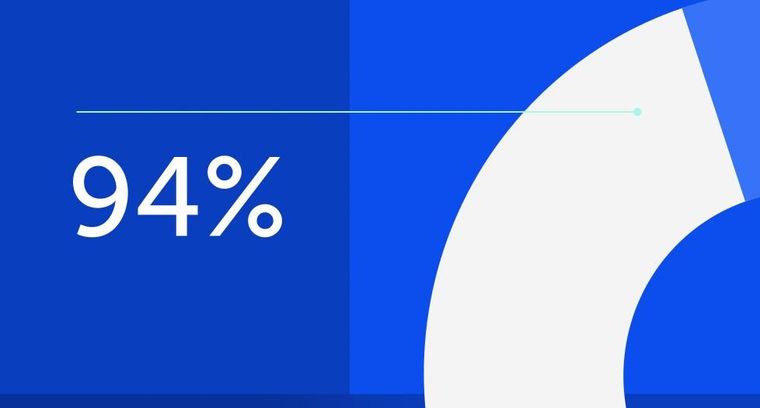
94% of researchers rate our articles as excellent or good
Learn more about the work of our research integrity team to safeguard the quality of each article we publish.
Find out more
REVIEW article
Front. Genet., 11 October 2022
Sec. Genomic Assay Technology
Volume 13 - 2022 | https://doi.org/10.3389/fgene.2022.996890
This article is part of the Research TopicEmerging Talents in Genomic Assay TechnologyView all 8 articles
Tumors are a class of diseases characterized by altered genetic information and uncontrolled growth. Sequencing technology provide researchers with a better way to explore specific tumor pathogenesis. In recent years, single-cell sequencing technology has shone in tumor research, especially in the study of liver cancer, revealing phenomena that were unexplored by previous studies. Single-cell sequencing (SCS) is a technique for sequencing the cellular genome, transcriptome, epigenome, proteomics, or metabolomics after dissociation of tissues into single cells. Compared with traditional bulk sequencing, single-cell sequencing can dissect human tumors at single-cell resolution, finely delineate different cell types, and reveal the heterogeneity of tumor cells. In view of the diverse pathological types and complex pathogenesis of hepatocellular carcinoma (HCC), the study of the heterogeneity among tumor cells can help improve its clinical diagnosis, treatment and prognostic judgment. On this basis, SCS has revolutionized our understanding of tumor heterogeneity, tumor immune microenvironment, and clonal evolution of tumor cells. This review summarizes the basic process and development of single-cell sequencing technology and its increasing role in the field of hepatocellular carcinoma.
Primary liver cancer (PLC) is one of the common gastrointestinal tract malignant tumors, and its morbidity and mortality have been high (Sung et al., 2021). LPC is histologically divided into three types: hepatocellular carcinoma (HCC), intrahepatic cholangiocarcinoma (ICC) and mixed hepatocellular carcinoma (cHCC-CCA). HCC accounts for more than 75% of PLC (McGlynn et al., 2015). China is a large country with high morbidity of liver cancer, which accounts for more than 50% of the total number of cases in the world (Zheng et al., 2018a). The current exploration of hepatocellular carcinoma has progressed from morphological studies in the early years to studies at the genetic level. As a disease closely associated with genetic material, tumors often show abnormal changes in cytogenetics and epigenetics, which are considered the major determinants of cancer at the both symptomatic and genetic levels (Lipinski et al., 2016). One of the long-standing challenges in biology and medicine is comprehensively characterize genotype-to-phenotype changes (Li and Clevers, 2010). However, the transcriptome information in any single cell reflects the activation of only a subset of genes, even though all cells in our body share nearly the same genotype (Huang, 2009). Furthermore, many different cell types in our bodies express unique transcriptomes, traditional bulk-sequencing are limited to measuring the average expression signal of cells contained in the tissue being tested (Shalek et al., 2014). A growing body of evidence further suggests that intracellular gene expression or transcriptome information is inconsistent even within similar cell types. However, at present, most transcriptome analysis are still based on the hypothesis that the genetic information of cells within the sampled tissue is consistent (Eldar and Elowitz, 2010). Thus, these researches may miss significant intercellular variability. For a better understanding of the biological processes of tumor progression, a more precise comprehension of the heterogeneity of genetic information in single cells is critical for elucidating their role in tissues and understanding how abnormal gene expression contributes to tumor progression.
Recent research has shown that there are a large number of gene mutations in the cellular genome of HCC, and the mutation pattern is related to its epidemiological background (Kan et al., 2013). Current studies suggest that the pathogenesis of HCC is related to a certain degree of aberrant activation of telomerase transcription, somatic mutation, and integration of hepatitis B virus (HBV) DNA. Critical pathways and key oncogenes are also involved in tumorigenesis. The intricate pathogenesis leads to differences in HCC pathological types and therapeutic susceptibility, resulting in significantly different prognoses between patients (Shibata and Aburatani, 2014). This inter-individual complexity of genome and pathology makes HCC highly heterogeneous and poses great challenges for personalized gene-based therapy of HCC patients.
Previous oncology studies have largely relied on sequencing results from large tissue samples consisting of millions of cells, therefore only focused on the average expression of specific transcripts within the tissue. This type of study is influenced by the expression level of each gene as well as the origin of different sample tissues. In recent years, single-cell sequencing based on next generation sequencing (NGS) has developed rapidly. The first single-cell mRNA sequencing analysis was performed in 2009 (Tang et al., 2009), the first single-cell DNA sequencing analysis in human cancerous cells was performed in 2011 (Navin et al., 2011), and the first single-cell exome sequencing analysis was performed in 2012 (Hou et al., 2012). Past research has suggested that single cells with the same phenotype were regarded as the basic functional unit of a tissue or organ, but complex heterogeneity between cells was observed by deep sequencing of single cells. Single-cell sequencing is currently most widely used for single-cell transcriptomic sequencing technology (single-cell RNA-seq, scRNA-seq), and has been widely used in research and some clinical settings (Hwang et al., 2018). Sample preparation, single-cell isolation, sequencing library construction, and data analysis are key steps in the entire single-cell sequencing workflow.
Compared to conventional sequencing, a unique feature of single-cell sequencing is the requirement to obtain single cells in a great condition. To avoid the effects of hypoxia and ischemia on tissue and cellular status after separation from the organism, common single-cell sequencing techniques initially require the use of freshly isolated tissue for cell dissociation. However, in practice, due to the restrictions of the required condition or professional equipment, it may be arduous to process samples in real-time. In addition, processing multiple samples after collecting them at different times can lead to batch effects, which can interfere with sequencing results (Lafzi et al., 2018). The above-mentioned requirements for samples present certain challenges for single-cell studies.
Traditional tissue preservation methods, such as ultra-low temperature freezing (-80°C or liquid nitrogen storage), can lead to intracellular crystallization and rupture of cell membranes, which can affect subsequent single-cell sequencing. However, the integrity of RNA molecules from cell lines or primary tissues can be maintained for several months when stored at -80°C or in liquid nitrogen with cryoprotectants (dimethyl sulfoxide or serum-free cell cryopreservation medium) (Guillaumet-Adkins et al., 2017). Furthermore, combining methanol fixation with droplet-based single-cell approach, isolated cells can be stabilized for several weeks while guaranteeing the accurateness of later single-cell sequencing data (Alles et al., 2017). The sample preservation methods described above allow the location and timing of sampling to be independent of following processing procedures, enabling the successful implementation of complex experimental designs and expanding the range of samples that can be sequenced.
Additionally, in the clinic, many of the tissue samples are stored in Formalin-Fixed and Paraffin-Embedded (FFPE) blocks. For several reasons, including variations in fixation and tissue processing, FFPE samples may harbor greater variation in quality than frozen samples, and researchers usually do not have much control of those histologically collected samples (Gaffney et al., 2018). The above factors complicate the study of FFPE blocks at the molecular level, hindering their application in single-cell sequencing. However, due to its easy long-term storage characteristics, many precious clinical samples are stored in FFPE blocks. It is expected that researchers will find a suitable solution to increase the utilization of FFPE blocks in the field of single-cell sequencing.
