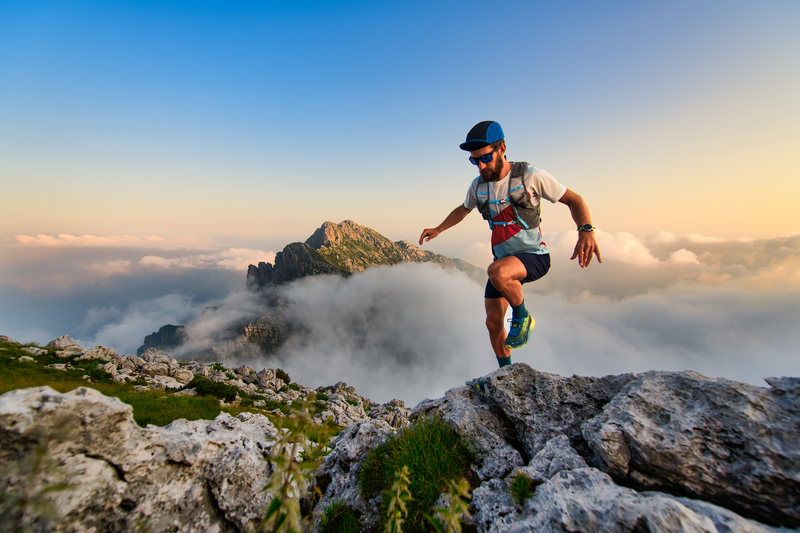
94% of researchers rate our articles as excellent or good
Learn more about the work of our research integrity team to safeguard the quality of each article we publish.
Find out more
ORIGINAL RESEARCH article
Front. Genet. , 23 February 2023
Sec. Cancer Genetics and Oncogenomics
Volume 14 - 2023 | https://doi.org/10.3389/fgene.2023.1007135
This article is part of the Research Topic Advances in Genetic and Epigenetic Mechanisms of Therapeutic Resistance in Cancer View all 11 articles
Introduction: GIST (gastrointestinal stromal tumor) is the most prominent mesenchymal neoplasms of the gastrointestinal tract, and liver is the most common metastasis site for GIST. The molecular mechanism leading to liver metastasis of GIST is currently unclear.
Methods: With the goal of revealing the underlying mechanism, we performed whole-genome gene expression profiling on 18 pairs of RNA samples comprised of GIST tissues (with liver metastasis) and corresponding non-tumor tissues. After identifying differentially expressed gene, functional annotation and signal pathway analyses were conducted. GSE13861, datasets that compare GIST (without liver metastasis) with adjacent tissues, served as a comparison.
Results: A total of 492 up-regulated genes and 629 down-regulated genes were identified as differentially expressed genes between liver metastasis tissues and non-tumor tissues. We characterized expression patterns of DEGs identified from our cohort and GSE13861 that show signatures of enrichment for functionality. In subsequent gene set enrichment analysis, differentially expressed genes were mainly enriched in Epithelial Mesenchymal Transition in both datasets. 493 genes were overlapped among our whole-genome gene expression profiling results and GSE13861, consisting 188 up-regulated genes and 305 down-regulated genes. By using CytoHubba plugin of Cytoscape, CDH1, CD34, KIT, PROM1, SOX9, FGF2, CD24, ALDH1A1, JAG1 and NES were identified as top ten hub genes in tumorigenesis and liver metastasis of GIST. higher expression levels of FGF2, JAG1, CD34, ALDH1A1 and the lower expression level of CDH1 were respectively associated with unfavorable overall survival. Meanwhile higher expression levels of CD34, FGF2, KIT, JAG1, ALDH1A were correlated with worse disease-free survival.
Discussion: The present study may help to provide candidate pathways and targets for treatment of GIST and prevention methods to liver metastasis.
GIST (gastrointestinal stromal tumor) is the most prominent mesenchymal neoplasms of the gastrointestinal tract, and their prevalence is on the rise (Corless et al., 2011). Activating mutations in the receptor tyrosine kinase encoding genes KIT (KIT proto-oncogene, receptor tyrosine kinase) or PDGFRA (platelet -derived growth factor receptor alpha) are extensively seen in GISTs (Serrano and George, 2020). These mutations cause constitutive activation of KIT or PDGFRA-mediated ligand independent activation and signaling (Joensuu et al., 2013). GISTs can appear everywhere in the gastrointestinal tract, although they’re most prevalent in the stomach (50%–60%) and small intestine (30%–35%), with the colon and rectum (5%) and oesophagus (1%) (Joensuu et al., 2012). Liver metastasis (LM) from GIST is very common, and a primary tumor is diagnosed simultaneously in 15%–50% of cases. Furthermore, after excision of a high-risk GIST, up to 40%–80% of individuals may emerge with liver metastasis over a period of about 2 years (Ng et al., 1992; DeMatteo et al., 2000; DeMatteo et al., 2009). However, the mechanisms of GIST invasion and acquisition of the potential to metastasize are still unknown. Acquiring a better knowledge of the molecular process behind liver metastasis of GIST is crucial, as it might result in new anticancer treatment targets and greatly contribute to advances in diagnostic approaches.
Gene chip, also known as gene profile, is a gene detection method that has been used for over a decade. Gene chips can instantly identify all of the genes’ expression information within the same sample time-point, making them ideal for detecting differentially expressed genes (DEGs) (Wang, 2000). Therefore, we collected GIST tissues of patients with liver metastasis and corresponding non-tumor tissues (stomach and intestinal tissue) yielding sufficient RNA for gene expression profiling. Meanwhile we also downloaded mRNA microarray data from the Gene Expression Omnibus (GEO) and jointly analyzed our gene expression profiling data with online data for identifying differentially expressed genes which may play an important role in tumorigenesis and liver metastasis of GIST. Gene Ontology (GO) annotation and Kyoto Encyclopedia of Genes and Genomes (KEGG) pathway enrichment analyses were applied to further provide an overview of the function of the screened DEGs. Then a protein-protein interaction (PPI) network was constructed to determine the hub genes and survival analyses of the screened hub genes were carried out using Gene Expression Profiling Interactive Analysis (GEPIA).
