- 1Department of General Surgery, Weifang People’s Hospital, Shandong, China
- 2The Second Department of Oncology, Beidahuang Industry Group General Hospital, Harbin, China
- 3Heilongjiang Second Cancer Hospital, Harbin, China
- 4Geneis Beijing Co., Ltd., Beijing, China
- 5Department of Oncology, Hainan General Hospital, Haikou, China
Colon cancer and colorectal cancer are two common cancer-related deaths worldwide. Identification of potential biomarkers for the two cancers can help us to evaluate their initiation, progression and therapeutic response. In this study, we propose a new microRNA-disease association identification method, BNNRMDA, to discover potential microRNA biomarkers for the two cancers. BNNRMDA better combines disease semantic similarity and Gaussian Association Profile Kernel (GAPK) similarity, microRNA function similarity and GAPK similarity, and the bound nuclear norm regularization model. Compared to other five classical microRNA-disease association identification methods (MIDPE, MIDP, RLSMDA, GRNMF, AND LPLNS), BNNRMDA obtains the highest AUC of 0.9071, demonstrating its strong microRNA-disease association identification performance. BNNRMDA is applied to discover possible microRNA biomarkers for colon cancer and colorectal cancer. The results show that all 73 known microRNAs associated with colon cancer in the HMDD database have the highest association scores with colon cancer and are ranked as top 73. Among 137 known microRNAs associated with colorectal cancer in the HMDD database, 129 microRNAs have the highest association scores with colorectal cancer and are ranked as top 129. In addition, we predict that hsa-miR-103a could be a potential biomarker of colon cancer and hsa-mir-193b and hsa-mir-7days could be potential biomarkers of colorectal cancer.
1 Introduction
Cancers are seriously threatening and endangering human health (Yang et al., 2013; Liu et al., 2021; Yang et al., 2022). Colon cancer and colorectal cancer are two of leading causes of cancer-related deaths worldwide (Lee et al., 2018; Piawah and Venook, 2019). Patients with colon cancer only have a survival rate of 10% when diagnosed at late stage. More importantly, colon cancer shows a higher incidence rate in elder populations. The survival rate of patients with colon cancer is densely associated with the size, location, and stage of the tumor. Metastasis may be the leading cause of deaths for patients suffered from late-stage colon cancer. Thus, understanding the mechanisms of colon cancer could contribute to designing more strong therapeutic options (Ma et al., 2021).
Nowadays, patients with colorectal cancer show a younger trend. In the last decade, incidence rates and death rates of colorectal cancers separately increased by 22 and 13% among adults under 50 years in the United State. However, their precise aetiologic factors still remain unknown. Many evidence demonstrate that early screening of colorectal cancer can reduce their incidence and mortality. Thus, the identification of diagnosis or prognosis biomarkers can contribute to assessment of tumour initiation, progression and therapeutic response for colorectal cancer (Sampath et al., 2021).
Many researches show that numerous RNA data play important roles in the development and metastasis of various diseases including cancers and COVID-19 (Huang et al., 2017; Peng L. et al., 2020; Xu et al., 2020; Yang et al., 2020; Zhang et al., 2021; Peng L. et al., 2022; Shen et al., 2022; Tian et al., 2022). In particular, noncoding RNAs could be biomarkers to boost drug design (Liu et al., 2020; Meng et al., 2022). For example, lncRNAs and circRNAs have been used as biomarkers of cancers (Peng et al., 2021a; Peng et al., 2021b; Chen et al., 2021; Li et al., 2021; Verduci et al., 2021; Wang et al., 2021; Peng L. H. et al., 2022). MicroRNAs (miRNAs) are a class of small non-coding RNAs with 22–24 nucleotides in length (Li et al., 2018; Chen et al., 2020). MicroRNAs can bind to mRNAs of target genes to inhibit expression of these genes. In addition, a few microRNAs may suppress tumors while other microRNAs may affect the progression and metastasis of tumors.
The dysfunction of microRNAs is densely linked to the inflammation of colon cancer. For example, Ma et al. (Ma et al., 2021) found that M2 macrophage-derived exosomal miR-155-5p may have an association with the immune escape of cells in colon cancer. Pagotto et al. (Pagotto et al., 2022) observed that the miR-483 gene could have a responsive to glucose availability for colon cancer. Miao et al. (Miao et al., 2021) identified that miR-4284 could be a therapeutic target in colon cancer. Dougherty et al. (Dougherty et al., 2021) inferred that the upregulations of microRNA-143 and microRNA-145 have close linkages with colonocytes suppresses colitis and inflammation-related colon cancer. Zhang et al. (Zhang et al., 2021) suggested that microRNA-24-3p could heighten the resistance of colon cancer cell to MTX. Yue et al. (Yue et al., 2021) reported that NEDD4 could trigger colon cancer progression through microRNA-340-5p suppression. In summary, the identification of microRNAs in the blood, tissues, and faecal matter will help us use these microRNA as biomarkers in early detection of colon cancer and thus design strong targeted therapeutic strategies for inflammation-mediated colon cancer (Peng et al., 2018; Sampath et al., 2021).