After more than a decade of development, the SCS sequencing process has been continuously updated and revised. The single-cell isolation technique is the first step in obtaining genetic information from a single cell, and its progress has greatly expanded the research in the field of single-cell sequencing. Among them, limiting dilution analysis (LDA) is the most simple but cumbersome method (Kalisky and Quake, 2011). Usually, only about one-third of the prepared well information can be obtained in the well plate when diluted to a concentration of 0.5 cells per well, which is inefficient. Capillary extraction of cells under microscopic manipulation is only used for embryology experiments or specific disease research, which is time-consuming and low-throughput (Brehm-Stecher and Johnson, 2004); Magnetic-positive cell sorting (MACS) or flow-activated cell sorting (FACS) has become the most common strategy for isolation of highly purified single cells (Julius et al., 1972). This method begins by labeling cells with monoclonal antibodies that recognize specific surface markers and classify different cell subpopulations. Alternatively, for unstained populations, negative selection can also be used to obtain specific cell populations. Laser capture microdissection (LCM) techniques use a laser system to separate cells from a solid sample assisted by a computer system and can preserve the original spatial information (Nichterwitz et al., 2016). However, the disadvantage is that there is no guarantee that the obtained sequencing information is from a single cell.
Microfluidics technology enables high-throughput sequencing research and has become the most widely used method for SCS research at this stage. It is popular for its low sample consumption, low analytical costs, and ability to achieve precise fluidic control (Whitesides, 2006). There are two main types of microfluidics technology, one is the microfluidic-based microplate (chip) technology. The commercial platform Fluidigm C1 is widely used to provide automated single-cell lysis, RNA extraction, and cDNA synthesis for up to 800 parallel cells on a single chip. Compared to microtube-based techniques, this platform provides lower errors and less deviations. However, its main disadvantages include the low number of cells captured (<1000) and the limitation of the size of the cells analyzed. Another widely used single-cell isolation technique is droplet-based microfluidics, which allows oil droplets to be monodispersed in a continuous aqueous phase (Thorsen et al., 2001; Utada et al., 2005). Compared to standard microfluidic-based chip technology, this system requires a smaller volume, enabling the manipulation and screening of thousands or even millions of cells at a much lower cost. The commercial Chromium system from 10× Genomics provides high-throughput analysis of the 3′ ends of single-cell RNA with high capture efficiency, making it the most widely used method for single-cell sequencing today. A summary of each single-cell isolation method is presented in Table 1.
The steps required to construct a scRNA-seq library mainly include cell lysis, first-strand reverse transcription, second-strand synthesis, and cDNA amplification.
Cell lysis is usually performed in hypotonic buffer, followed by poly(A)+ selection using poly (dT) primers to capture messenger RNA (mRNA) in the cells. However, due to the principle of Poisson distribution, only 10%–20% of transcripts can be captured for reverse transcription at this step (Islam et al., 2014). Low mRNA capture-efficiency is a great challenge in the current scRNA-seq technology, requiring researchers to find and develop efficient cell lysis strategies.
cDNA preparation includes two parts: first-strand reverse transcription and second-strand synthesis. Currently, reverse transcriptases with low RNase H activity and high thermostability are often used for first-strand synthesis (Gerard et al., 2002; Arezi and Hogrefe, 2009). The second-strand is subsequently generated using poly(A) tailing (Sasagawa et al., 2013) or through a template switching mechanism (Islam et al., 2011; Ramsköld et al., 2012). Finally, a small amount of synthetic cDNA is further amplified using conventional PCR or in vitro transcription. The in vitro transcription method (CEL-Seq) can be used to amplify linear templates (Hashimshony et al., 2012), but the whole process is time-consuming and prone to 3′ coverage bias due to the need for additional reverse transcription (Jaitin et al., 2014). Smart-seq2 (an improved version of Smart-seq) generates full length transcripts and is therefore suitable for discovering variable splicing events and allele-specific expression using single nucleotide polymorphisms (Picelli et al., 2013). Currently, Illumina platforms (e.g., HiSeq4000 a n d NextSeq500) are widely used for sequencing steps. With the continuous improvement and maturity of library construction technology, the efficiency is greatly improved. In particular, the benchtop MiSeq sequencer has a fast library construction speed, which greatly reduces the turnaround time and can generate approximately 30 million paired-end reads in a single day.
Deep sequencing analysis often requires the detection of large numbers of cells. To reduce the sequencing cost, previous methods only focus on the 5′ or 3′ end of the transcripts (Islam et al., 2011; Hashimshony et al., 2012). More recently, researchers have incorporated unique molecular identifiers (UMIs) or barcodes (random 4–8 bp sequences) in the reverse transcription step (Islam et al., 2011; Hashimshony et al., 2012; Macosko et al., 2015). Considering the presence of 105–106 mRNA molecules and >10,000 expressed genes in a single cell, at least 4-bp UMI is required for sequencing library construction. UMI can help remove PCR duplicates and barcodes (random 4-8 bp sequences) can assign reads to their original cells. Using this strategy, the accuracy can be improved. However, current UMI tag-based methods only sequence the 5′ or 3′ ends of transcripts. Therefore, they are not suitable for the use of allele-specific expression. A comparison of representative scRNA-seq library construction methods is given in Table 2.
The main challenge faced in scRNA-seq is the complex experimental design. The tissue should be preserved intact or dissociated as single-cell suspension, fixed by methanol or formaldehyde. Cryopreserved or live cells and tissue should be dissociated using trypsin, cold-active protease, or a traditional method of digestion at 37°C (Aliya et al., 2022). The viability of different cell types in the process of tissue dissociation could introduce artifacts in cellular proportions or even completely lead to the loss of certain cell types. Artifacts of stress responses, such as heat-shock response, were observed in scRNAseq datasets that involved tissue dissociation. These all alter the transcriptional profiles of the cell types. Single-nucleus RNA sequencing (snRNA-seq) is an approach that bypasses the cell dissociation step required for scRNA-seq by using detergents to release nuclei from intact cells (Andrews et al., 2022). Enzyme-free isolation of the cellular nucleus could avoid the various potential problems described above. snRNA-seq sometimes enables the identification of rare subtypes that are indistinguishable by scRNA-seq (Andrews et al., 2022). However, T, B, and NK cells are underrepresented in snRNA-seq data and is therefore not recommended for studies in immune populations (Denisenko et al., 2020; Andrews et al., 2022). Overall, scRNAseq and snRNAseq could complement each other and help provide a more accurate full picture. A comparison of those two could be helpful in terms of understanding the results from different studies and provide guidance for selecting the best approach for one’s own study (Denisenko et al., 2020).
In addition to the single-cell transcriptome, currently, the most widely used single-cell technologies are single-cell DNA sequencing (scDNA-seq) and single-cell epigenomics sequencing (scEpig-seq). A number of methods have been developed for single-cell DNA and epigenomics studies. Due to different amplification processes, the coverage, sensitivity, efficiency, and accuracy of these methods differ from each other. The characteristics and applications of the different methods are summarized in Table 3 and Table 4.
Moreover, researchers are not limited to single-omics or single-aspect studies in tumor research, but integrate multiple omics into a single cell or link different aspects of the epigenome. For example, two multitopic approaches, sn-m3C-seq (Lee et al., 2019) and scMethyl-HiC (Li et al., 2019), have described methods to obtain linked chromatin conformation and methylation data from the same single cell, using bisulfite conversion of crosslinked genomic DNA. The combination of DNA and RNA sequencing of a single cell by DNA-mRNA sequencing (DR-seq) (Dey et al., 2015) or genome and transcriptome sequencing (G&T-seq) (Macaulay et al., 2015) can reveal genomic variation between individual cells, thus explaining changes in transcript levels. Techniques to analyze the epigenome and transcriptome of the same cell have been used to reveal the regulatory role of methylation and chromatin accessibility in gene expression (Angermueller et al., 2016; Hu et al., 2016). Furthermore, single-cell triomics sequencing (scTrio-seq) has been developed to simultaneously obtain information about the genome, DNA methylome and transcriptome from a single cell (Hou et al., 2016).
In addition, spatial information is lost due to disruption of tissue samples during single-cell sequencing, Single-cell spatial transcriptomics, which combines scRNA-seq with spatial transcriptomics (ST), can help solve this problem (Longo et al., 2021). It preserves the native structure and interactions of cells within a tissue, helps to profile RNA expression in their native site, deepening our understanding of disease pathogenesis (Ramachandran et al., 2020). Of course, besides the single-cell sequencing-based approach, multiplexed single-molecule FISH-based approaches in spatial transcriptomics, such as Multiplex Error Robust Fluorescent In Situ Hybridization (MERFISH) (Chen et al., 2015) and Sequential FISH+ (seqFISH+) (Eng et al., 2019), also provide important information at the single cell resolution while preserving the spatial information.
