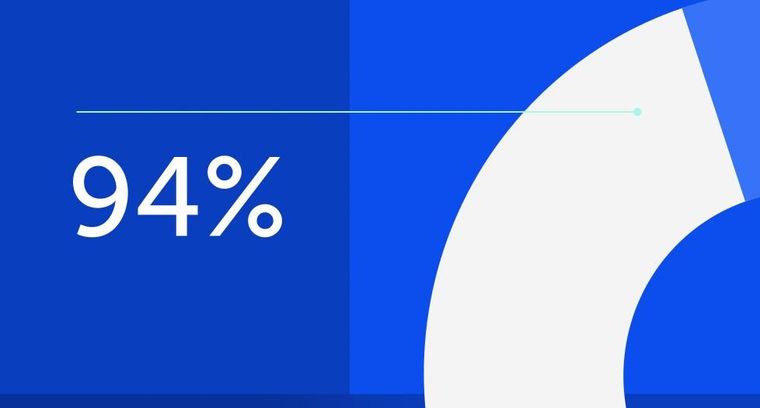
94% of researchers rate our articles as excellent or good
Learn more about the work of our research integrity team to safeguard the quality of each article we publish.
Find out more
ORIGINAL RESEARCH article
Front. Genet., 30 September 2022
Sec. Livestock Genomics
Volume 13 - 2022 | https://doi.org/10.3389/fgene.2022.974619
This article is part of the Research TopicTranscriptional/Post-Transcriptional Regulations in Agricultural Species After StressesView all 18 articles
Heterosis has been extensively exploited in chicken breeding to improve laying traits in commercial hybrid stock. However, the molecular mechanisms underlying it remains elusive. This study characterizes the miRNAome in the pre-hierarchical follicles of purebred and hybrid laying hens, and investigate the functions of miRNAs with non-additive expression in the pre-hierarchical follicles as they modulate heterosis for egg number and clutch size. To achieve that aim, White Leghorn and Rhode Island Red chicken lines were reciprocally crossed to generate hybrids. The crossbreds demonstrated heterosis for egg number and clutch size, and pre-hierarchical follicles from 4 birds of each genotype were collected at 53 weeks of age. Mode of miRNA expression was characterized after miRNA sequencing. A total of 50 miRNAs including 30 novel ones, were found to exhibit non-additive expression. Dominance was the predominant mode of expression exhibited by majority of the miRNAs. Functional analysis of target genes of the known miRNAs with non-additive expression revealed Gene Ontology terms related to regulation of transcription, metabolic processes and gene expression. KEGG and REACTOME pathways including hedgehog, cellular senescence, wnt, TGF-β, progesterone-mediated oocyte maturation, oocyte meiosis, GnRH signaling, signal transduction and generic transcription, which can be linked to primordial follicle activation, growth and ovulation, were significantly enriched by target genes of miRNAs with non-additive expression. Majority of the genes enriched in these biological pathways were targeted by gga-miR-19a, gga-miR-19b, gga-miR-375, gga-miR-135a, and gga-miR-7 and 7b, thus, revealing their synergistic roles in enhancing processes that could influence heterosis for egg number and clutch size in hybrid hens.
Heterosis remains a central theme in the field of poultry breeding, and previous investigations established non-additive gene action as the primary cause. In chickens, heterosis is accomplished through crossbreeding genetically distinct lines and breeds such that the average performance of the crossbred population is superior to the mid performance of the purebred parental lines expected under additive gene assumptions. In addition to heterosis, breed complementarity is also exploited by crossbreeding. The success of crossbreeding schemes largely depends on crossing of genetically diverse lines (Amuzu-Aweh, 2020).
Egg laying efficiency typified by high laying rates, large number of eggs and larger clutches seem to be the traits that are enhanced in the hybrids laying birds, and were linked to well-orchestrated and organized follicular hierarchy in the hens (Johnson, 2012). The selection process involves recruitment from pre-hierarchical pool, follicles into the pre-ovulatory hierarchy. The changes that occur after follicle recruitment during the pre-ovulatory period were propound and differ even between pre-ovulatory follicles. The dynamics in gene expression profiles of pre-ovulatory follicles with its attendant variation between pullets of the same genotype poses great challenge to transcriptomics studies. Prior to selection event, many pre-hierarchical follicles either undergo atresia or remain steroidogenic incompetent in an effort to preserve the sanctity of small viable cohorts of pre-selected follicles associated with follicular reserve and subsequently, clutches of eggs (Johnson, 2015).
Previous studies have elucidated the mechanisms of maintaining the state of primordial follicles by local factors and intracellular pathways. This occurs via the action of multiple activators including growth differentiation factor 9 (GDF9), anti-mullerian hormone (AMH), zona pellucida 2 (ZP2), wingless-type MMTV integration site family member 4 (WNT 4) (Zhang et al., 2019), and recently, PPAR pathway (Yoon et al., 2020).
The discovery of the first microRNA (miRNA) gene member (lin4) in the early 90s (Lee et al., 1993) has ushered an ever-expanding field of miRNA research with new members being reported every day. MicroRNAs are a class of small non-coding RNAs (∼22 nt) that regulate gene expression at a post-transcriptional level through complementary base pairing with the target mRNA, leading to degradation of mRNA and eventually repressing its translation (Riffo-Campos et al., 2016). Many investigations have shown that miRNAs are involved in the regulation of various pathways and exert influence on a wide variety of phenotypes. To date, there are 1,235 chicken miRNAs cataloged in the miRDB database (http://mirdb.org/statistics.html) and the number will continue to increase with the decline in the cost of high throughput sequencing, commensurate with Moore’s law (Brock and Moore, 2006) and increasing curiosity of molecular scientist to address myriads of complex molecular phenomena. The array of functions miRNAs perform in the translation of coded information in the DNA to phenotype lies with its unique ability to bind to the canonical site of other RNA species called microRNA response elements (MRE), which allows it to interact with a wide variety of RNA species (including mRNAs, lncRNAs, and circRNAs). MiRNAs are therefore central molecules in the theory of cross-talking of ceRNAs proposed at the beginning of the last decade (Salmena et al., 2011). This class of RNAs represses the expression of mRNAs thereby lowering the expression of the genes encoding the larger species of ceRNAs.
