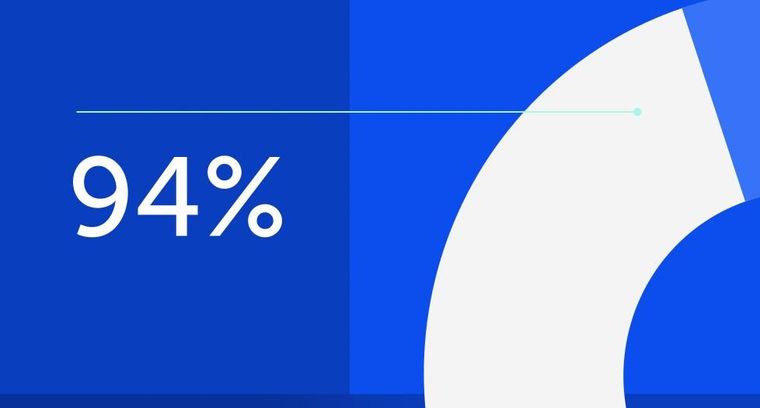
94% of researchers rate our articles as excellent or good
Learn more about the work of our research integrity team to safeguard the quality of each article we publish.
Find out more
ORIGINAL RESEARCH article
Front. Genet., 17 March 2023
Sec. Livestock Genomics
Volume 14 - 2023 | https://doi.org/10.3389/fgene.2023.1083106
This article is part of the Research TopicApplication of Genomics in Livestock Populations under Selection or ConservationView all 20 articles
Genomic selection (GS) plays an essential role in livestock genetic improvement programs. In dairy cattle, the method is already a recognized tool to estimate the breeding values of young animals and reduce generation intervals. Due to the different breeding structures of beef cattle, the implementation of GS is still a challenge and has been adopted to a much lesser extent than dairy cattle. This study aimed to evaluate genotyping strategies in terms of prediction accuracy as the first step in the implementation of GS in beef while some restrictions were assumed for the availability of phenotypic and genomic information. For this purpose, a multi-breed population of beef cattle was simulated by imitating the practical system of beef cattle genetic evaluation. Four genotyping scenarios were compared to traditional pedigree-based evaluation. Results showed an improvement in prediction accuracy, albeit a limited number of animals being genotyped (i.e., 3% of total animals in genetic evaluation). The comparison of genotyping scenarios revealed that selective genotyping should be on animals from both ancestral and younger generations. In addition, as genetic evaluation in practice covers traits that are expressed in either sex, it is recommended that genotyping covers animals from both sexes.
In livestock breeding programs, genomic selection (GS) is a method that uses genomic information to estimate breeding values and rank selection candidates. GS has shaped modern breeding programs and contributed substantially to the increase of genetic progress for various economically important traits, especially in dairy cattle (VanRaden et al., 2009; Meuwissen et al., 2016). The advantages of GS over traditional selection include; shorter generation intervals, increased selection intensity, greater selection accuracies, not limited to sex, and can be generalized to any trait that is recorded in the reference population (Schaeffer, 2006; Aguilar et al., 2010).
Genomic selection has a high potential for improving the genetic gain in beef cattle because reproduction, health, growth rate, meat quality, and feed efficiency are vital traits that contribute to the profitability of this industry, which are difficult and expensive to measure routinely (Van Eenennaam et al., 2011; Montaldo et al., 2012; Hayes et al., 2013). However, the accuracies of genomic breeding values for economic traits in beef cattle are low to moderate (Saatchi et al., 2011; Van Eenennaam et al., 2014). This is for two possible reasons: i) the reference populations that have been assembled for beef cattle are generally smaller than those for dairy cattle, and there are fewer sires with highly accurate progeny tests in comparison with dairy cattle; and ii) unlike dairy cattle, where populations around the world are dominated by just a couple of breeds, there are numerous breeds of importance and even two subspecies (Bos taurus and B. indicus) in the beef industry (Hayes et al., 2013).
