- 1Edwin S. H. Leong Healthy Aging Program, Faculty of Medicine, University of British Columbia, Vancouver, BC, Canada
- 2Department of Medical Genetics, British Columbia Children’s Hospital Research Institute, University of British Columbia, Vancouver, BC, Canada
Parkinson’s disease (PD) is a neurodegenerative disorder with a complex etiology and increasing prevalence worldwide. As PD is influenced by a combination of genetic and environment/lifestyle factors in approximately 90% of cases, there is increasing interest in identification of the interindividual mechanisms underlying the development of PD as well as actionable lifestyle factors that can influence risk. This narrative review presents an outline of the genetic and environmental factors contributing to PD risk and explores the possible roles of cytosine methylation and hydroxymethylation in the etiology and/or as early-stage biomarkers of PD, with an emphasis on epigenome-wide association studies (EWAS) of PD conducted over the past decade. Specifically, we focused on variants in the SNCA gene, exposure to pesticides, and physical activity as key contributors to PD risk. Current research indicates that these factors individually impact the epigenome, particularly at the level of CpG methylation. There is also emerging evidence for interaction effects between genetic and environmental contributions to PD risk, possibly acting across multiple omics layers. We speculated that this may be one reason for the poor replicability of the results of EWAS for PD reported to date. Our goal is to provide direction for future epigenetics studies of PD to build upon existing foundations and leverage large datasets, new technologies, and relevant statistical approaches to further elucidate the etiology of this disease.
1 Introduction
Parkinson’s disease (PD) is the second most common neurodegenerative disorder, affecting an estimated 9.4 million individuals worldwide in 2020 (Maserejian et al., 2020). While approximately 5%–10% of PD cases are monogenically inherited, the remaining 90%–95% are considered “sporadic,” with genetics, environment, and gene–environment interactions contributing to varying risk profiles in affected individuals (Lesage and Brice, 2009; Dunn et al., 2019; Pang et al., 2019). One of the genes most closely involved with PD risk is SNCA, which encodes alpha-synuclein (α-Syn), a multifunctional protein that is localized at synaptic terminals (Villar-Piqué et al., 2016). Whole-gene multiplications of SNCA seen in familial PD and allelic variants in the SNCA promoter (REP1) can lead to increased expression of the protein and Lewy body aggregation in dopaminergic neurons (Chiba-Falek and Nussbaum, 2001; Maraganore et al., 2006). Conversely, SNCA point mutations can result in loss of function, with consequent impairment of neuronal health. These SNCA multiplications and point mutations occur in familial PD, while single nucleotide polymorphisms (SNPs) in SNCA can also contribute to risk of sporadic PD (Lesage and Brice, 2009).
Despite the high penetrance of familial PD-associated SNCA variants, the reasons underlying incomplete penetrance observed in certain individuals are not well understood. In addition, there is increasing interest in identification of PD biomarkers that could be used to detect disease before onset of symptoms and to prescribe lifestyle interventions to either prevent or slow progression of the disease. Epigenetic marks are attractive candidates for involvement in PD etiology due to their ability to mediate genetic and environmental effects on phenotype, which may partially explain the “missing heritability” in PD (Angelopoulou et al., 2022). The epigenome is comprised of a myriad of factors other than the DNA sequence that influence gene transcription, chromatin interactions, splicing, and other mechanisms. Epigenetic modifications are dynamically influenced by the complex interactions between genotype, environment, lifestyle, and developmental stage (Kumar et al., 2018). DNA methylation (DNAm), which typically involves the addition of a methyl group to the fifth carbon of the pyrimidine ring of cytosine in the CpG dinucleotide context, is one such epigenetic mark that has been extensively characterized in human populations. In addition to its plasticity with respect to genes and environment, DNAm also has potential as a biomarker due to concordance of DNAm patterns between the blood and brain at specific loci (Edgar et al., 2017).
Although epigenome-wide association studies (EWAS) have demonstrated altered DNAm patterns in individuals with PD in comparison to healthy subjects, the majority of findings were not replicable between studies (Masliah et al., 2013; Moore et al., 2014; Henderson-Smith et al., 2019; Li et al., 2020; Marshall et al., 2020; Vallerga et al., 2020; Henderson et al., 2021; Kia et al., 2021; Nabais et al., 2021; Kaut et al., 2022) (Supplementary Tables S1, S2). However, PD-related DNAm changes in particular genes, such as CYP2E1, have been reported in brain and blood across multiple studies (Kaut et al., 2012; Henderson-Smith et al., 2019; Kaut et al., 2022). Although few PD EWAS have assessed DNAm patterns in brain and blood from the same individuals, a study in five PD patients and six controls found 124 differentially methylated genes that showed concordant changes in brain and blood, representing 30% of the total annotated genes with differential methylation (Masliah et al., 2013). These observations suggest that blood DNAm in PD could be informative for specific loci in the brain. In addition, the extent to which DNAm patterns are associated with particular genetic backgrounds or environmental/lifestyle exposures in individuals with PD is an area of active research (Dunn et al., 2019; Angelopoulou et al., 2022). Although a number of SNPs associated with PD appear to affect DNAm and some studies have uncovered differential DNAm patterns in PD patients exposed to various drugs or pesticides, how these factors interact to influence risk in undiagnosed individuals remains unclear (International Parkinson’s Disease Genomics Consortium and Wellcome Trust Case Control Consortium, 2011; Nalls et al., 2014; Henderson-Smith et al., 2019; Go et al., 2020; Vallerga et al., 2020). Multi-omics studies including analysis of genotype, gene expression, and/or other epigenetic modifications alongside DNAm will also help to clarify the role of this epigenetic mark in the molecular etiology of PD (Kia et al., 2021).
Accumulating evidence also suggests that other cytosine modifications beyond DNAm have specific functions in the brain, including non-CpG methylation and DNA hydroxymethylation (DNAhm) (Kinde et al., 2015). Research on the role of DNAhm in PD is in its infancy, with initial studies reporting increased DNAhm in PD cerebellar white matter and increases in DNAhm and Ten-Eleven Translocation 2 (TET2) expression in purified neurons from PD patients (Kaut et al., 2019; Marshall et al., 2020). Taken together, these findings indicate that analysis of cytosine methylation has great potential for increasing our understanding of the etiologies underlying complex neurological disorders, such as Alzheimer’s disease and PD (Delgado-Morales and Esteller, 2017).
Here, we provide a comprehensive overview of cytosine modifications in PD in the context of SNCA genetic background and environmental inputs. We also discuss recent developments in PD biomarker discovery and integrated multi-omics strategies, along with future perspectives for PD epigenetics research.
2 Parkinson’s disease
Parkinson’s disease has an estimated prevalence of 4% among adults over 85 years old (Corti et al., 2011; Coppedè, 2012). The disease is caused by the degeneration of dopaminergic neurons in the substantia nigra, across other brain regions including the cortex, hippocampus, and brainstem, and in components of the central and peripheral nervous systems, leading to a range of motor and nonmotor phenotypes, including resting tremor, rigidity, bradykinesia, constipation, depression, and dementia (Lang and Lozano, 1998; Inzelberg et al., 2004). Although several monogenic forms of PD have been identified, up to 95% of PD cases are classified as sporadic, with no previous family history (Pang et al., 2019). These cases are thought to result from a combination of environmental factors and complex gene–environment interactions by mechanisms that are not yet comprehensively understood (Dunn et al., 2019). Epigenetic factors, such as DNAm, represent one such molecular mechanism that could mediate the genetic and environmental underpinnings of the etiology of PD.
3 Genetic and environmental underpinnings of Parkinson’s disease
3.1 Genetic predisposition to Parkinson’s disease and the role of SNCA
The first genetic studies of PD in the late 1990s identified hereditary mutations in the 4q21–q23 region and the SNCA gene linked to the disease in specific families (Polymeropoulos et al., 1996, 1997). However, further studies showed no associations between these mutations and PD, suggesting that the genetic etiology of PD was more complex than initially thought (Muñoz et al., 1997; Scott et al., 1997, 1999; Farrer et al., 1998). Eventually, variants in 28 chromosomal regions formerly known as the PARK loci were shown to be associated with PD. Of these, six were confirmed to cause monogenic PD: SNCA, LRRK2, Parkin, PINK1, DJ-1, and ATP13A2 (Klein and Westenberger, 2012). Additional risk genes have been uncovered by genotyping and whole-exome sequencing, including GBA and VPS35 (Lin and Farrer, 2014). However, SNCA mutations are of particular interest, as they often lead to autosomal dominant, early-onset disease that presents with dementia (Klein and Westenberger, 2012).
The SNCA gene encodes α-Syn, a protein localized to synaptic terminals with roles in vesicle transport and dopamine release (Villar-Piqué et al., 2016). SNCA overexpression or mutation results in production of abnormal α-Syn aggregates known as Lewy bodies. Excessive Lewy body accumulation is thought to be toxic to dopaminergic neurons and is a major hallmark of PD pathology. In addition, α-Syn inclusions typically contain a high proportion of the protein phosphorylated at serine 129, which influences the impact of α-Syn on gene expression and DNA damage, and could therefore be relevant to the pathogenesis of the disease (Zhou et al., 2011; Pinho et al., 2019).
3.1.1 SNCA and familial Parkinson’s disease
Multiple forms of SNCA variation have been linked to familial and sporadic PD. Inherited SNCA duplications and triplications alter dosage and can lead to early-onset PD, with severity correlated to the degree of SNCA overexpression (Lesage and Brice, 2009; Klein and Westenberger, 2012). Rare inherited SNCA point mutations, including A53T, E46K, and A30P, are also associated with severe disease. The biochemical characteristics of these SNCA variants have been primarily studied in cell culture and animal models. SNCA multiplications are often replicated by overexpressing human α-Syn anywhere from two-to fivefold, producing excess protein, which is also seen in human multiplication carriers (Oliveira et al., 2015; Paiva et al., 2017; Wassouf et al., 2018). SNCA point mutations can also be studied by expressing mutant human α-Syn constructs in cell lines or animal models (Jo et al., 2002; Kontopoulos et al., 2006; Freichel et al., 2007; Paiva et al., 2017, 2018).
3.1.2 SNCA and sporadic Parkinson’s disease
SNCA can influence susceptibility to not only familial PD, but also to sporadic PD. The contributions of SNCA and other genes to the sporadic form of the disease have primarily been assessed using linkage or genome-wide association studies (GWAS) in human populations. Minor variants in a large number of genes discovered in these populations, including UCHL1, MAPT, and APOE, have been shown to influence penetrance, age of onset, severity, and progression of PD (Lesage and Brice, 2009; Klein and Westenberger, 2012). The total contribution of common genetic variants to sporadic PD risk was estimated to be 22% (Nalls et al., 2019). GWAS have implicated up to 90 loci in sporadic PD, including several SNPs at different positions in SNCA (International Parkinson’s Disease Genomics Consortium and Wellcome Trust Case Control Consortium, 2011; Escott-Price et al., 2015; Nalls et al., 2014, 2019; Chang et al., 2017; Goldman et al., 2019; Blauwendraat et al., 2020; Ihle et al., 2020). Variations in the length of the REP1 allele, a dinucleotide repeat found in the SNCA promoter, have also been shown to influence PD susceptibility (Chiba-Falek and Nussbaum, 2001; Maraganore et al., 2006).
3.2 Environmental factors influencing Parkinson’s disease risk
Several lines of evidence from human and animal studies indicate that lifestyle-related factors are associated with PD risk. In human epidemiological studies, smoking, coffee consumption, and exercise have been shown to reduce the risk of developing PD, while pesticide exposure, dairy consumption, and brain injury have been shown to increase risk of PD (Ascherio and Schwarzschild, 2016). The influence of the environment on PD risk may be partially mediated through alterations to the epigenome (Ho et al., 2012; Angelopoulou et al., 2022). This review focuses on the epigenomic impacts of pesticide exposure, one of the best-validated risk factors for PD, and physical activity, one of the best-validated protective factors against PD.
3.2.1 Pesticide exposure
The influence of pesticide exposure on PD risk was first discovered in observational studies (Supplementary Table S3). In 1983, 1-methyl-4-phenyl-1,2,3,6-tetrahydropyridine (MPTP), a compound found in heroin that has properties similar to the herbicide paraquat, was found to cause parkinsonism in drug users (Langston et al., 1983; Pang et al., 2019). Elevated PD incidence rates have also been reported in communities with pesticide-contaminated well water as the main source of drinking water (Rajput et al., 1987; Logroscino, 2005). Subsequently, epidemiological studies linked pesticide exposure to increased risk of sporadic PD in larger populations (Elbaz et al., 2009; Tanner et al., 2011; Pouchieu et al., 2018) (Supplementary Table S3). These pesticides and other environmental neurotoxins have been suggested to enter the body through the olfactory system or digestive tract, inducing inflammation, oxidative stress, and/or mitochondrial toxicity, and may initiate α-Syn neuropathology (Tanner et al., 2011; Chen and Ritz, 2018). Exposure to these chemicals was shown to induce parkinsonian molecular and behavioral phenotypes in cell culture and animal models, lending experimental support to studies performed in human populations (Marey-Semper et al., 1995; Betarbet et al., 2000; Przedborski et al., 2001; McCormack et al., 2002; Peng et al., 2004) (Supplementary Table S3).