In recent years, research utilizing SCS technology have exploded due to its ability to process a large number of samples and identify unknown cell types compared to traditional sequencing techniques (Proserpio and Lönnberg, 2016). Furthermore, pseudo time analysis via SCS visualization can explore possible cellular origins and delineate cell lineage trajectories in detail (Pijuan-Sala et al., 2019), and SCS has been widely used to explore the mechanism of tumorigenesis and metastasis, thereby improving the diagnosis and treatment of cancer (Navin et al., 2011).
Single-cell sequencing was primarily used in embryology and developmental research in the early days. Some scholars have used scRNA-seq to measure the full-length transcriptome of thousands of mouse hepatocytes, and used single-molecule fluorescence in situ hybridization (smFISH) to label specifically expressed genes to infer the specific distribution coordinates of cells in the hepatic lobules. Finally, a high spatial resolution zonal map of liver genes was obtained and significant regional expression was found in approximately 50% of liver genes (Halpern et al., 2017). Liver is the immunologically privileged organ of the human body. Some scholars selected liver samples from liver transplant donors and conducted a study on liver-resident immune cells (LrIC) after screening by CD45+ cells, which revealed the distribution rules and specific expression genes of T/NK cells, B cells, monocytes/macrophages (Zhao et al., 2020). In 2018, Toronto Hospital mapped the transcriptional profile of the human liver microenvironment through the transcriptional profiles of 8,444 parenchymal and non-parenchymal cells isolated from fresh tissues from 5 human livers, and for the first time described distinct macrophage populations in the human liver and identified their unique functional pathways (MacParland et al., 2018). In addition, some scholars explained the specific mechanism of the liver in adapting the immune cell network to maintain the body’s immune barrier through SCS (Stamataki and Swadling, 2020). In 2021, a study summarizes, integrates, and analyzes five scRNA-seq datasets and provides an interactive Open Access online cell browser for easy access to gene expression data from 28 healthy human livers (Brancale and Vilarinho, 2021). It provides highly valuable information for further insight into the transcriptomic architecture and stability of human liver in physiological conditions. A systematic comparison of matched scRNA-seq and snRNA-seq has provided high-resolution maps of parenchymal cell populations in healthy human livers and detected rare subtypes of cholangiocytes and mesenchymal cells (Andrews et al., 2022). In studies of human liver development, scRNA-seq analysis of human fetal and adult livers has described the developmental trajectories of the different cell types that make up the human liver (Wesley et al., 2022). Analysis of the structure and spatial location of the normal liver contributes to the study of the pathophysiology of liver diseases, especially liver cancer.
The existence of intratumor heterogeneity (ITH) was first described in the 1800s by the pathologist Rudolf Virchow, who observed microscopic inconsistencies in tumor cell morphology (Brown and Fee, 2006). Tumor heterogeneity, proposed by Fidler in 1977, is an important feature of malignancy (Fidler and Kripke, 1977). Tumor heterogeneity may be manifested by differences in clinicopathological type and degree of differentiation; Differences in the genome, transcriptome, and epigenome on a molecular basis, Differences in tumor cell aggressiveness, growth rate, and immune evasion in each patient; And differences in morbidity, mortality, and treatment sensitivity by country, ethnicity, race, gender, etc. All above factors lead to enormous challenges in the lengthy treatment process of tumors. HCC is one of the most heterogeneous tumors, and t traditional histology-based genome or transcriptome sequencing is difficult to elucidate the tumor’s genetic material alterations (Lu et al., 2016). Compared with traditional sequencing technologies, the most significant advantage of single-cell sequencing technology is the analysis of intercellular heterogeneity. We summarize the major single-cell hepatocellular carcinoma studies in recent years, as shown in Table 5.
TABLE 5. Overview of recent studies on the tumor microenvironment of hepatocellular carcinoma at the single-cell level.
Currently, the most widely accepted genetic theory is that tumorigenesis arises from the accumulation of somatic mutations, that is, tumors are formed by abnormal evolutionary accumulation of somatic mutations (McGranahan and Swanton, 2017). Copy-number variant (CNV) at the single-cell level are able to distinguish malignant from non-malignant cells, enabling single-cell analysis that is not possible with bulk-seq. There is a high degree of genetic heterogeneity within and between different tumor cell subsets, and in some tumors there are significant differences between primary tumor cell subsets and metastatic tumor cell subsets (Park et al., 2010). Tumor cell CNVs caused by genomic instability are a major feature of early tumor heterogeneity. Some scholars have explored how CNVs help tumor cells evolve in HCC and revealed a new biphasic copy number evolution model for HCC(100). Single-cell genome sequencing revealed distinct patterns of HBV-related HCC clonal evolution, and found that specific HCC tumor cells could be of monoclonal or polyclonal origin; and found that polyclonal tumors had a typical fused multinodular morphology and are the class of tumors with the highest intratumoral heterogeneity (Duan et al., 2018).
Intratumoral heterogeneity (ITH) is the main reason for the failure of targeted therapy and immunotherapy in HCC. Perturbations and Darwinian evolution among tumor cell sub-clonal populations drive the continuous evolution of newly generated tumor cells on a pre-existing genetic background (Saunders et al., 2012). However, in addition to tumor cell own mutations (intrinsic drivers), some nongenetic factors (tumor microenvironment, TME) also significantly increase tumor cell variability, resulting in a complex and evolving tumor cell population. Some scholars integrated RNA-seq, DNA sequencing, TCR-seq, and SNP array data from multiple regions of liver cancer samples to map the spatiotemporal interactions between cancer and immune cells. How these interactions reflect intra-tumor heterogeneity was investigated by correlating regional neoepitopes and viral antigen load with regional adaptive immune responses. Researchers have found that correlating different region-specific expression, viral antigen load, and regional adaptive immune responses can reflect the causes of ITH(94). The above findings suggest that the intrinsic genetic variation of tumor cells is the main driving force of heterogeneity, and the extrinsic microenvironment acts together to promote the increase of tumor heterogeneity, which ultimately leads to the failure of targeted therapy and immunotherapy and tumor progression of HCC.
The spatial heterogeneity of tumors is often thought to result from branched clonal evolution driven by random mutations that accumulate in different regions during solid tumor development (Davis et al., 2017). The spatial heterogeneity of tumors is reflected in that tumor cells at the tumor margin exhibit high invasive and metastatic characteristics, while cells within the tumor tissue maximize self-proliferation by promoting angiogenesis and increasing metabolism. Multi-omics fusion studies have found that the genome, transcriptome, proteome, and metabolome of HCC take the partial area in the microenvironment as the unit, and there is great heterogeneity in different areas (Guo et al., 2022).
Although single-cell transcriptomics can be finely divided into different cell types for analysis, the isolation of single cells destroys information about their spatial localization in natural tissues and their proximity to each other, which will lose the original spatial information and fail to reveal local networks of in situ intercellular communication. Recently, scRNA-seq combined with Spatial Transcriptomics (ST) has provided a new solution (Longo et al., 2021). The Fudan Zhongshan Cohort Study sequenced 97 paired samples using scRNA-seq and ST and found that the formation of metastatic microenvironment was mainly due to the immunosuppressive effect of MRC1+ CCL18+ M2-like macrophages, resulting in significant spatial reprogramming in the liver (Wu et al., 2022). Analysis of the spatial characteristics of the tumor microenvironment (TME) in primary liver cancer revealed that the envelope of some tumors affects the continuity of cell clusters at spatial locations within the tumor, resulting in tumor cell transcriptome diversity and suppression of immune cell infiltration in the microenvironment. And the study found that at the boundary between tumor and peripheral tissue, the bidirectional interaction of ligand-receptor help to maintain the intratumoral structure and the spatial distribution of PROM1+ and CD47+ CSCs; this process is associated with TME remodeling and tumor metastasis (Wu et al., 2021). The above studies suggest that HCC spatial heterogeneity significantly affects tumor progression, leading to regional imbalance of immune cells and consequently to remodeling of the tumor microenvironment in HCC.