More importantly, microRNAs densely link to the carcinogenic process of colorectal cancer. For example, microRNA-143-3p can limit colorectal cancer metastases (Guo et al., 2019), microRNA-375-3p can boost chemosensitivity to 5-fluorouracil through targeting thymidylate synthase in colorectal cancer (Xu et al., 2020), microRNA-451a influences colorectal cancer proliferation (Ruhl et al., 2018), and microRNA-146a can inhibit tumorigenic inflammation of colorectal cancer (Garo et al., 2021). Biomarkers are an important strategy in early screening, prognostication, survival, and treatment response prediction for cancers. Therefore, microRNAs have been explored as biomarkers in colorectal cancer (Peng LH. et al., 2020; Ogunwobi et al., 2020).
Recently, many researchers have been devoted to microRNA biomarker identification for cancer including colon cancer and colorectal cancer by computational microRNA-disease association prediction (Peng et al., 2017; Li et al., 2021). Huang et al. (Huang et al., 2021) innovatively represented microRNA-disease-type triples as a tensor and further designed a tensor decomposition model to detect new microRNA-disease associations. Li et al. (Li et al., 2021) considered that the abnormal expression of microRNAs is densely associated with the evolution and progression of human diseases and inferred disease-related microRNAs as new biomarkers through a graph auto-encoder model. Chen et al. (Chen et al., 2021) designed a deep learning model for microRNA-disease association identification based on deep belief network. Wang et al. (2022)) pretrained a stacked autoencoder to predict potential microRNA-disease associations in an unsupervised manner. These methods effectively improved microRNA biomarker identification of human complex diseases.
In this study, we design a MicroRNA-Disease Association prediction algorithm (BNNRMDA) to find potential microRNA biomarkers for colon cancer and colorectal cancer based on disease semantic similarity, microRNA functional similarity, Gaussian association profile kernel (GAPK) similarity, and the Bound Nuclear Norm Regularization model.
2 Materials and methods
2.1 Data
2.1.1 Dataset
Experimentally confirmed microRNA-disease association data can be downloaded from the HMDD database provided by Li et al. (Li et al., 2014). The hierarchical structures between diseases can be downloaded from the MeSH database (https://www.nlm.nih.gov/mesh/). Experimentally supported microRNA-gene interactions can be downloaded from TarBase (Vergoulis et al., 2012), miRTarBase (Hsu et al., 2014), and miRecords (Xiao et al., 2009). We acquired microRNA-disease associations between 495 microRNAs and 378 diseases, hierarchical structures for 4,663 diseases, and 38,089 microRNA-gene interactions between 477 microRNAs and 12,422 genes. Finally, we obtained 4,791 associations between 353 microRNAs and 327 diseases after removing microRNAs without target genes and diseases without hierarchical structures.
2.1.2 Disease semantic similarity
For a known disease
where
2.1.3 MicroRNA functional similarity
MicroRNA similarity can be computed based on microRNA-gene associations and gene functional network. First, the associated log-likelihood scores
Second,
where
Third, similarity between
where
Finally, the functional similarity between two microRNAs
where
2.1.4 GAPK similarity
For a known disease
where
Similarly, for a known microRNA
where
2.1.5 Similarity fusion
Disease semantic similarity
where the parameter
MicroRNA functional similarity
where the parameter
2.2 Heterogeneous microRNA-disease network construction
A heterogeneous microRNA-disease network is created by fusing microRNA similarity network, disease similarity network, and microRNA-disease association network. Each edge in similarity network is weighted based on the computed similarity. The heterogeneous microRNA-disease network can be described using a bipartite graph
where
2.3 BNNRMDA model
In known microRNA-disease association dataset, majority of microRNA-disease pairs are unknown-associated. Inspired by the bound nuclear norm regularization model provided by Yang et al. (Yang et al., 2019), in this study, we design the bounded nuclear norm regularization-based MDA prediction method to score each unknown microRNA-disease pair. We describe microRNA-disease association inference as a matrix completion problem and construct model (12) to predict new microRNA-disease associations in microRNA-disease association matrix:
where
Model (12) is a non-convex model and difficult to solve. Thus, we transform it to a nuclear norm model through the nuclear norm optimization method proposed by Candes et al. (2013) by Eq. 14:
where
Because the value of each element in microRNA and disease similarity matrices
where
We introduce a soft regularization term to tolerate data noise considering the difficulty in selecting an appropriate parameter in Eq. 15. Consequently, a bound nuclear norm regularization model is built to infer potential microRNA-disease associations by Eq. 16:
where the parameter
Consequently, we introduce an auxiliary matrix
where
Thus, the corresponding augmented Lagrange function is written as Eq. 18:
where
At the
3 Experiments
3.1 Experimental settings and evaluation
In this study, we perform five-fold cross validation for 10 times to investigate the microRNA-disease association inference ability of BNNRMDA. During five-fold cross validation, 80% of elements in microRNA-disease association matrix
3.2 Performance measurement
To measure the microRNA-disease association prediction performance of BNNRMDA, we compare it with MIDPE (Xuan et al., 2015), MIDP (Xuan et al., 2015), RLSMDA (Chen and Yan, 2014), GRNMF (Xiao et al., 2018), and LPLNS (Li et al., 2018). MIDP (Xuan et al., 2015) and MIDPE (Xuan et al., 2015) are two random walk-based microRNA-disease association prediction methods. MIDP is used to detect association information for microRNAs related to diseases. MIDPE is used to detect association information through the bilayer network. RLSMDA (Chen and Yan, 2014) is a semi-supervised learning-based microRNA-disease association inference framework. GRNMF (Xiao et al., 2018) is a graph regularized non-negative matrix factorization-based microRNA-disease association prediction model. In addition, GRNMF built an association probability profile for each disease or miRNA based on a weighted nearest
From Table 1, we can find that BNNRMDA obtains better AUC of 0.9071 than MIDPE, MIDP, RLSMDA, GRNMF, and LPLNS. Compared to MIDPE, MIDP, RLSMDA, GRNMF, and LPLNS, BNNRMDA increases the performance of 13.79, 8.98, 5.69, 1.19, and 0.41% based on the AUC value, respectively. The results show that our proposed BNNRMDA method can effectively predict new microRNA-disease associations.