In chickens, the roles of miRNAs in the expression of phenotypes including sperm motility in roosters (Liu et al., 2018) and egg number in laying hens (Zhang et al., 2017) have been documented in recent times. Despite the growing body of literature in the field of miRNA biogenesis, identification and target prediction on one hand and intensive search for molecular mechanisms of heterosis in domestic chickens (Mai et al., 2019; Zhuo et al., 2019) on the other hand, there is still a gap in literature on the mode of inheritance and possible influence of miRNAs on heterosis for egg number and clutch size in laying chickens. This study therefore, aims to identify miRNAs with non-additive mode of expression which may influence heterosis for egg number and clutch size in laying hens.
Number of eggs laid by the White Leghorn (W) and Rhode Island Red (R) parental purebred lines were significantly less than (p < 0.001) the number laid by their reciprocal hybrids at 53 weeks of age (Figure 1). The synthesized mid-parent value (MPV) for the purebred was similar to the two parental lines. Heterosis for egg number was 14.1 and 18.5% in Rhode Island Red × White Leghorn (RW) and White Leghorn × Rhode Island Red (WR) respectively. Furthermore, heterosis for clutch size was 12.29 and 38.43% in RW and WR hybrids respectively.
FIGURE 1. Egg number and clutch size at 53 weeks of age for parental lines and their reciprocal hybrids. Mean values with an asterisk (*) on top of them were significantly different (Tukey-Kramer HSD p ≤ 0.05). W: Purebred White Leghorn, R: Purebred Rhode Island Red, RW: Rhode Island Red × White Leghorn cross, WR: White Leghorn × Rhode Island Red cross, MPV: Mid-parent value.
MicroRNA sequences generated from the 16 libraries constructed from RNA extracted from pre-hierarchical white follicles in the two purebred (R and W) and their reciprocal hybrids (RW and WR) yield a total of 291, 129, 265 reads after filtering for quality (Phred score >20), size selection (18–30 nt) and trimming of adapter sequences. Out of this, 272,842, 977 (93.66%) reads aligned to the chicken genome built GRCg6a of the Ensembl database and were preserved for further analysis. Specifically, 169, 706, 629 reads (62.30%) mapped with a perfect match while the remaining 103, 136, 348 reads (37.8%) mapped with a single nucleotide mismatch. For each library, more than 90% of the clean reads were successfully mapped to the chicken genome (Supplementary Figure S1).
Furthermore, mapped reads belonging to other species of non-coding RNAs including rRNA (8,103,438 (2.97%)), tRNA (4,251,546 (1.55%)), snRNA (176,885 (0.06%)), snoRNA (5,646,100 (2.07%)) and others (563,900, (0.21%)) collectively accounted for 6.44% of the total reads, and were discarded (Supplementary Table S1). Similarly, reads that mapped to the low diversity region of the genome including SINES, LINES, and LTR which collectively accounted for 0.83% of the total raw reads were not retained in the subsequent analyses.
A total of 899 and 289 known and predicted novel miRNAs were found to be expressed in the pre-hierarchical follicles. After filtering out the lowly expressed miRNAs with <10 read counts per million in all the libraries, a total of 595 known and 235 novel miRNAs were preserved for further downstream analyses (Supplementary Table S2). Principal component analysis (PCA) of the retained clean reads depicts clear separation of the four genetic groups (Figure 2). PC1 vs. PC2 plot assigned the genotypes into three clusters; two clusters for W and R purebred parental lines and another cluster for the two reciprocal hybrids (RW and WR). Furthermore, PC2 vs. PC3 plot separates the genotypes into four distinct clusters.
FIGURE 2. Separation of the pure lines and reciprocal hybrids by PCA (A): PC1 vs. PC2 plot (B): PC2 vs. PC3 plot.
MiRNAs with abundant expression (∼1 million raw reads/per library) in the ovary of laying hens at 53 weeks of age include gga-miR-143-3p, gga-miR-148a-3p, gga-miR-26a-2-5p, gga-miR-26a-5p, gga-miR-99a-5p, gga-miR-21-5p and gga-miR-10a-5p. The most abundant miRNA (gga-miR-143-3p) had raw reads between 9 and 13 million reads, accounting for 3.9, 3.8, 4.9 and 4.3% of all expressed miRNAs in R, W, RW and WR genotypes respectively. Twenty most abundantly expressed known miRNAs expressed in the pre-hierarchal follicles in the purebred and hybrids laying chickens were same and are presented in Table 1.
Pair-wise comparisons of miRNA expression between the pre-hierarchical follicles in the ovaries of the reciprocal hybrids and their parental purebreds on one hand and between the hybrids and synthesized mid-parent average on the other hand are presented in Figure 3.
FIGURE 3. Differentially expressed miRNAs among parental lines (W and R), hybrids (WR and RW) and synthesized average expression of the parents (A).
Comparing miRNA expression in the two parental purebred lines, 30 differentially expressed miRNAs (DEMiRs) were detected, including nine upregulated and 21 downregulated miRNAs. In RW vs. A, 21 DEMiRs were identified, 8 and 13 were upregulated and downregulated respectively. In RW vs R, a total of 19 DEMiRs were identified while 23 DEMiRs were detected in RW vs W. Further, WR vs. R yielded 65 DEMiRs consisting of eight upregulated and 57 downregulated miRNAs, and 139 miRNAs showed differential expression in WR vs. R. In WR vs. W, 127 DEMiRs were detected including 54 upregulated and 73 downregulated miRNAs.
Overall, all pairwise comparisons yielded 70 unique DEMiRs. Further classification of the DEMiRs based on their mode of inheritance pattern was achieved by overlapping the DEMiRs in Venn diagrams. Total of 20 DEMiRs exhibited additive mode of expression (Supplementary Figure S2), while the remaining unique DEMiRs exhibited non-additive mode of expression (Figure 4). Of these, 20 known miRNAs were grouped in to 14 miRNA family clusters (Table 2), and dominance mode of expression pattern was exhibited by 19 of them. The remaining 30 were suggested novel miRNAs (Supplementary Table S4). Overall, 14, 17, 13 and six miRNAs exhibited high-parent dominance, low parent dominance, over-dominance and under-dominance inheritance patterns respectively (Table 2; Supplementary Table S3).
FIGURE 4. Venn diagram showing DEMiRs according to their pattern of inheritance (A) High parent dominance in RW (B) Low-parent dominance in RW (C) High parent dominance in WR (D) Low-parent dominance in WR (E) Overdominance (enclosed in red) and underdominance (enclosed in blue) expression of miRNAs in RW (F) Overdominance (enclosed in red) and underdominance (enclosed in blue) expression of miRNAs in WR.