Genomic selection in beef cattle was first performed based on pseudo-data with multiple-step methods, such as estimated breeding value (EBV) or daughter yield deviation (VanRaden et al., 2009). This method needs many animals (hundreds of thousands) to be genotyped and have phenotypic measurements for the trait of interest to serve as the reference population. The reference population also needs to be updated, i.e., new animals with both phenotype and genotype need to be added. Although the multiple-step method is practical, it rests on several assumptions that are not met in all situations; for instance, it is impossible to genotype all animals. Also, the predicted accuracy using the multistep procedure is lower when compared to single-step BLUP. Also, the large number of breeds and crossbreds, poor extent of phenotyping, limited use of artificial insemination, less advanced structures and breeding programs, low number of offspring per female, incomplete relationships between identical traits in different countries, and limited data recording on economically important traits have resulted in limited adoption of GS in beef cattle (Goddard, 2009; Johnston et al., 2012; Van Eenennaam et al., 2014). Despite these difficulties, results of applying GS have been reported in some studies (Hayes et al., 2019; Wang et al., 2019; Zhu et al., 2019). All studies reported the benefits of applying GS in beef cattle and showed that GS could be a practical alternative to traditional selection approaches. Due to the mentioned limitations, the single-step genomic best linear unbiased prediction (ssGBLUP) method that combines all types of information (phenotype records, pedigree, genotypes) seems to work best in practical genetic evaluation in beef cattle. The main benefit of this method is that all animals in evaluation can get genomic-enhanced breeding values, even if not all have been genotyped (Misztal et al., 2009; Legarra et al., 2014).
Berry et al., 2016 reviewed prediction accuracy with the use of genomic data for some traits in beef cattle. However, guidelines to implement the method in practice are lacking. The main challenge in the implementation of GS for a breeding organization would be which and how many animals to be genotyped as the initial step. Even though, the cost of genotyping is not an obstacle nowadays, but contrary to dairy, the beef industry is much smaller, and genetic evaluation is performed on a much smaller scale in most countries. This is because beef production is highly influenced by the dairy sector with calves and cattle not required for dairy products being fattened to produce meat (Deblitz, 2008). Smaller industries can easily translate to the fact that breed associations and companies have much fewer resources to spend on genotyping. In the ideal situation, there may be possibilities to spend some funding on genotyping of the semen sires that are in service. However, a large proportion of genotyping costs for younger animals would be paid by the farms which traditionally are slower adopters of technology than dairy farmers. This could be due to a multitude of reasons, including the lower business margin.
While genotyping of the ancestral sires in a sense that they have contributed much more than their contemporaries to the current generations seems logical, however, sustainable genetic gain in a breeding scheme needs accurate selection in younger generations as well. In addition, similar to dairy cattle, genetic progress in a breeding scheme in beef is not only driven by bulls but also depends on the superiority of the dams of the candidates. Based on this, we hypothesized that prediction accuracy in selection candidates might differ when genotyping is only on ancestors, younger generation, and is restricted for males or females. Thus, the main aim of this study was to evaluate genotyping strategies in terms of prediction accuracy as the first step in the implementation of GS in beef. In particular, the goal was to compare and to contrast the importance of genotyping the ancestors and distributing the genotyped individuals for the two sexes on the predicting accuracy. The study was conducted under the assumption that a number of animals being genotyped is the main constraint.
Using QMSim software, a historical population of beef cattle was simulated based on the forward-in-time process (Sargolzaei and Schenkel, 2009). In total, 2020 generations were considered for the historical population. For the first 1,000 generations, the population size (
In the second step, to enlarge the base population, 100 Founder males and 100 Founder females were selected randomly from the last generation of the historical population (Expanded generations) and were mated randomly for another eight generations. In the third step (Breed formation), five random samples, as the base for five breeds (A-E), were randomly chosen from the last generation of the previous step. In this step, mating and selection were also random within each breed, producing two offspring per dam for 30 generations. In the last step (step 4), population structure was simulated to mimic the production and genetic evaluation system in practice such that parameters were chosen to be realistic five breeds with different sizes were simulated for 15 generations. Selection in all breeds was based on EBVs using pedigree-based BLUP, and the culling of animals was based on age. It was assumed that pedigree was available for all breeds without error, and base animals in this step were considered as meta founders (i.e., one meta-founder per breed). Sire and dam replacement ratio was different across breeds (Table 1).
