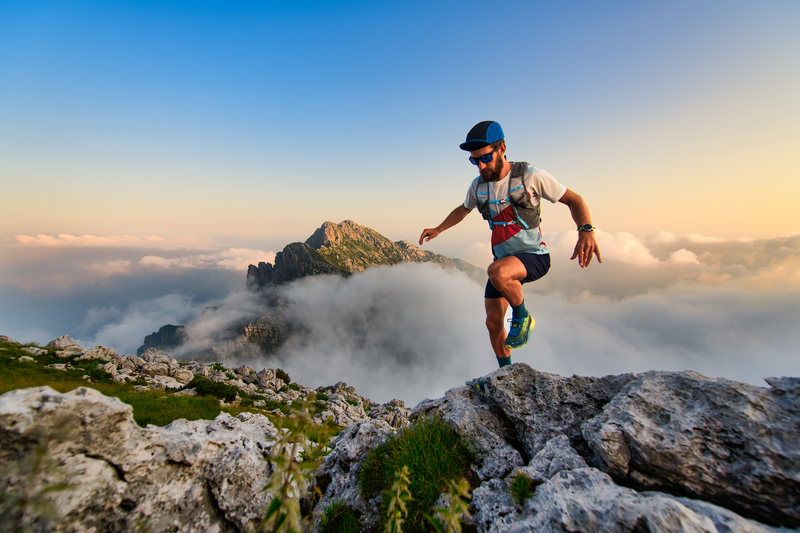
94% of researchers rate our articles as excellent or good
Learn more about the work of our research integrity team to safeguard the quality of each article we publish.
Find out more
ORIGINAL RESEARCH article
Front. Genet. , 12 September 2022
Sec. Genetics of Common and Rare Diseases
Volume 13 - 2022 | https://doi.org/10.3389/fgene.2022.990015
This article is part of the Research Topic Advancing Genomics for Rare Disease Diagnosis and Therapy Development Vol II View all 42 articles
Despite recent advancements in our understanding of genetic etiology and its molecular and physiological consequences, it is not yet clear what genetic features determine the inheritance pattern of a disease. To address this issue, we conducted whole exome sequencing analysis to characterize genetic variants in 1,180 Korean patients with neurological symptoms. The diagnostic yield for definitive pathogenic variant findings was 50.8%, after including 33 cases (5.9%) additionally diagnosed by reanalysis. Of diagnosed patients, 33.4% carried inherited variants. At the genetic level, autosomal recessive-inherited genes were characterized by enrichments in metabolic process, muscle organization and metal ion homeostasis pathways. Transcriptome and interactome profiling analyses revealed less brain-centered expression and fewer protein-protein interactions for recessive genes. The majority of autosomal recessive genes were more tolerant of variation, and functional prediction scores of recessively-inherited variants tended to be lower than those of dominantly-inherited variants. Additionally, we were able to predict the rates of carriers for recessive variants. Our results showed that genes responsible for neurodevelopmental disorders harbor different molecular mechanisms and expression patterns according to their inheritance patterns. Also, calculated frequency rates for recessive variants could be utilized to pre-screen rare neurodevelopmental disorder carriers.
Genetic disorders are caused by various alterations in gene function. According to the Online Mendelian Inheritance in Men (OMIM) compendium, 4,617 genes and their variants are associated with human disease as of April 2022 (Amberger et al., 2015; Amberger and Hamosh, 2017). Broadly, disease-associated alterations can be categorized as resulting in either gain-of-function (GoF) or loss-of-function (LoF) of a gene. GoF is mostly associated with dominant inheritance, whereas LoF can appear in recessive form as well as dominant (as haploinsufficiency) (Jimenez-Sanchez et al., 2001; Schuster-Böckler and Bateman, 2008). Mendelian disorders with recessive inheritance patterns are primarily observed in ethnic groups with high rates of consanguineous marriages (Verma and Puri, 2015; Abouelhoda et al., 2016), whereas those with dominant and recessive inheritance patterns appear in comparable rates in other “outbred” ethnic groups (Baird et al., 1988). Despite recent efforts into patient genome sequencing, diagnosis, and the discovery of novel genes that cause rare Mendelian disorders, it is not yet clear what drives genes to carry variants that are inherited in dominant or recessive patterns. It seems intuitive to postulate that genes that cause diseases in recessive pattern are less critical and more tolerant in development and physiology as one has to carry defective variants in both alleles for a disease to manifest. Nevertheless, there is need to systematically evaluate whether other properties that may represent gene function, expression, and previous disease associations also play a role in the process.
The study of genes that cause recessive diseases is important because knowledge of such genes can be utilized to predict marriages between carriers and avoid the generation of new patients. This strategy has been already proven successful in a number of diseases, such as β-thalassaemia and Tay–Sachs disease (Kaback, 2001; Cao and Kan, 2013). However, those diseases are single gene disorders and display ethnic biases, making the process of variant curation and evaluation for pathogenicity and also the prediction of patients more efficient. Meanwhile, applying such an approach to diseases with heuristic symptoms requires considerably more effort because of the involvement of many genes and variants and the diverse clinical symptoms. Therefore, understanding the features of genetic variants that cause disease in a recessive inheritance pattern will provide a novel approach for avoiding generation of patients.