Local area spread or distant metastasis of tumor is the main reason for the poor prognosis of cancer patients. The formation and occurrence mechanism of tumor spread and metastasis have not been fully revealed, which greatly limits the possibility of prolonging the survival time of tumor patients. However, the discovery of circulating tumor cells (CTCs), which lead to distant dissemination of tumors, and cancer stem cells (CSCs), which are required to maintain tumor growth, has revealed a possible mechanism for the metastatic cascade response. For this reason, CSC and CTC are becoming a hot topic of research in the field of hepatocellular carcinoma. It has been found that the intratumoral molecular heterogeneity of HCC is partly attributable to the presence of CSCs, and that CSCs at the single-cell level are phenotypically, functionally, and transcriptomically heterogeneous (Zheng et al., 2018b). In addition, some scholars have found that CD24+/CD44+- EPCAM+ cell subsets have specific gene expression profiles, and these stemness-related cell subclones in HCC enable tumor cells to acquire great genetic richness, leading to the failure of HCC targeted therapy (Ho et al., 2019). In addition, single-cell analysis of CTCs from different sources in HCC patients found that chemokine CCL5 is an important mediator of CTC immune escape (Sun et al., 2021b). Overexpression of CCL5 in CTCs is transcriptionally regulated by p38-MAX signaling, which recruits Tregs to facilitate immune escape and metastatic seeding of CTCs (Sun et al., 2021b).
Immune heterogeneity is an important aspect of tumor heterogeneity and is associated with drug resistance and immunotherapy. As shown in Figure 1, scRNA-seq confirmed that tumor tissue contains a complex immune component, including Innate immune cells such as dendritic cells (DC), immature dendritic cells (iDC), activated dendritic cells (aDC), eosinophils and neutrophils, mast cells, macrophages, natural killer cells (NK; NKCD56- cells, NKCD56+ cells); Adaptive immune cells such as T helper cells Th1 and Th2, regulatory T cells (Treg), CD8+ T cells, central memory T cell (Tcm), memory effector T cell (Tem), T follicular helper cells (Tfh), γδ T cell, etc. The above-mentioned immune cells play their unique roles together in the microenvironment and constitute a complex and variable tumor immune microenvironment (TIME).
FIGURE 1. Immune cells and their markers isolated and identified in the immune microenvironment of HCC tumors.
TIME plays a very important role in tumor heterogeneity and tumor progression (Wei et al., 2021). On the basis of the accumulation of random mutations in tumor cells during tumor development, there are differences in the immune effects of different regions of the tumor caused by TIME, and the evolution of tumor cell subclonal populations driven by this process is believed to be the main cause of the formation of spatial heterogeneity of HCC tumors (Lloyd et al., 2016). It is the selective role of the tumor microenvironment of liver cancer that leads to the continuous evolution of tumor cells toward the direction of optimal phenotypic characteristics, that is, the survival of a large number of well-adapted tumor cells (Lee et al., 2011). It has been proposed that the rate of tumor progression depends on a complex interaction between genetic and environmental factors (Wallace et al., 2015).
For the study of the immune microenvironment in HCC, SCS allows for more precise targeting of specific immune cell subpopulations. In 2017, The Center for Frontier Innovation in Biomedicine at Peking University selected T cells in HCC patients for deep single cell RNA sequencing, identified 11 T cell subsets based on their molecular and functional properties and delineated their developmental trajectories, and the genes characteristics of each subset were finally identified; And found that layilin was upregulated in activated CD8+ T cells and Tregs, and inhibited the function of CD8+ T cells in vitro (Zheng et al., 2017). In 2019, the same research team performed Single-cell sequencing of CD45+ immune cells selected from HCC tumors, liver lymph nodes (LN), blood and ascites, compared the two methods of SMART-seq and 10x Genomics, revealed that LAMP3+ DCs were mature DCs from tumor to local LN and play a major role in T-cell activation; different macrophage subpopulations exist in tumors, among which tumor-associated macrophages (TAM) are associated with the survival prognosis of HCC patients (Zhang et al., 2019b). Single-cell level studies have been conducted on HCC mucosa associated invariant T (MAIT) cells and found that MAIT cells were significantly enriched in the HCC microenvironment with upregulated expression of suppressor molecules such as PD-1, CTLA-4, and TIM-3, which correlated with poor clinical outcomes (Duan et al., 2019). Studies have found that TCR repertoires within a single tumor are low in similarity, and tumor-infiltrating lymphocyte (TIL) subsets differ between different regions of the same tumor; I Furthermore, correlation analysis showed that TIL diversity was significantly correlated with the expression of immunoreactive genes (Shi et al., 2017). According to the number and status of immune cells in the immune microenvironment, researchers divided HCC into three distinct HCC subtypes: immune-activated, immune-deficient, and immune-excluded (Zhang et al., 2019a).
In addition to acting on tumor cells, different immune cells also play an interactive role in TIME. Hong Kong scholars found that in HBV-HCC, TAM suppress tumor T-cell infiltration and regulate the immunosuppressive environment through TIGIT-NECTIN2 interactions (Ho et al., 2021). A single-cell study of early-stage recurrent HCC found that the number of Tregs decreased in recurrent foci, dendritic cells (DC) and intratumorally infiltrating CD8+ T cells increased; T cells in recurrent tumors overexpressed KLRB1(CD161) and exhibited a naive hypocytotoxic state (Sun et al., 2021a). Exploring the mechanisms of immune evasion associated with tumor recurrence provides deeper insights into the treatment of HCC tumor recurrence. In addition, some scholars sorted PD-1highCD4+CD8+ T cells by flow cytometry for single-cell sequencing, found the presence of 11 clustered CD4/CD8 double positive T cell (DPT) subpopulations with different cytotoxicity, depletion and activation scores, and which were enriched in the tumor marginal zone; The above DPT subsets are co-expressed with PD-1/HLA-DR/ICOS/CD45RO and affect the immune status of the microenvironment (Zheng et al., 2020). The above studies show that SCS technology (mainly scRNA-seq) can be used to discover the role of immune cells on tumor cells and the interaction between immune cells in TIME.
In the era of molecularly targeted tumor therapy, the identification of predictive biomarkers is critical to the successful implementation of personalized medicine. However, tumor heterogeneity can be one of the major challenges of precision medicine. SCS can perform sequencing analysis and subtype classification of single cells, providing a favorable way to resolve tumor heterogeneity and better define immune microenvironment types, and hopefully become the theoretical basis for a new era of precision medicine. Overall, the prospects of SCS for tumor diagnosis, targeted therapy, and prognosis prediction are bright. In the near future, advances in SCS will undoubtedly improve our understanding of tumor biological characteristics, help us find potential therapeutic targets for patients, and then achieve precise tumor treatment.
The datasets presented in this study can be found in online repositories. The names of the repository/repositories and accession number(s) can be found below: The transcriptome data are available in the Sequence Read Archive (https://www.ncbi.nlm.nih.gov/sra) at NCBI, with the BioProject ID: PRJNA859020 and SRA Accession Number: SAMN29766694-29766709.
RQ and HZ collected the related papers and was major contributors in writing the manuscript. QH and FL generated the data and prepared figures. YL and HZ(6th author) initiated the study and revised the manuscript. All authors read and approved the final manuscript.
This study was supported by the Shanxi Science and Technology Department (Grant No: 201903D421026, 201901D111404, 201901D211547, 201901D111408), the Shanxi Health Commission (Grant No: 2019059), Shanxi Scholarship Council of China (Grant No: 2021–165), Shanxi Educational Department (Grant No: 2021Y359), and Shanxi ‘136’ Leading Clinical Key Specialty (Grant No: 2019XY002).
The authors declare that the research was conducted in the absence of any commercial or financial relationships that could be construed as a potential conflict of interest.
All claims expressed in this article are solely those of the authors and do not necessarily represent those of their affiliated organizations, or those of the publisher, the editors and the reviewers. Any product that may be evaluated in this article, or claim that may be made by its manufacturer, is not guaranteed or endorsed by the publisher.