Five scenarios were compared in terms of prediction accuracy of selection candidates in the last generation (15th generation). Scenarios included a reference scenario (Ref. Sc) without genotypic data and scenarios with both genotypic and phenotypic information (Sc. 1 to Sc. 4). In all scenarios, it was assumed that phenotypic and pedigree data were available for the last 15 generations, and animals could be genotyped from the 7th generation onward. In all genomic scenarios, 5,000 animals could be genotyped. Genotyping scenarios differed in the method applied for the selection of 5,000 animals to be genotyped. In Sc. 1, the genotyping strategy was focused on young animals and only male progenies from the pool of selection candidates of generation 15 were selected randomly. In Sc. 2, genotyping was only on males but both young animals and ancestral sires could be genotyped. The criteria for selection of ancestral sires were that a sire should have at least 10 progenies in the population to be selected for genotyping. In Sc. 3, both male and female progenies from generation 15 could be genotyped. In Sc. 4, randomly selected ancestral sires, ancestral dams, and selection candidates (both males and females) were selected to be genotyped. The number of male and female animals with genotypic record in each scenario across breeds is presented in Table 2.
TABLE 2. Number of male and female animals with genotyping record in each scenario according to breeds.
In all scenarios, irrespective of the availability of genotypic data, two other factors were considered to evaluate their impact on the prediction accuracy of the selection candidates. The first factor was the number of phenotypic records which could be collected and used for the genetic evaluation. It was assumed that 20, 60, and 100 percent of the animals could have phenotypic observations (cases 1–3: 100 MF, 60 MF, and 20 MF). This phenotyping scenario was considered to cover a range of traits such as birth weight, where data are collected routinely in practice, and a scarcely recorded trait such as meat quality, where normally 20% of animals in the evaluation would have records available in practice. The second factor was the simulation of a sex-limited trait where either males (e.g., scrotal circumference) (cases 3–6: 100 M, 60 M, and 20 M) or females (cases 6–9: 100 F, 60 F, and 20 F) could have phenotypic records. A schematic representation of the genotyping and phenotyping scenarios is in Figure 1.
A genome consisting of 29 pairs of chromosomes with a total length of 2,319 cM was simulated. For each animal, single nucleotide polymorphisms (SNPs) markers with the density of 50 K and n = 800 QTL were considered. Both SNPs and QTL were selected from the segregating loci of the last generation of the historical population with a Minor Allele Frequency (MAF) of greater than 0.1 and were randomly spaced across the genome. Recurrent mutation rate of
A single trait with a heritability of 0.3 and phenotypic variance of 1.0 was simulated. The True Breeding Values (TBV) for each animal were calculated as follows:
Where
For the reference scenario, a single-trait BLUP with UPG was used to estimate the breeding values. In this scenario, all five breeds were analyzed together in a multi-breed model. EBVs were estimated based on the model (2):
Where
Where
where
For genomic scenarios (Sc. 1–Sc. 4), the ssGBLUP with meta-founder was used. In the meta-founder approach, a modified
Where
Where
Matrix 𝚪 represents within and across population relationship matrix. The structure of variance-covariance of meta-founder was estimated as
The genetic evaluation analysis was performed under the restricted maximum likelihood (REML) approach using an animal model in the BLUPF90 family software (Misztal et al., 2015). The prediction accuracy in each scenario was computed as the correlation between TBV and (G)EBV in the 15th generation.
In all scenarios, the use of genomic information irrespective of phenotyping strategy, increased the prediction accuracy compared to the Ref. Sc (Table 3). The range of prediction accuracy in Ref. Sc scenario was between 0.14 and 0.34 and for GS scenarios between 0.19 and 0.50. The average prediction accuracy across scenarios were 0.23, 0.31, 0.38, 0.28 and 0.41 for Ref. Sc and Sc. 1 to 4, respectively. Among the GS scenarios, Sc. 4 had the highest accuracy across phenotyping strategies, followed by Sc. 2. In both Sc. 4 and Sc. 2, ancestral animals with contributions to the population (i.e., had some progenies) were genotyped in addition to selection candidates. For scenarios where genotyping was limited to the young selection candidates (Sc. 1 and Sc. 3), prediction accuracy was lower than the scenarios where genotyping was on animals from young and older generations.
Figure 2 shows the prediction accuracy in males and females. Nearly in all scenarios and cases, males were predicted more accurately than females. Even when only 20% of males had a phenotypic record, the accuracy is higher than when 20% of male and female animals or only 20% of female animals had a phenotypic record, which shows the more significant effect of male phenotypic records on the prediction accuracy.
