- 1Department of Breast Surgery, The First Affiliated Hospital, Nanjing Medical University, Nanjing, China
- 2Department of Clinical Laboratory, The Affiliated Wuxi Maternity and Child Health Care Hospital of Nanjing Medical University, Wuxi, China
Background: CD161 has been identified as a prognostic biomarker in many neoplasms, but its role in breast cancer (BC) has not been fully explained. We aimed to investigate the molecular mechanism and prognostic value of CD161 in BC.
Methods: CD161 expression profile was extracted from TIMER, Oncomine, UALCAN databases, and verified by the Gene Expression Omnibus (GEO) database and quantitative real-time polymerase chain reaction (qRT-PCR). The prognostic value of CD161 was assessed via GEPIA, Kaplan–Meier plotter and PrognoScan databases. The Cox regression and nomogram analyses were conducted to further validate the association between CD161 expression and survival. Gene set enrichment analysis (GSEA), Gene Ontology (GO) analysis, and KEGG pathway enrichment analysis were performed to probe the tumor-associated annotations of CD161. CIBERSORT and ssGSEA were employed to investigate the correlation between CD161 expression and immune cell infiltration in BC, and the result was verified by TIMER and TISIDB.
Results: Multiple BC cohorts showed that CD161 expression was decreased in BC, and a high CD161 expression was associated with a preferable prognosis. Therefore, we identified the combined model including CD161, age and PR status to predict the survival (C index = 0.78) of BC patients. Functional enrichment analysis indicated that CD161 and its co-expressed genes were closely related to several cancerous and immune signaling pathways, suggesting its involvement in immune response during cancer development. Moreover, immune infiltration analysis revealed that CD161 expression was correlated with immune infiltration.
Conclusion: Collectively, our findings revealed that CD161 may serve as a potential biomarker for favorable prognosis and a promising immune therapeutic target in BC.
Introduction
Breast cancer (BC) is the most common cancer in females worldwide. Despite great advances in the diagnosis and treatment, BC relapses in a considerable number of patients. BC still accounts for 15.5% of all female cancer deaths (Pondé et al., 2019; Godeau et al., 2021; Sung et al., 2021). Currently, the treatment decisions and survival outcomes for BC patients mainly depend on the clinicopathological stage and type (Werner et al., 2022). However, patients with the same tumor stage, molecular subtype, and treatment regimens may have completely different clinical outcomes (Barry et al., 2010; Zardavas et al., 2015; Liu et al., 2022). This indicates that the existing staging system is not sufficient for accurate prognosis prediction, and the typing system cannot totally depict the tumor heterogeneity. More personalized treatment strategies and prognostic biomarkers based on tumors’ intrinsic characteristics are urgently needed. Therefore, digging deeply into biological characteristics of breast tumor may help better predict clinical outcomes and develop novel therapies for BC. Tumors consist of not only neoplastic cells, but also a dynamic surrounding stroma (Olson and Joyce, 2013; Bejarano et al., 2021; Xie et al., 2021). Emerging evidence suggests that BC is characterized by a highly inflammatory tumor microenvironment (TME), which is supported by the tumor infiltrating immune cells (TILs), cytokines, and growth factors etc. (Lim et al., 2018). Crosstalk between cancer cells and TILs continually influences the occurrence, development, and metastasis of breast tumors (Flister and Bergom, 2018; Lim et al., 2018; Li et al., 2020). TILs have been proved to be related to therapeutic response and can serve as novel therapeutic targets (Stanton and Disis, 2016; Byrne et al., 2020). Previous reports have supported that a high density of TILs is an important prognostic factor to improve the survival outcomes of patients (Savas et al., 2018; Maibach et al., 2020; Paijens et al., 2021). However, one common observation from functional studies is that many subsets of TILs failed to expand or function normally in the BC microenvironment, instead contributing to the tumor progression (Guo and Deng, 2018; Yang et al., 2020). Accordingly, identifying the factors that affect the dynamic changes of TILs at the gene level is crucial for targeted BC treatment and improved prognosis outcomes. CD161, encoded by killer cell lectin-like receptor B1 (KLRB1), is a C-type lectin-related type II transmembrane protein expressed on NK cells, NKT cells and subsets of CD8+ and CD4+ T cells (Braud et al., 2022). It was identified as favorable prognostic gene in most human cancers (Braud et al., 2018; Duurland et al., 2022). CD161 can act as a costimulatory receptor to increase the response to T cell receptor (TCR) stimulation (Fergusson et al., 2014). Therefore, in oropharyngeal squamous cell cancer (OPSCC), CD4+CD161+ T cells display a stronger type 1 response to suboptimal antigen stimulation and produce more cytokines upon antigen stimulation in, resulting in a better prognosis (Welters et al., 2018; Duurland et al., 2022). In addition, CD161 binds to CLEC2D/LLT1 to inhibit NK-mediated cytotoxicity in target cells (López-Soto et al., 2017). A recent study has discovered that the knockdown of KLRB1 or antibody-mediated blockade of CD161 enhances the ability of T cells to kill tumor cells (Mathewson et al., 2021), suggesting that the CD161-LLT1 pathway may serve as a potential target of immunotherapy for glioma. However, due to the heterogeneity of BC, the tumorigenic effects and clinical significance of abnormal expression of CD161 in BC remain largely unknown. In this study, we utilized the BC RNA-seq data from The Cancer Genome Atlas (TCGA) and Gene Expression Omnibus (GEO) to compare the expression of CD161 in normal breast tissues and BC samples. Then, we verified the findings by quantitative real-time polymerase chain reaction (qRT-PCR), using paired tissue samples from our center. Next, we assessed the relationship between CD161 expression and clinicopathological parameters of BC. Furthermore, we investigated the prognostic value of CD161 for BC, and predicted BC survival using a nomogram constructed with the independent prognostic factors derived from multivariate Cox regression analysis. Besides, we conducted functional enrichment analyses to probe the tumor-associated annotations of CD161. Finally, we analyzed the relationship between CD161 expression and immune infiltration. Our research indicated that CD161 could serve as a potential prognostic biomarker and immune therapeutic target for BC.
Materials and methods
Patients and sample collection
A total of 1097 BC patients from the TCGA database were enrolled. Included were patients with (Pondé et al., 2019) a primary site at the breast; (Godeau et al., 2021) data in the Program of TCGA; (Sung et al., 2021) data in the Project of TCGA-BRCA; (Werner et al., 2022) primary tumor tissue sampled. The clinicopathological parameters and RNA-seq data of these patients were downloaded and summarized in Supplementary Table S1 for further analysis. Furthermore, the paired tissue samples of 20 patients diagnosed as invasive BC without distant metastasis were randomly obtained from the breast center of the First Affiliated Hospital with Nanjing Medical University. The clinicopathological data for these patients are presented in Supplementary Table S2. After surgical resection, the tissues were preserved in RNAlater™ solution (ThermoFisher, United States), immediately frozen in liquid nitrogen and storage at −80°C until further use for qRT-PCR. The use of human tissues in this study was approved by the Research Ethics Committee and all patients enrolled signed the informed consent.
qRT-PCR
Total RNA was extracted from the paired breast tumors and adjacent tissue samples by Olasma Kit (QIAGEN, Germany). cDNA was prepared through The HiScript® III first Strand cDNA Synthesis Kit (+gDNA wiper) (Vazyme, Nanjing, China). Next, qRT-PCR was performed using the ChamQ SYBR qPCR Master Mix (Vazyme, Nanjing, China). The primers used were as follows: human CD161 -Forward: 5′-AAACAACAGAGAGACCGGGT-3′; human CD161 -Reverse: 5′-TCCAAGGGTTGACAGTGTGAT-3′; human GAPDH-Forward: 5′-GGAGTCCACTGGCGTCTTCA-3′; human GAPDH-Reverse, 5′-GTCATGAGTCCTTCCACGATACC-3′. Primers were synthesized by the GenScript Company.