In this study, we first performed gene chip detection on GIST tumor sample and peri cancerous tissues of 18 GIST patients with liver metastases, obtained microarray dataset, and obtained organized microarray dataset of GIST with no liver metastasis and paracancer tissues from the GEO database. Differentially expressed genes were analyzed separately, and the enrichment of DEGs in the two datasets were analyzed. The STRING website and Cytoscape software were used to find out the key genes that promote the tumorigenesis and liver metastasis of GIST. Finally, we explored the potential of these key genes as prognostic markers of gastrointestinal tumors using Kaplan–Meier Survival analyses. This study helps us better understand the molecular mechanism of GSIT tumorigenesis and liver metastasis.
GIST tissues of patients with liver metastasis and corresponding non-tumor tissue (stomach and intestinal tissue) samples were obtained from Sun Yat-sen University Cancer Center under protocols approved by the institutional review board at Sun Yat-sen University Cancer Center. Written informed consent was obtained from all patients enrolled in the study. All experiments using clinical samples were carried out in accordance with the approved guidelines.
All samples were frozen in liquid nitrogen at −80°C. The total RNA of samples was extracted by TRIZOL method, and the total RNA was examined by NanoDrop 2000 and Agilent Bioanalyzer 2100. The qualified sample goes into the chip experiment. The standards of quality control are: Thermo NanoDrop 2000:1.7 < A260/A280 < 2.2; Agilent 2100 Bioanalyzer: RIN ≥ 7.0 and 28S/18S > 0.7. Affymetrix GeneChip Human Primeview array (Affymetrix, Santa Clara, CA, United States) was used to analyze global expression pattern of 28,869 well-annotated genes. RNA samples were amplified and labeled using the 3′IVT Expression Kit and GeneChip WT Terminal Labeling and Control Kit from Affymetrix. Affymetrix’s GeneChip Fluidics Station 450 was used to carry out the normal washing treatment after the samples were hybridized at 45°C for 16 h. The arrays were then scanned using the GeneChip Scanner 7G procedure. Quantile normalization of gene expression was performed using the normalizeBetweenArrays function in limma.
We also downloaded the following gene expression profiles from the GEO: GSE13861 (including six GIST and 19 surrounding normal fresh frozen tissues) (Cho et al., 2011) for further analysis.
R language limma package was used to identify DEGs in our cohort and GSE13861 separately. The log-fold change (FC) in expression and adjusted p-values (adj. P) were determined. The adj. P using the Benjamini–Hochberg method with default values were applied to correct the potential false-positive results. DEGs were defined as genes that satisfied the specified cutoff criterion of adj. p > 0.05 and | logFC | > 2.0. The Venn diagram online tool was used to look at the intersecting genes. In order to illustrate the volcano plot of DEGs, visual hierarchical cluster analysis was also carried out.
To reveal the functions of DEGs, GO annotation and KEGG pathway enrichment analyses were conducted. Biological process (BP), cellular component (CC), and molecular function (MF) were the three categories that made up the GO terms. Statistical significance was determined to be adj. p < 0.05. Resulting p-values are adjusted for multiple testing using the “Benjamini–Hochberg” method.
To find out the different mechanisms between GIST with liver metastasis and GIST without metastasis, GSEA (Version: 3.0; http://software.broadinstitute.org/gsea/index.jsp) was performed (Subramanian et al., 2005). The threshold was set at p < 0.05.
A database called Search Tool for the Retrieval of Interacting Genes (STRING) is used to study the functional protein association networks (Szklarczyk et al., 2017). The filtered DEGs had already been added to the STRING database. All PPI pairs with a cumulative score greater than 0.4 were retrieved. High-degree nodes seem to be essential for maintaining the network’s overall stability. The degree of all nodes was calculated by Cytoscape (v3.6.1) plugin cytoHubba using the MCC algorithm (Chin et al., 2014), in this experiment, the genes with the top 10 highest MCC score values were considered as hub genes.
Survival analysis of hub genes was based on Kaplan–Meier Survival analyses, using GEPIA (http://gepia.cancer-pku.cn/) tool. According to the expression of each hub gene, the cancer patients were divided into low or high expression group based on the median mRNA expression of hub genes, at statistical significance of p < 0.05.
Our cohort consisting of 18 paired GIST tissues of patients with liver metastasis (LM) and corresponding non-tumor tissue (NT) samples. Details of mutations, clinical features for the 18 GIST patients with liver metastasis are presented in Table 1. Eight of the 18 patients were male and 10 were female. The youngest patient was 23 and the oldest was 71. Four of the 18 GISTs are small-intestine GISTs, and the remaining 14 are stomach GISTs. All patients presented with liver metastases. And all of the patients harbored a single non-synonymous mutation in KIT (Kit exon 11). The tumor size, mitotic index and location of primary tumors are demonstrated in Table 1.
We developed a flow diagram to show our process (Figure 1). To characterize the tumor biology of GIST with liver metastasis, we performed whole-genome gene expression profiling in 18 pairs of RNA samples comprised of GIST with LM and NT tissues. 1121 genes were found to differentially express between LM and adjacent tissues, including 492 upregulated genes and 629 downregulated genes (Supplementary Data Sheet S1). Volcano map of DEGs was shown in Figure 2A. Subsequently, heatmap of DEGs was created, in which the mRNA expression profiles of LM and NT resulted in obviously separate clusters (Figure 2B). Principle Component Analysis (PCA) and hierarchical cluster analysis results were demonstrated in Figures 2C, D. GSE13861 (including 6 GIST and 19 surrounding normal fresh frozen tissues) is a dataset that compare GIST without liver metastasis with adjacent tissues, which serves as a comparison. DEGs in GSE13861 were calculated according to the criteria of p < 0.05 and |logFC|>2.0. 924 genes were found to differentially express between GIST and adjacent tissues, including 313 upregulated genes and 611 downregulated genes (Supplementary Data Sheet S2). Volcano map of DEGs is shown in Supplementary Figure S1A. Hierarchical clustering heatmap of DEGs was shown in Supplementary Figures S1B, C Shows PCA results of GSE13861. Hierarchical cluster analysis was visualized and important details were demonstrated in Supplementary Figure S1D.