3.3 Case study
In the above section, we have computed the performance of BNNRMDA. The results show that BNNRMDA obtains better AUC and outperforms other five microRNA-disease association prediction methods. We continue to implement case analyses to identify possible microRNA biomarkers for colon cancer and colorectal cancer.
3.3.1 Inferring possible microRNA biomarkers for colon cancer
Colon cancer is a common malignant tumor and has a very high incidence rate in adult with age of 40–50 (Zhu et al., 2020; Liu et al., 2021). More importantly, it has no any symptoms in the early stage. Therefore, it is important to infer possible biomarkers to boost the diagnosis and treatment for colon cancer (Liu et al., 2021). Among the HMDD dataset, there are 73 known microRNAs associated with colon cancer among 353 microRNAs. Based on the proposed BNNRMDA method, we compute the association score for each microRNA-disease pair. The results show that all 73 known microRNAs associated with colon cancer in the HMDD database have the highest association scores with colon cancer and are ranked as top 73. We continue to investigate the following 30 miRNAs that have higher association scores with colon cancer and are ranked as 74–103. The results are shown in Table 2 and Figure 1. From Table 2 and Figure 1, we can find that 18 microRNAs are confirmed to associate with colon cancer by literature retrieval. In addition, 12 microRNAs are inferred to associate with colon cancer and are potential biomarkers of colon cancer.
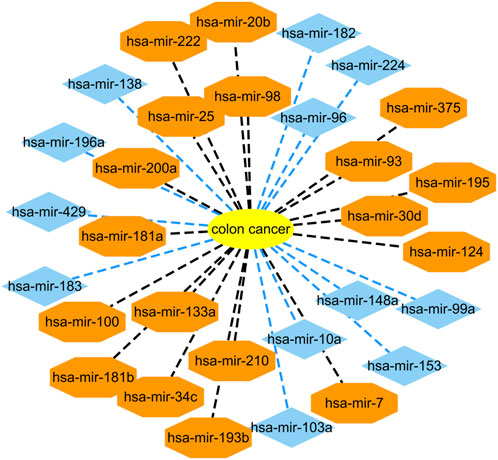
FIGURE 1. Associations between the predicted top 30 microRNAs and colon cancer except for known 73 microRNA-colon cancer associations in the HMDD database that are predicted to have the highest association scores with colon cancer. Black dot lines denote associations between microRNAs and colon cancer and these associations have been reported by publications. Blue dot lines denote associations between microRNAs and colon cancer and these associations are unknown and need to experimental validation.
In addition, we infer that microRNA hsa-mir-103a may associate with colon cancer. Wnt signaling pathway is hyper-activated in many human cancers. Therefore, Wnt pathway demonstrates promising diagnostic and therapeutic effect in cancer medicine. Fasihi et al. (2018) found that hsa-miR-103a may be a possible regulator of Wnt signaling pathway by detecting its effect on Wnt pathway components in colorectal cancer-originated cell lines and its expression in colorectal cancer tissues. They also found that hsa-miR-103a has an upregulation function in colorectal cancer tissues through RT-qPCR and its overexpression could cause elevated Wnt activity. Therefore, we infer that hsa-miR-103a could be a potential biomarker of colon cancer (Fasihi et al., 2017).
3.3.2 Inferring possible microRNA biomarkers for colorectal cancer
Colorectal cancer is the third leading cause of cancer-related deaths in the United States. In the United State, there are about 1.85 million cases and 850 thousand deaths annually. In 2020, there are 53,200 colorectal cancer deaths in the United State. Among new colorectal cancer diagnoses, approximately 20% of patients suffered from metastatic disease and approximately 25% of patients suffered from localized disease that may later develop metastases. Of patients who are diagnosed as metastatic colorectal cancer, about 70–75% of patients survive more than 1 year, about 30–35% patients survive more than 3 years, and less than 20% patients survive more than 5 years (Xie et al., 2020; Biller and Schrag, 2021).