CD161 expression
The expression of CD161 in BC was investigated by TIMER (https://cistrome.shinyapps.io/timer/), Oncomine (http://www.oncomine.org), UALCAN (http://ualcan.path.uab.edu), and validated by GSE10797 from GEO database and qRT-PCR of paired tissue samples. The binary logistic model was employed to explore the association between CD161 expression and clinicopathologic features, including stage, age, T, N, M, ER, PR, menopause and anatomic neoplasm subdivision downloaded from TCGA database.
Survival analysis
The GEPIA (http://gepia.cancer-pku.cn/), Kaplan–Meier plotter (http://kmplot.com/analysis) and PrognoScan (http://dna00.bio.kyutech.ac.jp/PrognoScan/index.html) were employed to evaluate the prognostic value of CD161 in BC, based on the data about mRNA expression and survival. Overall survival (OS) referred to the time from histological diagnosis to death or the last follow-up. Disease-free survival (DFS) was the time from histological diagnosis to disease progression, death, or last follow-up. Disease-specific survival (DSS) was defined as the time from histological diagnosis to death from BC. Besides, we conducted univariate and multivariate Cox analyses of BC data from TCGA. A number of variables were assessed to identify the independent prognostic factors, including stage, age, tumor size, lymph node status, distant metastasis, CD161 expression, ER status and PR status.
Construction and validation of the nomogram
A nomogram was conducted based on the independent prognostic factors identified by the multivariate Cox analysis. Calibration plots were estimated to assess the predictive power of the nomogram, and the C-index was calculated to evaluate the discriminative ability of the nomogram.
Functional enrichment analysis
Gene Set Enrichment Analysis (GSEA) is usually used to determine the statistical significance of a priorly defined set of genes and evaluate the difference between two biological subsets (Subramanian et al., 2007). We used GSEA software to classify the pathways enriched in different BC phenotypes based on the expression level of CD161. Genomes with false discovery rate (FDR) < 0.05 were considered remarkedly enriched. cBioPortal for Cancer Genomics (http://cbioportal.org) was used to identify the genes co-expressing with CD161. Then, we performed Gene Ontology (GO) and KEGG pathway analysis to obtain the functional annotations of these co-expressed genes.
Evaluation of tumor-infiltrating immune cells
All 1097 BC patients enrolled from TCGA database were divided into high and low CD161 expression groups based on the cutoff value of 50%. CIBERSORT was utilized to compare the proportions of 22 tumor infiltration immune cells in both groups. Then, the immune infiltration levels of 24 cell types in BC were downloaded from published literature. ssGSEA analysis was performed to assess the association between CD161 expression and immune infiltration. TISIDB (http://cis.hku.hk/TISIDB/), TIMER and GEPIA were used to verify this association.
Study design
Our study was designed according to the reporting guideline checklist Tripod (Supplementary Table S3). The RNA-seq data and clinicopathological data of 1097 BC patients from TCGA database were retrospectively reviewed. Using 50% of the CD161 expression value as a cutoff point, all patients were divided into high CD161 and low CD161 expression groups for survival analysis, GSEA analysis and immune infiltration analysis. The follow-up threshold of OS, DFS and DSS were displayed in the survival curve.
Statistical analysis
All statistical analyses were conducted using R-4.1.2. In stratification analysis, the case was deleted from the data set when the stratified variable was missing. The paired sample t-test was performed to evaluate the difference in CD161 expression between paired tissues. The binary logistic model was performed by R package ISLR. The Cox regression and nomogram analyses of survival were conducted by R package survival, ggforest and RMS. The GO and KEGG analyses were accomplished by R package clusterProfiler. The CIBERSORT R script and R package GSVA were used to evaluate the immune infiltration. All the tests were two-sided, and p < 0.05 was defined as statistically significant.
Results
Expression profiles of CD161
TIMER-based analysis showed that the mRNA expression of CD161 was lower in the tissue samples of most malignant tumors, like bladder cancer (BLCA), breast invasive carcinoma (BRCA), colon adenocarcinoma (COAD) (Figure 1A). Oncomine, UALCAN and GEO databases (GSE10797) further confirmed the decreased transcriptome level of CD161 in BC tissue samples (Figures 1B–D). This difference was further validated by qRT-PCR for 20 paired tumorous and adjacent tissue samples (Figure 1E). The clinicopathological information of the 20 patients enrolled are summarized in Supplementary Table S2.
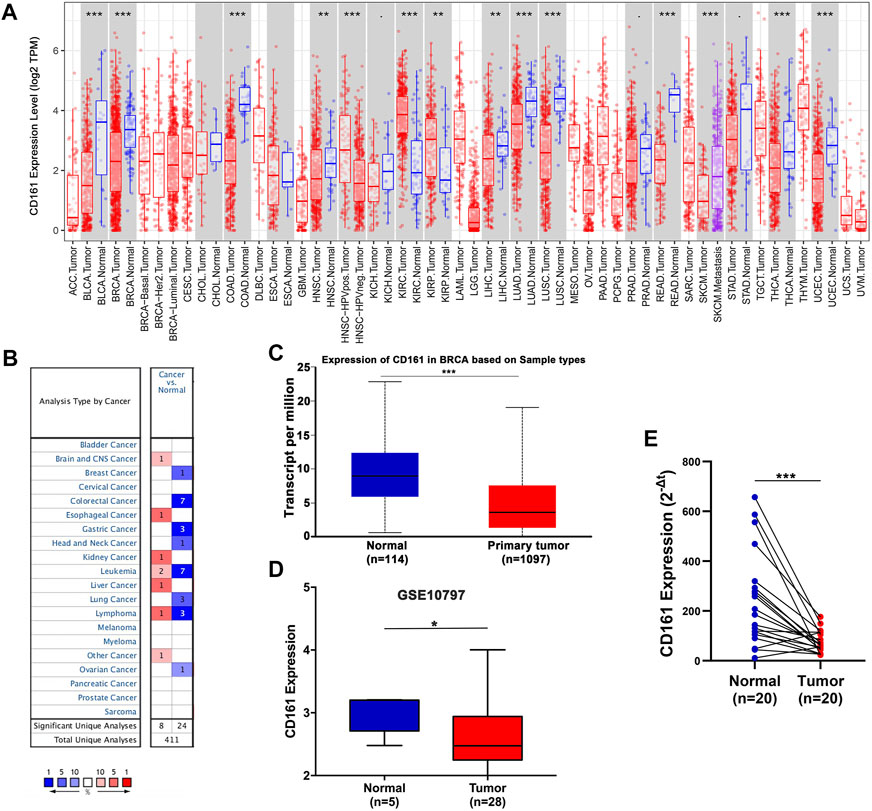
FIGURE 1. The mRNA expression levels of CD161 in various human cancers. (A) Differential expression of CD161 between paired tumor and normal tissues in various human cancers from the TIMER database. (B) Meta-analysis of CD161 expression between paired tumor and normal tissues across various human cancers from the Oncomine database. (C) Comparison of CD161 expression between breast tumor and normal samples in UALCAN database. (D) Comparison of CD161 expression between breast tumor and normal tissues in GEO GSE10797 datasets. (E) qRT-PCR results of CD161 expression between paired breast tumor and normal tissue samples from our cohort, n = 20. GEO, Gene Expression Omnibus. *p < 0.05, **p < 0.01, ***p < 0.001.