TABLE 2. Known miRNAs abundance and their non-additive modes of expression in the ovary of purebred White Leghorn, Rhode Island Red, and their reciprocal hybrids.
A total of 970 unique genes were identified as potential targets for 11 known miRNA with non-additive expression (Supplementary Table S4). Target genes with aggregate PTC score ≥50 were screened against the mRNA transcriptome of the pre-hierarchical follicles obtained by sequencing same sample from where miRNAome was sequenced. This yields a list of 970 unique mRNAs, which were uploaded into g. profiler for functional analysis including GO, KEGG and REAC. Overview of the result of gene enrichment analysis in GO, KEGG and REAC databases is depicted in Figure 5. The enriched GO terms consist of 918 biological processes (BP), 53 molecular functions (MF) and 80 cellular components (CC) (Supplementary Table S5). Specifically, GO annotation enrichment showed that target genes of miRNAs with non-additive expression were associated with regulation of cellular process (GO: BP term; FDR = 1.12 × 10–26), nucleus (GO:CC term; FDR = 4.77 × 10–12) and transcription regulatory activity (GO:MF term; FDR = 3.4 × 10–18).
FIGURE 5. Manhattan plot showing number of significantly enriched terms in GO, KEGG and REACT databases for target genes of miRNAs with non-additive expression.
Further, 21 and 16 pathways respectively in KEGG and REACTOME databases were significantly enriched by target genes of miRNAs with non-additive expression. In KEGG database, the pathways include Hedgehog signaling, cellular senescence, focal adhesion, FoxO signaling Wnt signaling, MAPK signaling, mTOR signaling, insulin signaling, TGF-β signaling, oocyte meiosis, autophagy, progesterone-mediated oocyte maturation and GnRH signaling (Table 3). These pathways share common genes and were interconnected (Figure 6).
TABLE 3. Pathways enriched in the KEGG database for target genes of miRNAs with non-additive mode of expression.
FIGURE 6. Network of enriched KEGG pathways for target genes of non-additively expressed miRNAs. Small cyan circles represent target genes of miRNAs with non-additive expression; Yellow circles represent KEGG pathways enriched by the target genes with p-values<0.05; Large blue circles represent KEGG pathways enriched by the target genes with corrected p-values<0.05.
In the REACTOME database, enriched pathways include glucagon-like peptide (GLP1) regulates insulin secretion, post-transcriptional regulation by small RNA, generic transcription, gene expression, signal transduction, signal by WNT and regulation of insulin secretion (Table 4).
TABLE 4. REACTOME terms enriched by target genes of miRNAs with non-additive modes of expression in crossbred laying hens.
The co-expression network for miRNAs with non-additive expression and their target genes is presented in Figure 7. The network revealed that more than one gene can be targeted by one miRNA. MicroRNAs that exhibited low-parent dominance such as gga-miR-19-3p targeted many genes in the follicles. Contrarily, gga-miR-375 with overdominance in WR genotype targeted only CHRNA1 and FRRS1 genes.
FIGURE 7. mRNA-miRNA regulatory network in the pre-hierarchical follicles of purebred and hybrid laying hens. Red diamonds represent miRNAs with low parent dominance expression; Cyan diamonds represent miRNAs with either high-parent dominance or over-dominance expression in the hybrids; Green ellipses represent target genes of miRNAs with non-additive expression.
Expression analysis of the four miRNAs revealed that pattern of expression was consistent between the Illumina small RNA sequencing and real-time quantitative PCR (RT-qPCR) with a correlation co-efficient of 0.647 (Figure 8).
FIGURE 8. RT-qPCR validation of four miRNAs that exhibited non-additive modes of expression identified using Illumina small RNA deep sequencing.
Mode of miRNAs inheritance in reciprocal hybrids and their influence on heterosis for egg number and clutch size traits in laying chickens was not hitherto documented. By sequencing miRNAome of pre-hierarchical follicles of laying hens, we delineate the modes of miRNAs expression in parental purebred lines (R and W) and their reciprocal crossbreds (RW and WR). We identified 595 known miRNAs and successfully predicted 325 novel miRNAs that were expressed in the ovary of the hybrid laying hens and their purebred parental lines. MicroRNAs with highest abundance were gga-miR-143-3p and gga-miR-148a-3p, which together accounted for approximately 8% of the total expressed miRNAs in the pre-hierarchical follicles of the hens. The top five most abundantly expressed miRNAs constitute approximately 10–15% of the expressed miRNAs in the purebred and the hybrids respectively. Interestingly, all of them were known miRNAs, suggesting that known miRNAs were most abundantly expressed while predicted novel miRNAs were lowly expressed, and hence, the inability to detect them in previous investigations. This is consistent with previous reports for most abundantly expressed miRNAs in the ovary of vertebrates (Kang et al., 2013; Wang et al., 2017; Wu et al., 2017; Wang and Ma, 2019). In particular, gga-miR-143-3p, gga-miR-145-5p, gga-miR-26, gga-miR-99, gga-miR-10a, gga-miR-21, gga-miR-148, gga-miR-199, gga-miR-126, gga-miR-125, gga-miR-101 and gga-let-7 family gene clusters were reported as the most abundantly expressed miRNAs in the ovaries of laying chickens (Kang et al., 2013; Wu et al., 2017). In addition, gga-miR-145-5p gene target mRNAs in the ovary of chickens were enriched in TGF-β signaling pathway by regulating the growth and development of primordial follicles (Kang et al., 2013). In mouse, gga-miR-143 homolog was also highly expressed in the ovary and regulates estradiol synthesis (Zhang et al., 2017) thereby controlling ovulation. Loss of gga-miR-145 function in the ovary of mouse model was successfully linked to hyper-activation of primordial follicles, and deregulation of zona pellucida in actively growing follicles (Yang et al., 2013) which might lead to depletion of ovarian reserve. Further, gga-miR-21 in ovaries of chickens was construed to be involved in follicular growth and ovulation (Kang et al., 2013). Taken together, these suggest that miRNAs with abundant expression in the ovary play critical roles in follicular development and ovulation, two processes that regulate egg reproduction in hens.
The main purpose of the present investigation was to identify miRNAs with non-additive expression in the pre-hierarchical ovarian follicles of laying hens. For the first time, our study identified 20 known and novel miRNAs with additive mode of expression, and 50 known and novel miRNAs that demonstrated non-additive expression. Of the 50 DEMiRs that demonstrated non-additive expression, 20 were known while 30 were not previously reported. MicroRNA families that showed non-additive mode of expression include gga-miR-1684, gga-miR-19 and gga-miR-7, which have two members each and gga-miR-34 family which has four members.