Aliya, S., Lee, H., Alhammadi, M., Umapathi, R., and Huh, Y. S. (2022). An Overview on single-cell technology for hepatocellular carcinoma diagnosis. Int. J. Mol. Sci. 23 (3), 1402. doi:10.3390/ijms23031402
Alles, J., Karaiskos, N., Praktiknjo, S. D., Grosswendt, S., Wahle, P., Ruffault, P. L., et al. (2017). Cell fixation and preservation for droplet-based single-cell transcriptomics. BMC Biol. 15 (1), 44. doi:10.1186/s12915-017-0383-5
Andrews, T. S., Atif, J., Liu, J. C., Perciani, C. T., Ma, X. Z., Thoeni, C., et al. (2022). Single‐cell, single-nucleus, and spatial RNA sequencing of the human liver identifies cholangiocyte and mesenchymal heterogeneity. Hepatol. Commun. 6 (4), 821–840. doi:10.1002/hep4.1854
Angermueller, C., Clark, S. J., Lee, H. J., Macaulay, I. C., Teng, M. J., Hu, T. X., et al. (2016). Parallel single-cell sequencing links transcriptional and epigenetic heterogeneity. Nat. Methods 13 (3), 229–232. doi:10.1038/nmeth.3728
Arezi, B., and Hogrefe, H. (2009). Novel mutations in moloney murine leukemia virus reverse transcriptase increase thermostability through tighter binding to template-primer. Nucleic Acids Res. 37 (2), 473–481. doi:10.1093/nar/gkn952
Arneson, N., Hughes, S., Houlston, R., and Done, S. (2008). Whole-genome amplification by degenerate oligonucleotide primed pcr (Dop-Pcr). Cold Spring Harb. Protoc. 2008, pdb.prot4919. doi:10.1101/pdb.prot4919
Brancale, J., and Vilarinho, S. (2021). A single cell gene expression atlas of 28 human livers. J. Hepatology 75 (1), 219–220. doi:10.1016/j.jhep.2021.03.005
Brehm-Stecher, B. F., and Johnson, E. A. (2004). Single-cell microbiology: Tools, technologies, and applications. Microbiol. Mol. Biol. Rev. 68 (3), 538–559. table of contents. doi:10.1128/mmbr.68.3.538-559.2004
Brown, T. M., and Fee, E. (2006). Rudolf carl Virchow. Am. J. Public Health 96 (12), 2104–2105. doi:10.2105/ajph.2005.078436
Buenrostro, J. D., Wu, B., Litzenburger, U. M., Ruff, D., Gonzales, M. L., Snyder, M. P., et al. (2015). Single-cell chromatin accessibility reveals principles of regulatory variation. Nature 523 (7561), 486–490. doi:10.1038/nature14590
Chen, C., Xing, D., Tan, L., Li, H., Zhou, G., Huang, L., et al. (2017). Single-cell whole-genome analyses by linear amplification via Transposon insertion (lianti). Science 356 (6334), 189–194. doi:10.1126/science.aak9787
Chen, K. H., Boettiger, A. N., Moffitt, J. R., Wang, S., and Zhuang, X. (2015). Spatially resolved, highly multiplexed RNA profiling in single cells. Science 348 (6233), aaa6090. doi:10.1126/science.aaa6090
Davis, A., Gao, R., and Navin, N. (2017). Tumor evolution: Linear, branching, neutral or punctuated? Biochimica Biophysica Acta (BBA) - Rev. Cancer 1867 (2), 151–161. doi:10.1016/j.bbcan.2017.01.003
Denisenko, E., Guo, B. B., Jones, M., Hou, R., de Kock, L., Lassmann, T., et al. (2020). Systematic assessment of tissue dissociation and storage biases in single-cell and single-nucleus rna-seq workflows. Genome Biol. 21 (1), 130. doi:10.1186/s13059-020-02048-6
Dey, S. S., Kester, L., Spanjaard, B., Bienko, M., and van Oudenaarden, A. (2015). Integrated genome and transcriptome sequencing of the same cell. Nat. Biotechnol. 33 (3), 285–289. doi:10.1038/nbt.3129
Dong, X., Wang, F., Liu, C., Ling, J., Jia, X., Shen, F., et al. (2021). Single-cell analysis reveals the intra-tumor heterogeneity and identifies mlxipl as a biomarker in the cellular trajectory of hepatocellular carcinoma. Cell. Death Discov. 7 (1), 14. doi:10.1038/s41420-021-00403-5
Duan, M., Goswami, S., Shi, J. Y., Wu, L. J., Wang, X. Y., Ma, J. Q., et al. (2019). Activated and exhausted mait cells foster disease progression and indicate poor outcome in hepatocellular carcinoma. Clin. Cancer Res. 25 (11), 3304–3316. doi:10.1158/1078-0432.Ccr-18-3040
Duan, M., Hao, J., Cui, S., Worthley, D. L., Zhang, S., Wang, Z., et al. (2018). Diverse modes of clonal evolution in hbv-related hepatocellular carcinoma revealed by single-cell genome sequencing. Cell. Res. 28 (3), 359–373. doi:10.1038/cr.2018.11
Eldar, A., and Elowitz, M. B. (2010). Functional roles for noise in genetic circuits. Nature 467 (7312), 167–173. doi:10.1038/nature09326
Eng, C. L., Lawson, M., Zhu, Q., Dries, R., Koulena, N., Takei, Y., et al. (2019). Transcriptome-scale super-resolved imaging in tissues by RNA seqFISH+. Nature 568 (7751), 235–239. doi:10.1038/s41586-019-1049-y
Fan, H. C., Fu, G. K., and Fodor, S. P. (2015). Combinatorial labeling of single cells for gene expression cytometry. Science 347 (6222), 1258367. doi:10.1126/science.1258367
Fidler, I. J., and Kripke, M. L. (1977). Metastasis results from preexisting variant cells within a malignant tumor. Science 197 (4306), 893–895. doi:10.1126/science.887927
Gaffney, E. F., Riegman, P. H., Grizzle, W. E., and Watson, P. H. (2018). Factors that drive the increasing use of ffpe tissue in basic and translational cancer research. Biotech. Histochem. 93 (5), 373–386. doi:10.1080/10520295.2018.1446101
Gerard, G. F., Potter, R. J., Smith, M. D., Rosenthal, K., Dhariwal, G., Lee, J., et al. (2002). The role of template-primer in protection of reverse transcriptase from thermal inactivation. Nucleic Acids Res. 30 (14), 3118–3129. doi:10.1093/nar/gkf417
Gierahn, T. M., Wadsworth, M. H., Hughes, T. K., Bryson, B. D., Butler, A., Satija, R., et al. (2017). Seq-Well: Portable, low-cost rna sequencing of single cells at high throughput. Nat. Methods 14 (4), 395–398. doi:10.1038/nmeth.4179
Guillaumet-Adkins, A., Rodríguez-Esteban, G., Mereu, E., Mendez-Lago, M., Jaitin, D. A., Villanueva, A., et al. (2017). Single-cell transcriptome conservation in cryopreserved cells and tissues. Genome Biol. 18 (1), 45. doi:10.1186/s13059-017-1171-9
Guo, F., Li, L., Li, J., Wu, X., Hu, B., Zhu, P., et al. (2017). Single-cell multi-omics sequencing of mouse early embryos and embryonic stem cells. Cell. Res. 27 (8), 967–988. doi:10.1038/cr.2017.82
Guo, H., Zhu, P., Guo, F., Li, X., Wu, X., Fan, X., et al. (2015). Profiling DNA methylome landscapes of mammalian cells with single-cell reduced-representation bisulfite sequencing. Nat. Protoc. 10 (5), 645–659. doi:10.1038/nprot.2015.039
Guo, J., and Cairns, B. R. (2019). Isolation and enrichment of spermatogonial stem cells from human testis tissues. Curr. Protoc. Stem Cell. Biol. 49 (1), e77. doi:10.1002/cpsc.77
Guo, L., Yi, X., Chen, L., Zhang, T., Guo, H., Chen, Z., et al. (2022). Single-cell DNA sequencing reveals punctuated and gradual clonal evolution in hepatocellular carcinoma. Gastroenterology 162 (1), 238–252. doi:10.1053/j.gastro.2021.08.052
Halpern, K. B., Shenhav, R., Matcovitch-Natan, O., Tóth, B., Lemze, D., Golan, M., et al. (2017). Single-cell spatial reconstruction reveals global division of labour in the mammalian liver. Nature 542 (7641), 352–356. doi:10.1038/nature21065
