doing the painstaking experiments (Gelman et al., 2021; Tareen et al., 2022). Also, the technology provides mechanistic insights into understanding and predicting mutational effects (Hsu et al., 2022), contributing to protein engineering and structure prediction (Rollins et al., 2019; Schmiedel and Lehner, 2019), biomedicine (Braun et al., 2018; Baeza-Centurion et al., 2019; Bridgford et al., 2020; Livesey and Marsh, 2020; Frazer et al., 2021) and evolution (Aakre et al., 2015; Li et al., 2016; Puchta et al., 2016; Starr et al., 2017; Domingo et al., 2018; Starr et al., 2020; Park et al., 2022; Starr et al., 2022). In light of accumulating DMS datasets and the challenge of reproducibility and source-data compilation, several pioneering labs in the field of massive parallel assays made an open-source platform called MaveDB (Esposito et al., 2019) available for DMS experiment data. By now (December 2022), more than a hundred DMS datasets have been listed in MaveDB that are available for download and analyse. An alliance called Atlas of Variant Effects (https://www.varianteffect.org) is also formed to maximise collaboration, benefits, and the influence of mutational scanning.
Still, there are limitations in DMS technology. Firstly, each DMS experiment could handle up to tens of thousands of mutations but not more. One of the limiting factors in scaling mutation libraries is transformation (transfection or transduction) efficiencies, as one does not want to generate a bottleneck by randomly sampling genetic variants that go into the destination cells. The number of successfully transformed (transfected or transduced) cells should be sufficiently higher than the library sizes to minimize the loss of some genetic variants during the transformation step. Secondly, performing DMS at the endogenous genomic loci of cells is still a big challenge. Nevertheless, endogenous DMS will become available soon with improved precision in genome editing technologies and transformation/transfection efficiencies. While DMS has been applied to various organisms, including humans, viruses, bacteria and yeast, interestingly, there is no DMS research on plant genes, even though mapping genotypes to phenotypes on the plant is both important and challenging (Voichek and Weigel, 2020; Deng et al., 2021). The reason could be the technical challenges in developing a high throughput phenotyping assay with the designed mutation pools.
To sum up, our ability to interpret genotypes is still lacking due to the complication of the genotype-phenotype maps (Domingo et al., 2019; Kinney and McCandlish, 2019). While sequencing technology is becoming more advanced and economical, ‘reading’ genetic codes has become the routine of many labs. We believe that DMS will become a laboratory routine in the near future together with further development in synthetic biology and sequencing technologies.
Author contributions
HW and XL wrote the manuscript together. This work is supported by the Young Scientists Fund of the National Natural Science Foundation of China (NSFC Grant No. 32100478).
Funding
XL is supported by the Young Scientists Fund of National Natural Science Foundation of China (NSFC Grant No.32100478). The study is also supported by the department (Zhejiang University-University of Edinburgh Institute) start-up funding and the seed-fund.
Acknowledgments
We thank members of the Li lab for comments on the manuscript.
Conflict of interest
The authors declare that the research was conducted in the absence of any commercial or financial relationships that could be construed as a potential conflict of interest.
Publisher’s note
All claims expressed in this article are solely those of the authors and do not necessarily represent those of their affiliated organizations, or those of the publisher, the editors and the reviewers. Any product that may be evaluated in this article, or claim that may be made by its manufacturer, is not guaranteed or endorsed by the publisher.