Several lines of evidence also suggest that pesticide exposure interacts with the genome and epigenome to influence PD risk (Supplementary Table S4). For example, the LRRK2 G2019S mutation increases the inflammatory response to paraquat in mice; DAT variants and herbicide exposure can jointly influence PD risk; and CYP2D6 variants are associated with altered DNAm and PD risk (Elbaz et al., 2004; Ritz et al., 2009; Tiili et al., 2015; Rudyk et al., 2019). Taken together, these observations suggest that pesticides may impact PD etiology through direct and/or indirect mechanisms. Further research regarding the interactions of such exposures with the genome and epigenome are required to identify specific pathways that lead to PD and to facilitate the targeting of these pathways in personalized medicine strategies.
3.2.2 Physical activity and enriched environment
In addition to the impact of toxin exposures on PD risk, the role of lifestyle-related factors in PD prevention has also been investigated. Exercise has been highlighted as having a protective effect against neurodegeneration, and was shown to be associated with reduced risk of PD in prospective cohort studies (Sasco et al., 1992; Chen et al., 2005; Crotty and Schwarzschild, 2020) (Supplementary Table S5). In addition, randomized controlled trials suggested that exercise may also improve motor symptoms of PD (Fisher et al., 2008; Rawson et al., 2019; Sujkowski et al., 2022). Exercise has been shown to ameliorate the effects of aging and neurodegeneration by a number of mechanisms, including increases in brain-derived neurotrophic factor (BDNF) and dopamine release, increased synaptic plasticity, and stabilization of antioxidant responses (Wassouf and Schulze-Hentrich, 2019; Crotty and Schwarzschild, 2020). In rodents, the neuroprotective effects of exercise, increased cognitive stimulation, and increased social stimulation can be modeled with an “enriched environment” (EE) paradigm, consisting of housing more mice per cage with increased access to toys and exercise wheels (Wassouf and Schulze-Hentrich, 2019). Housing mice in an EE has been shown to reduce aging and inflammatory phenotypes, and to remodel gene expression, DNA modifications, and histone modifications (Irier et al., 2014; Wassouf et al., 2018; Zhang et al., 2018; Espeso-Gil et al., 2021; Zocher et al., 2021) (Supplementary Table S5). Exercise is also associated with reduced α-Syn levels in the mouse brain (Zhou et al., 2017). Taken together, the results of human and rodent studies provide good support for the neuroprotective effects of exercise against PD and suggest several potential underlying mechanisms. Future studies should continue to build upon this work by assessing the interactions of exercise with PD genetic risk, environmental exposures, and the epigenome.
4 Epigenetic embedding of genetic and environmental influences
4.1 Epigenetics, DNA methylation, and DNA hydroxymethylation
Waddington introduced the concept of the “epigenetic landscape” in 1957, referring to the effects of gene regulation on phenotype during cellular differentiation and development (Waddington, 1957). Riggs later defined “epigenetics” as mitotically heritable factors other than the DNA sequence that can shape cellular phenotype (Russo et al., 1996). A number of adjustments to this definition have since been proposed, which take into account chromosomal structure, cellular reprogramming, and cellular tissue composition (Bird, 2007; Lappalainen and Greally, 2017).
Modern definitions of “epigenetics” typically encompass protein and DNA modifications that can affect transcription, such as DNAm and histone posttranslational modifications. Epigenetic factors play crucial roles in cellular/tissue differentiation, development, and aging. For example, DNAm helps to guide neurogenesis, and widespread DNAhm changes are observed at genes involved in synaptic function, dendrite morphogenesis, and axon guidance in the developing fetal brain (Spiers et al., 2017; Stricker and Götz, 2018). Aging is also associated with widespread changes to DNAm at genes involved in DNA repair, apoptosis, cellular metabolism, and other key pathways (Jones et al., 2015; Slieker et al., 2016; Horvath and Raj, 2018).
4.1.1 CpG methylation
DNA methylation is one of the most extensively characterized epigenetic marks in human population studies, and is involved in all of the above processes from differentiation to development, aging, and disease (Cutfield et al., 2007; Levine et al., 2018; Stricker and Götz, 2018). Both cytosine and adenine bases can be methylated, with 5-methylcytosine (5mC) representing the most commonly methylated DNA base in the human genome (Kumar et al., 2018). 5mC has a methyl group attached to the fifth carbon of the pyrimidine ring of cytosine and can occur in the CpG, CHG, or CHH context (with CHG and CHH being examples of non-CpG methylation, referred to as CpH methylation where H represents either A, C, or T). Approximately 4% of the cytosines in the human genome are methylated, including 80% of CpG dinucleotides and 2%–6% of CpH sites (Lister et al., 2013; Kumar et al., 2018). Cytosine methylation is catalyzed by the DNA methyltransferase (DNMT) family of enzymes, including DNMT1, which maintains DNAm patterns during cell division, and DNMT3A and DNMT3B, which deposit de novo DNAm (Kumar et al., 2018) (Figure 1A).
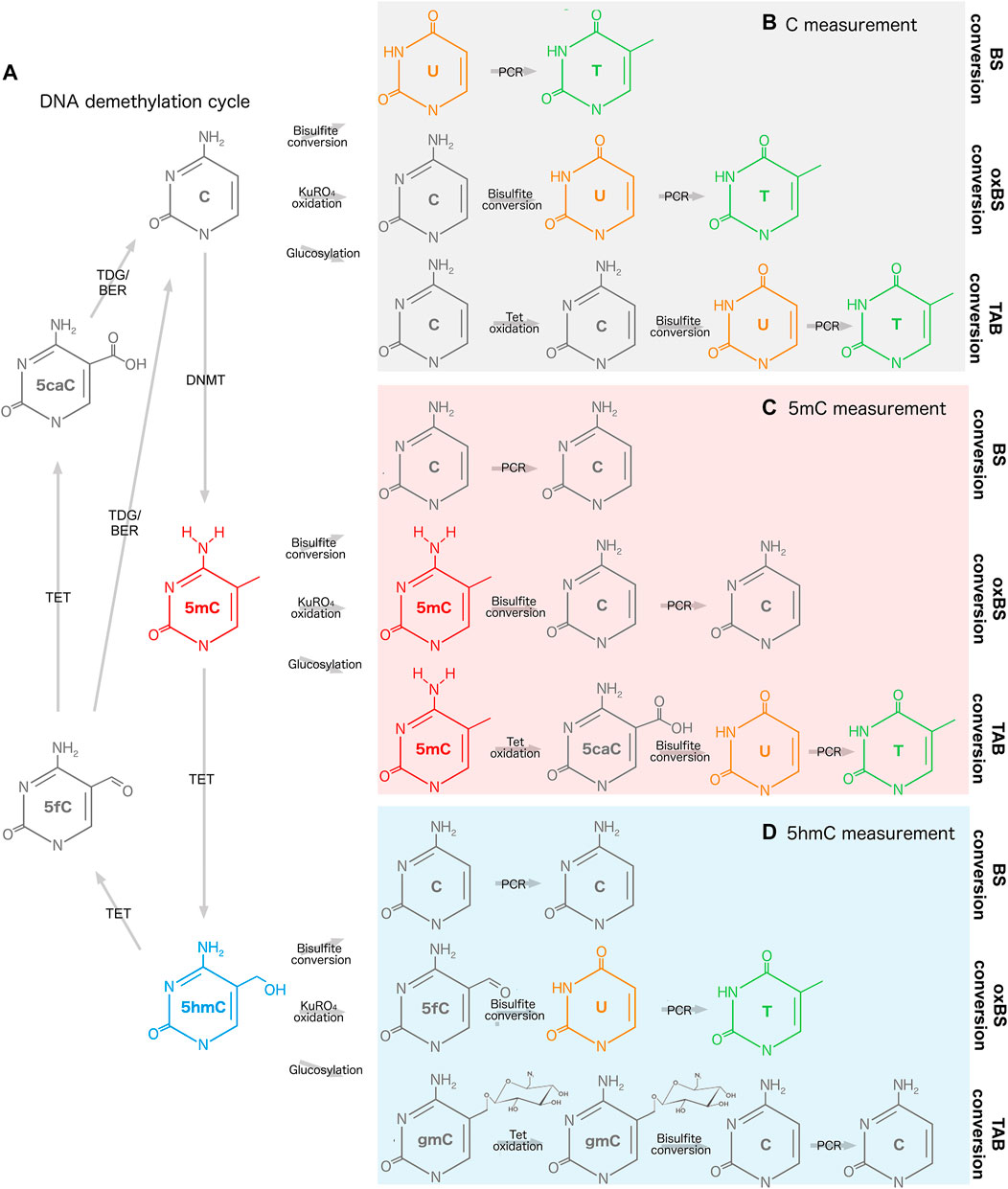
FIGURE 1. Measurement of DNA methylation and hydroxymethylation. (A) DNA demethylation cycle and reaction intermediates. (B) Bisulfite, oxidative bisulfite, and TET-assisted bisulfite conversion of cytosine. (C) Bisulfite, oxidative bisulfite, and TET-assisted bisulfite conversion of 5mC. (D) Bisulfite, oxidative bisulfite, and TET-assisted bisulfite conversion of 5hmC. 5caC, 5-carboxylcytosine; 5fC, 5-fluorocytosine; 5hmC, 5-hydroxymethylcytosine; 5mC, 5-methylcytosine; BER, base excision repair; BS, bisulfite; C, cytosine; DNMT, DNA methyltransferase; gmC, β-glucosyl-5-hydroxymethylcytosine; oxBS, oxidative bisulfite; PCR, polymerase chain reaction; T, thymine; TAB, TET-assisted bisulfite; TDG, thymine DNA glycosylase; TET, Ten-Eleven Translocation; U, uracil.
CpG sites are found in varying densities across the genome, with the highest concentration in evolutionarily conserved CpG island promoters. DNAm at regions flanking CpG islands, referred to as CpG shores, plays an important role in establishing differences between tissues during development (Irizarry et al., 2009; Lokk et al., 2014). The roles of CpG methylation in other areas of the genome are complex and highly dependent on context, varying according to tissue, CpG density, and other factors, including developmental stage and age. Deposition of DNAm at CpG island promoters can silence gene expression through steric hindrance of transcription initiation complex binding or recruitment of additional repressive protein complexes through methyl-binding domains (Deaton and Bird, 2011). Conversely, CpG methylation in gene bodies is often associated with active transcription and may play a role in splicing regulation (Jones, 2012). Finally, DNAm may be laid down at some promoters and enhancers following gene silencing to maintain a transcriptionally repressive state (Jones, 2012).
4.1.2 CpG hydroxymethylation
The Ten-Eleven Translocation (TET) family of methylcytosine dioxygenases actively removes DNAm from CpG sites, producing 5-hydroxymethylcytosine (5hmC) as the first intermediate in a multistep oxidation process (Wu and Zhang, 2011) (Figure 1A). While DNAhm was initially hypothesized to function only as a reaction intermediate, further studies supported an independent function for DNAhm, particularly in the brain (Szulwach et al., 2011; Wen and Tang, 2014; Kinde et al., 2015). DNAhm is highly enriched in human brain tissue, accounting for 17%–30% of all modified cytosines in the adult frontal cortex (Lister et al., 2013; Wen et al., 2014). DNAhm patterns change in response to neuronal activity, development, and aging, and may be key components of normal brain function (Szulwach et al., 2011). This epigenetic mark is also found at lower levels across a variety of human tissues, and may be involved in regulation of tissue-specific gene expression (He et al., 2021). However, the role of 5hmC in human gene regulation has not been fully elucidated, and its relevance to neurological health and disease is currently an area of active research.
4.1.3 Non-CpG methylation
In contrast to CpG methylation, CpH methylation occurs primarily in embryonic stem (ES) cells and neurons, and is estimated to represent half of the methylated cytosines in adult human and mouse neurons (Kinde et al., 2015). CpH methylation has been suggested to be a transcriptionally repressive mark due to its depletion in upstream, downstream, and body regions of actively transcribed genes (Lister et al., 2013; Guo et al., 2014; Kinde et al., 2015). The abundance of such CpH methylation in the brain and its alterations in disease suggest that this is an important epigenetic mark in the context of brain health and development (Blanch et al., 2016; Fuso et al., 2016; Nicolia et al., 2017). However, further research is needed to determine whether and to what degree CpH methylation is sensitive to environmental and lifestyle-related factors.
4.2 Factors influencing DNA methylation and DNA hydroxymethylation
DNA methylation can be influenced by a myriad of genetic and environmental factors, from SNPs and copy number variation (CNV) to exposures and lifestyle-related factors, such as diet, pollution, and stress. The impacts of these factors on CpG methylation have been well characterized, while their impacts on DNAhm and CpH methylation are less well understood. This section first addresses the influences of genetics and environment on CpG methylation patterns.