As expected within each scenario, higher percentage of phenotype availability, resulted in higher prediction accuracy. The trend was similar for all scenarios and irrespective of the availability of records on both or either of sex. Results also show that when the trait of interest could be measured on both sex (Cases 100, 60, 20 MF), on average prediction accuracy was higher than when trait was sex-limited. For sex-limited traits, prediction accuracy was similar whether it was measured on males or females, however, mean accuracy were slightly higher when trait of interest was measured on male animals (0.34 in females and 0.36 in males).
Table 4 shows the prediction accuracy for animals without phenotypic records in different cases for selection candidates. The aim would be to realize how genotyping strategy would affect prediction accuracy in animals without records in the last generation. Prediction accuracy in males without records was highest based on Sc. 2 and lowest based on Sc. 3 with an average of 0.41 and 0.24, respectively. Prediction accuracy for females without records was highest in Sc. 4 and Sc. 3 with an average of 0.41 and 0.28, respectively. Note that prediction accuracy for some phenotyping cases is not presented as either all animals had phenotypic records (Case 100 MF) or trait was sex limited (Case 100 M and Case 100 F). (The solution for UPG was similar among breeds and Supplementary Table S1 shows mean solution for UPG each breed across cases.)
TABLE 4. Prediction accuracy for animals without phenotypic records in males and females according to cases.
We investigated the potential of applying GS in beef cattle when the aim was improving prediction accuracy in selection candidates. Four selective genotyping scenarios were compared to traditional pedigree-based evaluation. Results showed fair improvement in prediction accuracy, albeit a limited number of animals being genotyped.
One challenge with applying GS in practice is that there are many selection candidates, and genotyping all of them is often impractical. Genotype scenarios should only genotype small proportions of selected candidates welcomed by breeders because results have shown that significant investments in the genotype of selected candidates are not necessary to take full advantage of the benefits of genomic selection (Pryce and Daetwyler, 2012; Howard et al., 2018). As the results showed that the determination of the genotype of only the selected candidates, regardless of gender, has the lowest prediction accuracy (Sc. 3). Our study investigated the importance and effect of genotyping and phenotyping scenarios on prediction accuracy. The results showed that genotyping of both the selection candidates), and male and female ancestors, could be used to maximize the advantage of genomic selection (Sc. 4). In fact, Sc. 4 confirms the more significant effect of the female genotype than the male genotype and the more significant influence of the ancestral genotype than the selected candidates on the prediction accuracy (because they have more offspring, more information). The lower effect of male genotypes on the accuracy of predictions in this study can be allocated to the effect of the pedigree relationship between individuals. This makes it difficult to make accurate sire selection decisions (Nwogwugwu et al., 2020). In addition, in beef cattle, the offspring are smaller per male and more significant in each female than in dairy cattle. Determining the female genotype in beef cattle can significantly contribute to genetic accuracy and development. As a result, selective genotyping of only part of the selection candidates, males and females of the ancestors, can increase the prediction accuracy. In addition, we can make the most of the benefits of genome selection while saving on genotype costs.
Natural mating of multiple sires is the most common mating system in beef cattle production, despite the management advantages of this mating system, it does not allow for identification the paternity of the progeny (Tonussi et al., 2017). So, because the information of the cows is known, the genotype of females is easier Given that the genotypic data of females are usually more available than males (Mrode, 2019), accuracy can be increased by increasing the genotypic data of females (Tsuruta et al., 2013). Various studies have examined the effect of female genotype on prediction accuracy, including a study using a multi-step method that reported a decrease in accuracy using female genotype (Wiggans et al., 2011). However, in our study using the ssGBLUP approach, accuracy was increased by including the female genotype, and also consistent with the results reported by Tsuruta et al., 2013; Lourenco et al., 2014. In addition, in all genomic scenarios, by examining prediction accuracy in males and females separately, it can be concluded that increasing the genotypic information of females, the prediction accuracy increases (Figure 2).
In practical situation, all animals being evaluated rarely have phenotypic information, and the records are not widely available for traits such as disease and meat quality. To address this issue, we considered different phenotypic scenarios in our simulation. In all studied scenarios, with decreasing phenotypic records, prediction accuracy also decreases, which indicates a direct relationship between phenotypic records and prediction accuracy (Goddard, 2009; Takeda et al., 2020).