References
Aakre, C. D., Herrou, J., Phung, T. N., Perchuk, B. S., Crosson, S., and Laub, M. T. (2015). Evolving new protein-protein interaction specificity through promiscuous intermediates. Cell 163, 594–606. doi:10.1016/j.cell.2015.09.055
Ahler, E., Register, A. C., Chakraborty, S., Fang, L., Dieter, E. M., Sitko, K. A., et al. (2019). A combined approach reveals a regulatory mechanism coupling src’s kinase activity, localization, and phosphotransferase-independent functions. Mol. Cell 74, 393–408. e20. doi:10.1016/j.molcel.2019.02.003
Araya, C. L., Fowler, D. M., Chen, W., Muniez, I., Kelly, J. W., and Fields, S. (2012). A fundamental protein property, thermodynamic stability, revealed solely from large-scale measurements of protein function. Proc. Natl. Acad. Sci. U. S. A. 109, 16858–16863. doi:10.1073/pnas.1209751109
Baeza-Centurion, P., Miñana, B., Schmiedel, J. M., Valcárcel, J., and Lehner, B. (2019). Combinatorial genetics reveals a scaling law for the effects of mutations on splicing. Cell 176, 549–563. doi:10.1016/j.cell.2018.12.010
Bandyopadhyay, S., Bhaduri, S., Örd, M., Davey, N. E., Loog, M., and Pryciak, P. M. (2020). Comprehensive analysis of G1 cyclin docking motif sequences that control CDK regulatory potency in vivo. Curr. Biol. 30, 4454–4466. doi:10.1016/j.cub.2020.08.099
Bassalo, M. C., Garst, A. D., Choudhury, A., Grau, W. C., Oh, E. J., Spindler, E., et al. (2018). Deep scanning lysine metabolism in Escherichia coli. Mol. Syst. Biol. 14, e8371. doi:10.15252/msb.20188371
Braun, S., Enculescu, M., Setty, S. T., Cortés-López, M., de Almeida, B. P., Sutandy, F. X. R., et al. (2018). Decoding a cancer-relevant splicing decision in the RON proto-oncogene using high-throughput mutagenesis. Nat. Commun. 9, 3315–3318. doi:10.1038/s41467-018-05748-7
Bridgford, J. L., Lee, S. M., Lee, C. M. M., Guglielmelli, P., Rumi, E., Pietra, D., et al. (2020). Novel drivers and modifiers of MPL-dependent oncogenic transformation identified by deep mutational scanning. Blood. Am. Soc. Hematol. 135, 287–292. doi:10.1182/blood.2019002561
Campa, C. C., Weisbach, N. R., Santinha, A. J., Incarnato, D., and Platt, R. J. (2019). Multiplexed genome engineering by Cas12a and CRISPR arrays encoded on single transcripts. Nat. Methods 16, 887–893. doi:10.1038/s41592-019-0508-6
Cantor, A. J., Shah, N. H., and Kuriyan, J. (2018). Deep mutational analysis reveals functional trade-offs in the sequences of EGFR autophosphorylation sites. Proc. Natl. Acad. Sci. 115, E7303–E7312. doi:10.1073/pnas.1803598115
Cao, L., Coventry, B., Goreshnik, I., Huang, B., Sheffler, W., Park, J. S., et al. (2022). Design of protein-binding proteins from the target structure alone. Nature 605, 551–560. doi:10.1038/s41586-022-04654-9
Capriotti, E., Fariselli, P., and CasadioI-Mutant2, R. (2005). I-Mutant2.0: Predicting stability changes upon mutation from the protein sequence or structure. Nucleic Acids Res. 33, W306–W310. doi:10.1093/nar/gki375
Chen, J. Z., Fowler, D. M., and Tokuriki, N. (2022). Environmental selection and epistasis in an empirical phenotype–environment–fitness landscape. Nat. Ecol. Evol. 6, 427–438. doi:10.1038/s41559-022-01675-5
Choudhury, A., Fenster, J. A., Fankhauser, R. G., Kaar, J. L., Tenaillon, O., and Gill, R. T. (2020). CRISPR/Cas9 recombineering-mediated deep mutational scanning of essential genes in Escherichia coli. Mol. Syst. Biol. 16, e9265. doi:10.15252/msb.20199265
Cui, L., and Bikard, D. (2016). Consequences of Cas9 cleavage in the chromosome of Escherichia coli. Nucleic Acids Res. 44, 4243–4251. doi:10.1093/nar/gkw223
Deng, Z., Zhang, J., Li, J., and Zhang, X. (2021). Application of deep learning in plant–microbiota association analysis. Front. Genet. 12, 697090. doi:10.3389/fgene.2021.697090