Correlation of CD161 expression with clinicopathological characteristics
All 1097 BC patients were divided into high and low CD161 expression groups, based on the cutoff value of 50%. The case was deleted from the data set when the stratified variable was missing. The binary logistic model showed that lower CD161 expression was associated with higher T stage (T2&T3&T4 vs T1, OR = 0.613, p < 0.001, N = 1080), distant metastasis (M1 vs M0, OR = 0.327, p = 0.032, N = 922), and higher pathologic stage (StageⅡ & StageⅢ & StageⅣ vs StageⅠ, OR = 0.642, p = 0.007, N = 1060). Meanwhile, there was no statistical correlation between CD161 expression and other clinicopathological parameters, like lymph node stage, PR status, ER status, and anatomic neoplasm subdivision (Table 1).
Prognostic value of CD161 in BC
Based on the RNA-seq data and clinicopathological data from TCGA, the prognostic value of CD161 expression in BC was evaluated by Kaplan-Meier plotter database and GEPIA database. The results showed that higher CD161 expression was significantly related to prolonged survival (OS, HR = 0.56, p < 0.001; DFS, HR = 0.77, p < 0.001; OS, HR = 0.77, p < 0.001; DFS, HR = 0.64, p = 0.033) (Figures 2A–D). We next verified the favorable prognostic value of CD161 in BC by PrognoScan database with GEO data (GSE7378, DFS, HR = 0.21, p < 0.001; GSE1456, DSS, HR = 0.61, p = 0.032) (Figures 2E,F). Moreover, the univariate and multivariate Cox analysis suggested that CD161 expression (HR = 0.864, p = 0.05), PR status (HR = 0.396, p = 0.006), and age (HR = 1.029, p < 0.001) were independent prognostic factors for OS in BC (Table 2 and Figure 3A). Therefore, we constructed a nomogram to predict the overall survival probability in BC based on CD161 expression, PR status and age (Figure 3B). The calibration plots and C-index (0.78) implied an outstanding predictive and discriminative power of the nomogram (Figure 3C).
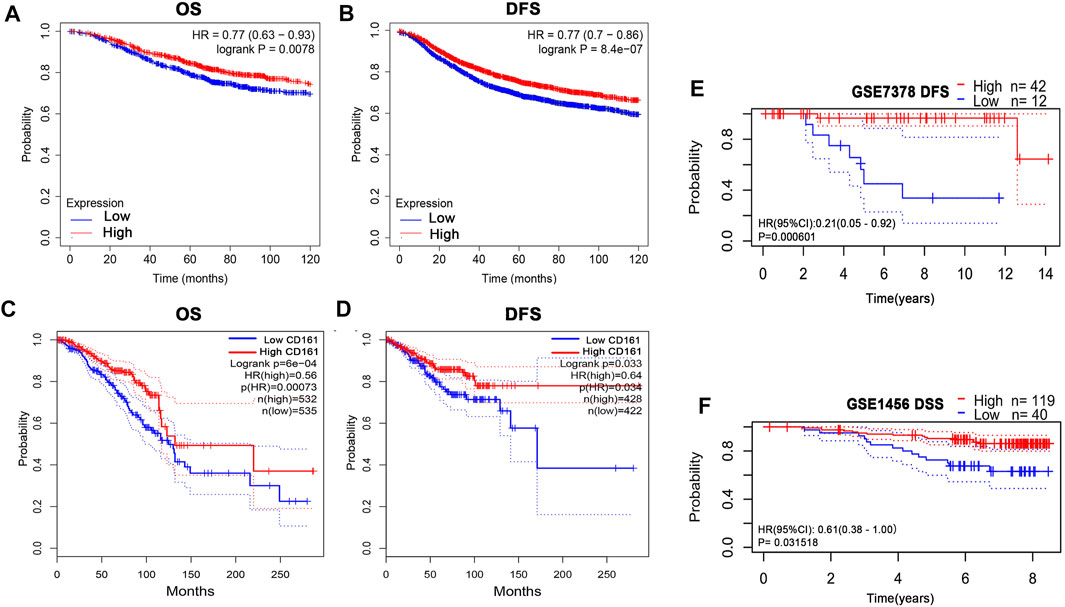
FIGURE 2. Kaplan-Meier assessment for survival outcomes according to CD161 expression in BC patients. (A) The OS estimate of high CD161 expression survivors was higher than low CD161 expression survivors from Kaplan–Meier Plotter database (p < 0.01). (B) The DFS estimate of high CD161 expression survivors was higher than low CD161 expression survivors from Kaplan–Meier Plotter database (p < 0.001). (C) The OS estimate of high CD161 expression survivors was higher than low CD161 expression survivors from GEPIA database (p < 0.001). (D) The DFS estimate of high CD161 expression survivors was higher than low CD161 expression survivors from GEPIA database (p = 0.03). (E) High CD161 expression survivors had improved DFS compared with low CD161 expression survivors in GSE7378 from PrognoScan database (p < 0.001).(F) High CD161 expression survivors had improved DSS compared with low CD161 expression survivors in GSE1456 from PrognoScan database (p = 0.03). BC, breast cancer; OS, overall survival; DFS, disease free survival; DSS, disease-specific survival.
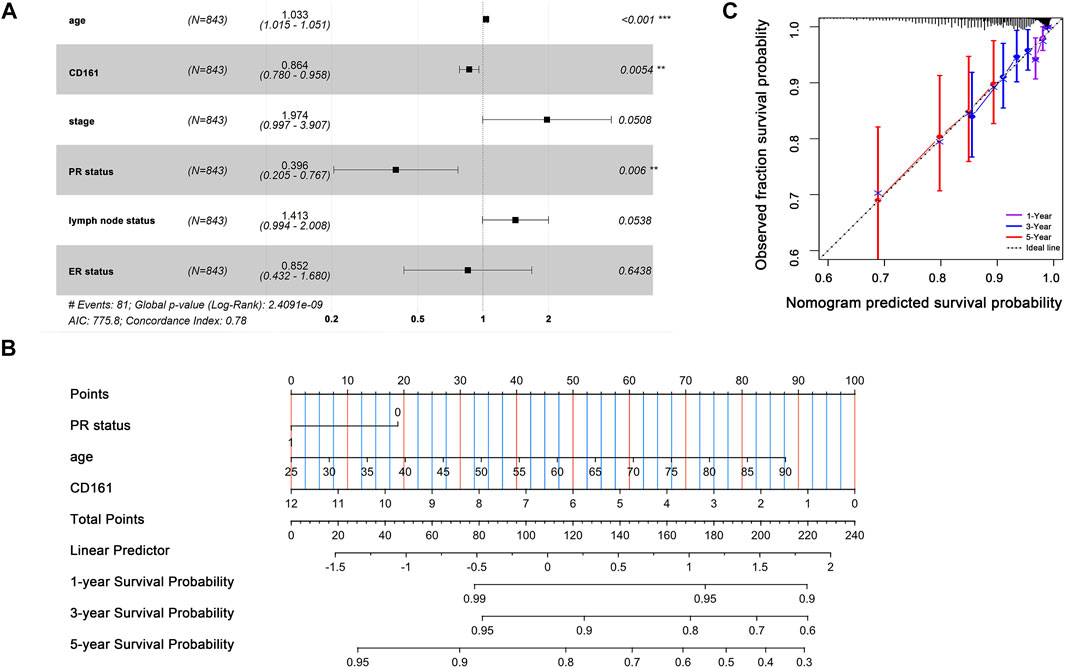
FIGURE 3. Prognostic value of CD161 expression in BC patients. (A) Multivariate Cox analyses on variables for the prediction of overall survival of BC patients. The effect of PR status, age and CD161 expression on survival was statistically significant. The results were demonstrated as a forest plot. (B) A constructed nomogram for prognostic prediction of BC patients. For PR status, 0 represented PR-negative status and 1 represented PR-positive status. The importance of each variable was ranked according to the standard deviation along nomogram scales. To use the nomogram, the specific points of individual patients were located on each variable axis. Lines and dots were drawn upward to determine the points received by each variable; the sum of these points was located on the Total Points axis to determine the probability of 1-year, 3-year and 5-year OS. (C) Calibration curves of the nomogram. The dotted line indicated the ideal reference line where predicted probabilities would match the observed survival rates. The dots were calculated by bootstrapping (resample: 1000) and represented the performance of the nomogram. The closer the solid lines were to the dotted line, the more accurately the model predicted survival. The purple solid line, blue solid line and red solid line represented the calibration curve of 1-year OS, 3-year OS and 5-year OS separately. OS, overall survival; BC, breast cancer; PR, progesterone receptor. *p < 0.05, **p < 0.01, ***p < 0.001.