Han, L., Wu, H. J., Zhu, H., Kim, K. Y., Marjani, S. L., Riester, M., et al. (2017). Bisulfite-independent analysis of cpg island methylation enables genome-scale stratification of single cells. Nucleic Acids Res. 45 (10), gkx026. doi:10.1093/nar/gkx026
Harada, A., Maehara, K., Handa, T., Arimura, Y., Nogami, J., Hayashi-Takanaka, Y., et al. (2019). A chromatin integration labelling method enables epigenomic profiling with lower input. Nat. Cell. Biol. 21 (2), 287–296. doi:10.1038/s41556-018-0248-3
Hashimshony, T., Senderovich, N., Avital, G., Klochendler, A., de Leeuw, Y., Anavy, L., et al. (2016). Cel-Seq2: Sensitive highly-multiplexed single-cell rna-seq. Genome Biol. 17, 77. doi:10.1186/s13059-016-0938-8
Hashimshony, T., Wagner, F., Sher, N., and Yanai, I. (2012). Cel-Seq: Single-Cell rna-seq by multiplexed linear amplification. Cell. Rep. 2 (3), 666–673. doi:10.1016/j.celrep.2012.08.003
Ho, D. W., Tsui, Y. M., Chan, L. K., Sze, K. M., Zhang, X., Cheu, J. W., et al. (2021). Single-cell rna sequencing shows the immunosuppressive landscape and tumor heterogeneity of hbv-associated hepatocellular carcinoma. Nat. Commun. 12 (1), 3684. doi:10.1038/s41467-021-24010-1
Ho, D. W., Tsui, Y. M., Sze, K. M., Chan, L. K., Cheung, T. T., Lee, E., et al. (2019). Single-cell transcriptomics reveals the landscape of intra-tumoral heterogeneity and stemness-related subpopulations in liver cancer. Cancer Lett. 459, 176–185. doi:10.1016/j.canlet.2019.06.002
Hou, Y., Guo, H., Cao, C., Li, X., Hu, B., Zhu, P., et al. (2016). Single-cell triple omics sequencing reveals genetic, epigenetic, and transcriptomic heterogeneity in hepatocellular carcinomas. Cell. Res. 26 (3), 304–319. doi:10.1038/cr.2016.23
Hou, Y., Song, L., Zhu, P., Zhang, B., Tao, Y., Xu, X., et al. (2012). Single-cell exome sequencing and monoclonal evolution of a jak2-negative myeloproliferative neoplasm. Cell. 148 (5), 873–885. doi:10.1016/j.cell.2012.02.028
Hu, Y., Huang, K., An, Q., Du, G., Hu, G., Xue, J., et al. (2016). Simultaneous profiling of transcriptome and DNA methylome from a single cell. Genome Biol. 17, 88. doi:10.1186/s13059-016-0950-z
Huang, L., Ma, F., Chapman, A., Lu, S., and Xie, X. S. (2015). Single-cell whole-genome amplification and sequencing: Methodology and applications. Annu. Rev. Genom. Hum. Genet. 16, 79–102. doi:10.1146/annurev-genom-090413-025352
Huang, S. (2009). Non-genetic heterogeneity of cells in development: More than just noise. Development 136 (23), 3853–3862. doi:10.1242/dev.035139
Hwang, B., Lee, J. H., and Bang, D. (2018). Single-cell rna sequencing technologies and bioinformatics pipelines. Exp. Mol. Med. 50 (8), 1–14. doi:10.1038/s12276-018-0071-8
Islam, S., Kjällquist, U., Moliner, A., Zajac, P., Fan, J. B., Lönnerberg, P., et al. (2011). Characterization of the single-cell transcriptional landscape by highly Multiplex rna-seq. Genome Res. 21 (7), 1160–1167. doi:10.1101/gr.110882.110
Islam, S., Kjällquist, U., Moliner, A., Zajac, P., Fan, J. B., Lönnerberg, P., et al. (2012). Highly multiplexed and strand-specific single-cell RNA 5′ end sequencing. Nat. Protoc. 7 (5), 813–828. doi:10.1038/nprot.2012.022
Islam, S., Zeisel, A., Joost, S., La Manno, G., Zajac, P., Kasper, M., et al. (2014). Quantitative single-cell rna-seq with unique molecular identifiers. Nat. Methods 11 (2), 163–166. doi:10.1038/nmeth.2772
Jaitin, D. A., Kenigsberg, E., Keren-Shaul, H., Elefant, N., Paul, F., Zaretsky, I., et al. (2014). Massively parallel single-cell rna-seq for marker-free decomposition of tissues into cell types. Science 343 (6172), 776–779. doi:10.1126/science.1247651
Julius, M. H., Masuda, T., and Herzenberg, L. A. (1972). Demonstration that antigen-binding cells are precursors of antibody-producing cells after purification with a fluorescence-activated cell sorter. Proc. Natl. Acad. Sci. U.S.A. 69 (7), 1934–1938. doi:10.1073/pnas.69.7.1934
Kalisky, T., and Quake, S. R. (2011). Single-cell genomics. Nat. Methods 8 (4), 311–314. doi:10.1038/nmeth0411-311
Kan, Z., Zheng, H., Liu, X., Li, S., Barber, T. D., Gong, Z., et al. (2013). Whole-genome sequencing identifies recurrent mutations in hepatocellular carcinoma. Genome Res. 23 (9), 1422–1433. doi:10.1101/gr.154492.113
Kaya-Okur, H. S., Wu, S. J., Codomo, C. A., Pledger, E. S., Bryson, T. D., Henikoff, J. G., et al. (2019). Cut&Tag for efficient epigenomic profiling of small samples and single cells. Nat. Commun. 10 (1), 1930. doi:10.1038/s41467-019-09982-5
Klein, A. M., Mazutis, L., Akartuna, I., Tallapragada, N., Veres, A., Li, V., et al. (2015). Droplet barcoding for single-cell transcriptomics applied to embryonic stem cells. Cell. 161 (5), 1187–1201. doi:10.1016/j.cell.2015.04.044
Ku, W. L., Nakamura, K., Gao, W., Cui, K., Hu, G., Tang, Q., et al. (2019). Single-cell chromatin immunocleavage sequencing (Scchic-Seq) to profile histone modification. Nat. Methods 16 (4), 323–325. doi:10.1038/s41592-019-0361-7
Lafzi, A., Moutinho, C., Picelli, S., and Heyn, H. (2018). Tutorial: Guidelines for the experimental design of single-cell rna sequencing studies. Nat. Protoc. 13 (12), 2742–2757. doi:10.1038/s41596-018-0073-y
Lee, D. S., Luo, C., Zhou, J., Chandran, S., Rivkin, A., Bartlett, A., et al. (2019). Simultaneous profiling of 3d genome structure and DNA methylation in single human cells. Nat. Methods 16 (10), 999–1006. doi:10.1038/s41592-019-0547-z
Lee, H. O., Silva, A. S., Concilio, S., Li, Y. S., Slifker, M., Gatenby, R. A., et al. (2011). Evolution of tumor invasiveness: The adaptive tumor microenvironment landscape model. Cancer Res. 71 (20), 6327–6337. doi:10.1158/0008-5472.Can-11-0304
Li, G., Liu, Y., Zhang, Y., Kubo, N., Yu, M., Fang, R., et al. (2019). Joint profiling of DNA methylation and chromatin architecture in single cells. Nat. Methods 16 (10), 991–993. doi:10.1038/s41592-019-0502-z
Li, L., and Clevers, H. (2010). Coexistence of quiescent and active adult stem cells in mammals. Science 327 (5965), 542–545. doi:10.1126/science.1180794
Lipinski, K. A., Barber, L. J., Davies, M. N., Ashenden, M., Sottoriva, A., and Gerlinger, M. (2016). Cancer evolution and the limits of predictability in precision cancer medicine. Trends Cancer 2 (1), 49–63. doi:10.1016/j.trecan.2015.11.003
Lloyd, M. C., Cunningham, J. J., Bui, M. M., Gillies, R. J., Brown, J. S., and Gatenby, R. A. (2016). Darwinian dynamics of intratumoral heterogeneity: Not solely random mutations but also variable environmental selection forces. Cancer Res. 76 (11), 3136–3144. doi:10.1158/0008-5472.Can-15-2962
Longo, S. K., Guo, M. G., Ji, A. L., and Khavari, P. A. (2021). Integrating single-cell and spatial transcriptomics to elucidate intercellular tissue dynamics. Nat. Rev. Genet. 22 (10), 627–644. doi:10.1038/s41576-021-00370-8
Losic, B., Craig, A. J., Villacorta-Martin, C., Martins-Filho, S. N., Akers, N., Chen, X., et al. (2020). Intratumoral heterogeneity and clonal evolution in liver cancer. Nat. Commun. 11 (1), 291. doi:10.1038/s41467-019-14050-z