Diss, G., and Lehner, B. (2018). The genetic landscape of a physical interaction. Elife 7, e32472. doi:10.7554/eLife.32472
Domingo, J., Baeza-Centurion, P., and Lehner, B. (2019). The causes and consequences of genetic interactions (epistasis). Annu. Rev. Genomics Hum. Genet. 20, 433–460. doi:10.1146/annurev-genom-083118-014857
Domingo, J., Diss, G., and Lehner, B. (2018). Pairwise and higher-order genetic interactions during the evolution of a tRNA. Nature 558, 117–121. doi:10.1038/s41586-018-0170-7
Doudna, J. A., and Charpentier, E. (2014). Genome editing. The new frontier of genome engineering with CRISPR-Cas9. Science 346, 1258096. doi:10.1126/science.1258096
Esposito, D., Weile, J., Shendure, J., Starita, L. M., Papenfuss, A. T., Roth, F. P., et al. (2019). MaveDB: An open-source platform to distribute and interpret data from multiplexed assays of variant effect. Genome Biol. 20, 223. doi:10.1186/s13059-019-1845-6
Faure, A. J., Domingo, J., Schmiedel, J. M., Hidalgo-Carcedo, C., Diss, G., and Lehner, B. (2022). Mapping the energetic and allosteric landscapes of protein binding domains. Nature 604, 175–183. doi:10.1038/s41586-022-04586-4
Faure, A. J., Schmiedel, J. M., Baeza-Centurion, P., and Lehner, B. (2020). DiMSum: An error model and pipeline for analyzing deep mutational scanning data and diagnosing common experimental pathologies. Genome Biol. 21, 207–223. doi:10.1186/s13059-020-02091-3
Findlay, G. M., Boyle, E. A., Hause, R. J., Klein, J. C., and Shendure, J. (2014). Saturation editing of genomic regions by multiplex homology-directed repair. Nature 513, 120–123. doi:10.1038/nature13695
Findlay, G. M., Daza, R. M., Martin, B., Zhang, M. D., Leith, A. P., Gasperini, M., et al. (2018). Accurate classification of BRCA1 variants with saturation genome editing. Nature 562, 217–222. doi:10.1038/s41586-018-0461-z
Firnberg, E., and Ostermeier, M. (2012). PFunkel: Efficient, expansive, user-defined mutagenesis. PLoS One 7, e52031. doi:10.1371/journal.pone.0052031
Fowler, D. M., Araya, C. L., Fleishman, S. J., Kellogg, E. H., Stephany, J. J., Baker, D., et al. (2010). High-resolution mapping of protein sequence-function relationships. Nat. Methods 7, 741–746. doi:10.1038/nmeth.1492
Fowler, D. M., Araya, C. L., Gerard, W., and Fields, S. (2011). Enrich: Software for analysis of protein function by enrichment and depletion of variants. Bioinformatics 27, 3430–3431. doi:10.1093/bioinformatics/btr577
Fowler, D. M., and Fields, S. (2014). Deep mutational scanning: A new style of protein science. Nat. Methods. 11, 801–807. doi:10.1038/nmeth.3027
Frazer, J., Notin, P., Dias, M., Gomez, A., Min, J. K., Brock, K., et al. (2021). Disease variant prediction with deep generative models of evolutionary data. Nature 599, 91–95. doi:10.1038/s41586-021-04043-8
Gelman, S., Fahlberg, S. A., Heinzelman, P., Romero, P. A., and Gitter, A. (2021). Neural networks to learn protein sequence–function relationships from deep mutational scanning data. Proc. Natl. Acad. Sci. 118, e2104878118. doi:10.1073/pnas.2104878118
Giver, L., Gershenson, A., Freskgard, P. O., and Arnold, F. H. (1998). Directed evolution of a thermostable esterase. Proc. Natl. Acad. Sci. U. S. A. 95, 12809–12813. doi:10.1073/pnas.95.22.12809
Gray, V. E., Hause, R. J., Luebeck, J., Shendure, J., and Fowler, D. M. (2018). Quantitative missense variant effect prediction using large-scale mutagenesis data. Cell Syst. 6, 116–124. doi:10.1016/j.cels.2017.11.003
Greaney, A. J., Starr, T. N., Barnes, C. O., Weisblum, Y., Schmidt, F., Caskey, M., et al. (2021). Mapping mutations to the SARS-CoV-2 RBD that escape binding by different classes of antibodies. Nat. Commun. 12, 4196. doi:10.1038/s41467-021-24435-8