Genomic prediction in beef cattle provides accuracy higher than the average of parents based on the pedigree of selected candidates. It can be equivalent to progeny tests based on a maximum of 10 offspring (Garrick, 2011). In addition, ssGBLUP is superior to traditional evaluation methods because ssGBLUP uses phenotypes instead of pseudo-phenotypes and considers the entire population structure for GEBV estimation (Lourenco et al., 2014). This can be used for beef cattle selection in that only a tiny proportion of animals have pedigree and genotype. Estimated breeding value assessment with BLUP depends on the phenotype, parents, and progeny. But the ssGBLUP method is less sensitive to scenarios where animals selectively genotyped and, or genomic preselection exists compared to the multiple-step methods. Hence, ssGBLUP in conventional evaluations is attractive (Masuda et al., 2018). The ssGBLUP method is conceptually and practically simpler than the multiple-step GBLUP method, and in addition, it does not have shortcomings such as bias and loss of information of with few progenies, as well as operational complexity (Christensen and Lund, 2010; Legarra et al., 2014). Therefore, the ssGBLUP method is simpler and applicable to complex models and is generally as accurate as multiple-step methods (VanRaden, 2012; Mehrban et al., 2019). Also, Due to the lower sensitivity of the ssGBLUP method to genotyping scenarios, this method can be used to determine the best genotyping scenario to reduce genotype costs while increasing accuracy (Howard et al., 2018).
In our study, genetic evaluation was based on multiple breeds information. When several breeds are combined in one assessment, there is generally no pedigree information among breeds. As a result, UPG (Quaas, 1988) has been developed to model missing pedigrees and to explain breed differences in multi-breed evaluations(Legarra et al., 2007; VanRaden et al., 2007). However, UPG solutions, when evaluated with the ssGBLUP model, may be biased due to genomic incompatibility (
The pedigrees used in genetic evaluations may go back to a few base populations thought to be unrelated due to a lack of access to information (Legarra et al., 2015). In addition, information is not available at the beginning of the pedigree, and animals of several generations may have missing pedigree information (Tsuruta et al., 2019). Also, populations are selected, and animals with missing parents are unlikely to be chosen as parents of the next-generation because their breeding value is reduced to zero (Legarra et al., 2015; Kluska et al., 2021). Unknown parent groups and Meta-Founder can be used to calculate missing pedigree and breed structure in multi-breed populations such as beef cattle (Kluska et al., 2021).
We investigated the potential of applying GS in beef cattle when the aim was improving prediction accuracy in selection candidates. Four selective genotyping scenarios were compared to traditional pedigree-based evaluation. Results showed fair improvement in prediction accuracy, albeit a limited number of animals being genotyped. Comparison of GS scenarios revealed that selective genotyping should be on animals from both ancestral and younger generations. In addition, as genetic evaluation in practice cover traits that are expressed on either sex, it is recommended that selective genotyping covers animals from both sexes as well.
The original contributions presented in the study are included in the article/Supplementary Materials, further inquiries can be directed to the corresponding authors.
SR and HE conceived and designed this simulation. ME performed the simulation and data analysis. SR and HE participated in the simulation and data analysis. ME drafted the article. All authors participated in editing and approved the final version.
HE was employed by the company Norwegian Beef Cattle Organizations, TYR.
The remaining authors declare that the research was conducted in the absence of any commercial or financial relationships that could be construed as a potential conflict of interest.
All claims expressed in this article are solely those of the authors and do not necessarily represent those of their affiliated organizations, or those of the publisher, the editors and the reviewers. Any product that may be evaluated in this article, or claim that may be made by its manufacturer, is not guaranteed or endorsed by the publisher.
The Supplementary Material for this article can be found online at: https://www.frontiersin.org/articles/10.3389/fgene.2023.1083106/full#supplementary-material
Aguilar, I., Misztal, I., Johnson, D. L., Legarra, A., Tsuruta, S., Lawlor, T. J., et al. (2010). Hot topic: A unified approach to utilize phenotypic, full pedigree, and genomic information for genetic evaluation of holstein final score. J. Dairy Sci. 93 (2), 743–752. doi:10.3168/jds.2009-2730
Berry, D. P., Garcia, J. F., and Garrick, D. J. (2016). Development and implementation of genomic predictions in beef cattle. Anim. Front. 6 (1), 32–38. doi:10.2527/af.2016-0005
Bradford, H. L., Masuda, Y., VanRaden, P. M., LegArrA, A., and Misztal, I. (2019). Modeling missing pedigree in single-step genomic BLUP. J. Dairy Sci. 102 (3), 2336–2346. doi:10.3168/jds.2018-15434
Christensen, O. F., Legarra, A., Lund, M. S., and Su, G. (2015). Genetic evaluation for three-way crossbreeding. Genet. Sel. Evol. 47 (1), 98–13. doi:10.1186/s12711-015-0177-6
Christensen, O., and Lund, M. (2010). Genomic relationship matrix when some animals are not genotyped. Genet. Sel. Evol. 42 (2), 1–8.