Complex structure and function of brain involve coordinated expression and function of many genes, and this is also reflected on a diverse array of rare Mendelian neurodevelopmental diseases (NDD) that we observe from patients. Such patients display abnormal brain function and/or structure which may affect motor function, learning ability, development, language, and other brain activities. Diagnosis of such diseases is a challenge because of the extreme genetic heterogeneity and rare occurrence, while whole exome sequencing (WES) has enhanced the yield of NDD diagnoses in clinical practice (Yang et al., 2013; Iglesias et al., 2014; Lee et al., 2014; Srivastava et al., 2014; Yang et al., 2014; Retterer et al., 2016; Trujillano et al., 2017).
Here we report analyses of the factors that confer effects on genes that cause diseases in dominant or recessive inheritance pattern. Based on a cohort of 1,180 Korean Neurodevelopmental Disorder (KND) patients and additional patient datasets from Deciphering Developmental Delay (DDD; n = 13,500) and the Simons Foundation Autism Research Initiative (SFARI; n = 34,868), we found that genes which follow a recessive inheritance pattern are more tolerant, harbor variants of lower functionality, interact with fewer other proteins, and are more enriched in metabolic and mitochondrial functional categories when compared to those that follow a dominant inheritance pattern. In addition, the ongoing accumulation of carrier information suggests possible future utility for carrier prediction as more NDD patient data become available.
Patients with NDD and their parents who visited the Seoul National University Children’s Hospital (SNUCH) pediatric neurology clinic were recruited to this study. Informed consent and blood samples for genomic DNA were obtained under the approval of the Seoul National University Hospital (SNUH) internal review board (#1406-081-588). Patients with confirmed genetic variants identified through candidate gene sequencing, targeted gene sequencing panel, microarray, metabolic work-up, brain/spine MRI, or muscle biopsy were excluded. A total of 1,180 patients with complex neurological symptoms of suspected genetic origin were selected by the pediatric neurologists in SNUCH.
Genomic DNA was extracted from whole blood using the QIAamp DNA Blood Midi Kit according to the manufacturer’s instructions (Qiagen, Valencia, CA). WES procedures including exome capturing and sequencing were performed at Theragen Etex Bio Institute (Suwon, Korea). The data were analyzed as described previously (Lee et al., 2020). Briefly, Burrows-wheeler Aligner [v.0.7.15 (Li and Durbin 2010)] was used to align sequenced reads and the Picard software [v.2.8.0 (Broad Institute, 2019)], samtools [v.1.8 (Li et al., 2009)] and Genome Analysis Toolkit [GATK, v.4.1.4 (Mckenna et al., 2010)] were used for subsequent data processing steps such as removal of PCR duplicates, base recalibration, and variant quality control. ANNOVAR and SnpEff were used for variant annotation (Wang et al., 2010; Cingolani et al., 2012).
The normalized transcript level (TPM) of each gene was extracted from the Genotype-Tissue Expression (GTEx) project [v8 (GTEx Consortium, 2020)]. The median TPM value across all brain regions for each gene was divided by the median value of all other regions to determine relative brain vs. body expression. These relative TPM values were then plotted to visualize the distribution of the gene set. RNA-seq data and exon microarray data from BrainSpan (http://www.brainspan.org) were used to analyze brain spatial and temporal gene expression in the brain (Kang et al., 2011). This dataset contains expression from 16 cortical and subcortical structures along the full course of human brain development.
Protein correlation profiling of seven mouse tissues was used to explore tissue-specific PPI (Skinnider et al., 2021). The dataset contained more than 190,000 high-confidence PPIs identified with stable isotope labelling of tissues. We extracted for analysis those pairs that included proteins corresponding to the causal genes identified in the KND, DDD and SFARI cohorts.
The pathogenicity of variants in our dataset was assessed with reference to multiple databases as follows. Normal population database such as gnomAD, ExAC, 1000 Genomes, and KOVA were used to evaluate dominant variants that were never seen as heterozygous and recessive variants that were never seen as homozygous or hemizygous when filtered by allele frequency of 0.001 in heterozygous status. In silico prediction scores such as CADD (Rentzsch et al., 2019), SIFT (Ng and Henikoff, 2003), and phyloP (Siepel et al., 2005) were used to gain information regarding whether variants were evolutionarily well conserved at the amino acid level. Disease databases such as OMIM (Amberger et al., 2015; Amberger and Hamosh, 2017; Amberger et al., 2019), HGMD (Stenson et al., 2003), and ClinVar (Landrum et al., 2016) were used to find causative genes with consideration of genotype-phenotype associations. Then the variants were classified based on inheritance patterns such as de novo, compound heterozygous, homozygous, and hemizygous by comparing to genotypes in the parents. Copy number variation (CNV) analysis through WES was carried out by comparing the mean coverage depth of each captured interval to the mean coverage depth of parental samples as described previously (Lee et al., 2020).
To analyze disease associations and biological pathways that are enriched in a selected genes, a web-based analysis tool from Metascape (https://metascape.org/gp/index.html) was used. Conventional GO sources were used: biological process (BP), Cellular Component (CC) and Molecular Function (MF). Disease Gene Network (DisGeNET) was used for disease ontology. Results were collected and grouped into clusters for comparative analyses of biological process and disease association between gene groups.
Wilcox test was used to determine the statistical significance of the observed differences in functional scores for genes with different inheritance modes. The statistical significance of the expression level in boxplots was measured by a two-sample t-test. Statistical analyses were performed with R version 3.6.2.