Function enrichment analysis
To explore the molecular mechanism of CD161 in BC, we performed GSEA analysis in high and low CD161 expression groups, and GO and KEGG enrichment analyses in CD161 co-expressed genes. In the GSEA analysis, NK cell-mediated cytotoxicity pathway, T cell receptor signaling pathway, B cell receptor signaling pathway, antigen processing and presentation, cell adhesion molecules cams, cytokine-cytokine receptor interaction, primary immunodeficiency, and hematopoietic cell lineage were significantly enriched in CD161 low expression group, according to NES, NOM p values, and FDR values (Figure 4). The GO and KEGG enrichment analyses were performed based on 728 co-expressed genes screened out of the cBioPortal database with |Spearman’s correlation| >0.5 and p < 0.05 (Supplementary Table S4) (Schober et al., 2018). GO analysis showed that CD161 co-expressed genes were enriched in immune responses, especially T cell-related adaptive immune response. They acted as structural constituents in the plasma membrane, and were involved in signaling receptor activity (Figures 5A–C). KEGG pathway analysis showed their enrichment in pathways of hematopoietic cell lineage, cell adhesion, and cytokine-cytokine receptor interaction (Figure 5D). These analyses suggested that CD161 regulated immune response to suppress BC progression and improve its prognosis.
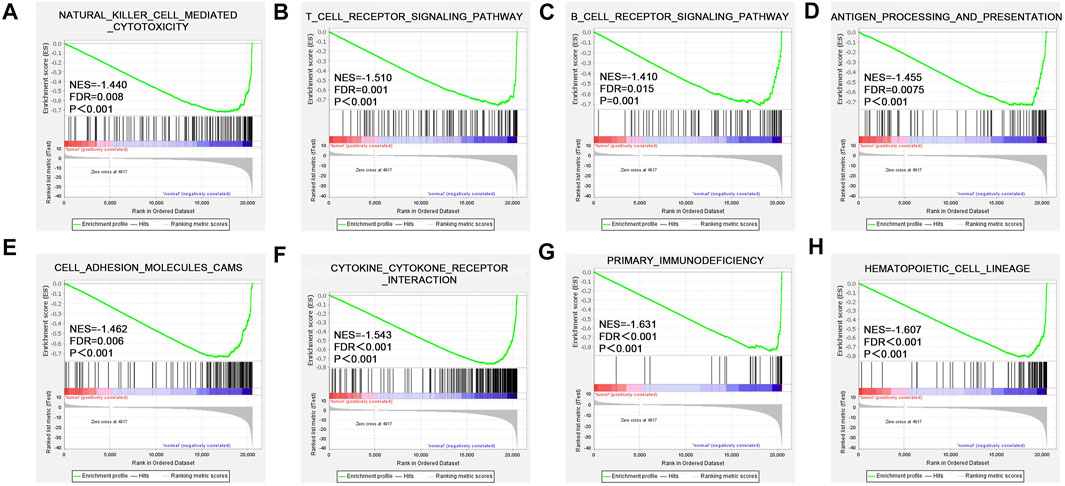
FIGURE 4. Enriched pathways of CD161 expression in BC analyzed by GSEA. Up-regulated genes located on the left approaching the origin of the coordinates, and down-regulated genes lay on the right of x-axis. Only gene sets with p < 0.05 and FDR <0.05 were considered significant. Top eight significant pathways associated with low CD161 expression were displayed in the plot. (A) NK cell-mediated cytotoxicity pathway, (B) T cell receptor signaling pathway, (C) B cell receptor signaling pathway, (D) antigen processing and presentation, (E) cell adhesion molecules cams, (F) cytokine-cytokine receptor interaction, (G) primary immunodeficiency, (H) hematopoietic cell lineage. BC, breast cancer; GSEA, gene set enrichment analysis; NES, normalized enrichment score; FDR, false discovery rate.
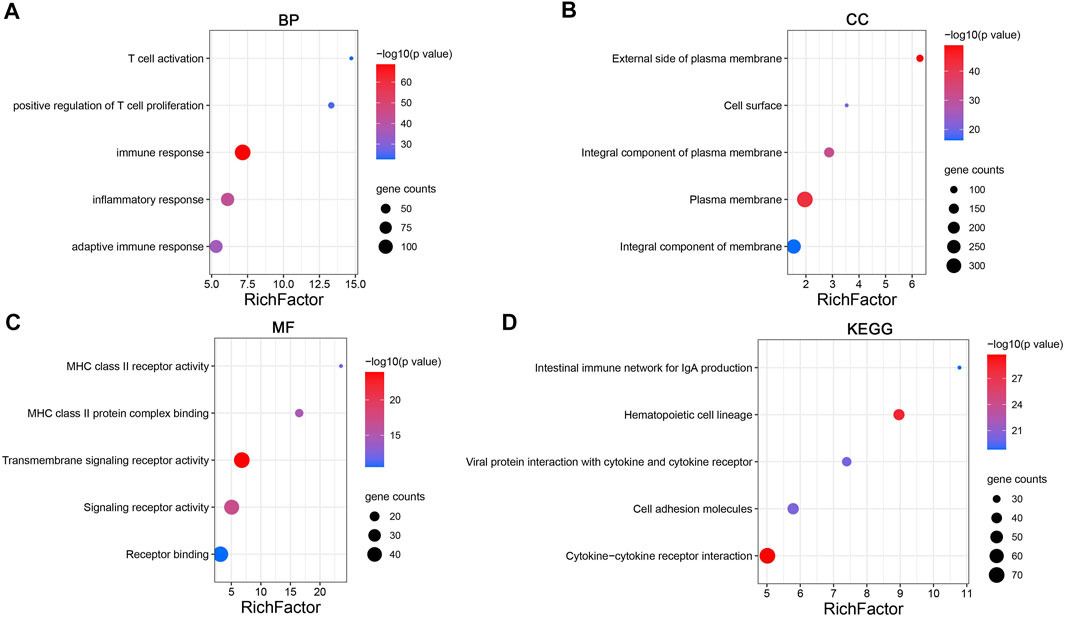
FIGURE 5. Enrichment analysis of CD161 co-expression gene network in BC. (A–C) Bubble plots displayed the top 5 BP, MF and CC terms that were significantly associated with CD161 co-expression genes. (D) Bubble plots displayed the top 5 KEGG enrichment pathways that were significantly associated with CD161 co-expression genes. X-axis: the ratio of genes belonged to the corresponding terms/pathways versus the whole co-expression genes. Y-axis: the top 5 GO terms/significant enrichment pathways. The color gradient of the bubble referred to the enrichment p-value, and the size of the bubble referred to the number of co-expression genes in corresponding GO terms/pathways. BP, biological progress; CC, cellular component; MF, molecular function; GO, Gene Ontology.