FIGURE 2. Identification of differentially expressed genes. (A) Volcano map of differentially expressed genes (Upregulated genes in red, downregulated genes in blue). (B) Hierarchical clustering heatmap of DEGs screened on the basis of FC > 2.0 and a corrected p-value < 0.05. (C) Shows PCA results of our cohort. (D) Visual hierarchical cluster analysis.
To characterize the biological mechanism of GIST liver metastasis, gene enrichment analysis including Gene ontology (GO) and Kyoto Encyclopedia of Genes and Genomes (KEGG) pathway enrichment analyses were conducted. DEGs acquired from the two datasets were subjected to enrichment separately. For GO biological process (BP), DEGs in our cohort were mainly enriched in cell junction assembly, cell-substrate adhesion and urogenital development, while DEGs in GSE13861 were mainly enriched in extracellular matrix organization, extracellular structure organization and external encapsulating structure organization. In terms of cellular component (CC), DEGs in our cohort were mainly enriched in collagen-containing extracellular matrix, cell-cell junction and apical part of cell. The CC enrichment results of GSE13861 were very similar to our cohort. For GO molecular function (MF), results were also similar between these two cohorts (Figures 3A, B). We further explored the function significance of these DEGs using KEGG pathway analysis. DEGs in our cohort were mainly enriched in PI3K-Akt signaling pathway and Tight junction, while DEGs in GSE13861 were mainly enriched in Fluid shear stress and atherosclerosis and Metabolism of xenobiotics by cytochrome P450 (Figures 3C, D). Changes in gene expression in PI3K-Akt signaling pathway and Tight junction signaling pathways in our cohort are depicted in detail in Figures 4A, B.
FIGURE 3. GO and KEGG analysis of DEGs. GO analysis (A), and KEGG analysis (C) of DEGs in our cohort. GO analysis (B), and KEGG analysis (D) of DEGs in GSE13861 dataset.
FIGURE 4. Pathview map of (A) PI3K-AKT Signaling Pathway (map 04,151) and (B) Tight Junction (map04530) using data of our cohort. Upregulated genes in red, downregulated genes in green.
GSEA was performed to identify the gene sets that were statistically different between the normal controls and GIST group (Taking p < 0.05 as the boundary value). The results illustrated that Epithelial mesenchymal transition (EMT) was the most significantly upregulated pathway in both cohorts (Figures 5A–C, E). DEGs in our cohort were also positively correlated and significantly enriched in IL2 Stat5 Signaling (Figures 5A, D, NES = 1.767 & P.adj <0.001). While in GSE13861, IL2 Stat5 Signaling was not in the top10-enriched pathways (Figure 5B).
FIGURE 5. GSEA analysis of DEGs in the data sets. (A) The top 10 enriched KEGG items for the DEGs in our cohort, and (B) DEGs in GSE13861 dataset. Taking p < 0.05 as the boundary value. Significant enrichment of the Epithelial Mesenchymal Transition (C) and IL-2 STAT5 Signaling (D) with DEGs in our cohort. Significant enrichment of the Epithelial Mesenchymal Transition (E) and IL-2 STAT5 Signaling (F) with DEGs in GSE13861.
To identify those genes which play significant roles in both tumorigenesis and liver metastasis of GIST, GSE13861 dataset containing GIST primary tumor tissues (PT) and corresponding non-tumor tissues (NT) was co-analyzed. The Venn diagram (Figure 7A) illustrated a total of 493 genes overlapped among our microarray results and GSE13861, consisting 188 upregulated genes and 305 downregulated genes (Supplementary Data Sheet S3). Using the STRING and Cytoscape databases, a PPI network of potential interactions between overlapping genes was constructed (Figure 6). The hub genes were selected from the PPI network using the MCC algorithm of CytoHubba plugin. According to the MCC scores, the top ten highest-scored genes included CDH1, CD34, KIT, PROM1, SOX9, FGF2, CD24, ALDH1A1, JAG1, and NES (Figure 7B and Supplementary Data Sheet S4). The abbreviations, names, and functions of these genes are displayed in Table 2. The function of these hub genes was analyzed by Metascape, in which as expected, these genes were mainly enriched in pathways in cell-cell adhesion (Figure 7C).
FIGURE 6. Protein–protein interaction network of 188 upregulated genes and 305 downregulated genes were analyzed using Cytoscape software. The edges between 2 nodes represent the gene-gene interactions. Upregulated hub genes in red, downregulated hub genes in teal.
FIGURE 7. Hub genes selection and analysis. (A) Venn diagram shows the 493 overlapping DEGs. (B) The top 10 hub genes in the PPI network were screened by Cytoscape plugin cytoHubba. The 10 identified hub genes such as CDH1, CD34, KIT, PROM1, SOX9, FGF2, CD24, ALDH1A1, JAG1, NES are displayed from red (high degree value) to yellow (low degree value). (C) GO and KEGG pathway enrichment analysis of the 10 hub genes.
Among above mentioned 10 hub genes, the expressions of CD34, KIT, PROM1, NES, and FGF2 respectively were higher in GIST (with LM) tissues (Figures 8A–E) compared to NT tissues (p-values all <0.001). Meanwhile reverse trend was found for the expressions of the rest hub genes CDH1, SOX9, CD24, ALDH1A1, and JAG1 (Figures 8E–J, p-values all <0.001). These results are nearly identical to the findings from the GES13861 dataset (Figures 8K–T). Prognostic significance of hub genes was investigated in several types of gastrointestinal tumors including stomach adenocarcinoma, colon adenocarcinoma, esophageal carcinoma and rectal adenocarcinoma by the GEPIA database. The Kaplan-Meier analyses suggested that higher expression levels of FGF2, JAG1, CD34, and ALDH1A1 and the lower expression level of CDH1 were respectively associated with worse overall survival (OS) (Figure 9). Meanwhile higher expression levels of CD34, FGF2, KIT, JAG1, and ALDH1A were correlated with worse disease-free survival (DFS) (Figure 10).
FIGURE 8. Expression of hub genes. (A–J). Expression of KIT, CD34, FGF2, PROM1, NES, CDH1, CD24, ALDH1A1, JAG1 and SOX9 in Our cohort and (K–T) GSE13861. *p < 0.05, **p < 0.01, ***p < 0.001.