Greaney, A. J., Starr, T. N., Gilchuk, P., Zost, S. J., Binshtein, E., Loes, A. N., et al. (2021). Complete mapping of mutations to the SARS-CoV-2 spike receptor-binding domain that escape antibody recognition. Cell Host Microbe 29, 44–57.e9. doi:10.1016/j.chom.2020.11.007
Hanna, R. E., Hegde, M., Fagre, C. R., DeWeirdt, P. C., Sangree, A. K., Szegletes, Z., et al. (2021). Massively parallel assessment of human variants with base editor screens. Cell 184, 1064–1080.e20. doi:10.1016/j.cell.2021.01.012
Hanning, K. R., Minot, M., Warrender, A. K., Kelton, W., and Reddy, S. T. (2022). Deep mutational scanning for therapeutic antibody engineering. Trends Pharmacol. Sci. 43, 123–135. doi:10.1016/j.tips.2021.11.010
Hart, T., Chandrashekhar, M., Aregger, M., Steinhart, Z., Brown, K. R., MacLeod, G., et al. (2015). High-Resolution CRISPR screens reveal fitness genes and genotype-specific cancer liabilities. Cell 163, 1515–1526. doi:10.1016/j.cell.2015.11.015
Hartman, E. C., Jakobson, C. M., Favor, A. H., Lobba, M. J., Álvarez-Benedicto, E., Francis, M. B., et al. (2018). Quantitative characterization of all single amino acid variants of a viral capsid-based drug delivery vehicle. Nat. Commun. 9, 1385–1395. doi:10.1038/s41467-018-03783-y
Hietpas, R., Roscoe, B., Jiang, L., and Bolon, D. N. A. (2012). Fitness analyses of all possible point mutations for regions of genes in yeast. Nat. Protoc. 7, 1382–1396. doi:10.1038/nprot.2012.069
Hilton, S. K., Huddleston, J., Black, A., North, K., Dingens, A. S., Bedford, T., et al. (2020). dms-view: Interactive visualization tool for deep mutational scanning data. J. open source Softw. 5, 2353. doi:10.21105/joss.02353
Hsu, C., Nisonoff, H., Fannjiang, C., and Listgarten, J. (2022). Learning protein fitness models from evolutionary and assay-labeled data. Nat. Biotechnol. 40, 1114–1122. doi:10.1038/s41587-021-01146-5
Javanmardi, K., Segall-Shapiro, T. H., Chou, C-W., Boutz, D. R., Olsen, R. J., Xie, X., et al. (2022). Antibody escape and cryptic cross-domain stabilization in the SARS-CoV-2 Omicron spike protein. Cell Host Microbe 30 (9), 1242–1254.e6. doi:10.1016/j.chom.2022.07.016
Karst, S. M., Ziels, R. M., Kirkegaard, R. H., Sørensen, E. A., McDonald, D., Zhu, Q., et al. (2021). High-accuracy long-read amplicon sequences using unique molecular identifiers with Nanopore or PacBio sequencing. Nat. Methods 18, 165–169. doi:10.1038/s41592-020-01041-y
Kemble, H., Nghe, P., and Tenaillon, O. (2019). Recent insights into the genotype–phenotype relationship from massively parallel genetic assays. Evol. Appl. 12, 1721–1742. doi:10.1111/eva.12846
Kinney, J. B., and McCandlish, D. M. (2019). Massively parallel assays and quantitative sequence-function relationships. Annu. Rev. Genomics Hum. Genet. Aug 31, 99–127. doi:10.1146/annurev-genom-083118-014845
Klesmith, J. R., Bacik, J. P., Wrenbeck, E. E., Michalczyk, R., and Whitehead, T. A. (2017). Trade-offs between enzyme fitness and solubility illuminated by deep mutational scanning. Proc. Natl. Acad. Sci. U. S. A. 114, 2265–2270. doi:10.1073/pnas.1614437114
Lappalainen, T., and MacArthur, D. G. (2021). From variant to function in human disease genetics. Sci. Am. Assoc. Adv. Sci. 373, 1464–1468. doi:10.1126/science.abi8207
Leander, M., Liu, Z., Cui, Q., and Raman, S. (2022). Deep mutational scanning and machine learning reveal structural and molecular rules governing allosteric hotspots in homologous proteins. Elife 11, e79932. doi:10.7554/eLife.79932
Leander, M., Yuan, Y., Meger, A., Cui, Q., and Raman, S. (2020). Functional plasticity and evolutionary adaptation of allosteric regulation. Proc. Natl. Acad. Sci. U. S. A. 117, 25445–25454. doi:10.1073/pnas.2002613117
Li, C., Qian, W., Maclean, C. J., and Zhang, J. (2016). The fitness landscape of a tRNA gene. Science 352, 837–840. doi:10.1126/science.aae0568