Participants consisted of pediatric patients (mean age = 11.3 years, range 1–62) displaying one or more neurological symptoms including developmental delay, intellectual disability, intractable seizure, involuntary movements, or muscle weakness who visited SNUCH in 2014–2020 with idiopathic or undiagnosed symptoms. For genetic analysis, DNA from peripheral blood was subjected to WES. Among the 1,180 patients, parents of 707 patients were also sequenced. The resulting genome data were processed and pathogenic variants called with a standard process (Subjects and Methods). The pathogenic variants were identified in the 284 disease-causing genes in 1,180 KND patients (Supplementary Table S1). Overall, 41.9% of the patients carried known variants and 4.7% carried known variants but displayed symptoms different from those previously reported, possibly extending the disease spectra of these variants (Figure 1; Supplementary Table S2). Including the 4.2% of the patients with known CNVs (Supplementary Table S3), 50.8% of patients with pathogenic variants in known causal genes were definitively diagnosed by WES analysis (Figure 1A). This group with known variants was further divided according to variant inheritance pattern (Figure 1B). De novo variants were identified in more than half of diagnosed patients (62.9%) and recessive variants in about a quarter (24.7%). Lastly, 8.7% carried variants on the X chromosome with hemizygous status, making the proportion of inherited variants 33.4% (Figure 1B). This distribution of pathogenic variant inheritance is comparable to those reported in other studies using rare disease patients from outbred populations (Yang et al., 2013; Wright et al., 2015; Kuperberg et al., 2016; Marinakis et al., 2021).
FIGURE 1. Genetic diagnosis of 1,180 KND patients (KND1180). (A) Diagnostic yields of the 553 KND patients in 2020 (KND553), reanalysis of KND553, and KND1180. (B) Breakdown of diagnosed patients by mode of inheritance.
Re-evaluation of patients previously determined to be without pathogenic variants often allows for the discovery of new variants due to accumulating understanding of gene-disease relationships and improved bioinformatic pipelines (Ewans et al., 2018; Epilepsy Genetics Initiative, 2019; Jalkh et al., 2019; Fung et al., 2020). Previous re-analysis studies have reported 5%–12% increases in diagnostic yield (Ewans et al., 2018; Epilepsy Genetics Initiative, 2019; Jalkh et al., 2019; Fung et al., 2020); here, we observed a 5.9% increase in diagnostic yield, discovering pathogenic variants in 33 patients among the 553 that were previously analyzed in 2020 (KND553; Figure 1A) (Lee et al., 2020). The variants implicated in these 33 cases can be broadly divided into two groups, 1) variants for which new entries in OMIM allowed defining them as pathogenic (n = 8; Table 1) and 2) pathogenic calls previously missed during the bioinformatic process (n = 25; Table 2).
NDDs display considerable clinical and biological heterogeneity. The innate function and expression pattern of a gene can impact both its inheritance mode and the phenotype when its function or expression is altered. Understanding their genetic properties associated with inheritance modes will help in gaining a more comprehensive view of NDD. To develop this understanding, we first analyzed functional enrichments of those pathogenic genes that follow a dominant or recessive pattern in KND, and compared them with corresponding gene sets from DDD or SFARI (Figure 2A). GO analysis revealed that KND genes are enriched in molecular function and biological process terms relating to brain developmental progression, such as regulation of membrane potential, chromatin organization, head development, and pyrophosphatase activity (Figure 2A). In addition, the published variants from DDD and SFARI shared biological mechanisms involved in brain development (Supplementary Figure S1). Interestingly, differential enrichments were observed between dominant and recessive genes, with remarkably little overlap between the two gene groups (Figure 2B; Supplementary Table S4). In particular, dominant genes were strongly enriched for synaptic functions, while recessive genes were characterized by metabolic process, mitochondrial function, and muscular disease terms. Remarkably, X-linked genes shared more terms with dominant genes than with recessive genes (Figure 2B). This is unexpected because the majority of X-linked genes follow a hemizygous pattern, where disease manifests when the only X-chromosome allele in a male patient is mutated, and may follow a recessive pattern.
FIGURE 2. Comparative analysis of genes that cause neurodevelopmental disorders across inheritance patterns. (A) GO result of genes that led to the definitive diagnosis in KND, DDD, and SFARI. (B) Breakdown of KND genes by inheritance pattern. (C) Relative expression of genes in the brain vs. the body (brain/body median TPM). (D) Boxplot of median gene expression in the brain for two developmental periods. (E) Proportion of genes having the given number of PPI events in brain tissue. (F) Proportion of genes having the given number of PPI-positive tissues. Tissues that correspond to two to seven are heart, kidney, liver, lung, muscle, and thymus. BP, biological process; CC, cellular component; MF, molecular function; DO, disease ontology; AD, autosomal dominant; AR, autosomal recessive; XL, X-linked.
Next, we used GTEx data to compare the expression profiles of KND genes in brain and other tissues to determine if expression profiles would also differ by inheritance pattern. The results showed that KND genes having brain-specific expression were more enriched in the dominant gene set compared to the recessive gene set (p = 1.4 × 10−7; Figure 2C). Interestingly, the relative brain expression of X-linked genes was more similar to dominant genes than to recessive genes. BrainSpan comprises a comprehensive survey of gene expression in the brain during development, and in the dataset, dominant genes displayed increased expression level relative to both recessive and X-linked genes (between dominant and recessive genes, p = 1.4 × 10−9 for the prenatal period and p = 3.5 × 10−6 for the postnatal period; Figure 2D). There was no substantial difference between prenatal and postnatal expression levels (Figure 2D). Therefore, our findings imply a clear distinction in function and expression level for genes of different inheritance modes.