Relationship between CD161 expression and TILs
As an indispensable element of immune response, TILs are an independent predictor for cancer survival. Therefore, we further explored whether CD161 expression is related to immune infiltration in BC. CIBERSORT was employed to infer the differences in the proportions of 22 immune cells between high CD161 and low CD161 expression groups (Figure 6). The proportions of naive B cells, M1 macrophages, CD8+ T cells, CD4+ memory resting T cells, and follicular helper T (Tfh) cells were upregulated in the high CD161 expression group. On the contrary, the proportions of resting dendritic cells, M0 macrophages, neutrophils, activated natural killing (NK) cells, and CD4+ memory activated T cells were upregulated in the low CD161 expression group (Figure 6A). The correlation heatmap exhibited various degrees of correlations within the proportions of different TILs in BC (Figure 6B). The ssGSEA, TIMER database and TISIDB database were next employed to explore the correlation between CD161 expression level and immune cell infiltration level in BC immune microenvironment. The ssGSEA method showed that CD161 expression level had a strong correlation with the abundance of T cells (r = 0.848, p < 0.001), cytotoxic cells (r = 0.796, p < 0.001), B cells (r = 0.719, p < 0.001) (Figure 7). TIMER database illustrated a positive association between CD161 expression level and immune infiltration levels of B cells, CD8+ T cells, CD4+ T cells, neutrophils, and Dendritic cells (Figure 8A). TISIDB database exhibited that CD161 expression level was positively correlated with the levels of activated CD8+ T cells, activated CD4+T cells, activated B cells, macrophages, and NKT cells (Figure 8B). We further employed TIMER and GEPIA databases to explore the relationship between CD161 expression and the levels of gene markers of immune cells and T cell exhaustion (Table 3 and Supplementary Table S5). The results showed that CD161 expression was positively correlated with CD8A and CD8B of CD8+ T cell, CD4 of CD4+ T cell, KIR2DL3 and KIR3DL2 of NK cell, CD19 and CD79A of B cell, PD-1 and CTLA-4 of T cell exhaustion.
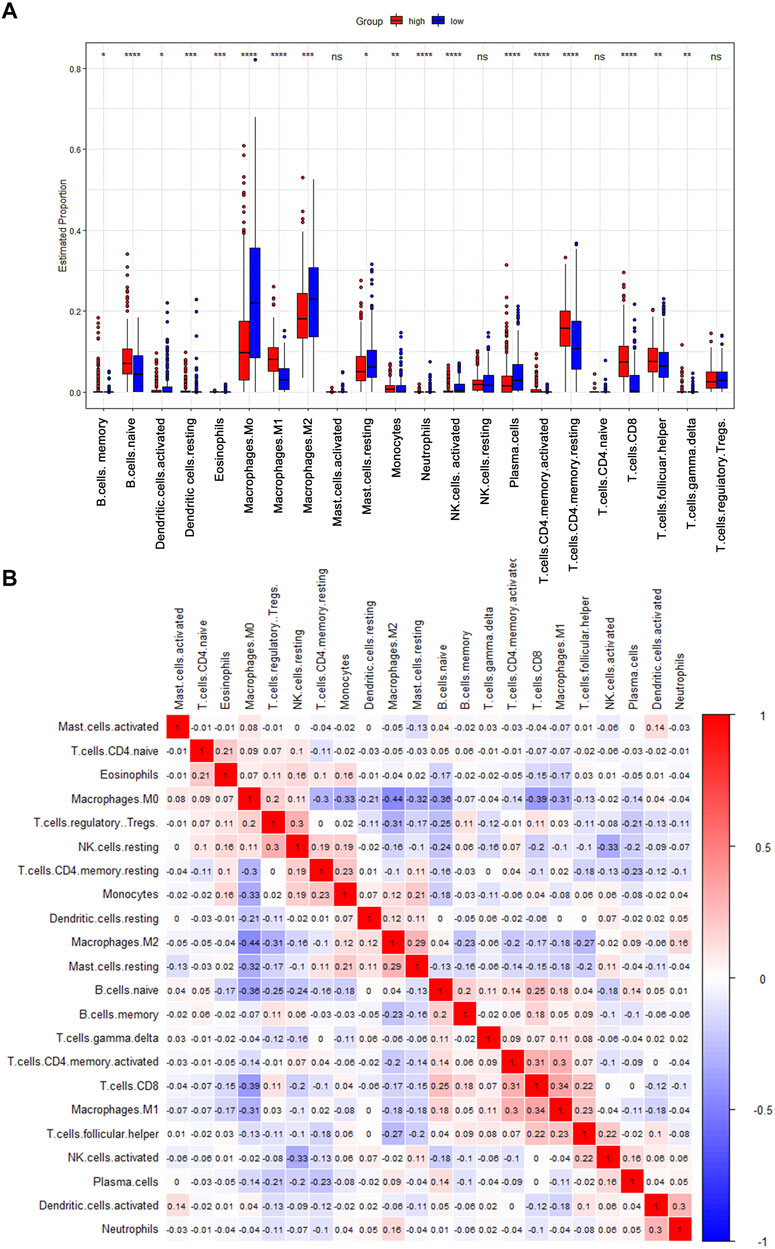
FIGURE 6. CIBERSORT analysis to evaluate the correlation between CD161 expression and the immune infiltration in BC. (A) The Boxplot showed the ratio differentiation of 22 kinds of immune cells between BC samples with high or low CD161 expression (red: high CD161 expression cohort; blue: low CD161 expression cohort). *p < 0.05, **p < 0.01, ***p < 0.001. (B) The correlation matrix showed the relevance between different TILs proportions in BC. The correlation coefficients were exhibited on the colored squares (red: positive Spearman’s rho; blue: negative Spearman’s rho). TILs, tumor infiltrating lymphocytes.
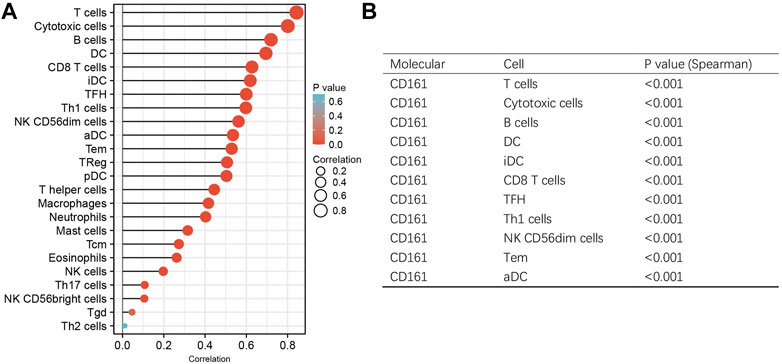
FIGURE 7. ssGSEA analysis to evaluate the correlation between CD161 expression and the immune infiltration in BC. (A) The Lollipop chart showed the association between CD161 expression and 24 kinds of immune cells. X-axis: Spearman’s rho. Y-axis: 24 types of immune cells. The color gradient of the lollipop referred to the correlation p-value, and the size of the lollipop referred to the correlation strength. (B) The Spearman’s correlation >0.5 and p < 0.05 groups were exhibited in the trilinear chart.