4.2.1 Influences of genetics and environment on CpG methylation
4.2.1.1 Methylation quantitative trait loci
Although DNAm has frequently been proposed as a mediator of environmental and lifestyle impacts on health and disease susceptibility, it has recently become clear that genetic variants have a significant influence on CpG methylation, in some cases to a larger degree than environmental exposures. Genetic influences account for approximately 20%–80% of overall variation in DNAm, with a mean genome-wide CpG methylation heritability of 0.19 in twins (Gertz et al., 2011; Cheung et al., 2017; Husquin et al., 2018; Villicaña and Bell, 2021). Genetically driven CpG methylation is often considered in the context of methylation quantitative trait loci (mQTLs), which refer to SNPs where genotype impacts DNAm level (Banovich et al., 2014). It has been reported that 93% of mQTLs influence CpG methylation in cis, within 1 Mb of the affected CpG site (Gaunt et al., 2016). Approximately half of all mQTLs are located in introns, and they are also more likely to be represented in microRNA binding sites than expected by chance (Smith et al., 2014). Many mQTLs discovered in blood are consistent across tissues, developmental stages, and ethnicities. For example, two studies found that 18.5%–31.6% of blood mQTLs were also present in brain; 34%–73% of blood mQTLs overlapped between blood, brain, and saliva; 44.1%–50.7% overlapped between umbilical cord blood and adult peripheral blood; and 21.3%–69.5% of umbilical cord blood mQTLs overlapped between African and Caucasian infants (Smith et al., 2014; Liu et al., 2019). mQTL status is also highly consistent throughout life from birth to middle age (Gaunt et al., 2016).
Identification of relevant mQTLs can aid in understanding the mechanisms underlying complex diseases with varied genetic and environmental etiologies. For example, loci shown in GWAS to be associated with neurological and psychiatric disorders, cholesterol level, bone density, and blood pressure are enriched in mQTLs, suggesting that genetically driven CpG methylation patterns may be important in the context of health and disease (Gaunt et al., 2016; Liu et al., 2019). Several PD-associated SNPs have been reported or predicted to affect DNAm based on publicly available datasets, and PD GWAS data have been used to predict DNAm levels at thousands of CpGs, suggesting a key role of genetic variation in determining PD-associated DNAm patterns (International Parkinson’s Disease Genomics Consortium and Wellcome Trust Case Control Consortium, 2011; Nalls et al., 2014; Rawlik et al., 2016; Kia et al., 2021). It will be necessary to determine the degrees to which genetic variants affect CpG methylation as well as the directions of these effects to understand complex disorders, and it may be possible to apply such approaches to peripheral tissues to obtain informative results (Islam et al., 2019).
4.2.1.2 Other genetic influences on CpG methylation
It is also important to consider the influences of non-SNP genetic factors on CpG methylation in the context of gene regulation, health, and disease. For example, allele-specific DNAm occurs at imprinted regions and as a consequence of X chromosome inactivation, a phenomenon in which one of the X chromosomes in females is epigenetically silenced; defects in these mechanisms can lead to developmental disorders (Horsthemke, 2014; Wang H. et al., 2019a). Deletions, duplications, or rearrangements of gene regions, entire genes, or larger genomic segments, such as CNV, can also affect CpG methylation through direct or indirect mechanisms. Some CNV regions may physically interact with CpG sequences, and disruption of these interactions affects the ability of DNMTs to access these sequences and catalyze CpG methylation. Other CNV contain transcription factor binding sites, which may alter gene expression and transcription-associated DNAm changes. Alternatively, dosage changes in cell signaling proteins and messengers can affect physiological signaling cascades that ultimately influence gene transcription and DNAm (Shi et al., 2020). In the context of familial PD, whole-gene multiplications of the SNCA locus may impact the epigenome through some of these mechanisms. For example, increased dosage of α-Syn in dopaminergic neurons impacts genome-wide DNAm and DNAhm patterns (Schaffner et al., 2022). However, the molecular epigenetic effects of SNCA CNV in human PD patients have not been fully elucidated.
4.2.1.3 Environmental influences on CpG methylation
While genetic factors are known to influence DNAm patterns, there is also a large body of evidence for the plasticity of CpG methylation in response to the environment (Feinberg, 2007; Aguilera et al., 2010; Ho et al., 2012; Cavalli and Heard, 2019; Angelopoulou et al., 2022). A number of factors, including smoking, nutrition, and early life stress, are associated with altered DNAm in humans (Lim and Song, 2012; Lee and Pausova, 2013; Matosin et al., 2017). These environmental and lifestyle-related factors also influence PD risk, which may be partially mediated by impacts on the epigenome (Chen and Ritz, 2018; Angelopoulou et al., 2022). Therefore, it is crucial to understand the environmental influences on CpG methylation to investigate the role of DNAm in PD etiology, for the design of epigenetic studies of PD, for selecting appropriate statistical approaches to minimize confounding, and to explore whether lifestyle interventions for PD act through epigenetic mechanisms.
Environment-induced DNAm changes can also be partly influenced by genetic background, which will be a key consideration in the design of future epigenetic studies of PD. For example, in neonatal umbilical cord blood, the interaction of genotype and in utero environment was found to explain 75% of DNAm variation across variably methylated regions (Teh et al., 2014). A similar analysis in neonates showed that additive and interaction effects of genotype and environment explained 29.41% and 40.58% of DNAm variance, respectively, while environment alone explained only 0.03% (Czamara et al., 2019). Environment-driven variation in the epigenome has been proposed to act as a “second hit” on top of genotype, possibly triggering onset of disorders to which an individual is genetically predisposed, such as CNV-associated neuropsychiatric conditions (Girirajan et al., 2010). The influence of environmental and lifestyle-related factors on DNAm may also partially ameliorate disease risk. For example, exercise is associated with DNAm changes in skeletal muscle, and bariatric surgery has been shown to partially reverse diet-induced DNAm changes in glucose uptake genes (Barrès et al., 2012; Multhaup et al., 2015). The impact of environment on the DNA methylome is particularly crucial during critical periods of early development, and DNAm has been proposed to be one of the mediators of developmental origins of health and disease (Barker, 1990; Cutfield et al., 2007). There is currently a great deal of active research regarding the possibility of disease prevention strategies targeting the epigenome, as well as epigenetic therapies that could be applied later in life.
4.2.2 Influence of genetics and environment on CpG hydroxymethylation
Similar to CpG methylation, it is also important to understand the impacts of genetics and environment on CpG hydroxymethylation and non-CpG methylation for epigenetics studies of PD. As these two marks are enriched in the brain, have the potential to influence gene transcription, and may also be affected by PD genetic and environmental risk factors, it may be prudent to incorporate analysis of DNAhm and/or CpH methylation into studies of PD etiology.
The degree to which DNAhm is susceptible to genetic and environmental influence is still under investigation. However, a small number of hydroxymethylation quantitative trait loci (hmQTLs) have been identified in the fetal brain, and human tissue-specific differentially hydroxymethylated regions have been shown to contain SNPs linked to tissue-specific diseases and phenotypes (Spiers et al., 2017; He et al., 2021). Changes in 5hmC have also been reported to be associated with exposure to stress, environmental enrichment, toxins, and diet (Irier et al., 2014; Hack et al., 2016; Kochmanski and Bernstein, 2020). Finally, several studies have implicated DNAhm in neuronal activity-regulated gene expression, learning and memory, and neuroplasticity, all of which are likely to involve external environmental changes and experience (Kaas et al., 2013; Li et al., 2014). However, some of these studies measured 5hmC on a global level. A shift toward base-pair-resolution 5hmC analysis is needed to develop a comprehensive understanding of the genes and pathways affected by environmental alterations in this epigenetic mark and its significance for disease development and prevention (Kochmanski and Bernstein, 2020).
4.2.3 Influence of genetics and environment on non-CpG methylation
Little is known about the impacts of genetics and environment on CpH methylation patterns. Some allele-specific CpH methylation has been observed in the context of schizophrenia, with lower levels of CpH methylation surrounding SNPs that correspond to schizophrenia risk haplotypes (Alfimova et al., 2020). CpH methylation changes have also been reported at 750 cytosines, primarily occurring in introns, in the hippocampi of mice housed in EE (Zocher et al., 2021). While DNAm at introns has the potential to influence transcription, differential CpH methylation was also shown to be depleted at promoters, CpG islands, and CpG shores in this study, making it difficult to draw any definitive conclusions about potential gene regulatory impacts (Zocher et al., 2021). Neuronal CpH methylation has been suggested to be more sensitive to environmental stimuli than neuronal CpG methylation due to its sparsity and replication independence (Fuso and Lucarelli, 2019). Further research is needed to determine whether this holds true, and to elucidate the full extent of genetic and environmental effects on CpH methylation.
4.3 Methods for measuring DNA modifications
Both when evaluating existing PD EWAS and when designing new experiments, it is valuable to consider the method used to profile genome-wide DNAm to understand its limits and biases. For example, studies in brain tissue may be biased by high levels of DNAhm, which are picked up in one compound signal when bisulfite conversion of DNA is applied (Darst et al., 2010). If bisulfite conversion is paired with oxidative or TET-assisted bisulfite conversion, 5mC and 5hmC levels can be estimated simultaneously (Booth et al., 2013; Yu et al., 2018) (Figure 1). The platform used for DNAm profiling will also influence the results. Illumina microarrays are often used for population-level studies and provide good coverage of regulatory elements, but overall sparse coverage of the genome (Bibikova et al., 2009; Pidsley et al., 2016). In contrast, next-generation sequencing approaches are often used with fewer samples, but may cover the genome at greater depth (Gu et al., 2011; Kernaleguen et al., 2018). There are also a multitude of statistical considerations for quality control and normalization of microarray and sequencing data, simultaneous 5mC/5hmC estimation in paired samples, and deconvolution of cell types in heterogeneous tissues (Krueger and Andrews, 2012; Guintivano et al., 2013; Pidsley et al., 2013; Liu and Siegmund, 2016; Lunnon et al., 2016; Xu et al., 2016; Kaushal et al., 2017; Zhang Y. et al., 2022a). Robust DNA modification studies should take all of the above into account to reduce bias and increase inferential capability. Further considerations for DNA modification profiling are reviewed in Laird, 2010 and Skvortsova et al., 2017.
5 Epigenetic contribution to Parkinson’s disease
DNAm and DNAhm are particularly attractive as candidates to identify and characterize gene–environment interactions in PD because of the large proportion of sporadic cases, and as potential modifiers of familial disease (Figure 2). Local and genome-wide DNAm alterations and impacts on the DNAm machinery have been reported to be associated with genetic and environmental risk factors for PD, suggesting that DNAm and/or DNAhm could be involved in the etiology of the disease (Dunn et al., 2019; Angelopoulou et al., 2022). Some of these DNAm changes are correlated with changes in gene expression, and may therefore represent functionally relevant molecular profiles involved in the development of PD. For example, decreased DNAm at SNCA intron one is associated with increased SNCA mRNA expression in PD patients (Jowaed et al., 2010). Differentially methylated genes in PD patients are enriched for cell–cell communication and apoptotic pathways, also suggestive of a potential etiological role of this epigenetic modification (Kaut et al., 2012; Masliah et al., 2013).
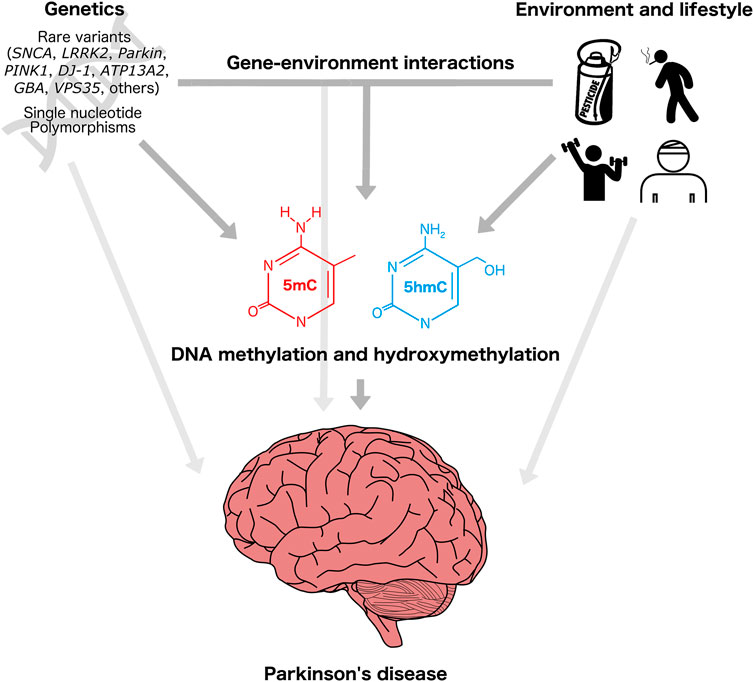
FIGURE 2. Genetic, environmental, and epigenetic underpinnings of Parkinson’s disease. Individual genetic background, environmental exposures (e.g., pesticides), and lifestyle-related factors (e.g., exercise, smoking, head trauma) influence PD risk. These factors may act directly or indirectly through modification of DNA methylation and/or DNA hydroxymethylation patterns, which in turn can influence the regulation of genes involved in neurodegenerative pathways. Head injury icon created by George E. Thomposon (thenounproject.com).
In addition to its usefulness in understanding the etiology of PD, DNAm may represent a suitable early-stage biomarker for the disease. DNAm has characteristics that make it an ideal biomarker due to its relative stability and detectability in peripheral tissues, such as blood and saliva (Edgar et al., 2017; Islam et al., 2019). One study in matched brain and blood samples showed that CpG methylation patterns associated with PD were approximately 30% concordant across these tissues, suggesting that certain loci may be able to act as biomarkers in blood (Masliah et al., 2013).