PPI information enables us to explore the biological function of a protein though its physical interactions with other proteins. A recent study provided data on protein pairs that interacted in seven mouse tissues, which we used to identify PPIs for KND genes in tissue-specific context (Skinnider et al., 2021). Focusing on brain tissue, we observed that the fraction of genes with PPIs was greater among dominant genes (59/163 = 36.2%) than for recessive genes (26/117 = 22.2%), and the mean number of interactions was also higher (7.2 for dominant genes vs. 4.5 for recessive genes) (Figure 2E). Among those recessive genes having PPIs at least one interaction in the brain, more than half also interacted with other proteins in all seven tissues (14/26 = 53.8%; Figure 2F), implying these genes to have a more ubiquitous functional pattern. PPIs from DDD and SFARI were also compared for validation (Supplementary Figure S2) and yielded similar patterns of brain-specific PPIs for dominant gene products and broader PPIs for recessive gene products.
The tolerance of a gene, indicating the degree to which a critical mutation in it may be detrimental to human development and physiology, is effectively represented by the probability of loss of function intolerance (pLI) and the observed/expected (O/E) constraint ratio scores in gnomAD (Lek et al., 2016). In KND, autosomal dominant genes tended to have pLI values close to 1, representing strong constraint, while autosomal recessive genes showed the opposite trend with pLI close to 0 (e.g., 75.0% of autosomal dominant genes are near 1, and 84.6% of autosomal recessive genes are near 0; Figure 3A). Consistent patterns were also observed for DDD and SFARI. Meanwhile, similar to the GO analysis findings, X-linked recessive genes exhibited patterns akin to dominant genes. These observations were recapitulated when using O/E values (Figure 3A). All told, these findings suggest that genes responsible for NDDs harbor different functions according to their inheritance patterns, and they share little in terms of the molecular pathways leading to disease phenotype.
FIGURE 3. Comparison of genetic characteristics of genes and variants that follow dominant or recessive inheritance. (A) Comparison of pLI or O/E scores of NDD causal genes across inheritance patterns. (B) Comparison of functional (CADD, SIFT, and phyloP) and conservation scores among NDD causal variants according to inheritance patterns. “AA conservation” denotes the number of species with different amino acids in 99 human ortholog proteins. *, p-value < 0.1, **, p-value < 0.01, ***, p-value < 0.001. ****, p-value < 0.00001.
We next investigated whether the functionality of genetic variants would differ according to their inheritance pattern using functional prediction scores like CADD, SIFT, and PhyloP. Variants from DDD and SFARI were also compared for validation. This analysis revealed that functional prediction scores for recessive variants tend to be lower than those of dominant variants (between dominant and recessive variants, p = 0.11 for CADD, p = 2.2 × 10−4 for SIFT, p = 9.6 × 10−6 for PhyloP, and p = 6.6 × 10−7 for AA conservation; Figure 3B). This finding indicates that variants under recessive inheritance are less damaging and less critical in function, hence demonstrate little physiological effect on carriers.
In our previous study using 553 KND patients, we estimated that one in every 17 healthy individuals is a carrier for at least one pathogenic variant for a recessive genes represented in the KND cohort. This estimate remains unchanged using 1,180 patients (Lee et al., 2020), but with a patient set twice as large, we inferred that the power to predict carriers would substantially increase. We first collected a list of pathogenic LoF and missense variants from ClinVar and KND, and aggregated their population frequencies using the gnomAD East Asian and Korean Variant Archive [KOVA 2; 5,305 healthy Korean individual set (Lee et al., 2017)]. This provided us with an estimation of the number of carriers of recessive neurodevelopmental diseases in the general Korean population (Figure 4A; Supplementary Table S5). Among the 161 genes that carriers were found in KND1180 set, the estimation yields were variable by gene, ranging up to 1.2% of the general population for VPS13B, and no carriers were predicted for 23 genes (Figure 4A). Among the 138 genes that carriers were predicted, only 34 genes were previously found in KND553. On average, the estimation yield for KND1180 variants on the 34 overlapping genes were 1.9-fold higher than those determined for KND553 variants, implying that larger cohort size is critical for increased sensitivity (Figure 4B).
FIGURE 4. Ability to pre-determine NDD carrier status based on KND. (A) Heatmap displaying the number of KND patients carrying a causal variant, pathogenic variant frequency, and ability to predict carrier frequency on 161 genes. (B) Comparison of the ability to predict pathogenic variant carriers based on KND553 or KND1180 on the 34 overlapping genes.
NDDs show considerable variability at both phenotypic and genetic levels. We conducted WES analysis to reveal genetic etiology for 1,180 undiagnosed patients in the KND cohort. Previously reported diagnostic rates of WES vary substantially among studies, ranging from 25% to 56% (Yang et al., 2013; Iglesias et al., 2014; Lee et al., 2014; Yang et al., 2014; Wright et al., 2015; Retterer et al., 2016; Jalkh et al., 2019; Marinakis et al., 2021). Herein, we report that the diagnostic yield for definitive pathogenic variant findings in KND patients was 50.8%. Among the diagnosed patients, 33.4% carried inherited variants, demonstrating that a large portion of KND patients inherited pathogenic variants from healthy parents.
It is expected that exome reanalysis applying the latest versions of databases and using improved bioinformatic tools would increase diagnostic yield (Ewans et al., 2018; Epilepsy Genetics Initiative, 2019; Jalkh et al., 2019; Fung et al., 2020). We performed reanalysis of 291 patients from the KND553 set who remained without clear pathogenic variants. This reanalysis increased diagnostic yield from 47.5% to 53.4%, which can be attributed to a number of factors: newly discovered and deposited gene-disease associations in OMIM, increased coverage allowing identification of variants that may previously have been missed, filtering out in the initial analysis of synonymous variants affecting gene splicing of KAT6B (p.Pro1049=), and re-evaluation of previously analyzed variants (Figure 1; Tables 1, 2). Therefore, we also suggest that exome sequencing data should be periodically re-evaluated.