MicroRNAs in the gga-miR-1684 family exhibited high-parent dominant expression in both hybrids, while all the four members of the gga-miR-34 family showed low-parent mode of expression. Previous investigation of miRNA transcriptome in the hierarchical follicles of laying chickens revealed that gga-miR-34b and gga-miR-34c members were up-regulated in more efficient laying hens (Wu et al., 2017). In our study, dominance was the major mode of non-additive expression exhibited by known miRNAs (5 high-parent dominant and 14 low-parent dominant). Of note, gga-miR-375 was the only known miRNAs that demonstrated over-dominance mode of expression in the WR hybrid hens. Previously, in vitro over-expression study in granulosa cell (GC) line from pig ovary has established that miR-375 regulates E2 synthesis (Yu et al., 2017) and its mode of expression in the present study has confirmed its involvement not only in reproduction but also hybrid vigor for egg laying and clutch traits in hens. However, it is not clear how higher expression of gga-miR-375 in the pre-hierarchical follicles of WR hybrids that demonstrated high heterosis in egg and clutch traits may promote laying of more eggs. Yu et al. has reported co-expression between gga-miR-357 and corticotrophin releasing hormone in the GCs, which synergistically regulate E2 synthesis (Yu et al., 2017). It can be asserted that the higher expression may be exclusive only to the pre-hierarchical follicles. If this was the case, it can be a strategy for preserving follicle pool where only hierarchical follicles will show low expression thereby reducing disrupted follicular selection process typical in low efficient laying birds.
Evidence provided by the result in our study suggests the presence of synergistic effect between miRNAs that exhibited low and high parent dominance in dictating heterosis for egg number and clutch size. Results of the GO and REACTOME enrichment were populated with terms and pathways related to regulation of transcription and gene expression, and suggests the importance of these processes in modulating heterosis for egg number and clutch size in the crossbred laying hens. Further evidences inferred by KEGG enrichment analysis supporting the crucial role of target genes of miRNAs exhibiting non-additive mode of expression are centered in processes related to organization of follicular hierarchy and recruitment. Finely orchestrated follicular development is essential for efficient egg laying typical in commercial hybrid hens. This complex process begins by systematic activation of the quiescent, non-growing primordial follicles to primary oocyte. Follicles that did not follow this cellular fate undergo atresia, thus, reducing follicular reserve. Results of the current study suggests the involvement of cellular senescence in achieving follicle atresia, and genes in the pathway were targeted by miRNAs exhibiting low-parent dominance (gga-miR-19a-3p, gga-miR-1351-5p and gga-miR34c-3p). Hedgehog signaling, the most enriched pathway in the current study, was reported to regulate follicle development and female germ line stem cell proliferation (Jiang et al., 2019). Majority of the target genes enriched in this pathway were targeted by gga-miR-19a-3p and gga-miR-1351-5p, both of which exhibited low-parent dominance in the crossbred hens. Furthermore, pathways for signal transduction including FoxO, WNT, insulin, mTOR, and ErbB were reported to be crucial for follicle activation, growth and development (Kang et al., 2013; Sun et al., 2015; Lee et al., 2016; Tao et al., 2017; Zhao et al., 2018; Zhang et al., 2019; Zhou et al., 2020). Specifically, it was shown that elevated activity of mTORC1 in oocytes causes follicular depletion and premature ovarian failure (Adhikari et al., 2010). Our data corroborates these reports. Genes involved in insulin signaling pathway were reported to be targets for gga-miR-375 (Kang et al., 2013) similar to the observation in the current study.
TGF-β signaling and the genes that enriched the pathway (INHBB, INHBA, PITX2, Activin) support maintenance of ovarian reserve which diminishes owing to either follicle activation, recruitment, maturation and subsequent ovulation, or through atresia (Pelosi et al., 2015). It is asserted here that oocyte maturation for follicles destined to ovulate were facilitated via key pathways including oocyte meiosis, GnRH-signaling and progesterone-mediated oocyte maturation. Many of these pathways were significantly enriched in the ovaries of actively laying geese, and laying chickens with high egg production (Luan et al., 2014; Zhang et al., 2019). Recent investigation reported that focal adhesion and gap junction pathways were enriched in the hypothalamus-pituitary-gonadal tissues, which were linked to high egg production in geese (Wu et al., 2020). Similarly, our data support the involvement of these pathways enriched by genes targeted by miRNAs with non-additive expression in the pre-hierarchical follicles of laying hens.
Based on mRNA-miRNA co-expression network, target genes for gga-miR-19-3p, gga-miR-34-5p, gga-miR-217-5p, gga-miR-135a-5p, gga-miR-205a, gga-miR-375, and gga-miR-7 may underlie heterosis for egg laying in hybrid hens. Specifically, gga-miR-34-5p and gga-miR-205a promote cell apoptosis and were significantly expressed in small yellow follicles of laying chickens and geese (Wu et al., 2020; Hou et al., 2021) while gga-miR-145a-5p promotes premature progesterone release in granulosa cells of pre-hierarchical follicles (McBride et al., 2012). These processes could induce atresia with consequent erosion of follicle reserve. Furthermore, the two candidate miRNAs with high-parent or overdominance expression (gga-miR-375 and gga-miR-7) were associated with regulation of cell proliferation (Yuan et al., 2015), inhibition of synthesis and secretion of gonadotropins (He et al., 2020) essential for finely regulated follicular hierarchy establishment. Taken together, the interplay of miRNAs with non-additive expression and their target genes could underlie heterosis in egg production and clutch traits observed in the hybrid chickens.
This study was designed to characterize miRNAs with non-additive expression in the follicles of purebred and crossbred hens, and investigate the functions of miRNAs in modulating heterosis for egg number and clutch size. To achieve that aim, mode of miRNA expression was characterized by miRNA sequencing. A total of 50 miRNAs including 30 novel, were found to exhibit non-additive expression. Dominance was the predominant mode of expression exhibited by majority of the miRNAs. Functional analysis of target genes of the known miRNAs with non-additive expression were significantly enriched in GO terms related to regulation of transcription, metabolic processes and gene expression. KEGG pathways enriched in target genes of non-additive genes include hedgehog, cellular senescence, WNT, TGF-beta, progesterone-mediated oocyte maturation, oocyte meiosis, GnRH signaling, signal transduction and transcription regulation, which could be linked to primordial follicle activation, growth and ovulation. mRNA-miRNA co-expression network constructed using mRNA and miRNA suggest gga-miR-19 family, gga-miR-375, gga-miR-205a-3p, gga-miR-375, and gga-miR-7 family are candidate miRNAs that play synergistic roles in maintenance of organized follicular growth and development which may influence heterosis for egg number and clutch size in crossbred hens.