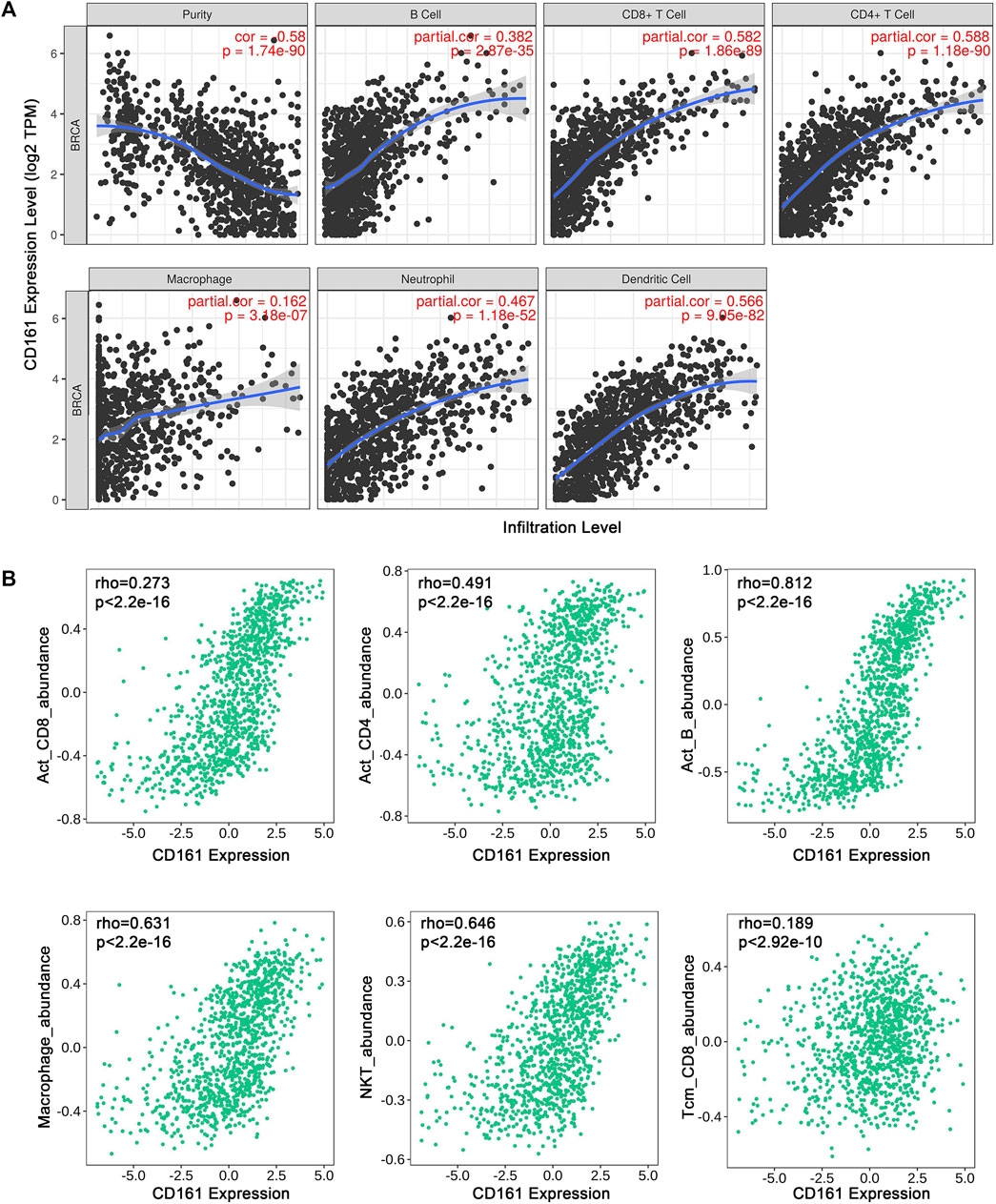
FIGURE 8. Correlation between CD161 expression and tumor purity or immune cell abundance in BC through different databases. (A) Scatter plots exhibited the correlations between CD161 expression and tumor purity, B cell, CD8+T cell, CD4+T cell, Macrophage, Neutrophil and Dendritic cell abundance from TIMER database. (B) Scatter plots exhibited the correlations between CD161 expression and Activated CD8+T cell, Activated CD4+T cell, Activated B cell, Macrophage, NKT cell and CD8+ Tcm abundance from TISIDB database.
Discussion
Due to the heterogeneity of breast tumor, the current clinicopathological staging and typing systems that provide clinical decision support and assist survival outcome prediction still have some limitations (Barry et al., 2010; Zardavas et al., 2015; Liu et al., 2022). New therapeutic strategies and prognostic biomarkers derived from intrinsic characteristics of breast tumor are urgently needed. Plenty of reports have described that breast tumor tissue consists of complex immune contexture (Korkaya et al., 2011; Koelwyn et al., 2017). Through a continuously dynamic interactions, the elements of TME especially tumor-infiltrating immune cells on one hand enhance antitumor immunity by destroying immunogenic tumor variants, and on the other hand promote tumor progression by shaping tumor immunogenicity (Baxevanis et al., 2021). Therefore, identifying the markers regulating tumor immune microenvironment is curcial for facilitating antitumor immunity and improving prognositic outcomes in BC patients. Natural killer cell receptors are found expressed on the surface of NK cells and T cells, participating in the regulation of activating/inhibitory signals and immune response (Zhou et al., 2021; Braud et al., 2022). CD161, encoded by KLRB1, is a C-type lectin-related type II transmembrane protein, which belongs to the natural killer cell receptors (Konduri et al., 2020; Braud et al., 2022). Among NK cells, CD161 acts as an inhibitory receptor to inhibit cytotoxicity and cytokine secretion (Richter et al., 2010; López-Soto et al., 2017). Among T cells, CD161 acts as a costimulatory receptor to increase the response to TCR stimulation (Halkias et al., 2019). Previous research has illustrated that high CD161 expression was associated with favorable clinical outcomes across 39 malignancies, including BC, non-small cell lung cancer, prostate adenocarcinoma, cholangiocarcinoma, and mesothelioma etc. (Braud et al., 2018; Zhou et al., 2021; Duurland et al., 2022). Although CD161 has been recognized as a protective factor for BC patients, the clinical significance and detailed mechanisms of abnormal expression of CD161 in BC have not been systematically discussed before. In our study, we found that compared with adjacent normal tissues, the expression level of CD161 was significantly decreased in BC tissues. The lower CD161 expression was associated with unfavorable clinicopathological features, including higher T stage, higher pathological stage, and distant metastasis. In addition, upregulated CD161 expression was closely correlated with prolonged OS, DFS and DSS, which is consistent with previous study results (Gentles et al., 2015; Zhou et al., 2021). By Cox regression analysis, we further discovered that CD161 expression was an independent prognostic factor for OS in BC. Next, we constructed a nomogram with CD161 expression, PR status and age to predict the OS of BC. The calibration plots and C-index (0.78) implied its outstanding predictive and discriminative power. These results demonstrate that CD161 can serve as a favorable prognostic biomarker for BC. In diffuse glioma and hepatocellular carcinoma, CD161 exerts carcinogenic effects by multiple cancer-related signaling pathways, including CD161-CLEC2D pathway, TCR signaling pathway and cytokine-cytokine receptor interaction (Author Anonymous, 2021; Mathewson et al., 2021; Sun et al., 2021). Nevertheless, the function, signaling pathway, and mechanism of CD161 in BC have not been fully elucidated and deserve further exploration. In our study, the GSEA analysis showed that NK cell-mediated cytotoxicity pathway, TCR signaling pathway, BCR signaling pathway were most significantly enriched in CD161 low expression group. The KEGG analysis showed that CD161 co-expressed genes were enriched in pathways of hematopoietic cell lineage, cell adhesion, and cytokine-cytokine receptor interaction. These findings suggest that CD161 may affect the survival outcomes of BC patients by regulating cancer-related immune response. This theoretical hypothesis requires further experimental validation. TILs are indispensable to an intact immune response to cancer, and their prognostic value has been verified in many solid tumors (Pagès et al., 2005; Maibach et al., 2020; Lopez de Rodas et al., 2022). Previous study has identified that the expression and regulation of CD161 define CD4+ T cells, thus improving the prognosis of OPSCC (Duurland et al., 2022). Accordingly, we next evaluated the correlation between CD161 expression and immune infiltration. The results indicated a remarkably positive association between CD161 expression and immune infiltration of B cells, CD8+ T cells, CD4+ T cells, and NK cells. Moreover, CD161 expression was also positively correlated with the levels of immune markers of NK cells, T cell, B cells, and T cell exhaustion, further validating the relevance between CD161 expression and immune infiltration. Based on the results of survival analysis, functional enrichment analysis and immune infiltration analysis, we speculated that CD161 may regulate immune infiltration to inhibit tumor progression and improve prognosis. We should realize several limitations of this study. First, it is a retrospective analysis based on existing public databases, and some important clinical information is not available. Selection bias and missing data may contribute to inaccuracy of results. Besides, the in vitro and in vivo experiments were not carried out to confirm the results. Last, the enrichment analysis was not enough to figure out the specific mechanisms involving CD161-related immune signaling pathways. The mechanism of CD161 in regulating the immune cell infiltration in BC should be further explored.