Since NDDs may variously be caused by alterations in genes with autosomal dominant, autosomal recessive, or X-linked inheritance modes, genotype-phenotype correlations are often difficult to establish. Also, we believe that studying the fundamental differences in genes that cause NDDs in recessive or dominant mode is crucial in understanding the mechanisms of NDD pathogenicity. As a first step, we analyzed the biological pathways of the KND genes to obtain systematic insights into the molecular mechanisms associated with different inheritance modes. The results revealed that dominant and recessive genes are most strongly associated with synaptic function and metabolic processes, respectively, implying that diseases can be caused through different molecular mechanisms according to their inheritance patterns. Moreover, we observed dominant and recessive gene sets to have opposite trends in pLI and O/E scores, which proved the differences in genetic architecture between these inheritance patterns. In addition, we found gene expression profiles to also reflected this fundamental difference. Profiling of brain expression patterns in GTEx and BrainSpan revealed the dominant gene sets to exhibit specific and increased expression in the brain compared to the recessive gene set, suggesting dominant genes to be more brain-specific. In addition to the GO and expression analyses, we investigated whether PPI data support an association of tissue-specific expression and function with inheritance mode. Tissue-specific PPI networks based on direct interactions have previously demonstrated biological relevance (Skinnider et al., 2021). Here, we observed that dominant genes to have more interactions specifically in brain tissue than recessive genes. In contrast, recessive genes tended to have interactions ubiquitous across all seven tissues. Therefore, combined biological studies including PPI networks, functional pathways and phenotype data may be effective in expanding our understanding of disease progression in NDD. We also investigated variant functional effects and found that variants with recessive pathogenic alleles were less deleterious than those having dominant alleles. This is well supported by the fact that parental carriers are mostly healthy, although recent large-scale analyses have revealed heterozygous carriers of rare diseases to harbor subtle effects in various aspects of individual health and reproductivity (Barton et al., 2021; Gardner et al., 2022).
The frequency of carriers varies among population groups and specific genetic conditions could be biased toward particular ethnic groups (Rozen et al., 1999; Lynch et al., 2004; Cao and Kan, 2013; Lazarin et al., 2013). Ethnic Koreans are an outbred population, and the culture has prohibited marriages between relatives and among members of family clans for more than 500 years (Deuchler, 1992). As a major tertiary clinical institution, SNUCH covers a large portion of rare NDD patients in the country. Therefore, this study provides an unprecedented opportunity to study the occurrence of recessive diseases in an outbred population. We estimated that 24.7% of patients in the KND1180 cohort were affected by recessive conditions, which allows us to use databases such as gnomAD East Asian and KOVA to calculate carrier frequencies for reported and predicted pathogenic variants in the general population. Our resulting carrier panel will have a sensitivity of 36.1%, which is not too much deviated from previous attempts on Chinese and Israeli populations (38.7% for well-defined recessive conditions and >30% for recessive retinal diseases, respectively) (Hanany et al., 2018; Chau et al., 2022).
As expected, the larger sample size of this cohort relative to the KND553 cohort resulted in a greater number of pathogenic genes and an increase in the reported disease-associated variants enrolled in ClinVar and OMIM. Although calculated carrier frequencies may differ from those observed in clinical practices, the findings from this study will provide genetic evidence for the utility of preconception carrier screening.
Recent efforts into genome-based diagnosis of rare Mendelian disorders have provided with many gene-disease relationships and understanding of disease pathophysiology. However, it has not been clearly elucidated whether there is any genetic feature that determine the modes of inheritance of sucj diseases. We took advantage of in-house as well as public patient genome data and found genetic features of recessive vs. dominant disorders. Furthermore, we demonstrate that we can utilize this understanding of recessive variants to carrier prediction to reduce future patients originated from rare recessive variants.
The variant data presented in the study are deposited in the repository (https://www.sysbiolab.org/knd1180).
The studies involving human participants were reviewed and approved by Seoul National University Hospital. Written informed consent to participate in this study was provided by the participants’ legal guardian/next of kin.
J-HC and MC designed the project. SL, SYK, MJK, JGY, BCL, WJK, KJK, JMK, AC, JSL, and J-HC provided clinical examinations for the participants. SP, SSJ, SL, MK, HS, HJ, SEH, Jean L, Jeongeun L, Jeongha L, and C-RL collected and analyzed the data. SP, MC, and J-HC wrote the article. All authors read and approved the final manuscript.
The study was supported in part by the National Research Foundation (2020M3E5D7086836 and 2014M3C9A2064686), the Korea Centers for Disease Control and Prevention (2021-ER0701-00 and 2017N-6901-00), and the Ministry of Health and Welfare (HI16C1986).
We thank the patients and families that participated in the study.
The authors declare that the research was conducted in the absence of any commercial or financial relationships that could be construed as a potential conflict of interest
All claims expressed in this article are solely those of the authors and do not necessarily represent those of their affiliated organizations, or those of the publisher, the editors and the reviewers. Any product that may be evaluated in this article, or claim that may be made by its manufacturer, is not guaranteed or endorsed by the publisher.