5.1 Influence of SNCA and other Parkinson’s disease risk genes on DNA methylation
Studies using animal and cell culture models showed that the protein product of the PD risk gene SNCA, α-Syn, is localized to the nucleus and has several potential avenues for impacting epigenetic regulation (Figure 3). First, nuclear α-Syn can reduce p300 histone acetyltransferase activity and inhibit histone H3 acetylation (Kontopoulos et al., 2006; Jin et al., 2011; Paiva et al., 2017). Second, α-Syn can bind to DNA, regulating the transcription of genes responsive to retinoic acid signaling and relevant for PD (Martins et al., 2011; Davidi et al., 2020). Third, α-Syn was shown to sequester the maintenance DNA methylation enzyme DNA methyltransferase 1 (Dnmt1) from the nucleus to the cytoplasm in mice, potentially reducing the capacity for maintenance of CpG methylation (Desplats et al., 2011). In addition to these mechanisms, the presence of excess wild type or mutant α-Syn protein throughout the cell may impact epigenetic modifications indirectly as a consequence of altered signaling cascades resulting in epigenetic and transcriptional alterations, changes to cellular metabolism, and/or DNA damage resulting from α-Syn toxicity (Emamzadeh, 2016; Paiva et al., 2017). While these studies provided strong evidence that α-Syn can alter the epigenome, the impacts of expressing α-Syn and its variants on the DNAm level have not been fully characterized. Some experiments have addressed the locus-specific CpG methylation status of mutant α-Syn in lymphoblastoid cells, or investigated the epigenome-wide impacts of other PD-associated mutations, such as LRRK2 G2019S, in PD patient-derived induced pluripotent stem cells (iPSCs) (Voutsinas et al., 2010; Fernández-Santiago et al., 2015, 2019). Recently, wild-type and A30P mutant α-Syn were shown to alter CpG methylation at thousands of loci in dopaminergic neurons, with correlations to expression of glutamate signaling genes (Schaffner et al., 2022). Studies such as these to characterize the effects of α-Syn on the DNA methylome will increase our understanding of the mechanisms underlying genetically driven PD, and whether the epigenetic consequences of SNCA variants can be reversed by lifestyle interventions or targeted therapies, such as epigenome editing (Kantor et al., 2018).
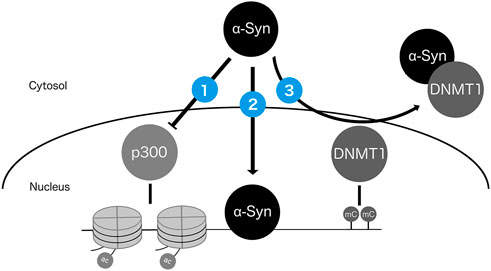
FIGURE 3. Potential mechanisms by which nuclear α-Syn protein can impact the epigenome. α-Syn can (1) inhibit activity of histone acetyltransferase p300 (Kontopoulos et al., 2006; Jin et al., 2011; Paiva et al., 2017); (2) directly bind DNA (Martins et al., 2011; Davidi et al., 2020); and (3) sequester DNMT1 from the nucleus to the cytosol (Desplats et al., 2011).
To date, few population-level studies of sporadic PD have addressed the contribution of genetic variants to DNAm patterns. DNA methylation levels of PD-associated SNPs discovered by GWAS have been assessed, and other studies have used public datasets to associate SNP genotypes with DNAm levels of PD-associated CpGs (International Parkinson’s Disease Genomics Consortium and Wellcome Trust Case Control Consortium, 2011; Nalls et al., 2014; Vallerga et al., 2020; Kia et al., 2021). The incorporation of mQTL analysis with matched samples and/or REP1 genotyping into further human cohort studies may be informative, and help to explain why some associations of DNAm with PD can be replicated, while others cannot.
5.2 Influence of Parkinson’s disease-associated environmental and lifestyle-related factors on DNA methylation patterns
5.2.1 Pesticide exposure and DNA methylation
There have been only a few studies regarding the epigenetic impacts of PD-associated environmental and lifestyle-related factors, such as neurotoxin exposure and exercise. In the context of neurotoxins, one group investigated DNAm patterns in PD patients with and without pesticide exposure, and other studies have examined the impacts of pesticide exposure in healthy individuals; however, there have been no studies involving the assessment of PD patients and controls simultaneously (van der Plaat et al., 2018; Go et al., 2020) (Figure 4A). Global DNAm loss has been reported in organochlorine-exposed hippocampal cell culture, and site-specific DNAm changes at the first intron of SNCA were observed in an MPTP-induced mouse model of PD (Wnuk et al., 2016; Zhao et al., 2019) (Figure 4A). Pesticide exposure may also alter histone acetylation in PD, as MPTP-treated cells and mice showed reduced histone deacetylase (HDAC) expression (Park et al., 2016) (Figure 4A). This pathway may be a viable target for intervention, as a recent study showed that naturally occurring short-chain fatty acids produced by gut microbes influence HDAC activity and can protect against rotenone-induced toxicity in rat dopaminergic neurons (Zhang Z. et al., 2022b). Additional human population studies to assess the associations between pesticide exposure and DNAm in PD and experimental studies in model systems to investigate whether it is mechanistically involved in the pathogenesis of PD would help to further elucidate the potential role of the epigenome in mediating the effects of pesticide exposure on disease risk.
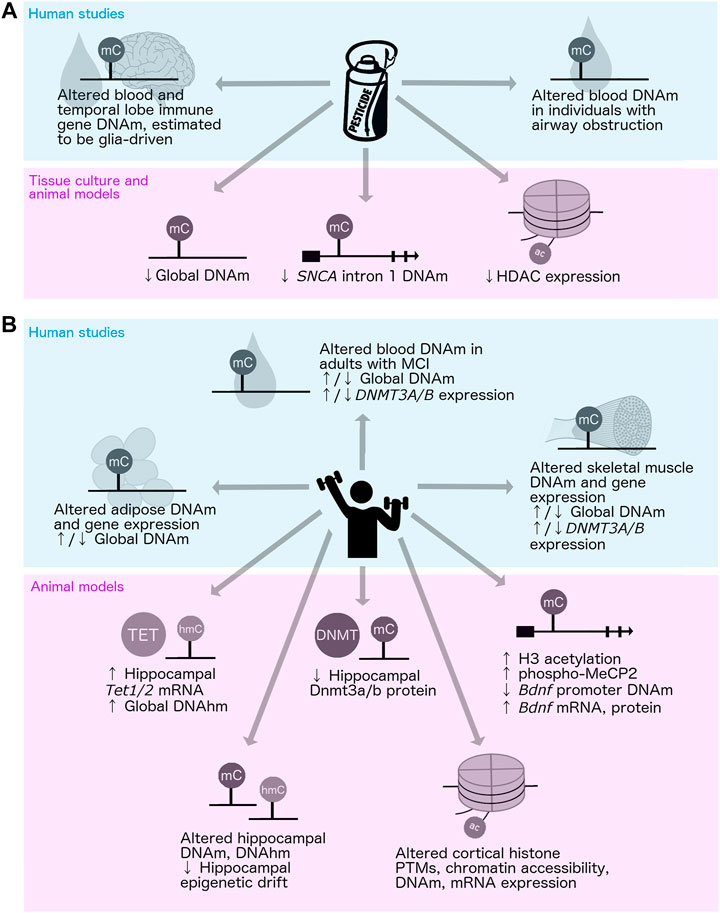
FIGURE 4. Impacts of pesticide exposure and physical activity on the epigenome. Up and down arrows (↑/↓) indicate conflicting results; i.e., both increases and decreases reported across different studies of the same mechanism. (A) Human (top: van der Plaat et al., 2018; Go et al., 2020; Furlong et al., 2020) and tissue culture/animal (bottom: Park et al., 2016; Wnuk et al., 2016; Zhao et al., 2019) studies of pesticide exposure and epigenetic alterations. (B) Human (top: Rönn et al., 2013; Lindholm et al., 2014; Światowy et al., 2021; Ngwa et al., 2022) and animal (bottom: Gomez-Pinilla et al., 2011; Elsner et al., 2013; Irier et al., 2014; Jessop and Toledo-Rodriguez, 2018; Wassouf et al., 2018; Zhang et al., 2018; Zocher et al., 2021; Espeso-Gil et al., 2021) studies of physical activity or enriched environment and epigenetic alterations. DNAhm, DNA hydroxymethylation; DNAm, DNA methylation; HDAC, histone deacetylase; MCI, mild cognitive impairment; PTMs, posttranslational modifications.
5.2.2 Physical activity, enriched environment, and DNA methylation
The impacts of PD-associated behavioral and lifestyle-related factors on DNAm have also been assessed to some extent. The effects of physical activity on DNAm in humans have been widely studied, primarily in skeletal muscle, adipose tissue, and blood (Figure 4B). However, conflicting results have been reported regarding whether and in what direction exercise impacts global DNAm patterns, most likely due to inconsistencies in the age of subjects and the type and duration of exercise applied in these investigations (Światowy et al., 2021). Interestingly, 6 months of exercise was shown to alter DNAm at genes related to amyloid biology, protein trafficking, and lipoprotein regulation in the blood of adults with mild cognitive impairment, suggesting possible impacts on neurologically relevant gene regulation (Ngwa et al., 2022). Most of our knowledge regarding the impacts of exercise on the brain epigenome has come from studies in rodent models (Figure 4B). Physical activity has been shown to increase Tet1 and Tet2 mRNA expression and DNAhm levels in the mouse hippocampus, and to decrease Dnmt1 and Dnmt3b protein levels in the rat hippocampus (Elsner et al., 2013; Jessop and Toledo-Rodriguez, 2018). Exercise was also shown to elevate the levels of phosphorylated MeCP2 in the rat hippocampus, which is associated with reduced DNAm at the Bdnf promoter and increased levels of Bdnf mRNA and protein expression (Gomez-Pinilla et al., 2011). These studies point to DNAm as one mechanism mediating BDNF upregulation and neuroprotection associated with physical activity.
The effects of exercise and increased cognitive and social stimulation on the epigenome have also been studied in rodents using EE paradigms (Figure 4B). These studies showed that environmental enrichment can alter hippocampal DNAm and DNAhm, and prevent some SNCA-induced alterations in gene expression (Irier et al., 2014; Wassouf et al., 2018; Zhang et al., 2018; Zocher et al., 2021). Intriguingly, EE was shown to reduce age-related epigenetic drift at the level of DNAm in the hippocampus, and to alter histone modifications, chromatin accessibility, DNAm, and gene expression in the cortex in mice (Espeso-Gil et al., 2021; Zocher et al., 2021). These studies support a potential role of DNAm as a mediator of the impacts of behavior and lifestyle-related factors on PD risk.
5.3 CpG methylation alterations in individuals with Parkinson’s disease
Global, gene-specific, and genome-wide DNAm alterations have been identified in PD patients. Lower levels of global DNAm have been reported in the brains of PD patients compared to controls (Kaut et al., 2012). This may be related to α-Syn-mediated sequestration of DNMT1 from the nucleus to the cytoplasm and/or targeting of DNMT1 by microRNA(s), perturbing neuronal DNAm patterns (Desplats et al., 2011; Zhang et al., 2021). Alterations to DNAm have also been demonstrated at specific loci in PD across several tissues, including the postmortem brain, PD patient-derived neurons, blood, and saliva (Supplementary Tables S1, S2). Increased DNAm has been reported at intron one of the SNCA gene in PD, but a correlation with α-Syn expression is controversial (Jowaed et al., 2010; de Boni et al., 2015). In addition to SNCA, several EWAS have reported site-specific changes in CpG methylation across a number of genes in individuals with PD, including CYP2E1, MIR886, MAPT, LARS2, and SLC7A11 (Masliah et al., 2013; Rawlik et al., 2016; Chuang et al., 2017; Henderson-Smith et al., 2019; Vallerga et al., 2020; Kaut et al., 2022) (Supplementary Tables S1, S2). Attempts to replicate the results of these EWAS have often failed due to factors such as cell type heterogeneity, small sample sizes, and differences in study design and data collection/processing. Larger populations and more robust methods of accounting for tissue differences, disease heterogeneity, and mixed ethnicity of study populations are required to produce reliable and replicable EWAS results for PD.
Aside from such studies focusing on DNAm patterns associated with PD diagnosis, some groups have begun to investigate how medications and pesticide exposures can affect DNAm patterns in PD (Schmitt et al., 2015; Furlong et al., 2020). However, it is unclear whether environmental and lifestyle-related factors, such as pesticide exposure, coffee consumption, and diet, can influence or mediate DNAm changes associated with PD risk in undiagnosed individuals (Angelopoulou et al., 2022).
5.4 CpG hydroxymethylation alterations in individuals with Parkinson’s disease
The study of DNAhm patterns in PD is also in its infancy. Recent work has shown that 5hmC is enriched globally in the white matter of PD patients as well as at enhancers in the neurons of PD patients (Kaut et al., 2019; Marshall et al., 2020). One of these groups also determined that PD patients exhibit upregulation of TET2, and that TET2 depletion can prevent dopaminergic neuron loss in mouse and cell culture models of PD (Marshall et al., 2020). These observations represent promising early evidence that DNAhm may be involved in the pathogenesis of PD, and therefore DNAhm changes in PD warrant further characterization.