Experimental procedure for all animal experiments was approved by the Animal Care and Use Committee of the Institute of Animal Science, Chinese Academy of Agricultural Sciences, Beijing (IAS-CAAS). The procedure for the generation of the experimental birds involves crossbreeding of R and W purebred lines to produce offspring of the purebred lines; W, R and their reciprocal crossbred RW and WR. Briefly, semen from R and W purebred sires were artificially inseminated to purebred dams of their lines and the other lines in a reciprocal crossing design to obtain half-sib purebred and crossbred chicks. Four genotypes of chicks were hatched. Female chicks so generated were vaccinated, wing banded and raised. All hens were managed under standard housing and fed appropriate kind of diet specific to age and developmental stage of the birds. The birds were offered unrestricted access to feed and water throughout the period of brooding, rearing and laying.
Egg laying was recorded for individual hens once daily. Total egg number and clutch size up to 53 weeks of age were computed for individual hens and selected hens with phenotypic records corresponding to their population average. Least square means for egg number was compared between genetic groups using Tukey-Kramer HSD at p < 0.05, using the model below;
Where
Heterosis was calculated using the model below;
Where F1 is the performance value of the hybrid, P1 and P2 are the performance values of the two parental lines.
Four birds each from the purebred parental and reciprocal crossbred populations (R, W, RW and WR) that have egg laying records corresponding to the average of their populations were exsanguinated by cervical dislocation. After slaughter, the pullets were dissected by ventral midline incision and the intact reproductive tract of the hens were collected, weighed and separated into ovary (and follicles) and oviduct. Pre-hierarchical follicles (4–8 mm in diameter) were sorted and collected from each bird, snap frozen in liquid nitrogen, taken to laboratory and stored at -80 °C until RNA extraction.
Pre-hierarchical follicles (30–50 mg/sample) were used for total RNA extracted using Trizol reagent (Invitrogen). A total of 16 samples with four each belonging to the W, R, RW and WR were used. RNA concentration and integrity were determined using NanoDrop. ND-1000 (NanoDrop Technologies, Wilmington, DE, United States) and Agilent 2100 Bioanalyzer (Agilent Technologies, CA, United States) respectively. Only RNA sample with concentration >200 ng/μL, RIN >7 and 28S/18S rRNA ratio >1.7 was used for the small RNA library construction. An amount of 1 μg total RNA was used in the construction of each of the libraries (n = 16) using Illumina TruSeq.
In silico processing of the generated sequence reads was carried out in accordance with established pipelines (Korpelainen et al., 2014; Do et al., 2018). Briefly, raw sequence reads (16 fasta files) were subjected to quality control using FastQC (He et al., 2020) where adapter primers and poor quality reads were removed. Trimming of 3′ and 5′ adapter sequences was achieved using in-house pipeline developed by Annaroad Gene Technology Co., Ltd. (Beijing, China). Only reads with Phred score >20 and sequence read between 18 and 30 nt were retained. Clean reads that passed the quality control criteria were parsed to build a reference genome index. Bowtie 1 (http://bowtie-bio.sourceforge.net/index.shtml) (Langmead et al., 2009) was used to align the built reference genome to GRCg6a (GCA_000002315.5) chicken genome downloaded from the Ensembl database.
The identification of known miRNAs was performed in miRBase (v22.1) (Andrews, 2017). Discovery of novel miRNAs was achieved after excluding known miRNAs. Reads that mapped to other small RNA species (rRNA, tRNA, snRNA, and snoRNA) Rfam (v.13.0) in the RNA family database (http://rfam.xfam.org/) (Kozomara et al., 2019) and those that mapped to the repeated regions of the genome were excluded. Identification of the repeated regions from the uniquely mapped reads was achieved using RepeatMasker v.4.0.9 (http://repeatmasker.org/cgi-bin/WEBRepeatMasker). MirDeep2 program that implements a probabilistic algorithm based on the miRNA biogenesis model was used to predict novel miRNAs in each library (Kalvari et al., 2018; Friedländer et al., 2012).
MiRNAs that met the criteria of being either known or novel were used for differential expression analysis. DEGseq (v1.18.0) package implemented in Bioconductor which follows the assumption of binomial distribution was used for differential gene expression analysis (http://www.bioconductor.org/packages/release/bioc/html/DEGseq.html). Only miRNAs that fulfill the Benjamini and Hochberg criteria for multiple testing correction (false discovery rate (q < 0.05) and |Log2_ratio| ≥1 were identified as DEMiRs. Differential expression of miRNAs was achieved based on pairwise comparisons of R vs W, R vs RW, R vs WR, W vs RW, and W vs WR. Additionally, the reciprocal crosses were also compared with a synthetic group of mid-parent miRNA expression values, which were calculated by taking the means of normalized gene count from combinations of paternal lines (A = 1/2 (R + W)). Consequently, miRNAs with additive and various forms of non-additive modes of expression were identified. Delineation of DEMiRs into additive, high-parent dominant, low-parent dominant, over-dominant and under-dominant were in accordance with previous studies (Swanson-Wagner et al., 2006; Mai et al., 2019) with little modification. Briefly, additivity occurs when expression of the miRNA was significantly different between the two parental purebred lines (R vs. W Padj.< 0.05, Log2FC ≥ 1) and that the miRNA expression in the crossbred was similar to the synthesized mean of their parental purebred lines. The high-parent dominant mode was when expression of the miRNA in the crossbred was significantly higher than one parent but similar to the other parental line. The low-parent dominant mode was when expression in the hybrid was significantly lower than one parental line but similar to the other parental line. Over-dominant mode occurs when miRNA expression in the crossbred was significantly higher than either of the two parental purebred lines. Under-dominance was when gene expression in the hybrid was significantly lower than the two parental purebred lines. This classification was achieved by subjection the DEMiRs to systematic comparisons in Venny 2.0 (Oliveros, 2007).