Li, X., Lalić, J., Baeza-Centurion, P., Dhar, R., and Lehner, B. (2019). Changes in gene expression predictably shift and switch genetic interactions. Nat. Commun. 10, 3886. doi:10.1038/s41467-019-11735-3
Li, X., and Lehner, B. (2020). Biophysical ambiguities prevent accurate genetic prediction. Nat. Commun. 11, 4923. doi:10.1038/s41467-020-18694-0
Lin-Goerke, J. L., Robbins, D. J., and Burczak, J. D. (1997). PCR-based random mutagenesis using manganese and reduced dNTP concentration. Biotechniques 23, 409–412. doi:10.2144/97233bm12
Lite, T-L. V., Grant, R. A., Nocedal, I., Littlehale, M. L., Guo, M. S., and Laub, M. T. (2020). Uncovering the basis of protein-protein interaction specificity with a combinatorially complete library. Elife 9, e60924. doi:10.7554/eLife.60924
Livesey, B. J., and Marsh, J. A. (2020). Using deep mutational scanning to benchmark variant effect predictors and identify disease mutations. Mol. Syst. Biol. 16, e9380. doi:10.15252/msb.20199380
Majithia, A. R., Tsuda, B., Agostini, M., Gnanapradeepan, K., Rice, R., Peloso, G., et al. (2016). Prospective functional classification of all possible missense variants in PPARG. Nat. Genet. 48, 1570–1575. doi:10.1038/ng.3700
Markin, C. J., Mokhtari, D. A., Sunden, F., Appel, M. J., Akiva, E., Longwell, S. A., et al. (2021). Revealing enzyme functional architecture via high-throughput microfluidic enzyme kinetics. Science 373, eabf8761. doi:10.1126/science.abf8761
Matreyek, K. A., Starita, L. M., Stephany, J. J., Martin, B., Chiasson, M. A., Gray, V. E., et al. (2018). Multiplex assessment of protein variant abundance by massively parallel sequencing. Nat. Genet. 50, 874–882. doi:10.1038/s41588-018-0122-z
Matreyek, K. A., Stephany, J. J., Chiasson, M. A., Hasle, N., and Fowler, D. M. (2020). An improved platform for functional assessment of large protein libraries in mammalian cells. Nucleic Acids Res. 48, e1. doi:10.1093/nar/gkz910
Matteucci, M. D., and Heyneker, H. L. (1983). Targeted random mutagenesis: The use of ambiguously synthesized oligonucleotides to mutagenize sequences immediately 5‘ of an ATG initiation codon. Nucleic Acids Res. 11, 3113–3121. doi:10.1093/nar/11.10.3113
Matuszewski, S., Hildebrandt, M. E., Ghenu, A-H., Jensen, J. D., and Bank, C. (2016). A statistical guide to the design of deep mutational scanning experiments. Genetics 204, 77–87. doi:10.1534/genetics.116.190462
McLaughlin, R. N., Poelwijk, F. J., Raman, A., Gosal, W. S., and Ranganathan, R. (2012). The spatial architecture of protein function and adaptation. Nature 491, 138–142. doi:10.1038/nature11500
Mendel, G. (1865). Versuche über Plflanzen-hybriden. Verhandlungen des naturforschenden Vereines 4, 3–47.
Mendel, G. (1941). Versuche über Pflanzen-Hybriden. Zauchter Z. fur Theor Angew Genet 13, 221–268. doi:10.1007/bf01804628
Mighell, T. L., Evans-Dutson, S., and O’Roak, B. J. (2018). A saturation mutagenesis approach to understanding PTEN lipid phosphatase activity and genotype-phenotype relationships. Am. J. Hum. Genet. 102, 943–955. doi:10.1016/j.ajhg.2018.03.018
Mighell, T. L., Thacker, S., Fombonne, E., Eng, C., and O’Roak, B. J. (2020). An integrated deep-mutational-scanning approach provides clinical insights on PTEN genotype-phenotype relationships. Am. J. Hum. Genet. 106, 818–829. doi:10.1016/j.ajhg.2020.04.014
Moore, G. L., Maranas, C. D., Gl, M., and Cd, M. (2000). Modeling DNA mutation and recombination for directed evolution experiments. J. Theor. Biol. 205 (3), 483–503. doi:10.1006/jtbi.2000.2082
Narayanan, K. K., and Procko, E. (2021). Deep mutational scanning of viral glycoproteins and their host receptors. Front. Mol. Biosci. 8, 636660. doi:10.3389/fmolb.2021.636660