The Supplementary Material for this article can be found online at: https://www.frontiersin.org/articles/10.3389/fgene.2022.990015/full#supplementary-material
Abouelhoda, M., Sobahy, T., El-Kalioby, M., Patel, N., Shamseldin, H., Monies, D., et al. (2016). Clinical genomics can facilitate countrywide estimation of autosomal recessive disease burden. Genet. Med. 18, 1244–1249. doi:10.1038/gim.2016.37
Amberger, J. S., Bocchini, C. A., Schiettecatte, F., Scott, A. F., and Hamosh, A. (2015). OMIM.org: Online Mendelian Inheritance in Man (OMIM®), an online catalog of human genes and genetic disorders. Nucleic Acids Res. 43, D789–D798. doi:10.1093/nar/gku1205
Amberger, J. S., Bocchini, C. A., Scott, A. F., and Hamosh, A. (2019). OMIM.org: Leveraging knowledge across phenotype-gene relationships. Nucleic Acids Res. 47, D1038–D1043. doi:10.1093/nar/gky1151
Amberger, J. S., and Hamosh, A. (2017). Searching online mendelian inheritance in man (OMIM): A knowledgebase of human genes and genetic phenotypes. Curr. Protoc. Bioinforma. 58, 1–2. doi:10.1002/cpbi.27.1-1.2.12
Baird, P. A., Anderson, T. W., Newcombe, H. B., and Lowry, R. B. (1988). Genetic disorders in children and young adults: A population study. Am. J. Hum. Genet. 42, 677–693.
Barton, A. R., Hujoel, M. L. A., Mukamel, R. E., Sherman, M. A., and Loh, P.-R. (2021). A spectrum of recessiveness among Mendelian disease variants in UK Biobank. MedRxiv.
Broad Institute (2019). Picard toolkit [online]. Broad Institute, GitHub repository: Broad Institute. Available at: https://broadinstitute.github.io/picard/.
Cao, A., and Kan, Y. W. (2013). The prevention of thalassemia. Cold Spring Harb. Perspect. Med. 3, a011775. doi:10.1101/cshperspect.a011775
Chau, J. F. T., Yu, M. H. C., Chui, M. M. C., Yeung, C. C. W., Kwok, A. W. C., Zhuang, X., et al. (2022). Comprehensive analysis of recessive carrier status using exome and genome sequencing data in 1543 Southern Chinese. NPJ Genom. Med. 7, 23. doi:10.1038/s41525-022-00287-z
Cingolani, P., Patel, V. M., Coon, M., Nguyen, T., Land, S. J., Ruden, D. M., et al. (2012). Using Drosophila melanogaster as a model for genotoxic chemical mutational studies with a new program, SnpSift. Front. Genet. 3, 35. doi:10.3389/fgene.2012.00035
Deuchler, M. (1992). The confucian transformation of Korea : A study of society and ideology. Cambridge, Massachusetts: Harvard University Asia Center.
Epilepsy Genetics Initiative (2019). The Epilepsy Genetics Initiative: Systematic reanalysis of diagnostic exomes increases yield. Epilepsia 60, 797–806. doi:10.1111/epi.14698
Ewans, L. J., Schofield, D., Shrestha, R., Zhu, Y., Gayevskiy, V., Ying, K., et al. (2018). Whole-exome sequencing reanalysis at 12 months boosts diagnosis and is cost-effective when applied early in Mendelian disorders. Genet. Med. 20, 1564–1574. doi:10.1038/gim.2018.39
Fung, J. L. F., Yu, M. H. C., Huang, S., Chung, C. C. Y., Chan, M. C. Y., Pajusalu, S., et al. (2020). A three-year follow-up study evaluating clinical utility of exome sequencing and diagnostic potential of reanalysis. NPJ Genom. Med. 5, 37. doi:10.1038/s41525-020-00144-x
Gardner, E. J., Neville, M. D. C., Samocha, K. E., Barclay, K., Kolk, M., Niemi, M. E. K., et al. (2022). Reduced reproductive success is associated with selective constraint on human genes. Nature 603, 858–863. doi:10.1038/s41586-022-04549-9
GTEx Consortium (2020). The GTEx Consortium atlas of genetic regulatory effects across human tissues. Science 369, 1318–1330. doi:10.1126/science.aaz1776
Hanany, M., Allon, G., Kimchi, A., Blumenfeld, A., Newman, H., Pras, E., et al. (2018). Carrier frequency analysis of mutations causing autosomal-recessive-inherited retinal diseases in the Israeli population. Eur. J. Hum. Genet. 26, 1159–1166. doi:10.1038/s41431-018-0152-0
Iglesias, A., Anyane-Yeboa, K., Wynn, J., Wilson, A., Truitt Cho, M., Guzman, E., et al. (2014). The usefulness of whole-exome sequencing in routine clinical practice. Genet. Med. 16, 922–931. doi:10.1038/gim.2014.58
Jalkh, N., Corbani, S., Haidar, Z., Hamdan, N., Farah, E., Abou Ghoch, J., et al. (2019). The added value of WES reanalysis in the field of genetic diagnosis: Lessons learned from 200 exomes in the Lebanese population. BMC Med. Genomics 12, 11. doi:10.1186/s12920-019-0474-y
Jimenez-Sanchez, G., Childs, B., and Valle, D. (2001). Human disease genes. Nature 409, 853–855. doi:10.1038/35057050
Kaback, M. M. (2001). Screening and prevention in tay-sachs disease: Origins, update, and impact. Adv. Genet. 44, 253–265. doi:10.1016/s0065-2660(01)44084-3