Target genes for known DEMiRs that exhibited non-additive pattern of expression were predicted with TargeScan release 7.2 (http://www.targetscan.org/vert_72/) (Agarwal et al., 2015). Catalogue of such target genes were filtered against the mRNA transcriptome obtained from the pre-hierarchical follicle tissues of the experimental birds. Only mRNAs common in target genes and expressed genes were retained for gene enrichment analysis. The filtered target genes for the non-additively expressed miRNAs in the hybrid chickens were uploaded into g. Profiler (https://biit.cs.ut.ee/gprofiler/gost) (Raudvere et al., 2019) for gene set enrichment of analysis. Gene set enrichment was carried out in Gene Ontology (GO), Kyoto Encyclopedia of Genes and Genomes (KEGG) and Reactome (REAC) databases. The g. profiler algorithm adopts over-representation analysis approach that uses hypergeometric test to measure the significance of functional terms in the input gene list (Raudvere et al., 2019). Significant threshold used was Benjamini–Hochberg corrected FDR threshold at 0.05. Network mapping of interconnectedness of significantly enriched KEGG pathways was achieved using GeneAnalyst (Zhou et al., 2019).
We constructed mRNA-miRNA regulatory network in order to identify candidate miRNAs with non-additive expression in the pre-hierarchical follicles of hybrid laying hens. Only mRNAs and miRNAs obtained from RNA sequencing of the samples were used. mRNA-miRNA pairs were delineated if their expression had pairwise correction coefficient of -0.9 or lower. The network was visualized using the cytoscape software.
The total RNA used for miRNA-sequencing was also used for Real-time quantitative PCR (RT-qPCR) to validate the expression of four non-additively expressed DEMiRs (gga-let-7f-5p, gga-miR-10b-5p, gga-miR-34c-3p and gga-miR-375) following previous report (Agarwal et al., 2015). miScript II RT kit (Qiagen) was used for the RT stage. Approximately 1 μL (1 μg) diluted RNA were added to the reaction mix containing 4 μL 5× miScript RT Buffer, 1 μL miScript Reverse Transcriptase Mix, and 15 μL RNase-free water to a final volume of 20 μL. RT reaction conditions consisted of incubation for 60 min at 37°C, incubating for 5 min at 95°C. Real-time PCR reactions were prepared using miScript SYBR Green PCR based on the manufacturer’s protocol. Briefly, 1 μL of the cDNA was added to the reaction mix containing 10 μL 2 × QuantiTect SYBR Green PCR Master Mix, 2 μL 10× miScript universal Primer, 2 μL 10× miScript Primer Assay to a final volume of 20 μL. The qRT-PCR condition consisted of denaturing at 95 °C for 15 min, followed by 40 cycles consisting 95 °C for 15 s, 55 °C 30 s, 70 °C 30 s. Samples were run in three technical replicates. Relative abundance of the micRNA transcripts was calculated using the 2-∆∆CT method. miRNAs expression result from qRT-PCR and their normalized expression from the TrueSeq were plotted in a histogram after calculating their correlation in each sample.
The datasets presented in this study can be found in online repositories. The names of the repository/repositories and accession number(s) can be found below: The transcriptome data are available in the Sequence Read Archive (https://www.ncbi.nlm.nih.gov/sra) at NCBI, with the BioProject ID: PRJNA859020 and SRA Accession Number: SAMN29766694-29766709.
The animal study was reviewed and approved by the Animal Care and Use Committee of the Institute of Animal Science, Chinese Academy of Agricultural Sciences (IAS-CAAS, No. IAS2020–12).
Conceptualization, JC, AI, and YS; methodology, AI, YL, JF, PW, AN, HT, and LS; software, AI, YS, and YL; validation, AN and YM; formal analysis, AI, JY, and YS; investigation, AI, AN, PW, and YL; resources, JC, YS, and HM.; data curation, AI and YS; writing—original draft preparation, AI; writing—review and editing, YS, JC, and HM; visualization, AI; supervision, JC, YS, and HM; project administration, JC and YS; funding acquisition, JC. All authors have read and approved to the publication of the manuscript.
This research was funded by the National Natural Science Foundation (grant number 32172721), Chinese Agricultural Research System (grant number CARS-40), the Fundamental Research Funds for Central Non-profit Scientific Institution (2021-YWF-ZYSQ-12), and the Agricultural Science and Technology Innovation Program (grant number ASTIP-IAS04).
The authors thank Yong Zhao and Chunjie Niu (Beijing Bainianliyuan Beijing-Yopu breeding company, Beijing, China) for their assistance in data recording.
The authors declare that the research was conducted in the absence of any commercial or financial relationships that could be construed as a potential conflict of interest.
All claims expressed in this article are solely those of the authors and do not necessarily represent those of their affiliated organizations, or those of the publisher, the editors and the reviewers. Any product that may be evaluated in this article, or claim that may be made by its manufacturer, is not guaranteed or endorsed by the publisher.
The Supplementary Material for this article can be found online at: https://www.frontiersin.org/articles/10.3389/fgene.2022.974619/full#supplementary-material
Supplementary Figure S1 | Mapping statistics for the 16 miRNA libraries in parental purebred lines and their reciprocal hybrids.
Supplementary Figure S2 | Venn diagram depicting number of DEMiRs with additive mode of expression.
Supplementary Table S1 | RNA-seq reads mapped to non-coding regions other than miRNAs expressed in the pre-hierarchical follicles of purebred chicken parental lines (R and W) and their reciprocal hybrids (RW and WR).
Supplementary Table S2 | Expressed miRNAs in the pre-hierarchical follicles of purebred chicken parental lines (R and W) and their reciprocal hybrids (RW and WR).
Supplementary Table S3 | Mode of expression of novel miRNAs in the pre-hierarchical follicles of purebred chicken parental lines (R and W) and their reciprocal hybrids (RW and WR).
Supplementary Table S4 | Target genes for known miRNAs with non-additive mode of expression in the pre-hierarchical follicles of purebred chicken parental lines (R and W) and their reciprocal hybrids (RW and WR).
Supplementary Table S5 | GO, KEGG and REAC terms enriched in target genes with non-additive mode of expression in the pre-hierarchical follicles of purebred chicken parental lines (R and W) and their reciprocal hybrids (RW and WR).
Adhikari, D., Zheng, W., Shen, Y., Gorre, N., Hämäläinen, T., Cooney, A. J., et al. (2010). Tsc/mTORC1 signaling in oocytes governs the quiescence and activation of primordial follicles. Hum. Mol. Genet. 19, 397–410. doi:10.1093/hmg/ddp483
Agarwal, V., Bell, G. W., Nam, J. W., and Bartel, D. P. (2015). Predicting effective microRNA target sites in mammalian mRNAs. eLife 4, e05005. doi:10.7554/eLife.05005
Amuzu-Aweh, E. N. (2020). Genomics of heterosis and egg production in White Leghorns. PhD thesis. Uppsala: Swedish University of Agricultural Sciences.