5.5 Non-CpG methylation in individuals with Parkinson’s disease
CpH methylation may also play a role in neurodegeneration, and has been shown to be enriched at genes involved in immune, neuroinflammation, and neurodegeneration-related pathways (Lee et al., 2020). CpH methylation changes have been reported in mitochondrial DNA from the substantia nigra of PD patients (Blanch et al., 2016). However, the majority of research in the context of PD disease etiology and biomarker potential to date has focused on CpG methylation. Future studies should continue to assess disease-associated cytosine modifications, including CpH methylation and DNAhm, to determine whether they have causal relationships with PD phenotypes.
6 Multi-omics integration approaches in neuroepigenetics research
Although both DNAm and DNAhm are interesting facets of the epigenome that may explain some of the variation observed in PD, the relationships of DNAm and DNAhm with gene expression are highly complex, making it difficult to draw functional conclusions from studies of the DNA methylome alone. Multi-omics profiling and data integration approaches can help to prioritize functionally relevant epigenetic loci, develop better predictive biomarkers, and in certain cases aid in determining causality (e.g., when genetic data are included and/or when data are sampled across multiple time points). This is particularly useful for understanding the etiology and progression of complex diseases and for developing personalized medicine strategies (Peterlin and Maver, 2012; Cazaly et al., 2019; Li et al., 2021).
Multi-omics integration can be accomplished using a wide range of approaches that vary in terms of their data inputs, assumptions, and qualitative versus quantitative nature, with no universally accepted “gold standard” (Richardson et al., 2016; Cazaly et al., 2019; Li et al., 2021). On the quantitative end, linear regression can be used to model one data type as the dependent variable and another as the independent variable (Stingo et al., 2011; Kim and Xing, 2012; Marttinen et al., 2014; Zhang et al., 2019). However, when both datasets are high dimensional, such as genome-wide DNAm, DNAhm, and gene expression profiles, it may be necessary to run thousands of models in a time- and computationally intensive manner, and multiple test correction can heavily penalize the results. Hierarchical clustering can also be used to find related features among such datasets (Curtis et al., 2012; Lock and Dunson, 2013; Singh et al., 2019). More qualitative approaches seek to identify common pathways, functions, and/or networks of genes profiled by multi-omics approaches, including gene ontology (GO) enrichment analysis, chromatin state prediction, and coexpression network analysis, among others (Ernst and Kellis, 2012; Wijetunga et al., 2017; Reimand et al., 2019). Finally, machine learning approaches can be applied to multiple omics layers in a supervised or unsupervised manner, selecting features that correlate with phenotype (Singh et al., 2019; Li et al., 2021).
While many different omics approaches have been applied in PD, only a few studies to date have combined multiple data types (Redenšek et al., 2018; Wang C. et al., 2019b; Henderson et al., 2021; Kia et al., 2021; Caldi Gomes et al., 2022). One group integrated genomics, transcriptomics, and proteomics using a position-based method, and discovered 29 dynamically regulated genes associated with PD (Maver and Peterlin, 2011). Another group compared the results of separate RNA-sequencing and proteomics analyses, and found that only 10/3,558 genes with differential mRNA expression in PD also had altered protein levels (Dumitriu et al., 2015). Importantly, this suggests that assumptions about downstream gene expression and function cannot be extrapolated from the results obtained using a single omics approach. More recently, two separate studies integrated gene expression and DNAm profiles in blood of PD patients. One study used linear regression to construct a biomarker panel of 85 genes with decreased expression and increased DNAm in PD, while the other applied a network-based approach and found six PD-related regulatory modules with altered expression and DNAm (Wang C. et al., 2019b; Henderson et al., 2021). A similar network approach was applied to discover modules of differentially expressed genes at the levels of mRNA, microRNA, and/or protein in PD (Caldi Gomes et al., 2022). Finally, multiple large-scale, publicly available omics datasets have been used to test hypotheses about how genetic variants influence gene expression, splicing, and DNAm in the brains of PD patients (Kia et al., 2021). These studies provide excellent examples of how multi-omics approaches combined with analyses of DNAm can be used to understand the impacts of genetic variants on the epigenome and transcriptome as well as to classify disease risk.
In addition to combining multiple types of omics data, several studies also explored single-omics network integration approaches in PD, using PD-associated candidate genes found in the literature or gene coexpression profiles to identify regulatory hubs (Liu et al., 2012; Chatterjee et al., 2017). Finally, global DNAm and DNAhm patterns in PD have been compared in the same individuals; however, it is still unclear how these two cytosine modifications interact at the site-specific level (Kaut et al., 2019). While the application of integrated multi-omics approaches toward understanding the pathogenesis of PD is still in its infancy, these recent studies provided promising evidence that leveraging multiple datasets can provide further insight regarding disease etiology than relying on one omics approach at a time.
7 Conclusion and future perspectives
Some of the greatest challenges in PD epigenetics research include complex interindividual etiology, disease heterogeneity, gradual progression, and the inaccessibility of human brain tissue to study the disease in real time. In addition, tissue culture and animal model studies cannot completely recapitulate human PD. However, the future of PD research is promising with the availability of a wide range of tools and resources to continue examining the etiological underpinnings and identify early-stage biomarkers of the disease. For example, analyses of patient cohorts in combination with model systems in the same study will facilitate the identification of loci associated with PD in humans, as well as providing a highly controlled setting in which to test the functionality of these variants and identify disease mechanisms. To address the issue of heterogeneity, experimental designs may also target associations with PD endophenotypes rather than taking a case–control approach. These two strategies have been successfully applied in a study of Alzheimer’s disease, where GWAS was used to identify variants associated with cerebrospinal fluid triggering receptor expressed on myeloid cells 2 (TREM2) levels, and tissue culture experiments were conducted to examine the functional implications of these variants (Deming et al., 2019). Secondary data analysis is also now possible due to the availability of large genomic databases, such as the UK Biobank, a prospective longitudinal study with detailed phenotyping that is estimated to include 14,000 individuals who will develop PD (Sudlow et al., 2015). The availability of large, well-phenotyped cohorts allows researchers to ask new questions regarding the etiology of PD as the field evolves. In addition, the breadth of integrated multi-omics methods and causal inference tools, such as Mendelian randomization, will aid in pinpointing gene regulatory networks related to disease. Overall, research in PD epigenomics will soon be limited only by the ability to ask pertinent research questions and apply creative analysis methods to uncover the “missing heritability” of this disorder.
Author contributions
SS and MK conceptualized the article. SS wrote the article and MK edited the article. All authors read and approved the final manuscript.
Funding
SS was supported by a Doctoral Research Award from the Canadian Institutes of Health Research. MK was supported by a grant from the Canadian Institutes of Health Research (EGM-141897) and is the Edwin S. H. Leong UBC Chair in Healthy Aging.
Acknowledgments
We thank Dr. Chaini Konwar and Alan Kerr for their valuable feedback on the manuscript.
Conflict of interest
The authors declare that the research was conducted in the absence of any commercial or financial relationships that could be construed as a potential conflict of interest.
Publisher’s note
All claims expressed in this article are solely those of the authors and do not necessarily represent those of their affiliated organizations, or those of the publisher, the editors and the reviewers. Any product that may be evaluated in this article, or claim that may be made by its manufacturer, is not guaranteed or endorsed by the publisher.
Supplementary material
The Supplementary Material for this article can be found online at: https://www.frontiersin.org/articles/10.3389/fgene.2022.971298/full#supplementary-material
References
Aguilera, O., Fernandez, A. F., Muñoz, A., and Fraga, M. F. (2010). Epigenetics and environment: A complex relationship. J. Appl. Physiology 109, 243–251. doi:10.1152/japplphysiol.00068.2010
Alfimova, M., Kondratyev, N., Golov, A., and Golimbet, V. (2020). Profiling haplotype specific CpG and CpH methylation within a schizophrenia GWAS locus on chromosome 14 in schizophrenia and healthy subjects. Sci. Rep. 10, 4704. doi:10.1038/s41598-020-61671-2
Angelopoulou, E., Paudel, Y. N., Papageorgiou, S. G., and Piperi, C. (2022). Environmental impact on the epigenetic mechanisms underlying Parkinson's disease pathogenesis: A narrative review. Brain Sci. 12, 175. doi:10.3390/brainsci12020175
Ascherio, A., and Schwarzschild, M. A. (2016). The epidemiology of Parkinson's disease: Risk factors and prevention. Lancet Neurology 15, 1257–1272. doi:10.1016/S1474-4422(16)30230-7
Banovich, N. E., Lan, X., McVicker, G., van de Geijn, B. V., Degner, J. F., Blischak, J. D., et al. (2014). Methylation QTLs are associated with coordinated changes in transcription factor binding, histone modifications, and gene expression levels. PLoS Genet. 10, e1004663. doi:10.1371/journal.pgen.1004663
Barker, D. J. (1990). The fetal and infant origins of adult disease. BMJ 301, 1111. doi:10.1136/bmj.301.6761.1111
Barres, R., Yan, J., Egan, B., Treebak, J. T., Rasmussen, M., Fritz, T., et al. (2012). Acute exercise remodels promoter methylation in human skeletal muscle. Cell Metab. 15, 405–411. doi:10.1016/j.cmet.2012.01.001
Betarbet, R., Sherer, T. B., MacKenzie, G., Garcia-Osuna, M., Panov, A. V., and Greenamyre, J. T. (2000). Chronic systemic pesticide exposure reproduces features of Parkinson's disease. Nat. Neurosci. 3, 1301–1306. doi:10.1038/81834
Bibikova, M., Le, J., Barnes, B., Saedinia-Melnyk, S., Zhou, L., Shen, R., et al. (2009). Genome-wide DNA methylation profiling using Infinium assay. Epigenomics 1, 177–200. doi:10.2217/epi.09.14
Blanch, M., Mosquera, J. L., Ansoleaga, B., Ferrer, I., and Barrachina, M. (2016). Altered mitochondrial DNA methylation pattern in alzheimer disease-related pathology and in Parkinson disease. Am. J. Pathology 186, 385–397. doi:10.1016/j.ajpath.2015.10.004
Blauwendraat, C., Nalls, M. A., and Singleton, A. B. (2020). The genetic architecture of Parkinson's disease. Lancet Neurology 19, 170–178. doi:10.1016/s1474-4422(19)30287-x
Booth, M. J., Ost, T. W. B., Beraldi, D., Bell, N. M., Branco, M. R., Reik, W., et al. (2013). Oxidative bisulfite sequencing of 5-methylcytosine and 5-hydroxymethylcytosine. Nat. Protoc. 8, 1841–1851. doi:10.1038/nprot.2013.115