Olson, C. A., Wu, N. C., and Sun, R. (2014). A comprehensive biophysical description of pairwise epistasis throughout an entire protein domain. Curr. Biol. 24, 2643–2651. doi:10.1016/j.cub.2014.09.072
Otwinowski, J., McCandlish, D. M., and Plotkin, J. B. (2018). Inferring the shape of global epistasis. Proc. Natl. Acad. Sci. U. S. A. 115, E7550–E7558. doi:10.1073/pnas.1804015115
Park, Y., Metzger, B. P. H. H., and Thornton, J. W. (2022). Epistatic drift causes gradual decay of predictability in protein evolution. Science 376, 823–830. doi:10.1126/science.abn6895
Peterman, N., and Levine, E. (2016). Sort-seq under the hood: Implications of design choices on large-scale characterization of sequence-function relations. BMC Genomics 17, 206–217. doi:10.1186/s12864-016-2533-5
Pfeiffer, F., Gröber, C., Blank, M., Händler, K., Beyer, M., Schultze, J. L., et al. (2018). Systematic evaluation of error rates and causes in short samples in next-generation sequencing. Sci. Rep. 8, 10950. doi:10.1038/s41598-018-29325-6
Plesa, C., Sidore, A. M., Lubock, N. B., Zhang, D., and Kosuri, S. (2018). Multiplexed gene synthesis in emulsions for exploring protein functional landscapes. Sci. Am. Assoc. Adv. Sci. 359, 343–347. doi:10.1126/science.aao5167
Puchta, O., Cseke, B., Czaja, H., Tollervey, D., Sanguinetti, G., and Kudla, G. (2016). Network of epistatic interactions within a yeast snoRNA. Science 352, 840–844. doi:10.1126/science.aaf0965
Rees, H. A., and Liu, D. R. (2018). Base editing: Precision chemistry on the genome and transcriptome of living cells. Nat. Rev. Genet. 19, 770–788. doi:10.1038/s41576-018-0059-1
Riesselman, A. J., Ingraham, J. B., and Marks, D. S. (2018). Deep generative models of genetic variation capture the effects of mutations. Nat. Methods 15, 816–822. doi:10.1038/s41592-018-0138-4
Rocklin, G. J., Chidyausiku, T. M., Goreshnik, I., Ford, A., Houliston, S., Lemak, A., et al. (2017). Global analysis of protein folding using massively parallel design, synthesis, and testing. Science 357, 168–175. doi:10.1126/science.aan0693
Rollins, N. J., Brock, K. P., Poelwijk, F. J., Stiffler, M. A., Gauthier, N. P., Sander, C., et al. (2019). Inferring protein 3D structure from deep mutation scans. Nat. Genet. 51, 1170–1176. doi:10.1038/s41588-019-0432-9
Rubin, A. F., Gelman, H., Lucas, N., Bajjalieh, S. M., Papenfuss, A. T., Speed, T. P., et al. (2017). A statistical framework for analyzing deep mutational scanning data. Genome Biol. 18, 150–164. doi:10.1186/s13059-017-1272-5
Sadhu, M. J., Bloom, J. S., Day, L., Siegel, J. J., Kosuri, S., and Kruglyak, L. (2018). Highly parallel genome variant engineering with CRISPR-Cas9. Nat. Genet. 50, 510–514. doi:10.1038/s41588-018-0087-y
Sarkisyan, K. S., Bolotin, D. A., Meer, M. V., Usmanova, D. R., Mishin, A. S., Sharonov, G. V., et al. (2016). Local fitness landscape of the green fluorescent protein. Nature 533, 397–401. doi:10.1038/nature17995
Schmiedel, J. M., and Lehner, B. (2019). Determining protein structures using deep mutagenesis. Nat. Genet. 51, 1177–1186. doi:10.1038/s41588-019-0431-x
Schymkowitz, J., Borg, J., Stricher, F., Nys, R., Rousseau, F., and Serrano, L. (2005). The FoldX web server: An online force field. Nucleic Acids Res. 33, W382–W388. doi:10.1093/nar/gki387
Seuma, M., Faure, A., Badia, M., Lehner, B., and Bolognesi, B. (2021). The genetic landscape for amyloid beta fibril nucleation accurately discriminates familial Alzheimer’s disease mutations. Elife 10, e63364–e63382. doi:10.7554/eLife.63364
Shafikhani, S., Siegel, R. A., Ferrari, E., and Schellenberger, V. (1997). Generation of large libraries of random mutants in Bacillus subtilis by PCR-based plasmid multimerization. Biotechniques 23, 304–310. doi:10.2144/97232rr01
Sharon, E., Chen, S. A. A., Khosla, N. M., Smith, J. D., Pritchard, J. K., and Fraser, H. B. (2018). Functional genetic variants revealed by massively parallel precise genome editing. Cell 175, 544–557. doi:10.1016/j.cell.2018.08.057