Brock, D. C., and Moore, G. E. (2006). Understanding moore's law: Four decades of innovation. Philadelphia, United States: Chemical Heritage Foundation.
Do, D. N., Dudemaine, P. L., Fomenky, B. E., and Ibeagha-Awemu, E. M. (2018). Integration of miRNA and mRNA co-expression reveals potential regulatory roles of miRNAs in developmental and immunological processes in calf ileum during early growth. Cells 7, 134. doi:10.3390/cells7090134
Friedländer, M. R., Mackowiak, S. D., Li, N., Chen, W., and Rajewsky, N. (2012). miRDeep2 accurately identifies known and hundreds of novel microRNA genes in seven animal clades. Nucleic Acids Res. 40, 37–52. doi:10.1093/nar/gkr688
He, J., Xu, S., Ji, Z., Sun, Y., Cai, B., Zhang, S., et al. (2020). The role of miR-7 as a potential switch in the mouse hypothalamus-pituitary-ovary axis through regulation of gonadotropins. Mol. Cell. Endocrinol. 518, 110969. doi:10.1016/j.mce.2020.110969
Hou, L., Ji, W., Gu, T., Weng, K., Liu, D., Zhang, Y., et al. (2021). MiR-34c-5p promotes granulosa cells apoptosis by targeting Bcl2 in broody goose (Anser cygnoides). Anim. Biotechnol., 1–9. doi:10.1080/10495398.2021.1886943
Jiang, Y., Zhu, D., Liu, W., Qin, Q., Fang, Z., and Pan, Z. (2019). Hedgehog pathway inhibition causes primary follicle atresia and decreases female germline stem cell proliferation capacity or stemness. Stem Cell. Res. Ther. 10, 198. doi:10.1186/s13287-019-1299-5
Johnson, A. L. (2015). Ovarian follicle selection and granulosa cell differentiation. Poult. Sci. 94, 781–785. doi:10.3382/ps/peu008
Johnson, P. A. (2012). Follicle selection in the avian ovary. Repr. Dom. Anim. 47, 283–287. doi:10.1111/j.1439-0531.2012.02087.x
Kalvari, I., Argasinska, J., Quinones-Olvera, N., Nawrocki, E. P., Rivas, E., Eddy, S. R., et al. (2018). Rfam 13.0: Shifting to a genome-centric resource for non-coding RNA families. Nucleic Acids Res. 46, D335–D342. doi:10.1093/nar/gkx1038
Kang, L., Cui, X., Zhang, Y., Yang, C., and Jiang, Y. (2013). Identification of miRNAs associated with sexual maturity in chicken ovary by Illumina small RNA deep sequencing. BMC Genomics 14, 352. doi:10.1186/1471-2164-14-352
Korpelainen, E., Tuimala, J., Somervuo, P., Huss, M., and Wong, G. (2014). RNA-Seq data analysis: A practical approach. New York, United States: CRC Press.
Kozomara, A., Birgaoanu, M., and Griffiths-Jones, S. (2019). miRBase: from microRNA sequences to function. Nucleic Acids Res. 47, D155–D162. doi:10.1093/nar/gky1141
Langmead, B., Trapnell, C., Pop, M., and Salzberg, S. L. (2009). Ultrafast and memory-efficient alignment of short DNA sequences to the human genome. Genome Biol. 10, R25. doi:10.1186/gb-2009-10-3-r25
Lee, H. C., Lim, S., and Han, Y. (2016). Wnt/β-catenin signaling pathway activation is required for proliferation of chicken primordial germ cells in vitro. Sci. Rep. 6, 34510–34518. doi:10.1038/srep34510
Lee, R., Feinbaum, R. L., and Ambros, V. (1993). The C. elegans heterochronic gene lin-4 encodes small RNAs with antisense complementarity to lin-14. Cell. 75, 843–854. doi:10.1016/0092-8674(93)90529-y
Liu, Y., Sun, Y., Li, Y., Bai, H., Xu, S., Xu, H., et al. (2018). Identification and differential expression of microRNAs in the testis of chicken with high and low sperm motility. Theriogenology 122, 94–101. doi:10.1016/j.theriogenology.2018.09.010
Luan, X., Liu, D., Cao, Z., Luo, L., Liu, M., Gao, M., et al. (2014). Transcriptome profiling identifies differentially expressed genes in Huoyan goose ovaries between the laying period and ceased period. PLOS One 9, e113211. doi:10.1371/journal.pone.0113211
Mai, C., Wen, C., Sun, C., Xu, Z., Chen, S., and Yang, N. J. G. (2019). Implications of gene inheritance patterns on the heterosis of abdominal fat deposition in chickens. Genes. 10, 824. doi:10.3390/genes10100824
McBride, D., Carre, W., Sontakke, S., Hogg, C. O., Law, A., Donadeu, F. X., et al. (2012). Identification of miRNAs associated with the follicular-lutealtransition in the ruminant ovary. Reproduction 144, 221–233. doi:10.1530/REP-12-0025
Oliveros, J. C. (2007). VENNY. An interactive tool for comparing lists with Venn Diagrams. https://bioinfogp.cnb.csic.es/tools/venny/index.html.