Among the HMDD dataset, there are 137 known microRNAs associated with colorectal cancer among 353 microRNAs. Based on the proposed BNNRMDA method, we compute the association score for each microRNA-colorectal cancer pair. The results show that 129 known microRNAs associated with colorectal cancer in the HMDD database have the highest association scores with colorectal cancer and are ranked as top 129. We continue to investigate the following 30 miRNAs that have higher association scores with colorectal cancer and are ranked as 130–159. The results are shown in Table 3 and Figure 2. From Table 3 and Figure 2, we can find that 8 microRNAs are known to associate with colorectal cancer in the HMDD database. In addition, the remaining 22 microRNAs are inferred to associate with colorectal cancer and are reported by publications. The results confirm the strong microRNA identification performance of BNNRMDA for colorectal cancer. In addition, we predict that hsa-mir-193b and hsa-mir-7 days may associate with colorectal cancer and need validation.
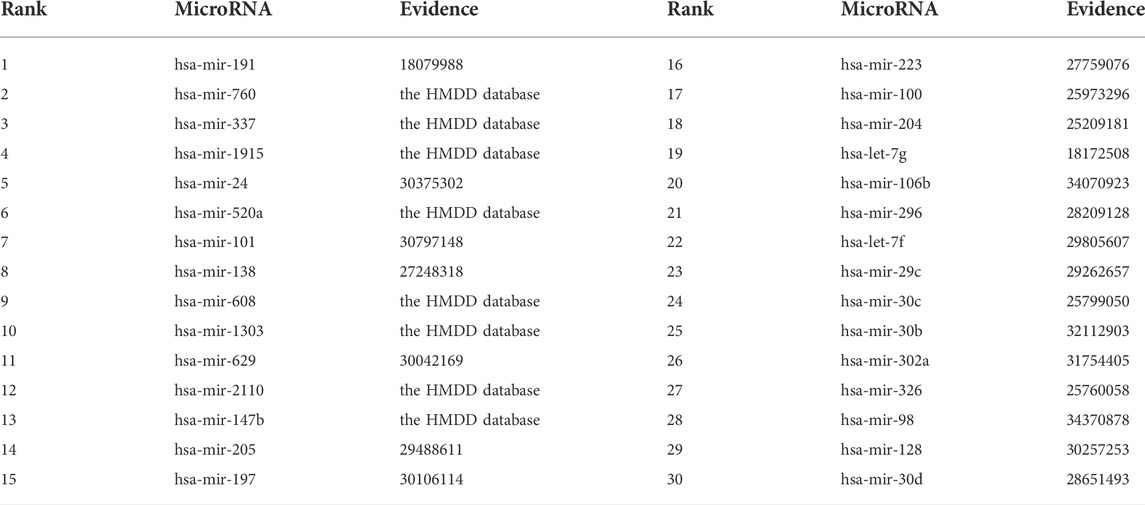
TABLE 3. The inferred top 30 microRNAs associated with colorectal cancer except for 129 known microRNAs.
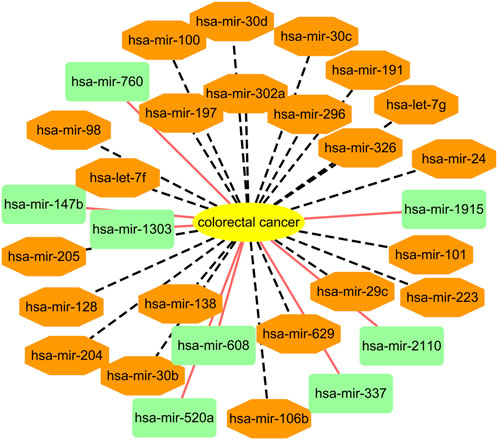
FIGURE 2. Associations between the predicted top 30 microRNAs and colorectal cancer except for known 129 microRNA-colorectal cancer associations in the HMDD database. Black dot lines denote associations between microRNAs and colorectal cancer and these associations have been reported by publications. Orange solid lines denote associations between microRNAs and colorectal cancer and these associations are unknown and need to experimental validation.
4 Conclusion
Colon cancer and colorectal cancer are two of leading causes of cancer-related deaths worldwide and are seriously threatening human health. Inference of diagnosis or prognosis biomarkers for colon cancer and colorectal cancer can help to evaluate their initiation, progression and therapeutic response. In this study, we developed a new microRNA-disease association prediction method, BNNRMDA, to find possible microRNA biomarkers for colon cancer and colorectal cancer. BNNRMDA effectively integrated disease semantic similarity and GAPK similarity, microRNA function similarity and GAPK similarity, and bound nuclear norm regularization.