Kang, H. J., Kawasawa, Y. I., Cheng, F., Zhu, Y., Xu, X., Li, M., et al. (2011). Spatio-temporal transcriptome of the human brain. Nature 478, 483–489. doi:10.1038/nature10523
Kuperberg, M., Lev, D., Blumkin, L., Zerem, A., Ginsberg, M., Linder, I., et al. (2016). Utility of whole exome sequencing for genetic diagnosis of previously undiagnosed pediatric neurology patients. J. Child. Neurol. 31, 1534–1539. doi:10.1177/0883073816664836
Landrum, M. J., Lee, J. M., Benson, M., Brown, G., Chao, C., Chitipiralla, S., et al. (2016). ClinVar: Public archive of interpretations of clinically relevant variants. Nucleic Acids Res. 44, D862–D868. doi:10.1093/nar/gkv1222
Lazarin, G. A., Haque, I. S., Nazareth, S., Iori, K., Patterson, A. S., Jacobson, J. L., et al. (2013). An empirical estimate of carrier frequencies for 400+ causal mendelian variants: Results from an ethnically diverse clinical sample of 23, 453 individuals. Genet. Med. 15, 178–186. doi:10.1038/gim.2012.114
Lee, H., Deignan, J. L., Dorrani, N., Strom, S. P., Kantarci, S., Quintero-Rivera, F., et al. (2014). Clinical exome sequencing for genetic identification of rare Mendelian disorders. Jama 312, 1880–1887. doi:10.1001/jama.2014.14604
Lee, S., Seo, J., Park, J., Nam, J. Y., Choi, A., Ignatius, J. S., et al. (2017). Korean variant archive (KOVA): A reference database of genetic variations in the Korean population. Sci. Rep. 7, 4287. doi:10.1038/s41598-017-04642-4
Lee, Y., Park, S., Lee, J. S., Kim, S. Y., Cho, J., Yoo, Y., et al. (2020). Genomic profiling of 553 uncharacterized neurodevelopment patients reveals a high proportion of recessive pathogenic variant carriers in an outbred population. Sci. Rep. 10, 1413. doi:10.1038/s41598-020-58101-8
Lek, M., Karczewski, K. J., Minikel, E. V., Samocha, K. E., Banks, E., Fennell, T., et al. (2016). Analysis of protein-coding genetic variation in 60, 706 humans. Nature 536, 285–291. doi:10.1038/nature19057
Li, H., and Durbin, R. (2010). Fast and accurate long-read alignment with Burrows-Wheeler transform. Bioinformatics 26, 589–595. doi:10.1093/bioinformatics/btp698
Li, H., Handsaker, B., Wysoker, A., Fennell, T., Ruan, J., Homer, N., et al. (2009). The sequence alignment/map format and SAMtools. Bioinformatics 25, 2078–2079. doi:10.1093/bioinformatics/btp352
Lynch, H. T., Rubinstein, W. S., and Locker, G. Y. (2004). Cancer in jews: Introduction and overview. Fam. Cancer 3, 177–192. doi:10.1007/s10689-004-9538-y
Marinakis, N. M., Svingou, M., Veltra, D., Kekou, K., Sofocleous, C., Tilemis, F. N., et al. (2021). Phenotype-driven variant filtration strategy in exome sequencing toward a high diagnostic yield and identification of 85 novel variants in 400 patients with rare Mendelian disorders. Am. J. Med. Genet. A 185, 2561–2571. doi:10.1002/ajmg.a.62338
Mckenna, A., Hanna, M., Banks, E., Sivachenko, A., Cibulskis, K., Kernytsky, A., et al. (2010). The genome analysis toolkit: A MapReduce framework for analyzing next-generation DNA sequencing data. Genome Res. 20, 1297–1303. doi:10.1101/gr.107524.110
Ng, P. C., and Henikoff, S. (2003). Sift: Predicting amino acid changes that affect protein function. Nucleic Acids Res. 31, 3812–3814. doi:10.1093/nar/gkg509
Rentzsch, P., Witten, D., Cooper, G. M., Shendure, J., and Kircher, M. (2019). Cadd: Predicting the deleteriousness of variants throughout the human genome. Nucleic Acids Res. 47, D886–D894. doi:10.1093/nar/gky1016
Retterer, K., Juusola, J., Cho, M. T., Vitazka, P., Millan, F., Gibellini, F., et al. (2016). Clinical application of whole-exome sequencing across clinical indications. Genet. Med. 18, 696–704. doi:10.1038/gim.2015.148
Rozen, P., Shomrat, R., Strul, H., Naiman, T., Karminsky, N., Legum, C., et al. (1999). Prevalence of the I1307K APC gene variant in Israeli Jews of differing ethnic origin and risk for colorectal cancer. Gastroenterology 116, 54–57. doi:10.1016/s0016-5085(99)70228-3
Schuster-Böckler, B., and Bateman, A. (2008). Protein interactions in human genetic diseases. Genome Biol. 9, R9. doi:10.1186/gb-2008-9-1-r9
Siepel, A., Bejerano, G., Pedersen, J. S., Hinrichs, A. S., Hou, M., Rosenbloom, K., et al. (2005). Evolutionarily conserved elements in vertebrate, insect, worm, and yeast genomes. Genome Res. 15, 1034–1050. doi:10.1101/gr.3715005
Skinnider, M. A., Scott, N. E., Prudova, A., Kerr, C. H., Stoynov, N., Stacey, R. G., et al. (2021). An atlas of protein-protein interactions across mouse tissues. Cell 184, 4073–4089.e17. e17. doi:10.1016/j.cell.2021.06.003
Srivastava, S., Cohen, J. S., Vernon, H., Barañano, K., Mcclellan, R., Jamal, L., Naidu, S., and Fatemi, A. (2014). Clinical whole exome sequencing in child neurology practice. Ann. Neurol. 76, 473–483. doi:10.1002/ana.24251