Caldi Gomes, L., Galhoz, A., Jain, G., Roser, A.-E., Maass, F., Carboni, E., et al. (2022). Multiomic landscaping of human midbrains identifies disease relevant molecular targets and pathways in advanced stage Parkinson's disease. Clin. Transl. Med 12, e692. doi:10.1002/ctm2.692
Cavalli, G., and Heard, E. (2019). Advances in epigenetics link genetics to the environment and disease. Nature 571, 489–499. doi:10.1038/s41586-019-1411-0
Cazaly, E., Saad, J., Wang, W., Heckman, C., Ollikainen, M., and Tang, J. (2019). Making sense of the epigenome using data integration approaches. Front. Pharmacol. 10, 126. doi:10.3389/fphar.2019.00126
Chang, D., Nalls, M. A., Nalls, I. B., Hallgrímsdóttir, J., Hunkapiller, M., van der Brug, F., et al. (2017). A meta-analysis of genome-wide association studies identifies 17 new Parkinson's disease risk loci. Nat. Genet. 49, 1511–1516. doi:10.1038/ng.3955
Chatterjee, P., Roy, D., Bhattacharyya, M., and Bandyopadhyay, S. (2017). Biological networks in Parkinson's disease: An insight into the epigenetic mechanisms associated with this disease. BMC Genomics 18, 721. doi:10.1186/s12864-017-4098-3
Chen, H., and Ritz, B. (2018). The search for environmental causes of Parkinson's disease: Moving forward. Jpd 8, S9–S17. doi:10.3233/JPD-181493
Chen, H., Zhang, S. M., Schwarzschild, M. A., Hernan, M. A., and Ascherio, A. (2005). Physical activity and the risk of Parkinson disease. Neurology 64, 664–669. doi:10.1212/01.WNL.0000151960.28687.93
Cheung, W. A., Shao, X., Morin, A., Siroux, V., Kwan, T., Ge, B., et al. (2019). Correction to: Functional variation in allelic methylomes underscores a strong genetic contribution and reveals novel epigenetic alterations in the human epigenome. Genome Biol. 20, 89. doi:10.1186/s13059-019-1702-7
Chiba-Falek, O., and Nussbaum, R. L. (2001). Effect of allelic variation at the NACP-Rep1 repeat upstream of the alpha-synuclein gene (SNCA) on transcription in a cell culture luciferase reporter system. Hum. Mol. Genet. 10, 3101–3109. doi:10.1093/hmg/10.26.3101
Chuang, Y.-H., Paul, K. C., Bronstein, J. M., Bordelon, Y., Horvath, S., and Ritz, B. (2017). Parkinson's disease is associated with DNA methylation levels in human blood and saliva. Genome Med. 9, 1–12. doi:10.1186/s13073-017-0466-5
Coppede, F. (2012). Genetics and epigenetics of Parkinson's disease. Sci. World J. 2012, 1–12. doi:10.1100/2012/489830
Corti, O., Lesage, S., and Brice, A. (2011). What genetics tells us about the causes and mechanisms of Parkinson's disease. Physiol. Rev. 91, 1161–1218. doi:10.1152/physrev.00022.2010
Crotty, G. F., and Schwarzschild, M. A. (2020). Chasing protection in Parkinson's disease: Does exercise reduce risk and progression? Front. Aging Neurosci. 12, 186. doi:10.3389/fnagi.2020.00186
Curtis, C., Shah, S. P., Shah, S.-F., Chin, G., Turashvili, O. M., Rueda, M. J., et al. (2012). The genomic and transcriptomic architecture of 2,000 breast tumours reveals novel subgroups. Nature 486, 346–352. doi:10.1038/nature10983
Cutfield, W. S., Hofman, P. L., Mitchell, M., and Morison, I. M. (2007). Could epigenetics play a role in the developmental origins of health and disease? Pediatr. Res. 61, 68R–75R. doi:10.1203/pdr.0b013e318045764c
Czamara, D., Eraslan, G., Eraslan, C. M., Page, J., Lahti, M., Lahti-Pulkkinen, E., et al. (2019). Integrated analysis of environmental and genetic influences on cord blood DNA methylation in new-borns. Nat. Commun. 10, 2548. doi:10.1038/s41467-019-10461-0
Darst, R. P., Pardo, C. E., Ai, L., Brown, K. D., and Kladde, M. P. (2010). Bisulfite sequencing of DNA. Curr. Protoc. Mol. Biol. 91, 7. doi:10.1002/0471142727.mb0709s91
Davidi, D., Schechter, M., Elhadi, S. A., Matatov, A., Nathanson, L., and Sharon, R. (2020). α-Synuclein translocates to the nucleus to activate retinoic-acid-dependent gene transcription. iScience 23, 100910. doi:10.1016/j.isci.2020.100910
de Boni, L., Riedel, L., Schmitt, I., Kraus, T. F. J., Kaut, O., Piston, D., et al. (2015). DNA methylation levels of α-synuclein intron 1 in the aging brain. Neurobiol. Aging 36, e7–3334. doi:10.1016/j.neurobiolaging.2015.08.028
Deaton, A. M., and Bird, A. (2011). CpG islands and the regulation of transcription. Genes Dev. 25, 1010–1022. doi:10.1101/gad.2037511
Delgado-Morales, R., and Esteller, M. (2017). Opening up the DNA methylome of dementia. Mol. Psychiatry 22, 485–496. doi:10.1038/mp.2016.242
Deming, Y., Filipello, F., Cignarella, F., Cantoni, C., Hsu, S., Mikesell, R., et al. (2019). The MS4A gene cluster is a key modulator of soluble TREM2 and Alzheimer's disease risk. Sci. Transl. Med. 11, eaau2291. doi:10.1126/scitranslmed.aau2291
Desplats, P., Spencer, B., Coffee, E., Patel, P., Michael, S., Patrick, C., et al. (2011). α-Synuclein sequesters Dnmt1 from the nucleus. J. Biol. Chem. 286, 9031–9037. doi:10.1074/jbc.C110.212589
Dumitriu, A., Golji, J., Labadorf, A. T., Gao, B., Beach, T. G., Myers, R. H., et al. (2015). Integrative analyses of proteomics and RNA transcriptomics implicate mitochondrial processes, protein folding pathways and GWAS loci in Parkinson disease. BMC Med. Genomics 9, 5. doi:10.1186/s12920-016-0164-y
Dunn, A. R., O’Connell, K. M. S., and Kaczorowski, C. C. (2019). Gene-by-environment interactions in Alzheimer's disease and Parkinson's disease. Neurosci. Biobehav. Rev. 103, 73–80. doi:10.1016/j.neubiorev.2019.06.018
Edgar, R. D., Jones, M. J., Meaney, M. J., Turecki, G., and Kobor, M. S. (2017). BECon: A tool for interpreting DNA methylation findings from blood in the context of brain. Transl. Psychiatry 7, e1187. doi:10.1038/tp.2017.171
Elbaz, A., Clavel, J., Rathouz, P. J., Moisan, F., Galanaud, J.-P., Delemotte, B., et al. (2009). Professional exposure to pesticides and Parkinson disease. Ann. Neurol. 66, 494–504. doi:10.1002/ana.21717
Elbaz, A., Levecque, C., Clavel, J., Vidal, J. S., Richard, F., Amouyel, P., et al. (2004). CYP2D6 polymorphism, pesticide exposure, and Parkinson's disease. Ann. Neurol. 55, 430–434. doi:10.1002/ana.20051
Elsner, V. R., Lovatel, G. A., Moysés, F., Bertoldi, K., Spindler, C., Cechinel, L. R., et al. (2013). Exercise induces age-dependent changes on epigenetic parameters in rat hippocampus: A preliminary study. Exp. Gerontol. 48, 136–139. doi:10.1016/j.exger.2012.11.011
Emamzadeh, F. N. (2016). Alpha-synuclein structure, functions, and interactions. J. Res. Med. Sci. 21, 29. doi:10.4103/1735-1995.181989
Ernst, J., and Kellis, M. (2012). ChromHMM: Automating chromatin-state discovery and characterization. Nat. Methods 9, 215–216. doi:10.1038/nmeth.1906
Escott‐Price, V., Nalls, M. A., Morris, H. R., Lubbe, S., Brice, A., Gasser, T., et al. (2015). Polygenic risk of P arkinson disease is correlated with disease age at onset. Ann. Neurol. 77, 582–591. doi:10.1002/ana.24335
Espeso-Gil, S., Holik, A. Z., Bonnin, S., Jhanwar, S., Chandrasekaran, S., Pique-Regi, R., et al. (2021). Environmental enrichment induces epigenomic and genome organization changes relevant for cognition. Front. Mol. Neurosci. 14, 664912. doi:10.3389/fnmol.2021.664912
Farrer, M., Wavrant-De Vrieze, F., Crook, R., Boles, L., Perez-Tur, J., Hardy, J., et al. (1998). Low frequency of ?-synuclein mutations in familial Parkinson's disease. Ann. Neurol. 43, 394–397. doi:10.1002/ana.410430320
Feinberg, A. P. (2007). Phenotypic plasticity and the epigenetics of human disease. Nature 447, 433–440. doi:10.1038/nature05919
Fernandez-Santiago, R., Carballo-Carbajal, I., Castellano, G., Torrent, R., Richaud, Y., Sánchez-Danes, A., et al. (2015). Aberrant epigenome in iPSC derived dopaminergic neurons from Parkinson's disease patients. EMBO Mol. Med. 7, 1529–1546. doi:10.15252/emmm.201505439
Fernandez-Santiago, R., Merkel, A., Castellano, G., Heath, S., Raya, A., Tolosa, E., et al. (2019). Whole-genome DNA hyper-methylation in iPSC-derived dopaminergic neurons from Parkinson's disease patients. Clin. Epigenet 11, 108. doi:10.1186/s13148-019-0701-6
Fisher, B. E., Wu, A. D., Salem, G. J., Song, J., Lin, C. H. J., Yip, J., et al. (2008). The effect of exercise training in improving motor performance and corticomotor excitability in people with early Parkinson's disease. Archives Phys. Med. Rehabilitation 89, 1221–1229. doi:10.1016/j.apmr.2008.01.013
Freichel, C., Neumann, M., Ballard, T., Müller, V., Woolley, M., Ozmen, L., et al. (2007). Age-dependent cognitive decline and amygdala pathology in α-synuclein transgenic mice. Neurobiol. Aging 28, 1421–1435. doi:10.1016/j.neurobiolaging.2006.06.013
Furlong, M. A., Paul, K. C., Yan, Q., Chuang, Y.-H., Cockburn, M. G., Bronstein, J. M., et al. (2020). An epigenome-wide association study of ambient pyrethroid pesticide exposures in California's central valley. Int. J. Hyg. Environ. Health 229, 113569. doi:10.1016/j.ijheh.2020.113569
Fuso, A., Iyer, A. M., van Scheppingen, J., Maccarrone, M., Scholl, T., Hainfellner, J. A., et al. (2016). Promoter-specific hypomethylation correlates with IL-1β overexpression in tuberous sclerosis complex (TSC). J. Mol. Neurosci. 59, 464–470. doi:10.1007/s12031-016-0750-7
Fuso, A., and Lucarelli, M. (2019). CpG and non-CpG methylation in the diet-epigenetics-neurodegeneration connection. Curr. Nutr. Rep. 8, 74–82. doi:10.1007/s13668-019-0266-1
Gaunt, T. R., Shihab, H. A., Hemani, G., Min, J. L., Woodward, G., Lyttleton, O., et al. (2016). Systematic identification of genetic influences on methylation across the human life course. Genome Biol. 17, 61. doi:10.1186/s13059-016-0926-z
Gertz, J., Varley, K. E., Reddy, T. E., Bowling, K. M., Pauli, F., Parker, S. L., et al. (2011). Analysis of DNA methylation in a three-generation family reveals widespread genetic influence on epigenetic regulation. PLoS Genet. 7, e1002228. doi:10.1371/journal.pgen.1002228
Girirajan, S., Rosenfeld, J. A., Cooper, G. M., Antonacci, F., Siswara, P., Itsara, A., et al. (2010). A recurrent 16p12.1 microdeletion supports a two-hit model for severe developmental delay. Nat. Genet. 42, 203–209. doi:10.1038/ng.534
Go, R. C. P., Corley, M. J., Ross, G. W., Petrovitch, H., Masaki, K. H., Maunakea, A. K., et al. (2020). Genome-wide epigenetic analyses in Japanese immigrant plantation workers with Parkinson's disease and exposure to organochlorines reveal possible involvement of glial genes and pathways involved in neurotoxicity. BMC Neurosci. 21, 1–18. doi:10.1186/s12868-020-00582-4
Goldman, S. M., Marek, K., Ottman, R., Meng, C., Comyns, K., Chan, P., et al. (2019). Concordance for Parkinson's disease in twins: A 20‐year update. Ann. Neurol. 85, 600–605. doi:10.1002/ana.25441
Gomez-Pinilla, F., Zhuang, Y., Feng, J., Ying, Z., and Fan, G. (2011). Exercise impacts brain-derived neurotrophic factor plasticity by engaging mechanisms of epigenetic regulation. Eur. J. Neurosci. 33, 383–390. doi:10.1111/j.1460-9568.2010.07508.x
Gu, H., Smith, Z. D., Bock, C., Boyle, P., Gnirke, A., and Meissner, A. (2011). Preparation of reduced representation bisulfite sequencing libraries for genome-scale DNA methylation profiling. Nat. Protoc. 6, 468–481. doi:10.1038/nprot.2010.190
Guintivano, J., Aryee, M. J., and Kaminsky, Z. A. (2013). A cell epigenotype specific model for the correction of brain cellular heterogeneity bias and its application to age, brain region and major depression. Epigenetics 8, 290–302. doi:10.4161/epi.23924
Guo, J. U., Su, Y., Shin, J. H., Shin, J., Li, H., Xie, B., et al. (2014). Distribution, recognition and regulation of non-CpG methylation in the adult mammalian brain. Nat. Neurosci. 17, 215–222. doi:10.1038/nn.3607
Hack, L. M., Dick, A. L. W., and Provencal, N. (2016). Epigenetic mechanisms involved in the effects of stress exposure: Focus on 5-hydroxymethylcytosine: Table 1:. Environ. Epigenet. 2, dvw016. doi:10.1093/eep/dvw016