FIGURE 9. Kaplan-Meier curves of hub genes expression and overall survival in gastrointestinal tumors. Data are presented as the hazard ratio with a 95% confidence interval. Log-rank p < 0.05 was regarded as statistically significant.
FIGURE 10. Kaplan-Meier curves of hub genes expression and disease-free survival in gastrointestinal tumors. Data are presented as the hazard ratio with a 95% confidence interval. Log-rank p < 0.05 was regarded as statistically significant.
During the past decade, GIST has become the prominent focus of molecularly targeted therapy for solid tumors (Poveda et al., 2017; Hemming et al., 2018). GIST are more prevalent than previously thought, according to population-based studies (Corless and Heinrich, 2008). The incidence of GIST was found to be 14.5 per million population, with the highest frequency being observed in older individuals and there was no gender difference (Gold and DeMatteo, 2006). The hallmarks of cancer consist of six biological traits: sustaining proliferative signaling, evading growth suppressors, evasion of apoptosis, limitless replicative potential, inducing angiogenesis, and ability to invade and metastasize (Hanahan and Weinberg, 2011). It is worth noting that the last characteristic, invasion and metastasis is vital for progressive nature of cancer. Many malignancies favor certain organs as metastatic sites, including the lungs, bone marrow, and liver. Liver metastases are a major cause of death in patients with colorectal cancer. The liver environment, which includes ECM and stromal cells, may encourage metastatic colonization. Metastatic colorectal cancer cell lines responded more favorably to ECM derived from primary rat hepatocytes than to ECM from fetal rat fibroblast cultures (Zvibel et al., 1998). The D6.1A tetraspanin, a cell-surface organizer, interacted with the 64 integrin and enhanced liver colonization by pancreatic cancer cells injected intraperitoneally (Herlevsen et al., 2003).
Patients with GIST have a high risk of recurrence (about 55–72 percent) and a dismal survival rate due to malignant cells preferentially metastasizing to liver tissue (DeMatteo et al., 2000; Bayraktar et al., 2010). Cho et al. discovered that Compared to KIT mutation-negative GISTs, KIT mutation-positive GISTs had more frequent liver metastases and worse mortality (Cho et al., 2006). Wang et al. reported that the KIT exon 11,557-558 deletion upregulates CXCR4 by increasing ETV1 binding to the CXCR4 promoter in GIST cells, which in turn encourages liver metastasis (Wang et al., 2016). As such, to better understand GIST biological behavior and inform the development of treatment strategies, it is critical to identify the significant genes that regulate the liver metastasis of GIST. Advances in bioinformatics have been conducive to identify molecular targets that indicate the progression of GIST (Amirnasr et al., 2019; Ohshima et al., 2019).
In this study, a total of 492 upregulated genes and 629 downregulated genes were identified in GIST with LM compared to corresponding NT. Function annotation based on GO and KEGG analyses demonstrated that DEGs were mainly enriched in cell junction assembly, tight junction, actin binding and PI3K-Akt signaling pathway. GSEA results indicated that IL-2 STAT5 Signaling may be a vital pathway which promotes liver metastasis of GIST. Meanwhile, EMT signal pathway is the most significant and positive enriched pathway in both our cohort and GSE13861, which indicated that EMT may play a significant role in tumorigenesis and liver metastasis of GIST. Furthermore, to identify genes which play essential roles in both tumorigenesis and liver metastasis of GIST, our data and GSE13861 dataset were co-analyzed. A totally of 493 genes overlapped among our microarray results and GSE13861, including 188 upregulated genes and 305 downregulated genes. Then a PPI network of putative interactions between overlapping genes was created using the STRING and Cytoscape databases and hub genes were selected from the PPI network using the MCC algorithm of CytoHubba plugin. According to the MCC scores, the top ten highest-scored genes were CDH1, CD34, KIT, PROM1, SOX9, FGF2, CD24, ALDH1A1, JAG1, and NES.
The phosphatidylinositol PI3K/AKT/mTOR pathway is a critical survival pathway for cell proliferation, apoptosis, autophagy and translation in neoplasms (Patel, 2013). Constitutive autophosphorylation of RTKs has an impact on the activation of the PI3K/AKT/mTOR pathway (Vara et al., 2004; Fruman and Rommel, 2014). In several preclinical and early-stage clinical trials PI3K/AKT/mTOR signaling inhibition has been considered as a promising targeted therapy strategy for GISTs (Duan et al., 2020). Our results suggest that, unlike GIST, liver-metastatic GIST has more genes enriched in the PI3K-Akt signaling pathway. We hypothesized that PI3K-Akt signaling pathway is an important pathway to promote liver metastasis of GIST. It can be used as a target to prevent and treat liver metastasis of GIST.
Tight junction is the most talked-about structure in epithelial and endothelial cells because they control permeability (Jiang et al., 1999; Tsukita et al., 1999). It is an area where neighboring cells’ plasma membranes make a sequence of connections that appear to totally obstruct the extracellular space, forming an intercellular barrier and intramembrane diffusion fence (Wong and Gumbiner, 1997). The majority of malignancies are characterized by abnormal growth control, tissue architecture loss, and loss of differentiation. The feature that cancer cells’ mutual adhesiveness is much less than that of normal cells is a key characteristic of cancer cells (Martin and Jiang, 2009). Reduced cell-cell interaction leads cancer cells to rebel against the social order, resulting in the breakdown of overall tissue architecture, a morphological hallmark of malignancy. The loss cell-cell junction and tight junction are changes associated with cancer progression to an invasive, metastatic state (Thomson et al., 2011).
The cytokine interleukin-2 (IL-2) was first discovered in 1976 as a T cell growth factor (Morgan et al., 1976). While IL-2 has been shown to activate several STAT family members, including STAT1, STAT3, and STAT5, STAT5 is the predominant IL-2 signaling molecule (Hou et al., 1995; Lin et al., 1995). Indeed, IL-2 has also been shown to signal via the Mitogen Activated Protein Kinase (MAPK) pathway, via extracellular signal-regulated kinase (ERK), as well as the PI3K pathway (González-García et al., 1997; Liao et al., 2013; Ross and Cantrell, 2018). In this study, we identified IL-2 STAT5 Signaling is the second and positively enriched pathway using GSEA in DEGs in our cohort, while in GSE13861, IL-2 Stat5 Signaling was not in the top10-enriched pathways. This result indicates that IL-2 STAT5 Signaling may be a vital pathway which promotes liver metastasis of GIST.