Compared to other five classical microRNA-disease association prediction methods, BNNRMDA obtains the best AUC of 0.9071, demonstrating its powerful microRNA-disease association prediction performance. We continue to use the proposed BNNRMDA method for finding possible microRNA biomarkers for colon cancer and colorectal cancer. The results show that hsa-miR-103a could be a potential biomarker of colon cancer and hsa-mir-193b and hsa-mir-7 days could be potential biomarkers of colorectal cancer.
Our proposed BNNRMDA method fully considers the affect of Gaussian association profile similarity on the prediction performance. In addition, the bound nuclear norm regularization approach can effectively learn the intrinsic distribution of data. Therefore, BNNRMDA significantly outperform other MDA prediction methods. Although BNNRMDA obtains better AUC, its performance including AUC, precision, recall, and accuracy need to further improve. In the future, we will improve the bound nuclear norm regularization model to discover possible biomarkers for colon cancer and colorectal cancer.
Data availability statement
The original contributions presented in the study are included in the article/supplementary material, further inquiries can be directed to the corresponding author.
Author contributions
Conceptualization: S-YZ and CQ; Methodology: S-YZ, X-LL and CQ; Project administration: CQ, YW, X-LS and B-BJ; Software: S-YZ, X-LL and CQ; Writing-original draft: S-YZ, X-LL and CQ; Writing-review and editing: S-YZ, and CQ.
Funding
This work was supported by the Medical and Health Science Technology Development Program in Shandong Province (202104080159) and Science Technology Development Program in Weifang City (2021YX007).
Conflict of interest
Authors YW, XS, and BJ were employed by the company Geneis (Beijing) Co. Ltd. In addition, this manuscript was conducted by a multicenter study initiated by the corresponding author (CQ). Geneis Beijing Co., Ltd. Contacted the doctors in hospitals around the country.
The remaining authors declare that the research was conducted in the absence of any commercial or financial relationships that could be construed as a potential conflict of interest.
Publisher’s note
All claims expressed in this article are solely those of the authors and do not necessarily represent those of their affiliated organizations, or those of the publisher, the editors and the reviewers. Any product that may be evaluated in this article, or claim that may be made by its manufacturer, is not guaranteed or endorsed by the publisher.
References
Biller, L. H., and Schrag, D. (2021). Diagnosis and treatment of metastatic colorectal cancer: A review. Jama 325 (7), 669–685. doi:10.1001/jama.2021.0106
Candes, E., and Recht, B. (2013). Simple bounds for recovering low-complexity models[J]. Mathematical Programming 141 (1), 577–589.
Chen, X., and Yan, G. Y. (2014). Semi-supervised learning for potential human microRNA-disease associations inference[J]. Scientific reports 4 (1), 1–10.
Chen, B., Xia, Z., Deng, Y. N., Yang, Y., Zhang, P., Zhu, H., et al. (2019). Emerging microRNA biomarkers for colorectal cancer diagnosis and prognosis. Open Biol. 9 (1), 180212. doi:10.1098/rsob.180212
Chen, H., Guo, R., Li, G., Zhang, W., and Zhang, Z. (2020). Comparative analysis of similarity measurements in miRNAs with applications to miRNA-disease association predictions. BMC Bioinforma. 21 (1), 176–214. doi:10.1186/s12859-020-3515-9
Chen, S., Yang, X., Yu, C., Zhou, W., Xia, Q., Liu, Y., et al. (2021). The potential of circRNA as a novel diagnostic biomarker in cervical cancer. J. Oncol. 2021, 2021–2026. doi:10.1155/2021/5529486
Chen, X., Li, T. H., Zhao, Y., Wang, C. C., and Zhu, C. C. (2021). Deep-belief network for predicting potential miRNA-disease associations. Brief. Bioinform. 22 (3), bbaa186. doi:10.1093/bib/bbaa186
Dougherty, U., Mustafi, R., Zhu, H., Zhu, X., Deb, D., Meredith, S. C., et al. (2021). Upregulation of polycistronic microRNA-143 and microRNA-145 in colonocytes suppresses colitis and inflammation-associated colon cancer. Epigenetics 16 (12), 1317–1334. doi:10.1080/15592294.2020.1863117
Fasihi, A. M., Soltani, B., and Atashi, A. (2018). Introduction of hsa–miR–103a and hsa–miR–1827 and hsa–miR–137 as new regulators of Wnt signaling pathway and their relation to colorectal carcinoma[J]. J. cellular biochemistry 119 (7), 5104–5117.