Deblitz, C. (2008). Brömmer and D. Brüggemann/Landbauforschung-vTI agriculture and forestry research. Landbauforschung 1 (2), 29–44.
Garrick, D. J. (2011). The nature, scope and impact of genomic prediction in beef cattle in the United States. Genet. Sel. Evol. 43, 17. doi:10.1186/1297-9686-43-17
Goddard, M. (2009). Genomic selection: Prediction of accuracy and maximisation of long term response. Genetica 136, 245. doi:10.1007/s10709-008-9308-0
Hayes, B. J., Corbet, N. J., Allen, J. M., Laing, A. R., Fordyce, G., Lyons, R., et al. (2019). Towards multi-breed genomic evaluations for female fertility of tropical beef cattle. J. Animal Sci. 97 (1), 55–62. doi:10.1093/jas/sky417
Hayes, B. J., Lewin, H. A., and Goddard, M. E. (2013). The future of livestock breeding: Genomic selection for efficiency, reduced emissions intensity, and adaptation. Trends Genet. 29 (4), 206–214. doi:10.1016/j.tig.2012.11.009
Howard, J. T., Rathje, T. A., Bruns, C. E., Wilson-Wells, D. F., Kachman, S. D., and Spangler, M. L. (2018). The impact of selective genotyping on the response to selection using single-step genomic best linear unbiased prediction. J. Animal Sci. 96 (11), 4532–4542. doi:10.1093/jas/sky330
Johnston, D. J., Tier, B., and Graser, H. U. (2012). Beef cattle breeding in Australia with genomics: Opportunities and needs. Animal Prod. Sci. 52 (2–3), 100–106. doi:10.1071/AN11116
Junqueira, V. S., Lopes, P. S., Lourenco, D., Silva, F. F. E., and Cardoso, F. F. (2020). Applying the metafounders approach for genomic evaluation in a multibreed beef cattle population. Front. Genet. 11 (12), 556399. doi:10.3389/fgene.2020.556399
Kluska, S., Masuda, Y., Ferraz, J. B. S., Tsuruta, S., Eler, J. P., Baldi, F., et al. (2021). Metafounders may reduce bias in composite cattle genomic predictions. Front. Genet. 12 (8), 678587. doi:10.3389/fgene.2021.678587
Kudinov, A. A., Mantysaari, E. A., Aamand, G. P., Uimari, P., and Stranden, I. (2020). Metafounder approach for single-step genomic evaluations of Red Dairy cattle. J. Dairy Sci. 103 (7), 6299–6310. doi:10.3168/jds.2019-17483
Legarra, A., Christensen, O. F., Aguilar, I., and Misztal, I. (2014). Single Step, a general approach for genomic selection. Livest. Sci. 166 (1), 54–65. doi:10.1016/j.livsci.2014.04.029
Legarra, A., Christensen, O. F., Vitezica, Z. G., Aguilar, I., and Misztal, I. (2015). Ancestral relationships using metafounders: Finite ancestral populations and across population relationships. Genetics 200 (2), 455–468. doi:10.1534/genetics.115.177014
Legarra, A., Strabel, T., Sapp, R. L., Sanchez, J. P., and Misztal, I. (2007). Multi-breed genetic evaluation in a Gelbvieh population. J. Animal Breed. Genet. 124 (5), 286–295. doi:10.1111/j.1439-0388.2007.00671.x
Lourenco, D. A. L., Misztal, I., TSuruta, S., AguIlar, I., Ezra, E., Ron, M., et al. (2014). Methods for genomic evaluation of a relatively small genotyped dairy population and effect of genotyped cow information in multiparity analyses. J. Dairy Sci. 97 (3), 1742–1752. doi:10.3168/jds.2013-6916
Masuda, Y., VanRaden, P. M., Misztal, I., and Lawlor, T. J. (2018). Differing genetic trend estimates from traditional and genomic evaluations of genotyped animals as evidence of preselection bias in US Holsteins. J. Dairy Sci. 101 (6), 5194–5206. doi:10.3168/jds.2017-13310
Mehrban, H., Lee, D. H., Naserkheil, M., Moradi, M. H., and Ibáñez-Escriche, N. (2019). ‘Comparison of conventional BLUP and singlestep genomic BLUP evaluations for yearling weight and carcass traits in Hanwoo beef cattle using single trait and multi-trait models. PLoS ONE 14, e0223352. doi:10.1371/journal.pone.0223352