Lu, L. C., Hsu, C. H., Hsu, C., and Cheng, A. L. (2016). Tumor heterogeneity in hepatocellular carcinoma: Facing the challenges. Liver Cancer 5 (2), 128–138. doi:10.1159/000367754
Ma, L., Hernandez, M. O., Zhao, Y., Mehta, M., Tran, B., Kelly, M., et al. (2019). Tumor cell biodiversity drives microenvironmental reprogramming in liver cancer. Cancer Cell. 36 (4), 418–e6. e6. doi:10.1016/j.ccell.2019.08.007
Macaulay, I. C., Haerty, W., Kumar, P., Li, Y. I., Hu, T. X., Teng, M. J., et al. (2015). G&T-Seq: Parallel sequencing of single-cell genomes and transcriptomes. Nat. Methods 12 (6), 519–522. doi:10.1038/nmeth.3370
Macosko, E. Z., Basu, A., Satija, R., Nemesh, J., Shekhar, K., Goldman, M., et al. (2015). Highly parallel genome-wide expression profiling of individual cells using nanoliter droplets. Cell. 161 (5), 1202–1214. doi:10.1016/j.cell.2015.05.002
MacParland, S. A., Liu, J. C., Ma, X. Z., Innes, B. T., Bartczak, A. M., Gage, B. K., et al. (2018). Single cell rna sequencing of human liver reveals distinct intrahepatic macrophage populations. Nat. Commun. 9 (1), 4383. doi:10.1038/s41467-018-06318-7
McGlynn, K. A., Petrick, J. L., and London, W. T. (2015). Global epidemiology of hepatocellular carcinoma. Clin. Liver Dis. 19 (2), 223–238. doi:10.1016/j.cld.2015.01.001
McGranahan, N., and Swanton, C. (2017). Clonal heterogeneity and tumor evolution: Past, present, and the future. Cell. 168 (4), 613–628. doi:10.1016/j.cell.2017.01.018
Navin, N., Kendall, J., Troge, J., Andrews, P., Rodgers, L., McIndoo, J., et al. (2011). Tumour evolution inferred by single-cell sequencing. Nature 472 (7341), 90–94. doi:10.1038/nature09807
Nichterwitz, S., Chen, G., Aguila Benitez, J., Yilmaz, M., Storvall, H., Cao, M., et al. (2016). Laser capture microscopy coupled with smart-seq2 for precise spatial transcriptomic profiling. Nat. Commun. 7, 12139. doi:10.1038/ncomms12139
Park, S. Y., Gönen, M., Kim, H. J., Michor, F., and Polyak, K. (2010). Cellular and genetic diversity in the progression of in situ human breast carcinomas to an invasive phenotype. J. Clin. Investig. 120 (2), 636–644. doi:10.1172/jci40724
Picelli, S., Björklund, K., Faridani, O. R., Sagasser, S., Winberg, G., and Sandberg, R. (2013). Smart-Seq2 for sensitive full-length transcriptome profiling in single cells. Nat. Methods 10 (11), 1096–1098. doi:10.1038/nmeth.2639
Picelli, S., Faridani, O. R., Björklund, A. K., Winberg, G., Sagasser, S., and Sandberg, R. (2014). Full-length rna-seq from single cells using smart-seq2. Nat. Protoc. 9 (1), 171–181. doi:10.1038/nprot.2014.006
Pijuan-Sala, B., Griffiths, J. A., Guibentif, C., Hiscock, T. W., Jawaid, W., Calero-Nieto, F. J., et al. (2019). A single-cell molecular map of mouse gastrulation and early organogenesis. Nature 566 (7745), 490–495. doi:10.1038/s41586-019-0933-9
Proserpio, V., and Lönnberg, T. (2016). Single‐cell technologies are revolutionizing the approach to rare cells. Immunol. Cell. Biol. 94 (3), 225–229. doi:10.1038/icb.2015.106
Ramachandran, P., Matchett, K. P., Dobie, R., Wilson-Kanamori, J. R., and Henderson, N. C. (2020). Single-cell technologies in hepatology: New insights into liver biology and disease pathogenesis. Nat. Rev. Gastroenterol. Hepatol. 17 (8), 457–472. doi:10.1038/s41575-020-0304-x
Ramsköld, D., Luo, S., Wang, Y. C., Li, R., Deng, Q., Faridani, O. R., et al. (2012). Full-length mrna-seq from single-cell levels of rna and individual circulating tumor cells. Nat. Biotechnol. 30 (8), 777–782. doi:10.1038/nbt.2282
Rosenberg, A. B., Roco, C. M., Muscat, R. A., Kuchina, A., Sample, P., Yao, Z., et al. (2018). Single-cell profiling of the developing mouse brain and spinal cord with split-pool barcoding. Science 360 (6385), 176–182. doi:10.1126/science.aam8999
Sasagawa, Y., Danno, H., Takada, H., Ebisawa, M., Tanaka, K., Hayashi, T., et al. (2018). Quartz-Seq2: A high-throughput single-cell rna-sequencing method that effectively uses limited sequence reads. Genome Biol. 19 (1), 29. doi:10.1186/s13059-018-1407-3
Sasagawa, Y., Nikaido, I., Hayashi, T., Danno, H., Uno, K. D., Imai, T., et al. (2013). Quartz-seq: A highly reproducible and sensitive single-cell rna sequencing method, reveals non-genetic gene-expression heterogeneity. Genome Biol. 14 (4), R31. doi:10.1186/gb-2013-14-4-r31
Saunders, N. A., Simpson, F., Thompson, E. W., Hill, M. M., Endo‐Munoz, L., Leggatt, G., et al. (2012). Role of intratumoural heterogeneity in cancer drug resistance: Molecular and clinical perspectives. EMBO Mol. Med. 4 (8), 675–684. doi:10.1002/emmm.201101131
Shalek, A. K., Satija, R., Shuga, J., Trombetta, J. J., Gennert, D., Lu, D., et al. (2014). Single-cell rna-seq reveals dynamic paracrine control of cellular variation. Nature 510 (7505), 363–369. doi:10.1038/nature13437
Shapiro, E., Biezuner, T., and Linnarsson, S. (2013). Single-cell sequencing-based technologies will revolutionize whole-organism science. Nat. Rev. Genet. 14 (9), 618–630. doi:10.1038/nrg3542
Shi, L., Zhang, Y., Feng, L., Wang, L., Rong, W., Wu, F., et al. (2017). Multi-omics study revealing the complexity and spatial heterogeneity of tumor-infiltrating lymphocytes in primary liver carcinoma. Oncotarget 8 (21), 34844–34857. doi:10.18632/oncotarget.16758
Shibata, T., and Aburatani, H. (2014). Exploration of liver cancer genomes. Nat. Rev. Gastroenterol. Hepatol. 11 (6), 340–349. doi:10.1038/nrgastro.2014.6
Smallwood, S. A., Lee, H. J., Angermueller, C., Krueger, F., Saadeh, H., Peat, J., et al. (2014). Single-cell genome-wide bisulfite sequencing for assessing epigenetic heterogeneity. Nat. Methods 11 (8), 817–820. doi:10.1038/nmeth.3035
Spits, C., Le Caignec, C., De Rycke, M., Van Haute, L., Van Steirteghem, A., Liebaers, I., et al. (2006). Whole-genome multiple displacement amplification from single cells. Nat. Protoc. 1 (4), 1965–1970. doi:10.1038/nprot.2006.326
Stamataki, Z., and Swadling, L. (2020). The liver as an immunological barrier redefined by single-cell analysis. Immunology 160 (2), 157–170. doi:10.1111/imm.13193
Sun, Y., Wu, L., Zhong, Y., Zhou, K., Hou, Y., Wang, Z., et al. (2021). Single-cell landscape of the ecosystem in early-relapse hepatocellular carcinoma. Cell. 184 (2), 404–421. e16. doi:10.1016/j.cell.2020.11.041
Sun, Y. F., Wu, L., Liu, S. P., Jiang, M. M., Hu, B., Zhou, K. Q., et al. (2021). Dissecting spatial heterogeneity and the immune-evasion mechanism of ctcs by single-cell rna-seq in hepatocellular carcinoma. Nat. Commun. 12 (1), 4091. doi:10.1038/s41467-021-24386-0
Sung, H., Ferlay, J., Siegel, R. L., Laversanne, M., Soerjomataram, I., Jemal, A., et al. (2021). Global cancer statistics 2020: Globocan estimates of incidence and mortality worldwide for 36 cancers in 185 countries. CA A Cancer J. Clin. 71 (3), 209–249. doi:10.3322/caac.21660
Tang, F., Barbacioru, C., Wang, Y., Nordman, E., Lee, C., Xu, N., et al. (2009). Mrna-seq whole-transcriptome analysis of a single cell. Nat. Methods 6 (5), 377–382. doi:10.1038/nmeth.1315