Garo, L. P., Ajay, A. K., Fujiwara, M., Gabriely, G., Raheja, R., Kuhn, C., et al. (2021). MicroRNA-146a limits tumorigenic inflammation in colorectal cancer. Nat. Commun. 12 (1), 2419–2516. doi:10.1038/s41467-021-22641-y
Hsu, S. D., Tseng, Y. T., Shrestha, S., Lin, Y. L., Khaleel, A., Chou, C. H., et al. (2014). miRTarBase update 2014: an information resource for experimentally validated miRNA-target interactions. Nucleic Acids Res. 42 (D1), D78–D85. doi:10.1093/nar/gkt1266
Huang, F., Yue, X., Xiong, Z., Yu, Z., Liu, S., and Zhang, W. (2021). Tensor decomposition with relational constraints for predicting multiple types of microRNA-disease associations. Brief. Bioinform. 22 (3), bbaa140. doi:10.1093/bib/bbaa140
Huang, L., Li, X., Guo, P., Yao, Y., Liao, B., Zhang, W., et al. (2017). Matrix completion with side information and its applications in predicting the antigenicity of influenza viruses. Bioinformatics 33 (20), 3195–3201. doi:10.1093/bioinformatics/btx390
Lee, C. H., Im, E. J., Moon, P. G., and Baek, M. C. (2018). Discovery of a diagnostic biomarker for colon cancer through proteomic profiling of small extracellular vesicles. BMC cancer 18 (1), 1058–1111. doi:10.1186/s12885-018-4952-y
Lee, I., Blom, U. M., Wang, P. I., Shim, J. E., and Marcotte, E. M. (2011). Prioritizing candidate disease genes by network-based boosting of genome-wide association data. Genome Res. 21 (7), 1109–1121. doi:10.1101/gr.118992.110
Li, G., Luo, J., Xiao, Q., Liang, C., and Ding, P. (2018). Predicting microRNA-disease associations using label propagation based on linear neighborhood similarity. J. Biomed. Inf. 82, 169–177. doi:10.1016/j.jbi.2018.05.005
Li, T., Huang, T., Guo, C., Wang, A., Shi, X., Mo, X., et al. (2021). Genomic variation, origin tracing, and vaccine development of SARS-CoV-2: A systematic review. Innovation. 2 (2), 100116. doi:10.1016/j.xinn.2021.100116
Li, Y., Qiu, C., Tu, J., Geng, B., Yang, J., Jiang, T., et al. (2014). HMDD v2.0: A database for experimentally supported human microRNA and disease associations. Nucleic Acids Res. 42 (D1), D1070–D1074. doi:10.1093/nar/gkt1023
Li, Z., Li, J., Nie, R., et al. (2021). A graph auto-encoder model for miRNA-disease associations prediction[J]. Briefings Bioinforma. (4), 22, bbaa240. doi:10.1093/bib/bbaa240
Liu, C., Wei, D., Xiang, J., Ren, F., Huang, L., Lang, J., et al. (2020). An improved anticancer drug-response prediction based on an ensemble method integrating matrix completion and ridge regression. Mol. Ther. Nucleic Acids 21, 676–686. doi:10.1016/j.omtn.2020.07.003
Liu, D., Huang, Y., Nie, W., Zhang, J., and Deng, L. (2021). Smalf: miRNA-disease associations prediction based on stacked autoencoder and XGBoost. BMC Bioinforma. 22 (1), 219–318. doi:10.1186/s12859-021-04135-2
Liu, H., Qiu, C., Wang, B., Bing, P., Tian, G., Zhang, X., et al. (2021). Evaluating DNA methylation, gene expression, somatic mutation, and their combinations in inferring tumor tissue-of-origin. Front. Cell Dev. Biol. 9, 886. doi:10.3389/fcell.2021.619330
Ma, Y. S., Wu, T. M., Ling, C. C., Yu, F., Zhang, J., Cao, P. S., et al. (2021). M2 macrophage-derived exosomal microRNA-155-5p promotes the immune escape of colon cancer by downregulating ZC3H12B. Mol. Ther. Oncolytics 20, 484–498. doi:10.1016/j.omto.2021.02.005
Meng, Y., Lu, C., Jin, M., Xu, J., Zeng, X., and Yang, J. (2022). A weighted bilinear neural collaborative filtering approach for drug repositioning. Brief. Bioinform. 23, bbab581. doi:10.1093/bib/bbab581
Miao, X., Li, Z., Zhang, Y., and Wang, T. (2021). MicroRNA-4284 inhibits colon cancer epithelial-mesenchymal transition by down-regulating Perilipin 5. STEMedicine 2 (6), e85. doi:10.37175/stemedicine.v2i6.85
Ogunwobi, O. O., Mahmood, F., and Akingboye, A. (2020). Biomarkers in colorectal cancer: Current research and future prospects. Int. J. Mol. Sci. 21 (15), 5311. doi:10.3390/ijms21155311
Pagotto, S., Colorito, M. L., Nicotra, A., Apuzzo, T., Tinari, N., Protasi, F., et al. (2022). A perspective analysis: microRNAs, glucose metabolism, and drug resistance in colon cancer stem cells. Cancer Gene Ther. 29 (1), 4–9. doi:10.1038/s41417-021-00298-5
Peng, L., Chen, Y., Ma, N., and Chen, X. (2017). Narrmda: Negative-aware and rating-based recommendation algorithm for miRNA-disease association prediction. Mol. Biosyst. 13 (12), 2650–2659. doi:10.1039/c7mb00499k
Peng, L. H., Sun, C. N., Guan, N. N., Li, J. Q., and Chen, X. (2018). Hnmda: Heterogeneous network-based miRNA-disease association prediction. Mol. Genet. Genomics 293 (4), 983–995. doi:10.1007/s00438-018-1438-1
Peng, L. H., Zhou, L. Q., Chen, X., and Piao, X. (2020b). A computational study of potential miRNA-disease association inference based on ensemble learning and kernel ridge regression. Front. Bioeng. Biotechnol. 8, 40. doi:10.3389/fbioe.2020.00040
Peng, L., Tian, X., Shen, L., et al. (2020a). Drug designing and repositioning against severe acute respiratory coronavirus 2 (SARS-Cov-2) through computational simulation: Current progress and hopes. Front. Genet. 5, 1–5. doi:10.23880/oajmb-16000168
Peng, L., Wang, F., Wang, Z., Tan, J., Huang, L., Tian, X., et al. (2022a). Cell-cell communication inference and analysis in the tumour microenvironments from single-cell transcriptomics: Data resources and computational strategies. Brief. Bioinform. 23 (4), bbac234. doi:10.1093/bib/bbac234
Peng, L. H., Tan, J. W., Tian, X. F., et al. (2022b). EnANNDeep: An ensemble-based lncRNA–protein interaction prediction framework with adaptive k-nearest neighbor classifier and deep models[J]. Interdiscip. Sci. Comput. Life Sci. 14, 1–24.