Meuwissen, T., Hayes, B., and Goddard, M. (2016). Genomic selection: A paradigm shift in animal breeding. Anim. Front. 6 (1), 6–14. doi:10.2527/af.2016-0002
Misztal, I., Legarra, A., and Aguilar, I. (2009). Computing procedures for genetic evaluation including phenotypic, full pedigree, and genomic information. J. Dairy Sci. 92 (9), 4648–4655. doi:10.3168/jds.2009-2064
Misztal, I., and Legarra, A. (2017). Invited review: Efficient computation strategies in genomic selection. Animal 11 (5), 731–736. doi:10.1017/S1751731116002366
Misztal, I., Tsuruta, S., Aguilar, I., Legarra, A., and Vitezica, Z. (2015). BLUPF90 family of programs. Athens, USA: University of Georgia, 1–125. Available at: http://nce.ads.uga.edu/wiki/lib/exe/fetch.php?media=blupf90_all2.pdf.
Montaldo, H. H., Casas, E., Ferraz, J. B. S., Vega-Murillo, V. E., and Roman-Ponce, S. I. (2012). Opportunities and challenges from the use of genomic selection for beef cattle breeding in Latin America. Anim. Front. 2 (1), 23–29. doi:10.2527/af.2011-0029
Mrode, R. (2019). Genomic selection and use of molecular tools in breeding programs for indigenous and crossbred cattle in developing countries: Current status and future prospects. Front. Genet. 9, 694. doi:10.3389/fgene.2018.00694
Nwogwugwu, C. P., Kim, Y., Choi, H., Lee, J. H., and Lee, S. H. (2020). Assessment of genomic prediction accuracy using different selection and evaluation approaches in a simulated Korean beef cattle population. Asian-Australasian J. Animal Sci. 33 (12), 1912–1921. doi:10.5713/ajas.20.0217
Pryce, J. E., and Daetwyler, H. D. (2012). Designing dairy cattle breeding schemes under genomic selection: A review of international research. Animal Prod. Sci. 52 (2–3), 107–114. doi:10.1071/AN11098
Quaas, R. L. (1988). Additive genetic model with groups and relationships. J. Dairy Sci. 71 (5), 1338–1345. doi:10.3168/jds.S0022-0302(88)79691-5
Saatchi, M., McClure, M. C., McKay, S. D., Rolf, M. M., Kim, J., Decker, J. E., et al. (2011). Accuracies of genomic breeding values in American Angus beef cattle using K-means clustering for cross-validation. Genet. Sel. Evol. 43 (1), 40–16. doi:10.1186/1297-9686-43-40
Sargolzaei, M., and Schenkel, F. S. (2009). QMSim: A large-scale genome simulator for livestock. Bioinformatics 25 (5), 680–681. doi:10.1093/bioinformatics/btp045
Schaeffer, L. R. (2006). Strategy for applying genome-wide selection in dairy cattle. J. Animal Breed. Genet. 123 (4), 218–223. doi:10.1111/j.1439-0388.2006.00595.x
Takeda, M., Uemoto, Y., and Satoh, M. (2020). Effect of genotyped bulls with different numbers of phenotyped progenies on quantitative trait loci detection and genomic evaluation in a simulated cattle population. Animal Sci. J. 91 (1), e13432–e13439. doi:10.1111/asj.13432
Tonussi, R. L., de Oliveira Silva, R. M., Magalhaes, A. F. B., Espigolan, R., Peripolli, E., Olivieri, B. F., et al. (2017). Application of single step genomic BLUP under different uncertain paternity scenarios using simulated data. PLoS ONE 12 (9), 01817522. doi:10.1371/journal.pone.0181752
Tsuruta, S., Lourenco, D. A. L., Masuda, Y., Misztal, I., and Lawlor, T. J. (2019). Controlling bias in genomic breeding values for young genotyped bulls. J. Dairy Sci. 102 (11), 9956–9970. doi:10.3168/jds.2019-16789
Tsuruta, S., Misztal, I., and Lawlor, T. J. (2013). Short communication: Genomic evaluations of final score for US Holsteins benefit from the inclusion of genotypes on cows. J. Dairy Sci. 96 (5), 3332–3335. doi:10.3168/jds.2012-6272