He, B., Zhang, C., Zhang, X., Fan, Y., Zeng, H., Liu, J., et al. (2021). Tissue-specific 5-hydroxymethylcytosine landscape of the human genome. Nat. Commun. 12, 4249. doi:10.1038/s41467-021-24425-w
Henderson, A. R., Wang, Q., Meechoovet, B., Siniard, A. L., Naymik, M., De Both, M., et al. (2021). DNA methylation and expression profiles of whole blood in Parkinson's disease. Front. Genet. 12, 640266. doi:10.3389/fgene.2021.640266
Henderson-Smith, A., Fisch, K. M., Hua, J., Liu, G., Ricciardelli, E., Jepsen, K., et al. (2019). DNA methylation changes associated with Parkinson's disease progression: Outcomes from the first longitudinal genome-wide methylation analysis in blood. Epigenetics 14, 365–382. doi:10.1080/15592294.2019.1588682
Ho, S.-M., Johnson, A., Tarapore, P., Janakiram, V., Zhang, X., and Leung, Y.-K. (2012). Environmental epigenetics and its implication on disease risk and health outcomes. ILAR J. 53, 289–305. doi:10.1093/ilar.53.3-4.289
Horsthemke, B. (2014). In brief: Genomic imprinting and imprinting diseases. J. Pathol. 232, 485–487. doi:10.1002/path.4326
Horvath, S., and Raj, K. (2018). DNA methylation-based biomarkers and the epigenetic clock theory of ageing. Nat. Rev. Genet. 19, 371–384. doi:10.1038/s41576-018-0004-3
Husquin, L. T., Rotival, M., Fagny, M., Quach, H., Zidane, N., McEwen, L. M., et al. (2018). Exploring the genetic basis of human population differences in DNA methylation and their causal impact on immune gene regulation. Genome Biol. 19, 222. doi:10.1186/s13059-018-1601-3
Ihle, J., Artaud, F., Bekadar, S., Mangone, G., Sambin, S., Mariani, L., et al. (2020). Parkinson's disease polygenic risk score is not associated with impulse control disorders: A longitudinal study. Park. Relat. Disord. 75, 30–33. doi:10.1016/j.parkreldis.2020.03.017
International Parkinson’s Disease Genomics Consortium and Wellcome Trust Case Control Consortium (2011). A two-stage meta-analysis identifies several new loci for Parkinson's disease. PLoS Genet. 7, e1002142. doi:10.1371/journal.pgen.1002142
Inzelberg, R., Schecthman, E., Paleacu, D., Zach, L., Bonwitt, R., Carasso, R. l., et al. (2004). Onset and progression of disease in familial and sporadic Parkinson's disease. Am. J. Med. Genet. 124A, 255–258. doi:10.1002/ajmg.a.20405
Irier, H., Street, R. C., Dave, R., Lin, L., Cai, C., Davis, T. H., et al. (2014). Environmental enrichment modulates 5-hydroxymethylcytosine dynamics in hippocampus. Genomics 104, 376–382. doi:10.1016/j.ygeno.2014.08.019
Irizarry, R. A., Ladd-Acosta, C., Wen, B., Wu, Z., Montano, C., Onyango, P., et al. (2009). The human colon cancer methylome shows similar hypo- and hypermethylation at conserved tissue-specific CpG island shores. Nat. Genet. 41, 178–186. doi:10.1038/ng.298
Islam, S. A., Goodman, S. J., MacIsaac, J. L., Obradovic, J., Barr, R. G., Boyce, W. T., et al. (2019). Integration of DNA methylation patterns and genetic variation in human pediatric tissues help inform EWAS design and interpretation. Epigenetics Chromatin 12, 1. doi:10.1186/s13072-018-0245-6
Jessop, P., and Toledo-Rodriguez, M. (2018). Hippocampal TET1 and TET2 expression and DNA hydroxymethylation are affected by physical exercise in aged mice. Front. Cell Dev. Biol. 6, 45. doi:10.3389/fcell.2018.00045
Jin, H., Kanthasamy, A., Ghosh, A., Yang, Y., Anantharam, V., and Kanthasamy, A. G. (2011). -synuclein negatively regulates protein kinase C expression to suppress apoptosis in dopaminergic neurons by reducing p300 histone acetyltransferase activity. J. Neurosci. 31, 2035–2051. doi:10.1523/JNEUROSCI.5634-10.2011
Jo, E., Fuller, N., Rand, R. P., St George-Hyslop, P., and Fraser, P. E. (2002). Defective membrane interactions of familial Parkinson's disease mutant A30P α-synuclein 1 1Edited by I. B. Holland. J. Mol. Biol. 315, 799–807. doi:10.1006/jmbi.2001.5269
Jones, M. J., Goodman, S. J., and Kobor, M. S. (2015). DNAmethylation and healthy human aging. Aging Cell 14, 924–932. doi:10.1111/acel.12349
Jones, P. A. (2012). Functions of DNA methylation: Islands, start sites, gene bodies and beyond. Nat. Rev. Genet. 13, 484–492. doi:10.1038/nrg3230
Jowaed, A., Schmitt, I., Kaut, O., and Wullner, U. (2010). Methylation regulates alpha-synuclein expression and is decreased in Parkinson's disease patients' brains. J. Neurosci. 30, 6355–6359. doi:10.1523/JNEUROSCI.6119-09.2010
Kaas, G. A., Zhong, C., Eason, D. E., Ross, D. L., Vachhani, R. V., Ming, G.-L., et al. (2013). TET1 controls CNS 5-methylcytosine hydroxylation, active DNA demethylation, gene transcription, and memory formation. Neuron 79, 1086–1093. doi:10.1016/j.neuron.2013.08.032
Kantor, B., Tagliafierro, L., Gu, J., Zamora, M. E., Ilich, E., Grenier, C., et al. (2018). Downregulation of SNCA expression by targeted editing of DNA methylation: A potential strategy for precision therapy in PD. Mol. Ther. 26, 2638–2649. doi:10.1016/j.ymthe.2018.08.019
Kaushal, A., Zhang, H., Karmaus, W. J. J., Ray, M., Torres, M. A., Smith, A. K., et al. (2017). Comparison of different cell type correction methods for genome-scale epigenetics studies. BMC Bioinforma. 18, 216. doi:10.1186/s12859-017-1611-2
Kaut, O., Kuchelmeister, K., Moehl, C., and Wullner, U. (2019). 5-methylcytosine and 5-hydroxymethylcytosine in brains of patients with multiple system atrophy and patients with Parkinson's disease. J. Chem. Neuroanat. 96, 41–48. doi:10.1016/j.jchemneu.2018.12.005
Kaut, O., Schmitt, I., Stahl, F., Fröhlich, H., Hoffmann, P., Gonzalez, F. J., et al. (2022). Epigenome-wide analysis of DNA methylation in Parkinson's disease cortex. Life 12, 502. doi:10.3390/life12040502
Kaut, O., Schmitt, I., and Wüllner, U. (2012). Genome-scale methylation analysis of Parkinson's disease patients' brains reveals DNA hypomethylation and increased mRNA expression of cytochrome P450 2E1. Neurogenetics 13, 87–91. doi:10.1007/s10048-011-0308-3
Kernaleguen, M., Daviaud, C., Shen, Y., Bonnet, E., Renault, V., Deleuze, J.-F., et al. (2018). “Whole-genome bisulfite sequencing for the analysis of genome-wide DNA methylation and hydroxymethylation patterns at single-nucleotide resolution,” in Epigenome editing: Methods and protocols methods in molecular biology. Editors A. Jeltsch, and M. G. Rots (New York, NY: Springer), 311–349. doi:10.1007/978-1-4939-7774-1_18
Kia, D. A., Zhang, D., Guelfi, S., Manzoni, C., Hubbard, L., Reynolds, R. H., et al. (2021). Identification of candidate Parkinson disease genes by integrating genome-wide association study, expression, and epigenetic data sets. JAMA Neurol. 78, 464–472. doi:10.1001/jamaneurol.2020.5257
Kim, S., and Xing, E. P. (2012). Tree-guided group lasso for multi-response regression with structured sparsity, with an application to eQTL mapping. Ann. Appl. Stat. 6, 1095–1117. doi:10.1214/12-aoas549
Kinde, B., Gabel, H. W., Gilbert, C. S., Griffith, E. C., and Greenberg, M. E. (2015). Reading the unique DNA methylation landscape of the brain: Non-CpG methylation, hydroxymethylation, and MeCP2. Proc. Natl. Acad. Sci. U.S.A. 112, 6800–6806. doi:10.1073/pnas.1411269112
Klein, C., and Westenberger, A. (2012). Genetics of Parkinson's disease. Cold Spring Harb. Perspect. Med. 2, a008888. doi:10.1101/cshperspect.a008888
Kochmanski, J., and Bernstein, A. I. (2020). The impact of environmental factors on 5-hydroxymethylcytosine in the brain. Curr. Envir Health Rpt 7, 109–120. doi:10.1007/s40572-020-00268-3
Kontopoulos, E., Parvin, J. D., and Feany, M. B. (2006). α-synuclein acts in the nucleus to inhibit histone acetylation and promote neurotoxicity. Hum. Mol. Genet. 15, 3012–3023. doi:10.1093/hmg/ddl243
Krueger, F., and Andrews, S. R. (2012). Quality control, trimming and alignment of Bisulfite-Seq data. AvaliableAt: https://www.epigenesys.eu/images/stories/protocols/pdf/20120720103700_p57.pdf (Accessed June 14, 2022).
Kumar, S., Chinnusamy, V., and Mohapatra, T. (2018). Epigenetics of modified DNA bases: 5-methylcytosine and beyond. Front. Genet. 9, 640. doi:10.3389/fgene.2018.00640
Laird, P. W. (2010). Principles and challenges of genome-wide DNA methylation analysis. Nat. Rev. Genet. 11, 191–203. doi:10.1038/nrg2732
Lang, A. E., and Lozano, A. M. (1998). Parkinson's disease. N. Engl. J. Med. 339, 1044–1053. doi:10.1056/NEJM199810083391506
Langston, J. W., Ballard, P., Tetrud, J. W., and Irwin, I. (1983). Chronic parkinsonism in humans due to a product of meperidine-analog synthesis. Science 219, 979–980. doi:10.1126/science.6823561
Lappalainen, T., and Greally, J. M. (2017). Associating cellular epigenetic models with human phenotypes. Nat. Rev. Genet. 18, 441–451. doi:10.1038/nrg.2017.32
Lee, J.-H., Saito, Y., Park, S.-J., and Nakai, K. (2020). Existence and possible roles of independent non-CpG methylation in the mammalian brain. DNA Res. 27, dsaa020. doi:10.1093/dnares/dsaa020
Lee, K. W. K., and Pausova, Z. (2013). Cigarette smoking and DNA methylation. Front. Genet. 4, 132. doi:10.3389/fgene.2013.00132
Lesage, S., and Brice, A. (2009). Parkinson's disease: From monogenic forms to genetic susceptibility factors. Hum. Mol. Genet. 18, R48–R59. doi:10.1093/hmg/ddp012
Levine, M. E., Lu, A. T., Quach, A., Chen, B. H., Assimes, T. L., Bandinelli, S., et al. (2018). An epigenetic biomarker of aging for lifespan and healthspan. Aging 10, 573–591. doi:10.18632/aging.101414
Li, P., Ensink, E., Lang, S., Marshall, L., Schilthuis, M., Lamp, J., et al. (2020). Hemispheric asymmetry in the human brain and in Parkinson's disease is linked to divergent epigenetic patterns in neurons. Genome Biol. 21, 1–23. doi:10.1186/s13059-020-01960-1
Li, X., Wei, W., Zhao, Q.-Y., Widagdo, J., Baker-Andresen, D., Flavell, C. R., et al. (2014). Neocortical Tet3-mediated accumulation of 5-hydroxymethylcytosine promotes rapid behavioral adaptation. Proc. Natl. Acad. Sci. U.S.A. 111, 7120–7125. doi:10.1073/pnas.1318906111
Li, Y., Ma, L., Wu, D., and Chen, G. (2021). Advances in bulk and single-cell multi-omics approaches for systems biology and precision medicine. Brief. Bioinform. 22, bbab024. doi:10.1093/bib/bbab024
Lim, U., and Song, M.-A. (2012). “Dietary and lifestyle factors of DNA methylation,” in Cancer epigenetics: Methods and protocols methods in molecular biology. Editors R. G. Dumitrescu, and M. Verma (Totowa, NJ: Humana Press), 359–376. doi:10.1007/978-1-61779-612-8_23
Lin, M. K., and Farrer, M. J. (2014). Genetics and genomics of Parkinson's disease. Genome Med. 6, 48. doi:10.1186/gm566
Lindholm, M. E., Marabita, F., Gomez-Cabrero, D., Rundqvist, H., Ekström, T. J., Tegnér, J., et al. (2014). An integrative analysis reveals coordinated reprogramming of the epigenome and the transcriptome in human skeletal muscle after training. Epigenetics 9, 1557–1569. doi:10.4161/15592294.2014.982445
Lister, R., Mukamel, E. A., Nery, J. R., Urich, M., Puddifoot, C. A., Johnson, N. D., et al. (2013). Global epigenomic reconfiguration during mammalian brain development. Science 341, 1237905. doi:10.1126/science.1237905
Liu, J., Lin, D., Chen, J., Perrone-Bizzozero, N., Calhoun, V., and Bustillo, J. (2019). Characterization of cross-tissue mQTL effects and their relevance in psychiatric disorders. Eur. Neuropsychopharmacol. 29, S796–S797. doi:10.1016/j.euroneuro.2017.08.030
Liu, J., and Siegmund, K. D. (2016). An evaluation of processing methods for HumanMethylation450 BeadChip data. BMC Genomics 17, 469. doi:10.1186/s12864-016-2819-7
Liu, Y., Koyutürk, M., Maxwell, S., Zhao, Z., and Chance, M. R. (2012). Integrative analysis of common neurodegenerative diseases using gene association, interaction networks and mRNA expression data. AMIA Jt. Summits Transl. Sci. Proc. 2012, 62–71.