Pelosi, E., Simonsick, E., Forabosco, A., Garcia-Ortiz, J. E., and Schlessinger, D. (2015). Dynamics of the ovarian reserve and impact of genetic and epidemiological factors on age of menopause. Biol. Reprod. 92, 130–139. doi:10.1095/biolreprod.114.127381
Raudvere, U., Kolberg, L., Kuzmin, I., Arak, T., Adler, P., Peterson, H., et al. (2019). g:Profiler: a web server for functional enrichment analysis and conversions of gene lists (2019 update). Nucleic Acids Res. 47, W191–W198. doi:10.1093/nar/gkz369
Riffo-Campos, Á. L., Riquelme, I., and Brebi-Mieville, P. (2016). Tools for sequence-based miRNA target prediction: What to choose? Int. J. Mol. Sci. 17, 1987. doi:10.3390/ijms17121987
Salmena, L., Poliseno, L., Tay, Y., Kats, L., and Pandolfi, P. P. (2011). A ceRNA hypothesis: The rosetta stone of a hidden RNA language? Cell. 146, 353–358. doi:10.1016/j.cell.2011.07.014
Sun, X., Su, Y., He, Y., Zhang, J., Liu, W., Zhang, H., et al. (2015). New strategy for in vitro activation of primordial follicles with mTOR and PI3K stimulators. Cell. cycle 14, 721–731. doi:10.1080/15384101.2014.995496
Swanson-Wagner, R. A., Jia, Y., DeCook, R., Borsuk, L. A., Nettleton, D., and Schnable, P. S. (2006). All possible modes of gene action are observed in a global comparison of gene expression in a maize F1 hybrid and its inbred parents. Proc. Natl. Acad. Sci. U. S. A. 103, 6805–6810. doi:10.1073/pnas.0510430103
Tao, Z., Song, W., Zhu, C., Xu, W., Liu, H., Zhang, S., et al. (2017). Comparative transcriptomic analysis of high and low egg-producing duck ovaries. Poult. Sci. 96, 4378–4388. doi:10.3382/ps/pex229
Wang, C., and Ma, W. (2019). Hypothalamic and pituitary transcriptome profiling using RNA-sequencing in high-yielding and low-yielding laying hens. Sci. Rep. 9, 10285. doi:10.1038/s41598-019-46807-3
Wang, P., Li, X., Cao, L., Huang, S., Li, H., Zhang, Y., et al. (2017). MicroRNA-148a overexpression improves the early development of porcine somatic cell nuclear transfer embryos. PLoS One, e0180535. doi:10.1371/journal.pone.0180535
Wu, N., Gaur, U., Zhu, Q., Chen, B., Xu, Z., Zhao, X., et al. (2017). Expressed micro RNA associated with high rate of egg production in chicken ovarian follicles. Anim. Genet. 48, 205–216. doi:10.1111/age.12516
Wu, Y., Zhao, X., Chen, L., Wang, J., Duan, Y., Li, H., et al. (2020). Transcriptomic analyses of the hypothalamic-pituitary-gonadal Axis identify candidate genes related to egg production in xinjiang yili geese. Animals. 10, 90. doi:10.3390/ani10010090
Yang, S., Wang, S., Luo, A., Ding, T., Lai, Z., Shen, W., et al. (2013). Expression patterns and regulatory functions of microRNAs during the initiation of primordial follicle development in the neonatal mouse ovary. Biol. Reprod. 89 (126), 126–111. doi:10.1095/biolreprod.113.107730
Yoon, S. Y., Kim, R., Jang, H., Shin, D. H., Lee, J. I., Seol, D., et al. (2020). Peroxisome Proliferator-activated receptor gamma modulator promotes neonatal mouse primordial follicle activation in vitro. Int. J. Mol. Sci. 21, 3120. doi:10.3390/ijms21093120
Yu, C., Li, M., Wang, Y., Liu, Y., Yan, C., Pan, J., et al. (2017). Exposure to the Chinese famine in early life and hypertension prevalence risk in adults. J. Hypertens. 153, 63–68. doi:10.1097/HJH.0000000000001122
Yuan, B., Sun, G. J., Zhang, G. L., Wu, J., Xu, C., Dai, L. S., et al. (2015). Identification of target genes for adenohypophysis-prefer miR-7 and miR-375 in cattle. Genet. Mol. Res. 14 (3), 9753–9763. doi:10.4238/2015.August.19.8
Zhang, L., Zhang, X-J., Lu, Y., Li, L., Sheng, C., and Cui, S. (2017). MiRNA-143 mediates the proliferative signaling pathway of FSH and regulates estradiol production. J. Endocrinol. 234, 1–14. doi:10.1530/JOE-16-0488
Zhang, T., Chen, L., Han, K., Zhang, X., Zhang, G., Dai, G., et al. (2019). Transcriptome analysis of ovary in relatively greater and lesser egg producing Jinghai Yellow Chicken. Anim. Reprod. Sci. 208, 106114. doi:10.1016/j.anireprosci.2019.106114
Zhao, Y., Zhang, Y., Li, J., Zheng, N., Xu, X., Yang, J., et al. (2018). MAPK3/1 participates in the activation of primordial follicles through mTORC1‐KITL signaling. J. Cell. Physiol. 233, 226–237. doi:10.1002/jcp.25868
Zhou, G., Soufan, O., Ewald, J., Hancock, R. E., Basu, N., and Xia, J. (2019). NetworkAnalyst 3.0: A visual analytics platform for comprehensive gene expression profiling and meta-analysis. Nucleic Acids Res. 47, W234–W241. doi:10.1093/nar/gkz240
Zhou, S., Ma, Y., Zhao, D., Mi, Y., and Zhang, C. (2020). Transcriptome profiling analysis of underlying regulation of growing follicle development in the chicken. Poult. Sci. 99, 2861–2872. doi:10.1016/j.psj.2019.12.067
Keywords: crossbreeding, heterosis, egg (production), clutch size, miRNA, dominance
Citation: Isa AM, Sun Y, Li Y, Wang Y, Ni A, Yuan J, Ma H, Shi L, Tesfay HH, Fan J, Wang P and Chen J (2022) MicroRNAs with non-additive expression in the ovary of hybrid hens target genes enriched in key reproductive pathways that may influence heterosis for egg laying traits. Front. Genet. 13:974619. doi: 10.3389/fgene.2022.974619
Received: 21 June 2022; Accepted: 29 August 2022;
Published: 30 September 2022.
Edited by:
Shaojun Liu, Hunan Normal University, ChinaReviewed by:
Biao Chen, Jiangxi Agricultural University, ChinaCopyright © 2022 Isa, Sun, Li, Wang, Ni, Yuan, Ma, Shi, Tesfay, Fan, Wang and Chen. This is an open-access article distributed under the terms of the Creative Commons Attribution License (CC BY). The use, distribution or reproduction in other forums is permitted, provided the original author(s) and the copyright owner(s) are credited and that the original publication in this journal is cited, in accordance with accepted academic practice. No use, distribution or reproduction is permitted which does not comply with these terms.
*Correspondence: Yanyan Sun, eWFueWFuc3VuMjAxNEAxNjMuY29t; Jilan Chen, Y2hlbi5qaWxhbkAxNjMuY29t
Disclaimer: All claims expressed in this article are solely those of the authors and do not necessarily represent those of their affiliated organizations, or those of the publisher, the editors and the reviewers. Any product that may be evaluated in this article or claim that may be made by its manufacturer is not guaranteed or endorsed by the publisher.
Research integrity at Frontiers
Learn more about the work of our research integrity team to safeguard the quality of each article we publish.