Van Eenennaam, A. L., van der Werf, J. H. J., and Goddard, M. E. (2011). The value of using DNA markers for beef bull selection in the seedstock sector. J. Animal Sci. 89 (2), 307–320. doi:10.2527/jas.2010-3223
Van Eenennaam, A. L., Weigel, K. A., Young, A. E., Cleveland, M. A., and Dekkers, J. C. M. (2014). Applied animal genomics: Results from the field. Annu. Rev. Animal Biosci. 2, 105–139. doi:10.1146/annurev-animal-022513-114119
VanRaden, P. M. (2012). Avoiding bias from genomic pre-selection in converting daughter information across countries. Interbull Bull. 45 (45), 1–5. Available at: https://journal.interbull.org/index.php/ib/article/view/1243%5Cnhttps://journal.interbull.org/index.php/ib/article/download/1243/1241.
VanRaden, P. M., Tooker, M. E., Cole, J. B., Wiggans, G. R., and Megonigal, J. H. (2007). Genetic evaluations for mixed-breed populations. J. Dairy Sci. 90 (5), 2434–2441. doi:10.3168/jds.2006-704
VanRaden, P. M., Van Tassell, C. P., Wiggans, G. R., Sonstegard, T. S., Schnabel, R. D., Taylor, J. F., et al. (2009). Invited review: Reliability of genomic predictions for North American Holstein bulls. J. Dairy Sci. 92 (1), 16–24. doi:10.3168/jds.2008-1514
Wang, X., Miao, J., Chang, T., Xia, J., Li, Y., Xu, L., et al. (2019). Evaluation of GBLUP, BayesB and elastic net for genomic prediction in Chinese simmental beef cattle. PLoS One 14 (2), 02104422. doi:10.1371/journal.pone.0210442
Wiggans, G. R., Cooper, T. A., Vanraden, P. M., and Cole, J. B. (2011). Technical note: Adjustment of traditional cow evaluations to improve accuracy of genomic predictions. J. Dairy Sci. 94 (12), 6188–6193. doi:10.3168/jds.2011-4481
Keywords: beef, genomic estimated breeding value, pedigree, SSGblup, meta-founder
Citation: Esrafili Taze Kand Mohammaddiyeh M, Rafat SA, Shodja J, Javanmard A and Esfandyari H (2023) Selective genotyping to implement genomic selection in beef cattle breeding. Front. Genet. 14:1083106. doi: 10.3389/fgene.2023.1083106
Received: 28 October 2022; Accepted: 28 February 2023;
Published: 17 March 2023.
Edited by:
Anupama Mukherjee, Indian Council of Agricultural Research (ICAR), IndiaReviewed by:
George R. Wiggans, Council on Dairy Cattle Breeding, United StatesCopyright © 2023 Esrafili Taze Kand Mohammaddiyeh, Rafat, Shodja, Javanmard and Esfandyari. This is an open-access article distributed under the terms of the Creative Commons Attribution License (CC BY). The use, distribution or reproduction in other forums is permitted, provided the original author(s) and the copyright owner(s) are credited and that the original publication in this journal is cited, in accordance with accepted academic practice. No use, distribution or reproduction is permitted which does not comply with these terms.
*Correspondence: Maryam Esrafili Taze Kand Mohammaddiyeh, bS5tLmVzcmFmaWxpQGdtYWlsLmNvbQ==; Seyed Abbas Rafat, YWJiYXNyYWZhdEBob3RtYWlsLmNvbQ==
Disclaimer: All claims expressed in this article are solely those of the authors and do not necessarily represent those of their affiliated organizations, or those of the publisher, the editors and the reviewers. Any product that may be evaluated in this article or claim that may be made by its manufacturer is not guaranteed or endorsed by the publisher.
Research integrity at Frontiers
Learn more about the work of our research integrity team to safeguard the quality of each article we publish.