The extracellular matrix (ECM) performs many functions in addition to its structural role; as a major component of the cellular microenvironment it influences cell behaviors such as proliferation, adhesion and migration, and regulates cell differentiation and death (Hynes, 2009). Abnormal ECM dynamics can result in uncontrolled cell proliferation and invasion, failure of cell death, and loss of cell differentiation, which can lead in congenital abnormalities and pathological processes such as tissue fibrosis and cancer. As the ECM’s significance in tumor progression becomes more evident, cancer treatment strategies have started to focus on specific ECM components in an effort to reduce metastasis (Walker et al., 2018; Paolillo and Schinelli, 2019; Girigoswami et al., 2021).
Epithelial mesenchymal transition (EMT) is a crucial developmental process that triggers the transdifferentiation of polarized epithelial cells into mesenchymal cells during tumor invasion and metastasis (Kalluri and Weinberg, 2009; Polyak and Weinberg, 2009). Cancer cells acquire invasive and metastatic characteristics with activation of EMT, which facilitates effective colonization of distal target organs (Tsai and Yang, 2013). In line with previous study, we found that EMT signal pathway enriched in GIST tissues of patients with liver metastasis compared to corresponding pericancerous tissues, which indicated that EMT may play a significant role in liver metastasis of GIST.
E-cadherin (also known as cadherin-1 or CDH1), a protein belonging to the cadherin family, is possibly one of the most potent and extensively researched regulators of adhesion. Together with associated Catenins, E-cadherin is essential for regulating cell adhesion, signaling and transcription in cancers and controlling metastatic progression (Jiang and Mansel, 2000). Alteration in cell adhesion molecules (CAMs), such as E-cadherin affect the processes of cell-cell adhesion and cell-matrix adhesion and subsequently their metastatic potential. It also regulates the cell cycle regulators p27kip1 and p57kip2, which are essential for cell-cell contact inhibition in healthy tissue but are lost or disrupted in cancer cells, primarily due to the loss of E-cadherin in cancer cells (Croix et al., 1998; Cavallaro and Christofori, 2004a; Migita et al., 2008). Therefore, decreased cell-cell adhesion not only increases the potential for metastatic dissemination of cancer cells, but also encourages unchecked cell proliferation through the absence of contact inhibition (Cavallaro and Christofori, 2004b). Indeed, studies has shown a correlation between reduced E-cadherin and α-catenin expression with increased tumor cell invasiveness (Zschiesche et al., 1997). Sheng Liu et al. demonstrated that reduced E-cadherin expression was correlated with distant metastasis of GIST and E-cadherin was thus considered as risk factor for GIST metastasis. In our study, E-cadherin had been identified as the top hub gene and to be involved in the process of tumorigenesis and liver metastasis of GIST. The results of our study demonstrated decreased expression levels of E-cadherin were associated with unfavorable OS in gastrointestinal tumors. Therefore, we believe that it mediates the liver metastasis of GIST and can be used as a target for the treatment of metastatic GIST.
ETV1, a transcription factor from the ETS family, is a master regulator of the normal lineage specification and development of the ICCs which are the precursors to GIST (Chi et al., 2010). Hao-Chen Wang et al. reported that upregulating ETV1 expression induced CXCR4 expression, which promoted liver metastasis of GIST (Wang et al., 2016). We compared ETV1 expression in our cohort and found that ETV1 are upregulated in GIST tissues of patients with liver metastasis compared with corresponding non-tumor tissue (Supplementary Figures S2A, C). Our result supports ETV1’s stimulative role in liver metastasis of GIST. Besides, it has been demonstrated that ETV4 expression impacted Wnt/catenin signaling and was correlated to an aggressive phenotype in GIST (Zeng et al., 2017). However, our results showed no significant difference in ETV1 expression levels in GIST compared to the adjacent tissues in both our cohort and GSE13861 (Supplementary Figures S2B, D). Further research in this area is needed.
The major limitation of the present study is that Tumor transcriptome programs are rather diverse, both within tumor cells due to somatic genetic changes and within tumor microenvironments due to extensive infiltration of the stroma and other cell types in the tumor. An average gene expression profile from microarray can mask the real signals causing the liver metastasis of GIST from a rare cell population or cell type. Besides, it has been indicated that long non-coding RNAs (LncRNAs) participate in certain pro-metastatic stages, such as the epithelial mesenchymal transition, invasion and migration, and organotrophic colonization, and they also have an impact on the metastatic tumor microenvironment (Amirnasr et al., 2020; Liu et al., 2021). The gene chips we used in current study only contain probes for protein-coding mRNAs but not LncRNAs. Thus, further researches should be conducted to elucidate the potential function of LncRNAs in liver metastasis of GIST. Moreover, a direct comparison of liver metastases and primary sites of GIST maybe a better study protocol. But, on one hand, liver metastases from GIST patients are difficult to obtain because they are usually treated by ablation. On the other hand, we think that the transcription level of GIST with liver metastasis has already changed before metastasis, the potential role of these genes in promoting liver metastasis cannot be ignored. This information is lost if direct compare liver metastases samples and primary lesions. It would be better if we collected GIST specimens without liver metastasis and adjacent tissues at the same time. This reduces batch effects compared to using data from GEO databases for comparison. Furthermore, there is currently no public database contains both prognostic and gene sequencing data of GIST. And, our cohort contained too few cases (only 18 patients) to survival analysis. So, we can only retreat to the next best, using TCGA database for survival analysis. Whether these hub genes in GIST have prognostic value remains to be further confirmed.
In summary, through analyzing data of self-made whole-genome gene expression profiling and GEO dataset, we identified those signal pathways and hub genes that played significant roles in the tumorigenesis and liver metastasis of GIST. Further studies with larger sample sizes should be carried out to validate the present findings. Additionally, experimental evidence is warranted to investigate the functional roles of the identified hub genes in the liver metastasis of GIST. We sincerely hope that this present study will contribute to the discovery of therapeutic target for liver metastatic GIST.