Shen, X., Song, S., Li, C., and Zhang, J. (2022). Synonymous mutations in representative yeast genes are mostly strongly non-neutral. Nature 606, 725–731. doi:10.1038/s41586-022-04823-w
Shendure, J., Balasubramanian, S., Church, G. M., Gilbert, W., Rogers, J., Schloss, J. A., et al. (2017). DNA sequencing at 40: Past, present and future. Nature 550, 345–353. doi:10.1038/nature24286
Shin, H., and Cho, B-K. (2015). Rational protein engineering guided by deep mutational scanning. Int. J. Mol. Sci. 16, 23094–23110. doi:10.3390/ijms160923094
Song, H., Bremer, B. J., Hinds, E. C., Raskutti, G., and Romero, P. A. (2021). Inferring protein sequence-function relationships with large-scale positive-unlabeled learning. Cell Syst. 12, 92–101.e8. doi:10.1016/j.cels.2020.10.007
Soskine, M., and Tawfik, D. S. (2010). Mutational effects and the evolution of new protein functions. Nat. Rev. Genet. 11, 572–582. doi:10.1038/nrg2808
Staller, M. V., Holehouse, A. S., Swain-Lenz, D., Das, R. K., Pappu, R. V., and Cohen, B. A. (2018). A high-throughput mutational scan of an intrinsically disordered acidic transcriptional activation domain. Cell Syst. 6, 444–455. doi:10.1016/j.cels.2018.01.015
Starita, L. M., and Fields, S. (2015). Deep mutational scanning: Library construction, functional selection, and high-throughput sequencing. Cold Spring Harb. Protoc. 2015, 777–780. doi:10.1101/pdb.prot085225
Starita, L. M., Young, D. L., Islam, M., Kitzman, J. O., Gullingsrud, J., Hause, R. J., et al. (2015). Massively parallel functional analysis of BRCA1 RING domain variants. Genetics 200, 413–422. doi:10.1534/genetics.115.175802
Starr, T. N., Greaney, A. J., Hannon, W. W., Loes, A. N., Hauser, K., Dillen, J. R., et al. (2022). Shifting mutational constraints in the SARS-CoV-2 receptor-binding domain during viral evolution. Science 377, 420–424. doi:10.1126/science.abo7896
Starr, T. N., Greaney, A. J., Hilton, S. K., Ellis, D., Crawford, K. H. D., Dingens, A. S., et al. (2020). Deep mutational scanning of SARS-CoV-2 receptor binding domain reveals constraints on folding and ACE2 binding. Cell 182, 1295–1310. doi:10.1016/j.cell.2020.08.012
Starr, T. N., Picton, L. K., and Thornton, J. W. (2017). Alternative evolutionary histories in the sequence space of an ancient protein. Nature 549, 409–413. doi:10.1038/nature23902
Stein, A., Fowler, D. M., Hartmann-Petersen, R., and Lindorff-Larsen, K. (2019). Biophysical and mechanistic models for disease-causing protein variants. Trends Biochem. Sci. 44, 575–588. doi:10.1016/j.tibs.2019.01.003
Stiffler, M. A., Hekstra, D. R., and Ranganathan, R. (2015). Evolvability as a function of purifying selection in TEM-1 β-lactamase. Cell 160, 882–892. doi:10.1016/j.cell.2015.01.035
Tack, D. S., Tonner, P. D., Pressman, A., Olson, N. D., Levy, S. F., Romantseva, E. F., et al. (2021). The genotype-phenotype landscape of an allosteric protein. Mol. Syst. Biol. 17, e10847. doi:10.15252/msb.202110847
Tarassov, K., Messier, V., Landry, C. R., Radinovic, S., Molina, M. M. S., Shames, I., et al. (2008). An in vivo map of the yeast protein interactome. Science 320, 1465–1470. doi:10.1126/science.1153878
Tareen, A., Kooshkbaghi, M., Posfai, A., Ireland, W. T., McCandlish, D. M., and Kinney, J. B. (2022). MAVE-NN: Learning genotype-phenotype maps from multiplex assays of variant effect. Genome Biol. 23, 98–27. doi:10.1186/s13059-022-02661-7
Vaishnav, E. D., de Boer, C. G., Molinet, J., Yassour, M., Fan, L., Adiconis, X., et al. (2022). The evolution, evolvability and engineering of gene regulatory DNA. Nature 603, 455–463. doi:10.1038/s41586-022-04506-6