Peng, L. H., Wang, C., Tian, X. F., Zhou, L. Q., and Li, K. Q. (2021b). Finding lncRNA-protein interactions based on deep learning with dual-net neural architecture. IEEE/ACM Trans. Comput. Biol. Bioinform. 29, 1. doi:10.1109/TCBB.2021.3116232
Peng, L. H., Yuan, R. Y., Shen, L., Gao, P. F., and Zhou, L. Q. (2021a). LPI-EnEDT: An ensemble framework with extra tree and decision tree classifiers for imbalanced lncRNA-protein interaction data classification[J]. BioData Min. 14 (1), 1–22.
Piawah, S., and Venook, A. P. (2019). Targeted therapy for colorectal cancer metastases: A review of current methods of molecularly targeted therapy and the use of tumor biomarkers in the treatment of metastatic colorectal cancer. Cancer 125 (23), 4139–4147. doi:10.1002/cncr.32163
Ruhl, R., Rana, S., Kelley, K., Espinosa-Diez, C., Hudson, C., Lanciault, C., et al. (2018). microRNA-451a regulates colorectal cancer proliferation in response to radiation. BMC cancer 18 (1), 517–519. doi:10.1186/s12885-018-4370-1
Sampath, S. S., Venkatabalasubramanian, S., and Ramalingam, S. (2021). Role of MicroRNAs in the progression and metastasis of colon cancer. Endocr. Metab. Immune Disord. Drug Targets 21 (1), 35–46. doi:10.2174/1871530320666200825184924
Shen, L., Liu, F., Huang, L., Liu, G., Zhou, L., and Peng, L. (2022). VDA-RWLRLS: An anti-SARS-CoV-2 drug prioritizing framework combining an unbalanced bi-random walk and Laplacian regularized least squares. Comput. Biol. Med. 140, 105119. doi:10.1016/j.compbiomed.2021.105119
Tian, X., Shen, L., Gao, P., Huang, L., Liu, G., Zhou, L., et al. (2022). Discovery of potential therapeutic drugs for COVID-19 through logistic matrix factorization with kernel diffusion. Front. Microbiol., 13, 740382. doi:10.3389/fmicb.2022.740382
Verduci, L., Tarcitano, E., Strano, S., Yarden, Y., and Blandino, G. (2021). CircRNAs: Role in human diseases and potential use as biomarkers. Cell Death Dis. 12 (5), 468–512. doi:10.1038/s41419-021-03743-3
Vergoulis, T., Vlachos, I. S., Alexiou, P., Georgakilas, G., Maragkakis, M., Reczko, M., et al. (2012). TarBase 6.0: Capturing the exponential growth of miRNA targets with experimental support. Nucleic Acids Res. 40 (D1), D222–D229. doi:10.1093/nar/gkr1161
Wang, D., Wang, J., Lu, M., Song, F., and Cui, Q. (2010). Inferring the human microRNA functional similarity and functional network based on microRNA-associated diseases. Bioinformatics 26 (13), 1644–1650. doi:10.1093/bioinformatics/btq241
Wang, S., Dong, Y., Gong, A., Kong, H., Gao, J., Hao, X., et al. (2021). Exosomal circRNAs as novel cancer biomarkers: Challenges and opportunities. Int. J. Biol. Sci. 17 (2), 562–573. doi:10.7150/ijbs.48782
Wang, C. C., Li, T. H., and Huang, L. (2022). Prediction of potential miRNA–disease associations based on stacked autoencoder[J]. Briefings in Bioinformatics 23 (2), 1–11.