The original contributions presented in the study are included in the article/Supplementary Materials, further inquiries can be directed to the corresponding author.
HQ: Conceptualization; Supervision; Project administration. JG: Investigation; Formal analysis; Writing—review and editing. SF: Investigation; Writing–original Draft. HY, BO, and DJ: Data collecting. CD, XC, and MZ: Writing–Review and Editing. YL and YZ: Data Curation. WZ: Collecting of clinical samples.
This work was supported by NSFC grants (NSFC 81602061).
The authors would like to thank their patients for their participation in the study.
The authors declare that the research was conducted in the absence of any commercial or financial relationships that could be construed as a potential conflict of interest.
All claims expressed in this article are solely those of the authors and do not necessarily represent those of their affiliated organizations, or those of the publisher, the editors and the reviewers. Any product that may be evaluated in this article, or claim that may be made by its manufacturer, is not guaranteed or endorsed by the publisher.
The Supplementary Material for this article can be found online at: https://www.frontiersin.org/articles/10.3389/fgene.2023.1007135/full#supplementary-material
SUPPLEMENTARY FIGURE S1 | Identification of differentially expressed genes of GSE13861 dataset. (A) Volcano map of differentially expressed genes (Upregulated genes in red, downregulated genes in blue). (B) Hierarchical clustering heatmap of DEGs screened on the basis of FC >2.0 and a corrected P value <0.05. (C) Shows PCA results of our cohort. (D) Visual hierarchical cluster analysis.
SUPPLEMENTARY FIGURE S2 | Expression of ETV1 and ETV4. (A, B) Expression of ETV1 and ETV4 in GIST (with liver metastasis) tissues and correspond non-tumor tissues in our cohort. (C, D). Expression of ETV1 and ETV4 in primary GIST tissues and correspond non-tumor tissues in GSE13861 dataset.
Amirnasr, A., Gits, C. M. M., van Kuijk, P. F., Smid, M., Vriends, A. L. M., Rutkowski, P., et al. (2019). Molecular comparison of imatinib-naïve and resistant gastrointestinal stromal tumors: Differentially expressed microRNAs and mRNAs. Cancers (Basel) 11, 882. doi:10.3390/cancers11060882
Amirnasr, A., Sleijfer, S., and Wiemer, E. A. C. (2020). Non-coding RNAs, a novel paradigm for the management of gastrointestinal stromal tumors. Int. J. Mol. Sci. 21, 6975. doi:10.3390/ijms21186975
Bayraktar, U. D., Bayraktar, S., and Rocha-Lima, C. M. (2010). Molecular basis and management of gastrointestinal stromal tumors. World J. Gastroenterol. 16, 2726–2734. doi:10.3748/wjg.v16.i22.2726
Cavallaro, U., and Christofori, G. (2004). Cell adhesion and signalling by cadherins and Ig-CAMs in cancer. Nat. Rev. Cancer 4, 118–132. doi:10.1038/nrc1276
Cavallaro, U., and Christofori, G. (2004). Multitasking in tumor progression: Signaling functions of cell adhesion molecules. Ann. N. Y. Acad. Sci. 1014, 58–66. doi:10.1196/annals.1294.006
Chi, P., Chen, Y., Zhang, L., Guo, X., Wongvipat, J., Shamu, T., et al. (2010). ETV1 is a lineage survival factor that cooperates with KIT in gastrointestinal stromal tumours. Nature 467, 849–853. doi:10.1038/nature09409
Chin, C.-H., Chen, S. H., Wu, H. H., Ho, C. W., Ko, M. T., and Lin, C. Y. (2014). cytoHubba: identifying hub objects and sub-networks from complex interactome. BMC Syst. Biol. 8, S11. doi:10.1186/1752-0509-8-S4-S11
Cho, S., Kitadai, Y., Yoshida, S., Tanaka, S., Yoshihara, M., Yoshida, K., et al. (2006). Deletion of the KIT gene is associated with liver metastasis and poor prognosis in patients with gastrointestinal stromal tumor in the stomach. Int. J. Oncol. 28, 1361–1367. doi:10.3892/ijo.28.6.1361
Cho, J. Y., Lim, J. Y., Cheong, J. H., Park, Y. Y., Yoon, S. L., Kim, S. M., et al. (2011). Gene expression signature–based prognostic risk score in gastric cancer. Clin. Cancer Res. 17, 1850–1857. doi:10.1158/1078-0432.CCR-10-2180
Corless, C. L., and Heinrich, M. C. (2008). Molecular pathobiology of gastrointestinal stromal sarcomas. Annu. Rev. Pathol. Mech. Dis. 3, 557–586. doi:10.1146/annurev.pathmechdis.3.121806.151538
Corless, C. L., Barnett, C. M., and Heinrich, M. C. (2011). Gastrointestinal stromal tumours: Origin and molecular oncology. Nat. Rev. Cancer 11, 865–878. doi:10.1038/nrc3143
Croix, B., Rak, J. W., Florenes, V. A., Slingerland, J. M., and Kerbel, R. S. (1998). E-Cadherin–dependent growth suppression is mediated by the cyclin-dependent kinase inhibitor p27KIP1. J. Cell Biol. 142, 557–571. doi:10.1083/jcb.142.2.557
DeMatteo, R. P., Lewis, J. J., Leung, D., Mudan, S. S., Woodruff, J. M., and Brennan, M. F. (2000). Two hundred gastrointestinal stromal tumors: Recurrence patterns and prognostic factors for survival. Ann. Surg. 231, 51–58. doi:10.1097/00000658-200001000-00008
DeMatteo, R. P., Ballman, K. V., Antonescu, C. R., Maki, R. G., Pisters, P. W. T., Demetri, G. D., et al. (2009). Adjuvant imatinib mesylate after resection of localised, primary gastrointestinal stromal tumour: A randomised, double-blind, placebo-controlled trial. Lancet 373, 1097–1104. doi:10.1016/S0140-6736(09)60500-6