Conclusion
CD161 is an independent prognostic factor in BC, and a high expression of CD161 is significantly correlated with favorable clinicopathological parameters, better clinical outcomes and increased immune infiltration. CD161 may serve as a potential prognostic and therapeutic biomarker for BC.
Data availability statement
The original contributions presented in the study are included in the article/Supplementary Material, further inquiries can be directed to the corresponding author.
Ethics statement
The studies involving human participants were reviewed and approved by the Medical Ethics Review Committee of the first affiliated hospital of Nanjing Medical University. The patients/participants provided their written informed consent to participate in this study. Written informed consent was obtained from the individual(s) for the publication of any potentially identifiable images or data included in this article.
Author contributions
The corresponding authors, YH and SW, designed the study; MW, MZ, and XH acquired and analyzed the data; YH and MW drafted the manuscript; SW and HX reviewed the manuscript and performed a part of the statistical analysis. The authors read and approved the final manuscript.
Funding
This study was financially supported by the Young Scholars Fostering Fund of the First Affiliated Hospital of Nanjing Medical University (PY2021011) and the Scientific Research Project of Jiangsu Women and Children Health Hospital for Youth Talents (FYRC202003).
Conflict of interest
The authors declare that the research was conducted in the absence of any commercial or financial relationships that could be construed as a potential conflict of interest.
Publisher’s note
All claims expressed in this article are solely those of the authors and do not necessarily represent those of their affiliated organizations, or those of the publisher, the editors and the reviewers. Any product that may be evaluated in this article, or claim that may be made by its manufacturer, is not guaranteed or endorsed by the publisher.
Supplementary material
The Supplementary Material for this article can be found online at: https://www.frontiersin.org/articles/10.3389/fgene.2022.996345/full#supplementary-material
Abbreviations
BC, breast cancer; BLCA, bladder cancer; BRCA, breast invasive carcinoma; COAD, colon adenocarcinoma; DFS, disease-free survival; DSS, disease-specific survival; GEO, Gene Expression Omnibus; GO, Gene Ontology; GSEA, gene set enrichment analysis; HR, hazard ratio; KLRB1, killer cell lectin-like receptor B1; NK cells, natural killing cells; OPSCC, oropharyngeal squamous cell cancer; OR, odds ratio; OS, overall survival; qRT-PCR, quantitative real-time polymerase chain reaction; TCR, T cell receptor; Tfh, follicular helper T; TILs, tumor-infiltrating lymphocytes; TME, tumor microenvironment.
References
Author Anonymous (2021). Inhibitory CD161 receptor is expressed on glioma-infiltrating T cells. Cancer Discov. 11, Of19. doi:10.1158/2159-8290.Cd-rw2021-027
Barry, W. T., Kernagis, D. N., Dressman, H. K., Griffis, R. J., Hunter, J. D., Olson, J. A., et al. (2010). Intratumor heterogeneity and precision of microarray-based predictors of breast cancer biology and clinical outcome. J. Clin. Oncol. 28, 2198–2206. doi:10.1200/jco.2009.26.7245
Baxevanis, C. N., Fortis, S. P., and Perez, S. A. (2021). The balance between breast cancer and the immune system: Challenges for prognosis and clinical benefit from immunotherapies. Semin. Cancer Biol. 72, 76–89. doi:10.1016/j.semcancer.2019.12.018
Bejarano, L., Jordāo, M. J. C., and Joyce, J. A. (2021). Therapeutic targeting of the tumor microenvironment. Cancer Discov. 11, 933–959. doi:10.1158/2159-8290.Cd-20-1808
Braud, V. M., Biton, J., Becht, E., Knockaert, S., Mansuet-Lupo, A., Cosson, E., et al. (2018). Expression of LLT1 and its receptor CD161 in lung cancer is associated with better clinical outcome. Oncoimmunology 7, e1423184. doi:10.1080/2162402x.2017.1423184
Braud, V. M., Meghraoui-Kheddar, A., Elaldi, R., Petti, L., Germain, C., and Anjuère, F. (2022). LLT1-CD161 interaction in cancer: Promises and challenges. Front. Immunol. 13, 847576. doi:10.3389/fimmu.2022.847576
Byrne, A., Savas, P., Sant, S., Li, R., Virassamy, B., Luen, S. J., et al. (2020). Tissue-resident memory T cells in breast cancer control and immunotherapy responses. Nat. Rev. Clin. Oncol. 17, 341–348. doi:10.1038/s41571-020-0333-y
Duurland, C. L., Santegoets, S. J., Abdulrahman, Z., Loof, N. M., Sturm, G., Wesselink, T. H., et al. (2022). CD161 expression and regulation defines rapidly responding effector CD4+ T cells associated with improved survival in HPV16-associated tumors. J. Immunother. Cancer 10, e003995. doi:10.1136/jitc-2021-003995
Fergusson, J. R., Smith, K. E., Fleming, V. M., Rajoriya, N., Newell, E. W., Simmons, R., et al. (2014). CD161 defines a transcriptional and functional phenotype across distinct human T cell lineages. Cell Rep. 9, 1075–1088. doi:10.1016/j.celrep.2014.09.045
Flister, M. J., and Bergom, C. (2018). Genetic modifiers of the breast tumor microenvironment. Trends Cancer 4, 429–444. doi:10.1016/j.trecan.2018.04.003
Gentles, A. J., Newman, A. M., Liu, C. L., Bratman, S. V., Feng, W., Kim, D., et al. (2015). The prognostic landscape of genes and infiltrating immune cells across human cancers. Nat. Med. 21, 938–945. doi:10.1038/nm.3909
Godeau, D., Petit, A., Richard, I., Roquelaure, Y., and Descatha, A. (2021). Return-to-work, disabilities and occupational health in the age of COVID-19. Scand. J. Work Environ. Health 47, 408–409. doi:10.5271/sjweh.3960
Guo, S., and Deng, C. X. (2018). Effect of stromal cells in tumor microenvironment on metastasis initiation. Int. J. Biol. Sci. 14, 2083–2093. doi:10.7150/ijbs.25720
Halkias, J., Rackaityte, E., Hillman, S. L., Aran, D., Mendoza, V. F., Marshall, L. R., et al. (2019). CD161 contributes to prenatal immune suppression of IFNγ-producing PLZF+ T cells. J. Clin. Invest. 129, 3562–3577. doi:10.1172/jci125957
Koelwyn, G. J., Quail, D. F., Zhang, X., White, R. M., and Jones, L. W. (2017). Exercise-dependent regulation of the tumour microenvironment. Nat. Rev. Cancer 17, 620–632. doi:10.1038/nrc.2017.78
Konduri, V., Oyewole-Said, D., Vazquez-Perez, J., Weldon, S. A., Halpert, M. M., Levitt, J. M., et al. (2020). CD8(+)CD161(+) T-cells: Cytotoxic memory cells with high therapeutic potential. Front. Immunol. 11, 613204. doi:10.3389/fimmu.2020.613204