Thorsen, T., Roberts, R. W., Arnold, F. H., and Quake, S. R. (2001). Dynamic pattern formation in a vesicle-generating microfluidic device. Phys. Rev. Lett. 86 (18), 4163–4166. doi:10.1103/PhysRevLett.86.4163
Utada, A. S., Lorenceau, E., Link, D. R., Kaplan, P. D., Stone, H. A., and Weitz, D. A. (2005). Monodisperse double emulsions generated from a microcapillary device. Science 308 (5721), 537–541. doi:10.1126/science.1109164
Vitak, S. A., Torkenczy, K. A., Rosenkrantz, J. L., Fields, A. J., Christiansen, L., Wong, M. H., et al. (2017). Sequencing thousands of single-cell genomes with combinatorial indexing. Nat. Methods 14 (3), 302–308. doi:10.1038/nmeth.4154
Wallace, M. C., Preen, D., Jeffrey, G. P., and Adams, L. A. (2015). The evolving epidemiology of hepatocellular carcinoma: A global perspective. Expert Rev. Gastroenterology Hepatology 9 (6), 765–779. doi:10.1586/17474124.2015.1028363
Wei, X., Chen, Y., Jiang, X., Peng, M., Liu, Y., Mo, Y., et al. (2021). Mechanisms of vasculogenic mimicry in hypoxic tumor microenvironments. Mol. Cancer 20 (1), 7. doi:10.1186/s12943-020-01288-1
Wesley, B. T., Ross, A. D. B., Muraro, D., Miao, Z., Saxton, S., Tomaz, R. A., et al. (2022). Single-cell atlas of human liver development reveals pathways directing hepatic cell fates. Nat. Cell. Biol. doi:10.1038/s41556-022-00989-7
Whitesides, G. M. (2006). The origins and the future of microfluidics. Nature 442 (7101), 368–373. doi:10.1038/nature05058
Wu, R., Guo, W., Qiu, X., Wang, S., Sui, C., Lian, Q., et al. (2021). Comprehensive analysis of spatial architecture in primary liver cancer. Sci. Adv. 7 (51), eabg3750. doi:10.1126/sciadv.abg3750
Wu, Y., Yang, S., Ma, J., Chen, Z., Song, G., Rao, D., et al. (2022). Spatiotemporal immune landscape of colorectal cancer liver metastasis at single-cell level. Cancer Discov. 12 (1), 134–153. doi:10.1158/2159-8290.Cd-21-0316
Zhang, Q., He, Y., Luo, N., Patel, S. J., Han, Y., Gao, R., et al. (2019). Landscape and dynamics of single immune cells in hepatocellular carcinoma. Cell. 179 (4), 829–845. e20. doi:10.1016/j.cell.2019.10.003
Zhang, Q., Lou, Y., Yang, J., Wang, J., Feng, J., Zhao, Y., et al. (2019). Integrated multiomic analysis reveals comprehensive tumour heterogeneity and novel immunophenotypic classification in hepatocellular carcinomas. Gut 68 (11), 2019–2031. doi:10.1136/gutjnl-2019-318912
Zhao, J., Zhang, S., Liu, Y., He, X., Qu, M., Xu, G., et al. (2020). Single-cell rna sequencing reveals the heterogeneity of liver-resident immune cells in human. Cell. Discov. 6, 22. doi:10.1038/s41421-020-0157-z
Zheng, B., Wang, D., Qiu, X., Luo, G., Wu, T., Yang, S., et al. (2020). Trajectory and functional analysis of PD-1 high CD4 + CD8 + T cells in hepatocellular carcinoma by single‐cell cytometry and transcriptome sequencing. Adv. Sci. 7 (13), 2000224. doi:10.1002/advs.202000224
Zheng, C., Zheng, L., Yoo, J. K., Guo, H., Zhang, Y., Guo, X., et al. (2017). Landscape of infiltrating T cells in liver cancer revealed by single-cell sequencing. Cell. 169 (7), 1342–1356. e16. doi:10.1016/j.cell.2017.05.035
Zheng, H., Pomyen, Y., Hernandez, M. O., Li, C., Livak, F., Tang, W., et al. (2018). Single-cell analysis reveals cancer stem cell heterogeneity in hepatocellular carcinoma. Hepatology 68 (1), 127–140. doi:10.1002/hep.29778
Zheng, R., Qu, C., Qu, S., Zhang, H., Zeng, K., Sun, X., et al. (2018). Liver cancer incidence and mortality in China: Temporal trends and projections to 2030. Chin. J. Cancer Res. 30 (6), 571–579. doi:10.21147/j.issn.1000-9604.2018.06.01
Zong, C., Lu, S., Chapman, A. R., and Xie, X. S. (2012). Genome-wide detection of single-nucleotide and copy-number variations of a single human cell. Science 338 (6114), 1622–1626. doi:10.1126/science.1229164
PLC primary liver cancer
HCC hepatocellular carcinoma
ICC intrahepatic cholangiocarcinoma
CSC single-cell sequencing
HBV hepatitis B virus
MACS magnetic-activated cell sorting
FACS flow-activated cell sorting
LCM laser capture microdissection
scRNA-seq single-cell RNA sequencing
UMI unique molecular identifiers
PCR polymerase chain reaction
IVT in vitro transcription
scEpig-seq Single-cell epigenomics sequencing
DOP-PCR degenerate oligonucleotide–primed polymerase chain reaction
MDA multiple displacement amplification
MALBAC multiple annealing and looping-based amplification cycles
LIANTI Linear Amplification via Transposon Insertion
CNV copy number variation
SNV singlenucleotide polymorphisms
DR-seq DNA-mRNA sequencing)
G&T-seq genome and transcriptome sequencing
scTrio-seq single-cell triomics sequencing
LrIC liver-resident immune cells
ITH intra-tumor heterogeneity
ST spatial Transcriptomics
TME tumor microenvironment
CTCs circulating tumor cells
CSCs cancer stem cells
EMT mesenchymal transition
DC dendritic cells
iDC immature dendritic cells
aDC activated dendritic cells
Treg regulatory T cell
Tcm central memory T cell
Tem memory effector T cell
Tfh T follicular helper cell
TAMs tumor-associated macrophages
VEGF vascular endothelial growth factor
MLXIPL MLX interacting protein-like
Keywords: single cell sequencing, hepatocellular carcinoma, tumor heterogeneity, tumor immune microenvironment, circulating tumor cells, cancer stem cells
Citation: Qin R, Zhao H, He Q, Li F, Li Y and Zhao H (2022) Advances in single-cell sequencing technology in the field of hepatocellular carcinoma. Front. Genet. 13:996890. doi: 10.3389/fgene.2022.996890
Received: 18 July 2022; Accepted: 28 September 2022;
Published: 11 October 2022.
Edited by:
H. Steven Wiley, Pacific Northwest National Laboratory (DOE), United StatesReviewed by:
Zhibo Ma, Salk Institute for Biological Studies, United StatesCopyright © 2022 Qin, Zhao, He, Li, Li and Zhao. This is an open-access article distributed under the terms of the Creative Commons Attribution License (CC BY). The use, distribution or reproduction in other forums is permitted, provided the original author(s) and the copyright owner(s) are credited and that the original publication in this journal is cited, in accordance with accepted academic practice. No use, distribution or reproduction is permitted which does not comply with these terms.
*Correspondence: Yanjun Li, bGl5anlpc2hlbmcxMDE3QDE2My5jb20=; Haoliang Zhao, aGFvbGlhbmd6aGFvQGhvdG1haWwuY29t
†These authors contributed equally to this work and share first authorship
Disclaimer: All claims expressed in this article are solely those of the authors and do not necessarily represent those of their affiliated organizations, or those of the publisher, the editors and the reviewers. Any product that may be evaluated in this article or claim that may be made by its manufacturer is not guaranteed or endorsed by the publisher.
Research integrity at Frontiers
Learn more about the work of our research integrity team to safeguard the quality of each article we publish.