Xiao, F., Zuo, Z., Cai, G., Kang, S., Gao, X., and Li, T. (2009). miRecords: an integrated resource for microRNA-target interactions. Nucleic Acids Res. 37 (1), D105–D110. doi:10.1093/nar/gkn851
Xiao, Q., Luo, J., and Liang, C. (2018). A graph regularized non-negative matrix factorization method for identifying microRNA-disease associations[J]. Bioinformatics 34 (2), 239–248.
Xie, Y. H., Chen, Y. X., and Fang, J. Y. (2020). Comprehensive review of targeted therapy for colorectal cancer[J]. Signal Transduct. Target. Ther. 5 (1), 1–30. doi:10.1038/s41392-020-0116
Xu, F., Ye, M. L., Zhang, Y. P., Li, W. J., Li, M. T., Wang, H. Z., et al. (2020). MicroRNA-375-3p enhances chemosensitivity to 5-fluorouracil by targeting thymidylate synthase in colorectal cancer. Cancer Sci. 111 (5), 1528–1541. doi:10.1111/cas.14356
Xu, J., Cai, L., Liao, B., Zhu, W., and Yang, J. (2020). CMF-impute: An accurate imputation tool for single-cell RNA-seq data. Bioinformatics 36 (10), 3139–3147. doi:10.1093/bioinformatics/btaa109
Xuan, P., Han, K., and Guo, Y. (2015). Prediction of potential disease-associated microRNAs based on random walk[J]. Bioinformatics 31 (11), 1805–1815.
Yang, J., Grünewald, S., and Wan, X. F. (2013). Quartet-net: A quartet-based method to reconstruct phylogenetic networks. Mol. Biol. Evol. 30 (5), 1206–1217. doi:10.1093/molbev/mst040
Yang, J., Ju, J., Guo, L., Ji, B., Shi, S., Yang, Z., et al. (2022). Prediction of HER2-positive breast cancer recurrence and metastasis risk from histopathological images and clinical information via multimodal deep learning. Comput. Struct. Biotechnol. J. 20, 333–342. doi:10.1016/j.csbj.2021.12.028
Yang, J., Peng, S., Zhang, B., Houten, S., Schadt, E., Zhu, J., et al. (2020). Human geroprotector discovery by targeting the converging subnetworks of aging and age-related diseases. Geroscience 42 (1), 353–372. doi:10.1007/s11357-019-00106-x
Yang, M., Luo, H., Li, Y., and Wang, J. (2019). Drug repositioning based on bounded nuclear norm regularization. Bioinformatics 35 (14), i455–i463. doi:10.1093/bioinformatics/btz331
Yuan, Y., Liu, W., Zhang, Y., and Sun, S. (2018). CircRNA circ_0026344 as a prognostic biomarker suppresses colorectal cancer progression via microRNA-21 and microRNA-31. Biochem. Biophys. Res. Commun. 503 (2), 870–875. doi:10.1016/j.bbrc.2018.06.089
Yue, M., Yun, Z., Li, S., Yan, G., and Kang, Z. (2021). NEDD4 triggers FOXA1 ubiquitination and promotes colon cancer progression under microRNA-340-5p suppression and ATF1 upregulation. RNA Biol. 18 (11), 1981–1995. doi:10.1080/15476286.2021.1885232
Zhang, H. W., Shi, Y., Liu, J. B., Wang, H. M., Wang, P. Y., Wu, Z. J., et al. (2021). Cancer-associated fibroblast-derived exosomal microRNA-24-3p enhances colon cancer cell resistance to MTX by down-regulating CDX2/HEPH axis. J. Cell. Mol. Med. 25 (8), 3699–3713. doi:10.1111/jcmm.15765
Keywords: colon cancer, colorectal cancer, microRNA, biomarker, microRNA-disease association, bound nuclear norm regularization
Citation: Zhai S, Li X, Wu Y, Shi X, Ji B and Qiu C (2022) Identifying potential microRNA biomarkers for colon cancer and colorectal cancer through bound nuclear norm regularization. Front. Genet. 13:980437. doi: 10.3389/fgene.2022.980437
Received: 28 June 2022; Accepted: 01 August 2022;
Published: 22 September 2022.
Edited by:
Liqian Zhou, Hunan University of Technology, ChinaReviewed by:
Guohua Huang, Shaoyang University, ChinaYing Liang, Jiangxi Agricultural University, China
Copyright © 2022 Zhai, Li, Wu, Shi, Ji and Qiu. This is an open-access article distributed under the terms of the Creative Commons Attribution License (CC BY). The use, distribution or reproduction in other forums is permitted, provided the original author(s) and the copyright owner(s) are credited and that the original publication in this journal is cited, in accordance with accepted academic practice. No use, distribution or reproduction is permitted which does not comply with these terms.
*Correspondence: Chun Qiu, MTM5NzYyNDIxMjdAMTM5LmNvbSYjeDAyMDBhOw==
†These authors have contributed equally to this work and share first authorship