Vanhercke, T., Ampe, C., Tirry, L., and Denolf, P. (2005). Reducing mutational bias in random protein libraries. Anal. Biochem. 339, 9–14. doi:10.1016/j.ab.2004.11.032
Voichek, Y., and Weigel, D. (2020). Identifying genetic variants underlying phenotypic variation in plants without complete genomes. Nat. Genet. 52, 534–540. doi:10.1038/s41588-020-0612-7
Wan, L., Twitchett, M. B., Eltis, L. D., Mauk, A. G., and Smith, M. (1998). In vitro evolution of horse heart myoglobin to increase peroxidase activity. Proc. Natl. Acad. Sci. U. S. A. 95, 12825–12831. doi:10.1073/pnas.95.22.12825
Wang, T., Wei, J. J., Sabatini, D. M., and Lander, E. S. (2014). Genetic screens in human cells using the CRISPR-Cas9 system. Science 343, 80–84. doi:10.1126/science.1246981
Weile, J., and Roth, F. P. (2018). Multiplexed assays of variant effects contribute to a growing genotype–phenotype atlas. Hum. Genet. 137, 665–678. doi:10.1007/s00439-018-1916-x
Weile, J., Sun, S., Cote, A. G., Knapp, J., Verby, M., Mellor, J. C., et al. (2017). A framework for exhaustively mapping functional missense variants. Mol. Syst. Biol. 13, 957. doi:10.15252/msb.20177908
Weng, C., Faure, A., and Lehner, B. (2022). The energetic and allosteric landscape for KRAS inhibition. bioRxiv 12, 840–844. doi:10.1101/2022.12.06.519122
Wenger, A. M., Peluso, P., Rowell, W. J., Chang, P-C., Hall, R. J., Concepcion, G. T., et al. (2019). Accurate circular consensus long-read sequencing improves variant detection and assembly of a human genome. Nat. Biotechnol. 37, 1155–1162. doi:10.1038/s41587-019-0217-9
Wrenbeck, E. E., Klesmith, J. R., Stapleton, J. A., Adeniran, A., Tyo, K. E. J., and Whitehead, T. A. (2016). Plasmid-based one-pot saturation mutagenesis. Nat. Methods 13, 928–930. doi:10.1038/nmeth.4029
Wu, Z., Cai, X., Zhang, X., Liu, Y., Tian, G., Yang, J-R., et al. (2022). Expression level is a major modifier of the fitness landscape of a protein coding gene. Nat. Ecol. Evol. 6, 103–115. doi:10.1038/s41559-021-01578-x
Yoo, J. I., Daugherty, P. S., and O’Malley, M. A. (2020). Bridging non-overlapping reads illuminates high-order epistasis between distal protein sites in a GPCR. Nat. Commun. 11, 690. doi:10.1038/s41467-020-14495-7
Zerbini, F., Zanella, I., Fraccascia, D., König, E., Irene, C., Frattini, L. F., et al. (2017). Large scale validation of an efficient CRISPR/Cas-based multi gene editing protocol in Escherichia coli. Microb. Cell Fact. 16, 68. doi:10.1186/s12934-017-0681-1
Zhang, N., Chen, Y., Lu, H., Zhao, F., Alvarez, R. V., Goncearenco, A., et al. (2020). MutaBind2: Predicting the impacts of single and multiple mutations on protein-protein interactions. iScience 23, 100939. doi:10.1016/j.isci.2020.100939
Keywords: deep mutational scanning, genotype-phenotype mapping, massively parallel mutagenesis, high-throughput analysis, systems biology, biotechnology
Citation: Wei H and Li X (2023) Deep mutational scanning: A versatile tool in systematically mapping genotypes to phenotypes. Front. Genet. 14:1087267. doi: 10.3389/fgene.2023.1087267
Received: 02 November 2022; Accepted: 02 January 2023;
Published: 12 January 2023.
Edited by:
Honey V. Reddi, Medical College of Wisconsin, United StatesReviewed by:
Xiujun Zhang, Wuhan Botanical Garden (CAS), ChinaQian Nie, Medical College of Wisconsin, United States
Copyright © 2023 Wei and Li. This is an open-access article distributed under the terms of the Creative Commons Attribution License (CC BY). The use, distribution or reproduction in other forums is permitted, provided the original author(s) and the copyright owner(s) are credited and that the original publication in this journal is cited, in accordance with accepted academic practice. No use, distribution or reproduction is permitted which does not comply with these terms.
*Correspondence: Xianghua Li, v1xli226@exseed.ed.ac.uk