Korkaya, H., Liu, S., and Wicha, M. S. (2011). Breast cancer stem cells, cytokine networks, and the tumor microenvironment. J. Clin. Invest. 121, 3804–3809. doi:10.1172/jci57099
Li, Z., Zheng, B., Qiu, X., Wu, R., Wu, T., Yang, S., et al. (2020). The identification and functional analysis of CD8+PD-1+CD161+ T cells in hepatocellular carcinoma. NPJ Precis. Oncol. 4, 28. doi:10.1038/s41698-020-00133-4
Lim, B., Woodward, W. A., Wang, X., Reuben, J. M., and Ueno, N. T. (2018). Inflammatory breast cancer biology: The tumour microenvironment is key. Nat. Rev. Cancer 18, 485–499. doi:10.1038/s41568-018-0010-y
Liu, S. Q., Gao, Z. J., Wu, J., Zheng, H. M., Li, B., Sun, S., et al. (2022). Single-cell and spatially resolved analysis uncovers cell heterogeneity of breast cancer. J. Hematol. Oncol. 15, 19. doi:10.1186/s13045-022-01236-0
Lopez de Rodas, M., Nagineni, V., Ravi, A., Datar, I. J., Mino-Kenudson, M., Corredor, G., et al. (2022). Role of tumor infiltrating lymphocytes and spatial immune heterogeneity in sensitivity to PD-1 axis blockers in non-small cell lung cancer. J. Immunother. Cancer 10, e004440. doi:10.1136/jitc-2021-004440
López-Soto, A., Gonzalez, S., Smyth, M. J., and Galluzzi, L. (2017). Control of metastasis by NK cells. Cancer Cell 32, 135–154. doi:10.1016/j.ccell.2017.06.009
Maibach, F., Sadozai, H., Seyed Jafari, S. M., Hunger, R. E., and Schenk, M. (2020). Tumor-infiltrating lymphocytes and their prognostic value in cutaneous melanoma. Front. Immunol. 11, 2105. doi:10.3389/fimmu.2020.02105
Mathewson, N. D., Ashenberg, O., Tirosh, I., Gritsch, S., Perez, E. M., Marx, S., et al. (2021). Inhibitory CD161 receptor identified in glioma-infiltrating T cells by single-cell analysis. Cell 184, 1281–1298.e26. doi:10.1016/j.cell.2021.01.022
Olson, O. C., and Joyce, J. A. (2013). Microenvironment-mediated resistance to anticancer therapies. Cell Res. 23, 179–181. doi:10.1038/cr.2012.123
Pagès, F., Berger, A., Camus, M., Sanchez-Cabo, F., Costes, A., Molidor, R., et al. (2005). Effector memory T cells, early metastasis, and survival in colorectal cancer. N. Engl. J. Med. 353, 2654–2666. doi:10.1056/NEJMoa051424
Paijens, S. T., Vledder, A., de Bruyn, M., and Nijman, H. W. (2021). Tumor-infiltrating lymphocytes in the immunotherapy era. Cell. Mol. Immunol. 18, 842–859. doi:10.1038/s41423-020-00565-9
Pondé, N. F., Zardavas, D., and Piccart, M. (2019). Progress in adjuvant systemic therapy for breast cancer. Nat. Rev. Clin. Oncol. 16, 27–44. doi:10.1038/s41571-018-0089-9
Richter, J., Benson, V., Grobarova, V., Svoboda, J., Vencovsky, J., Svobodova, R., et al. (2010). CD161 receptor participates in both impairing NK cell cytotoxicity and the response to glycans and vimentin in patients with rheumatoid arthritis. Clin. Immunol. 136, 139–147. doi:10.1016/j.clim.2010.03.005
Savas, P., Virassamy, B., Ye, C., Salim, A., Mintoff, C. P., Caramia, F., et al. (2018). Single-cell profiling of breast cancer T cells reveals a tissue-resident memory subset associated with improved prognosis. Nat. Med. 24, 986–993. doi:10.1038/s41591-018-0078-7
Schober, P., Boer, C., and Schwarte, L. A. (2018). Correlation coefficients: Appropriate use and interpretation. Anesth. Analg. 126, 1763–1768. doi:10.1213/ane.0000000000002864
Stanton, S. E., and Disis, M. L. (2016). Clinical significance of tumor-infiltrating lymphocytes in breast cancer. J. Immunother. Cancer 4, 59. doi:10.1186/s40425-016-0165-6
Subramanian, A., Kuehn, H., Gould, J., Tamayo, P., and Mesirov, J. P. (2007). GSEA-P: A desktop application for gene set enrichment analysis. Bioinformatics 23, 3251–3253. doi:10.1093/bioinformatics/btm369
Sun, Y., Wu, L., Zhong, Y., Zhou, K., Hou, Y., Wang, Z., et al. (2021). Single-cell landscape of the ecosystem in early-relapse hepatocellular carcinoma. Cell 184, 404–421. doi:10.1016/j.cell.2020.11.041
Sung, H., Ferlay, J., Siegel, R. L., Laversanne, M., Soerjomataram, I., Jemal, A., et al. (2021). Global cancer statistics 2020: GLOBOCAN estimates of incidence and mortality worldwide for 36 cancers in 185 countries. Ca. Cancer J. Clin. 71, 209–249. doi:10.3322/caac.21660
Welters, M. J. P., Ma, W., Santegoets, S., Goedemans, R., Ehsan, I., Jordanova, E. S., et al. (2018). Intratumoral HPV16-specific T cells constitute a type I-oriented tumor microenvironment to improve survival in HPV16-driven oropharyngeal cancer. Clin. Cancer Res. 24, 634–647. doi:10.1158/1078-0432.Ccr-17-2140
Werner, S., Heidrich, I., and Pantel, K. (2022). Clinical management and biology of tumor dormancy in breast cancer. Semin. Cancer Biol. 78, 49–62. doi:10.1016/j.semcancer.2021.02.001
Xie, Q., Ding, J., and Chen, Y. (2021). Role of CD8(+) T lymphocyte cells: Interplay with stromal cells in tumor microenvironment. Acta Pharm. Sin. B 11, 1365–1378. doi:10.1016/j.apsb.2021.03.027
Yang, Z., Gao, D., Guo, X., Jin, L., Zheng, J., Wang, Y., et al. (2020). Fighting immune cold and reprogramming immunosuppressive tumor microenvironment with red blood cell membrane-camouflaged nanobullets. ACS Nano 14, 17442–17457. doi:10.1021/acsnano.0c07721
Zardavas, D., Irrthum, A., Swanton, C., and Piccart, M. (2015). Clinical management of breast cancer heterogeneity. Nat. Rev. Clin. Oncol. 12, 381–394. doi:10.1038/nrclinonc.2015.73
Keywords: CD161, breast cancer, prognostic biomarker, immune infiltration, function enrichment analysis
Citation: Weng M, Xie H, Zheng M, Hou X, Wang S and Huang Y (2022) Identification of CD161 expression as a novel prognostic biomarker in breast cancer correlated with immune infiltration. Front. Genet. 13:996345. doi: 10.3389/fgene.2022.996345
Received: 17 July 2022; Accepted: 12 September 2022;
Published: 30 September 2022.
Edited by:
Jared C Roach, Institute for Systems Biology (ISB), United StatesReviewed by:
Seila Lorenzo-Herrero, University of Oviedo, SpainYu Fan, Southwest Medical University, China
Copyright © 2022 Weng, Xie, Zheng, Hou, Wang and Huang. This is an open-access article distributed under the terms of the Creative Commons Attribution License (CC BY). The use, distribution or reproduction in other forums is permitted, provided the original author(s) and the copyright owner(s) are credited and that the original publication in this journal is cited, in accordance with accepted academic practice. No use, distribution or reproduction is permitted which does not comply with these terms.
*Correspondence: Shui Wang, d3MwODAxQGhvdG1haWwuY29t; Yue Huang, aGFwcHk2ODY5OTRAMTYzLmNvbQ==
†These authors have contributed equally to this work and share first authorship