Stenson, P. D., Ball, E. V., Mort, M., Phillips, A. D., Shiel, J. A., Thomas, N. S., et al. (2003). Human gene mutation database (HGMD): 2003 update. Hum. Mutat. 21, 577–581. doi:10.1002/humu.10212
Trujillano, D., Bertoli-Avella, A. M., Kumar Kandaswamy, K., Weiss, M. E., Köster, J., Marais, A., et al. (2017). Clinical exome sequencing: Results from 2819 samples reflecting 1000 families. Eur. J. Hum. Genet. 25, 176–182. doi:10.1038/ejhg.2016.146
Verma, I. C., and Puri, R. D. (2015). Global burden of genetic disease and the role of genetic screening. Semin. Fetal Neonatal Med. 20, 354–363. doi:10.1016/j.siny.2015.07.002
Wang, K., Li, M., and Hakonarson, H. (2010). Annovar: Functional annotation of genetic variants from high-throughput sequencing data. Nucleic Acids Res. 38, e164. doi:10.1093/nar/gkq603
Wright, C. F., Fitzgerald, T. W., Jones, W. D., Clayton, S., Mcrae, J. F., Van Kogelenberg, M., et al. (2015). Genetic diagnosis of developmental disorders in the DDD study: A scalable analysis of genome-wide research data. Lancet 385, 1305–1314. doi:10.1016/S0140-6736(14)61705-0
Yang, Y., Muzny, D. M., Reid, J. G., Bainbridge, M. N., Willis, A., Ward, P. A., et al. (2013). Clinical whole-exome sequencing for the diagnosis of mendelian disorders. N. Engl. J. Med. 369, 1502–1511. doi:10.1056/NEJMoa1306555
Yang, Y., Muzny, D. M., Xia, F., Niu, Z., Person, R., Ding, Y., et al. (2014). Molecular findings among patients referred for clinical whole-exome sequencing. Jama 312, 1870–1879. doi:10.1001/jama.2014.14601
OMIM the Online Mendelian Inheritance in Men
GoF gain-of-function
LoF loss-of-function
NDD neurodevelopmental diseases
WES whole exome sequencing
KND Korean Neurodevelopmental Disorder
DDD Deciphering Developmental Delay
SFARI Simons Foundation Autism Research Initiative
SNUH the Seoul National University Hospital
BWA Burrows-wheeler Aligner
GATK Genome Analysis Toolkit
TPM Transcripts Per Million
GTEx the Genotype-Tissue Expression
PPI Protein-protein interaction
CNV Copy number variation
GO Gene ontology
pLI the probability of loss of function intolerance
O/E the observed/expected
KOVA Korean Variant Archive
SNUCH the Seoul National University Children’s Hospital
ID intellectual disability
GDD global developmental delay
CHARGE coloboma, heart defects, atresia choanae, growth retardation, genital abnormalities, and ear abnormalities
FD facial dysmorphism
EE epileptic encephalopathy
BP biological process
CC cellular component
MF molecular function
DO disease ontology
AD autosomal dominant
AR autosomal recessive
XL X-linked
Keywords: neurodevelopmental disorder, inheritance pattern, carrier prediction, whole exome sequencing, recessive disorders
Citation: Park S, Jang SS, Lee S, Kim M, Sim H, Jeon H, Hong SE, Lee J, Lee J, Jeon EY, Lee J, Lee C-R, Kim SY, Kim MJ, Yoon JG, Lim BC, Kim WJ, Kim KJ, Ko JM, Cho A, Lee JS, Choi M and Chae J-H (2022) Systematic analysis of inheritance pattern determination in genes that cause rare neurodevelopmental diseases. Front. Genet. 13:990015. doi: 10.3389/fgene.2022.990015
Received: 09 July 2022; Accepted: 23 August 2022;
Published: 12 September 2022.
Edited by:
Ruth Roberts, ApconiX, United KingdomReviewed by:
Ngoc-Lan Nguyen,Vietnam Academy of Science and Technology, VietnamCopyright © 2022 Park, Jang, Lee, Kim, Sim, Jeon, Hong, Lee, Lee, Jeon, Lee, Lee, Kim, Kim, Yoon, Lim, Kim, Kim, Ko, Cho, Lee, Choi and Chae. This is an open-access article distributed under the terms of the Creative Commons Attribution License (CC BY). The use, distribution or reproduction in other forums is permitted, provided the original author(s) and the copyright owner(s) are credited and that the original publication in this journal is cited, in accordance with accepted academic practice. No use, distribution or reproduction is permitted which does not comply with these terms.
*Correspondence: Murim Choi, bXVyaW1jaG9pQHNudS5hYy5rcg==; Jong-Hee Chae, Y2hhZXBlZDFAc251LmFjLmty
†These authors share senior authorship
Disclaimer: All claims expressed in this article are solely those of the authors and do not necessarily represent those of their affiliated organizations, or those of the publisher, the editors and the reviewers. Any product that may be evaluated in this article or claim that may be made by its manufacturer is not guaranteed or endorsed by the publisher.
Research integrity at Frontiers
Learn more about the work of our research integrity team to safeguard the quality of each article we publish.