Duan, Y., Haybaeck, J., and Yang, Z. (2020). Therapeutic potential of PI3K/AKT/mTOR pathway in gastrointestinal stromal tumors: Rationale and progress. Cancers (Basel) 12, 2972. doi:10.3390/cancers12102972
Fruman, D. A., and Rommel, C. (2014). PI3K and cancer: Lessons, challenges and opportunities. Nat. Rev. Drug Discov. 13, 140–156. doi:10.1038/nrd4204
Girigoswami, K., Saini, D., and Girigoswami, A. (2021). Extracellular matrix remodeling and development of cancer. Stem Cell Rev Rep 17, 739–747. doi:10.1007/s12015-020-10070-1
Gold, J. S., and DeMatteo, R. P. (2006). Combined surgical and molecular therapy: The gastrointestinal stromal tumor model. Ann. Surg. 244, 176–184. doi:10.1097/01.sla.0000218080.94145.cf
González-García, A., Mérida, I., Martinez-A, , C., and Carrera, A. C. (1997). Intermediate affinity interleukin-2 receptor mediates survival via a phosphatidylinositol 3-Kinase-dependent pathway. J. Biol. Chem. 272, 10220–10226. doi:10.1074/jbc.272.15.10220
Hanahan, D., and Weinberg, R. A. (2011). Hallmarks of cancer: The next generation. Cell 144, 646–674. doi:10.1016/j.cell.2011.02.013
Hemming, M. L., Heinrich, M. C., Bauer, S., and George, S. (2018). Translational insights into gastrointestinal stromal tumor and current clinical advances. Ann. Oncol. 29, 2037–2045. doi:10.1093/annonc/mdy309
Herlevsen, M., Schmidt, D.-S., Miyazaki, K., and Zöller, M. (2003). The association of the tetraspanin D6.1A with the alpha6beta4 integrin supports cell motility and liver metastasis formation. J. Cell Sci. 116, 4373–4390. doi:10.1242/jcs.00760
Hou, J., Schindler, U., Henzel, W. J., Wong, S. C., and McKnight, S. L. (1995). Identification and purification of human stat proteins activated in response to interleukin-2. Immunity 2, 321–329. doi:10.1016/1074-7613(95)90140-x
Hynes, R. O. (2009). The extracellular matrix: Not just pretty fibrils. Science 326, 1216–1219. doi:10.1126/science.1176009
Jiang, W. G., and Mansel, R. E. (2000). E-cadherin complex and its abnormalities in human breast cancer. Surg. Oncol. 9, 151–171. doi:10.1016/s0960-7404(01)00010-x
Jiang, W. G., Martin, T. A., Matsumoto, K., Nakamura, T., and Mansel, R. E. (1999). Hepatocyte growth factor/scatter factor decreases the expression of occludin and transendothelial resistance (TER) and increases paracellular permeability in human vascular endothelial cells. J. Cell. Physiol. 181, 319–329. doi:10.1002/(SICI)1097-4652(199911)181:2<319::AID-JCP14>3.0.CO;2-S
Joensuu, H., Vehtari, A., Riihimaki, J., Nishida, T., Steigen, S. E., Brabec, P., et al. (2012). Risk of recurrence of gastrointestinal stromal tumour after surgery: An analysis of pooled population-based cohorts. Lancet Oncol. 13, 265–274. doi:10.1016/S1470-2045(11)70299-6
Joensuu, H., Hohenberger, P., and Corless, C. L. (2013). Gastrointestinal stromal tumour. Lancet 382, 973–983. doi:10.1016/S0140-6736(13)60106-3
Kalluri, R., and Weinberg, R. A. (2009). The basics of epithelial-mesenchymal transition. J. Clin. Invest. 119, 1420–1428. doi:10.1172/JCI39104
Liao, W., Lin, J.-X., and Leonard, W. J. (2013). Interleukin-2 at the crossroads of effector responses, tolerance, and immunotherapy. Immunity 38, 13–25. doi:10.1016/j.immuni.2013.01.004
Lin, J.-X., Migone, T. S., Tsang, M., Friedmann, M., Weatherbee, J. A., Zhou, L., et al. (1995). The role of shared receptor motifs and common stat proteins in the generation of cytokine pleiotropy and redundancy by IL-2, IL-4, IL-7, IL-13, and IL-15. Immunity 2, 331–339. doi:10.1016/1074-7613(95)90141-8
Liu, S. J., Dang, H. X., Lim, D. A., Feng, F. Y., and Maher, C. A. (2021). Long noncoding RNAs in cancer metastasis. Nat. Rev. Cancer 21, 446–460. doi:10.1038/s41568-021-00353-1
Martin, T. A., and Jiang, W. G. (2009). Loss of tight junction barrier function and its role in cancer metastasis. Biochim. Biophys. Acta (BBA) - Biomembr. 1788, 872–891. doi:10.1016/j.bbamem.2008.11.005
Migita, T., Oda, Y., Masuda, K., Hirata, A., Kuwano, M., Naito, S., et al. (2008). Inverse relationship between E-cadherin and p27Kip1 expression in renal cell carcinoma. Int. J. Oncol. 33, 41–47. doi:10.3892/ijo.33.1.41
Morgan, D. A., Ruscetti, F. W., and Gallo, R. (1976). Selective in vitro growth of T lymphocytes from normal human bone marrows. Science 193, 1007–1008. doi:10.1126/science.181845
Ng, E. H., Pollock, R. E., Munsell, M. F., Atkinson, E. N., and Romsdahl, M. M. (1992). Prognostic factors influencing survival in gastrointestinal leiomyosarcomas. Implications for surgical management and staging. Ann. Surg. 215, 68–77. doi:10.1097/00000658-199201000-00010
Ohshima, K., Fujiya, K., Nagashima, T., Ohnami, S., Hatakeyama, K., Urakami, K., et al. (2019). Driver gene alterations and activated signaling pathways toward malignant progression of gastrointestinal stromal tumors. Cancer Sci. 110, 3821–3833. doi:10.1111/cas.14202
Paolillo, M., and Schinelli, S. (2019). Extracellular matrix alterations in metastatic processes. Int. J. Mol. Sci. 20, 4947. doi:10.3390/ijms20194947
Patel, S. (2013). Exploring novel therapeutic targets in GIST: Focus on the PI3K/Akt/mTOR pathway. Curr. Oncol. Rep. 15, 386–395. doi:10.1007/s11912-013-0316-6
Polyak, K., and Weinberg, R. A. (2009). Transitions between epithelial and mesenchymal states: Acquisition of malignant and stem cell traits. Nat. Rev. Cancer 9, 265–273. doi:10.1038/nrc2620
Poveda, A., Garcia Del Muro, X., Lopez-Guerrero, J. A., Cubedo, R., Martinez, V., Romero, I., et al. (2017). GEIS guidelines for gastrointestinal sarcomas (GIST). Cancer Treat. Rev. 55, 107–119. doi:10.1016/j.ctrv.2016.11.011
Ross, S. H., and Cantrell, D. A. (2018). Signaling and function of interleukin-2 in T lymphocytes. Annu. Rev. Immunol. 36, 411–433. doi:10.1146/annurev-immunol-042617-053352
Serrano, C., and George, S. (2020). Gastrointestinal stromal tumor: Challenges and opportunities for a new decade. Clin. Cancer Res. 26, 5078–5085. doi:10.1158/1078-0432.CCR-20-1706
Subramanian, A., Tamayo, P., Mootha, V. K., Mukherjee, S., Ebert, B. L., Gillette, M. A., et al. (2005). Gene set enrichment analysis: A knowledge-based approach for interpreting genome-wide expression profiles. Proc. Natl. Acad. Sci. U.S.A. 102, 15545–15550. doi:10.1073/pnas.0506580102
Szklarczyk, D., Morris, J. H., Cook, H., Kuhn, M., Wyder, S., Simonovic, M., et al. (2017). The STRING database in 2017: Quality-controlled protein–protein association networks, made broadly accessible. Nucleic Acids Res. 45, D362–D368. doi:10.1093/nar/gkw937
Thomson, S., Petti, F., Sujka-Kwok, I., Mercado, P., Bean, J., Monaghan, M., et al. (2011). A systems view of epithelial–mesenchymal transition signaling states. Clin. Exp. Metastasis 28, 137–155. doi:10.1007/s10585-010-9367-3
Tsai, J. H., and Yang, J. (2013). Epithelial–mesenchymal plasticity in carcinoma metastasis. Genes Dev. 27, 2192–2206. doi:10.1101/gad.225334.113
Tsukita, S., Furuse, M., Tsukita, S., and Furuse, M. (1999). Occludin and claudins in tight-junction strands: Leading or supporting players? Trends Cell Biol. 9, 268–273. doi:10.1016/s0962-8924(99)01578-0
Vara, J. Á. F., Casado, E., de Castro, J., Cejas, P., Belda-Iniesta, C., and Gonzalez-Baron, M. (2004). PI3K/Akt signalling pathway and cancer. Cancer Treat. Rev. 30, 193–204. doi:10.1016/j.ctrv.2003.07.007
Walker, C., Mojares, E., and del Río Hernández, A. (2018). Role of extracellular matrix in development and cancer progression. Int. J. Mol. Sci. 19, 3028. doi:10.3390/ijms19103028
Wang, H.-C., Li, T. Y., Chao, Y. J., Hou, Y. C., Hsueh, Y. S., Hsu, K. H., et al. (2016). KIT exon 11 codons 557–558 deletion mutation promotes liver metastasis through the CXCL12/CXCR4 Axis in gastrointestinal stromal tumors. Clin. Cancer Res. 22, 3477–3487. doi:10.1158/1078-0432.CCR-15-2748
Wang, J. (2000). From DNA biosensors to gene chips. Nucleic Acids Res. 28, 3011–3016. doi:10.1093/nar/28.16.3011
Wong, V., and Gumbiner, B. M. (1997). A synthetic peptide corresponding to the extracellular domain of occludin perturbs the tight junction permeability barrier. J. Cell Biol. 136, 399–409. doi:10.1083/jcb.136.2.399
Zeng, S., Seifert, A. M., Zhang, J. Q., Kim, T. S., Bowler, T. G., Cavnar, M. J., et al. (2017). ETV4 collaborates with Wnt/β-catenin signaling to alter cell cycle activity and promote tumor aggressiveness in gastrointestinal stromal tumor. Oncotarget 8, 114195–114209. doi:10.18632/oncotarget.23173
Zschiesche, W., Schonborn, I., Behrens, J., Herrenknecht, K., Hartveit, F., Lilleng, P., et al. (1997). Expression of E-cadherin and catenins in invasive mammary carcinomas. Anticancer Res. 17, 561–567.
Keywords: gastrointestinal stromal tumor, epithelial mesenchymal transition, liver metastasis, tumorigenesis, differentially expressed genes (DEG)
Citation: Guo J, Feng S, Yu H, Ou B, Jiang D, Zhuang W, Ding C, Chen X, Zhang M, Ling Y, Zeng Y and Qiu H (2023) Transcriptomic study of gastrointestinal stromal tumors with liver metastasis. Front. Genet. 14:1007135. doi: 10.3389/fgene.2023.1007135
Received: 30 July 2022; Accepted: 10 February 2023;
Published: 23 February 2023.
Edited by:
Yilin Zhang, The University of Chicago, United StatesReviewed by:
Silke Cameron, University Medical Center Göttingen, GermanyCopyright © 2023 Guo, Feng, Yu, Ou, Jiang, Zhuang, Ding, Chen, Zhang, Ling, Zeng and Qiu. This is an open-access article distributed under the terms of the Creative Commons Attribution License (CC BY). The use, distribution or reproduction in other forums is permitted, provided the original author(s) and the copyright owner(s) are credited and that the original publication in this journal is cited, in accordance with accepted academic practice. No use, distribution or reproduction is permitted which does not comply with these terms.
*Correspondence: Haibo Qiu, cWl1aGJAc3lzdWNjLm9yZy5jbg==
†These authors have contributed equally to this work and share first authorship
Disclaimer: All claims expressed in this article are solely those of the authors and do not necessarily represent those of their affiliated organizations, or those of the publisher, the editors and the reviewers. Any product that may be evaluated in this article or claim that may be made by its manufacturer is not guaranteed or endorsed by the publisher.
Research integrity at Frontiers
Learn more about the work of our research integrity team to safeguard the quality of each article we publish.