Lock, E. F., and Dunson, D. B. (2013). Bayesian consensus clustering. Bioinformatics 29, 2610–2616. doi:10.1093/bioinformatics/btt425
Logroscino, G. (2005). The role of early life environmental risk factors in Parkinson disease: What is the evidence? Environ. Health Perspect. 113, 1234–1238. doi:10.1289/ehp.7573
Lokk, K., Modhukur, V., Rajashekar, B., Märtens, K., Mägi, R., Kolde, R., et al. (2016). Erratum to: DNA methylome profiling of human tissues identifies global and tissue-specific methylation patterns. Genome Biol. 17, 224. doi:10.1186/s13059-016-1091-0
Lunnon, K., Hannon, E., G.Smith, R. G., Dempster, E., Wong, C., Burrage, J., et al. (2016). Erratum to: Variation in 5-hydroxymethylcytosine across human cortex and cerebellum. Genome BiolGenome Biol. 1717, 13127. doi:10.1186/s13059-016-0958-410.1186/s13059-016-0871-x
Maraganore, D. M., de Andrade, M., Elbaz, A., Farrer, M. J., Ioannidis, J. P., Krüger, R., et al. (2006). Collaborative analysis of α-synuclein gene promoter variability and Parkinson disease. JAMA 296, 661–670. doi:10.1001/jama.296.6.661
Marey-Semper, I., Gelman, M., and Levi-Strauss, M. (1995). A selective toxicity toward cultured mesencephalic dopaminergic neurons is induced by the synergistic effects of energetic metabolism impairment and NMDA receptor activation. J. Neurosci. 15, 5912–5918. doi:10.1523/jneurosci.15-09-05912.1995
Marshall, L. L., Killinger, B. A., Ensink, E., Li, P., Li, K. X., Cui, W., et al. (2020). Epigenomic analysis of Parkinson's disease neurons identifies Tet2 loss as neuroprotective. Nat. Neurosci. 23, 1203–1214. doi:10.1038/s41593-020-0690-y
Martins, M., Rosa, A., Guedes, L. C., Fonseca, B. V., Gotovac, K., Violante, S., et al. (2011). Convergence of miRNA expression profiling, α-synuclein interacton and GWAS in Parkinson's disease. PLoS ONE 6, e25443. doi:10.1371/journal.pone.0025443
Marttinen, P., Pirinen, M., Sarin, A.-P., Gillberg, J., Kettunen, J., Surakka, I., et al. (2014). Assessing multivariate gene-metabolome associations with rare variants using Bayesian reduced rank regression. Bioinformatics 30, 2026–2034. doi:10.1093/bioinformatics/btu140
Maserejian, N., Vinikoor-Imler, L., and Dilley, A. (2020). Estimation of the 2020 global population of Parkinson’s disease (PD). Available at: https://www.mdsabstracts.org/abstract/estimation-of-the-2020-global-population-of-parkinsons-disease-pd/(Accessed June 14, 2022).
Masliah, E., Dumaop, W., Galasko, D., and Desplats, P. (2013). Distinctive patterns of DNA methylation associated with Parkinson disease. Epigenetics 8, 1030–1038. doi:10.4161/epi.25865
Matosin, N., Cruceanu, C., and Binder, E. B. (2017). Preclinical and clinical evidence of DNA methylation changes in response to trauma and chronic stress. Chronic Stress 1, 247054701771076. doi:10.1177/2470547017710764
Maver, A., and Peterlin, B. (2011). Positional integratomic approach in identification of genomic candidate regions for Parkinson's disease. Bioinformatics 27, 1971–1978. doi:10.1093/bioinformatics/btr313
McCormack, A. L., Thiruchelvam, M., Manning-Bog, A. B., Thiffault, C., Langston, J. W., Cory-Slechta, D. A., et al. (2002). Environmental risk factors and Parkinson's disease: Selective degeneration of nigral dopaminergic neurons caused by the herbicide paraquat. Neurobiol. Dis. 10, 119–127. doi:10.1006/nbdi.2002.0507
Moore, K., McKnight, A. J., Craig, D., and O’Neill, F. (2014). Epigenome-wide association study for Parkinson's disease. Neuromol. Med. 16, 845–855. doi:10.1007/s12017-014-8332-8
Multhaup, M. L., Seldin, M., Jaffe, A. E., Lei, X., Kirchner, H., Mondal, P., et al. (2015). Mouse-human experimental epigenetic analysis unmasks dietary targets and genetic liability for diabetic phenotypes. Cell Metab. 21, 138–149. doi:10.1016/j.cmet.2014.12.014
Muñoz, E., Oliva, R., Obach, V., Marti, M. J., Pastor, P., Ballesta, F., et al. (1997). Identification of Spanish familial Parkinson’s disease and screening for the Ala53Thr mutation of the α-synuclein gene in early onset patients. Neurosci. Lett. 235, 57–60. doi:10.1016/S0304-3940(97)00710-6
Nabais, M. F., Laws, S. M., Laws, T., Lin, C. L., Vallerga, N. J., Armstrong, I. P., et al. (2021). Meta-analysis of genome-wide DNA methylation identifies shared associations across neurodegenerative disorders. Genome Biol. 22, 1–30. doi:10.1186/s13059-021-02275-5
Nalls, M. A., Blauwendraat, C., Vallerga, C. L., Heilbron, K., Bandres-Ciga, S., Chang, D., et al. (2019). Identification of novel risk loci, causal insights, and heritable risk for Parkinson's disease: A meta-analysis of genome-wide association studies. Lancet Neurol. 18, 1091–1102. doi:10.1016/S1474-4422(19)30320-5
Nalls, M. A., Pankratz, N., Pankratz, C. M., Lill, C. B., Do, D. G., Hernandez, M., et al. (2014). Large-scale meta-analysis of genome-wide association data identifies six new risk loci for Parkinson's disease. Nat. Genet. 46, 989–993. doi:10.1038/ng.3043
Ngwa, J. S., Nwulia, E., Ntekim, O., Bedada, F. B., Kwabi-Addo, B., Nadarajah, S., et al. (2022). Aerobic exercise training-induced changes on DNA methylation in mild cognitively impaired elderly african Americans: Gene, exercise, and memory study - GEMS-I. Front. Mol. Neurosci. 14, 752403. doi:10.3389/fnmol.2021.752403
Nicolia, V., Cavallaro, R. A., Lopez-Gonzalez, I., Maccarrone, M., Scarpa, S., Ferrer, I., et al. (2017). DNA methylation profiles of selected pro-inflammatory cytokines in Alzheimer disease. J. Neuropathol. Exp. Neurol. 76, nlw099–31. doi:10.1093/jnen/nlw099
Oliveira, L. M. A., Falomir-Lockhart, L. J., Botelho, M. G., Lin, K.-H., Wales, P., Koch, J. C., et al. (2015). Elevated α-synuclein caused by SNCA gene triplication impairs neuronal differentiation and maturation in Parkinson's patient-derived induced pluripotent stem cells. Cell Death Dis. 6, e1994. doi:10.1038/cddis.2015.318
Paiva, I., Jain, G., Lazaro, D. F., Jercic, K. G., Hentrich, T., Kerimoglu, C., et al. (2018). Alpha-synuclein deregulates the expression of COL4A2 and impairs ER-Golgi function. Neurobiol. Dis. 119, 121–135. doi:10.1016/j.nbd.2018.08.001
Paiva, I., Pinho, R., Pavlou, M. A., Hennion, M., Wales, P., Schütz, A.-L., et al. (2017). Sodium butyrate rescues dopaminergic cells from alpha-synuclein-induced transcriptional deregulation and DNA damage. Hum. Mol. Genet. 26, 2231–2246. doi:10.1093/hmg/ddx114
Pang, S. Y.-Y., Ho, P. W.-L., Liu, H.-F., Leung, C.-T., Li, L., Chang, E. E. S., et al. (2019). The interplay of aging, genetics and environmental factors in the pathogenesis of Parkinson's disease. Transl. Neurodegener. 8, 1–11. doi:10.1186/s40035-019-0165-9
Park, G., Tan, J., Garcia, G., Kang, Y., Salvesen, G., and Zhang, Z. (2016). Regulation of histone acetylation by autophagy in Parkinson disease. J. Biol. Chem. 291, 3531–3540. doi:10.1074/jbc.M115.675488
Peng, J., Mao, X. O., Stevenson, F. F., Hsu, M., and Andersen, J. K. (2004). The herbicide paraquat induces dopaminergic nigral apoptosis through sustained activation of the JNK pathway. J. Biol. Chem. 279, 32626–32632. doi:10.1074/jbc.M404596200
Peterlin, B., and Maver, A. (2012). Integrative 'omic' approach towards understanding the nature of human diseases. Balk. J. Med. Genet. 15, 45–50. doi:10.2478/v10034-012-0018-7
Pidsley, R., Y Wong, C. C., Volta, M., Lunnon, K., Mill, J., and Schalkwyk, L. C. (2013). A data-driven approach to preprocessing Illumina 450K methylation array data. BMC Genomics 14, 293. doi:10.1186/1471-2164-14-293
Pidsley, R., Zotenko, E., Peters, T. J., Lawrence, M. G., Risbridger, G. P., Molloy, P., et al. (2016). Critical evaluation of the Illumina MethylationEPIC BeadChip microarray for whole-genome DNA methylation profiling. Genome Biol. 17, 208. doi:10.1186/s13059-016-1066-1
Pinho, R., Paiva, I., Jercic, K. G., Fonseca-Ornelas, L., Gerhardt, E., Fahlbusch, C., et al. (2019). Nuclear localization and phosphorylation modulate pathological effects of alpha-synuclein. Hum. Mol. Genet. 28, 31–50. doi:10.1093/hmg/ddy326
Polymeropoulos, M. H., Higgins, J. J., Golbe, L. I., Johnson, W. G., Ide, S. E., Di Iorio, G., et al. (1996). Mapping of a gene for Parkinson's disease to chromosome 4q21-q23. Science 274, 1197–1199. doi:10.1126/science.274.5290.1197
Polymeropoulos, M. H., Lavedan, C., Leroy, E., Ide, S. E., Dehejia, A., Dutra, A., et al. (1997). Mutation in the α-synuclein gene identified in families with Parkinson's disease. Science 276, 2045–2047. doi:10.1126/science.276.5321.2045
Pouchieu, C., Piel, C., Carles, C., Gruber, A., Helmer, C., Tual, S., et al. (2018). Pesticide use in agriculture and Parkinson's disease in the AGRICAN cohort study. Int. J. Epidemiol. 47, 299–310. doi:10.1093/ije/dyx225
Przedborski, S., Jackson-Lewis, V., Naini, A. B., Jakowec, M., Petzinger, G., Miller, R., et al. (2001). The parkinsonian toxin 1-methyl-4-phenyl-1,2,3,6-tetrahydropyridine (MPTP): A technical review of its utility and safety. J. Neurochem. 76, 1265–1274. doi:10.1046/j.1471-4159.2001.00183.x
Ronn, T., Volkov, P., Davegardh, C., Dayeh, T., Hall, E., Olsson, A. H., et al. (2013). A six months exercise intervention influences the genome-wide DNA methylation pattern in human adipose tissue. PLoS Genet. 9, e1003572. doi:10.1371/journal.pgen.1003572
Rajput, A. H., Uitti, R. J., Stern, W., Laverty, W., O'Donnell, K., O'Donnell, D., et al. (1987). Geography, drinking water chemistry, pesticides and herbicides and the etiology of Parkinson's disease. Can. J. Neurol. Sci. 14, 414–418. doi:10.1017/S0317167100037823
Rawlik, K., Rowlatt, A., and Tenesa, A. (2016). Imputation of DNA methylation levels in the brain implicates a risk factor for Parkinson's disease. Genetics 204, 771–781. doi:10.1534/genetics.115.185967
Rawson, K. S., McNeely, M. E., Duncan, R. P., Pickett, K. A., Perlmutter, J. S., and Earhart, G. M. (2019). Exercise and Parkinson disease: Comparing tango, treadmill, and stretching. J. Neurol. Phys. Ther. 43, 26–32. doi:10.1097/NPT.0000000000000245
Redensek, S., Dolzan, V., and Kunej, T. (2018). From genomics to omics landscapes of Parkinson's disease: Revealing the molecular mechanisms. OMICS A J. Integr. Biol. 22, 1–16. doi:10.1089/omi.2017.0181
Reimand, J., Isserlin, R., Voisin, V., Kucera, M., Tannus-Lopes, C., Rostamianfar, A., et al. (2019). Pathway enrichment analysis and visualization of omics data using g:Profiler, GSEA, Cytoscape and EnrichmentMap. Nat. Protoc. 14, 482–517. doi:10.1038/s41596-018-0103-9
Richardson, S., Tseng, G. C., and Sun, W. (2016). Statistical methods in integrative genomics. Annu. Rev. Stat. Appl. 3, 181–209. doi:10.1146/annurev-statistics-041715-033506
Ritz, B. R., Manthripragada, A. D., Costello, S., Lincoln, S. J., Farrer, M. J., Cockburn, M., et al. (2009). Dopamine transporter genetic variants and pesticides in Parkinson's disease. Environ. Health Perspect. 117, 964–969. doi:10.1289/ehp.0800277
Rudyk, C., Dwyer, Z., Dwyer, S., and Hayley, C. (2019). Leucine-rich repeat kinase-2 (LRRK2) modulates paraquat-induced inflammatory sickness and stress phenotype. J. Neuroinflammation 16, 120. doi:10.1186/s12974-019-1483-7
Russo, V. E. A., Martienssen, R. A., and Riggs, A. D. (1996). Epigenetic mechanisms of gene regulation. Plainview, N.Y: Cold Spring Harbor Laboratory Press.
Swiatowy, W. J., Drzewiecka, H., Kliber, M., Sąsiadek, M., Karpinski, P., Pławski, A., et al. (2021). Physical activity and DNA methylation in humans. Ijms 22, 12989. doi:10.3390/ijms222312989
Sasco, A. J., Paffenbarger, R. S., Gendre, I., and Wing, A. L. (1992). The role of physical exercise in the occurrence of Parkinson's disease. Archives Neurology 49, 360–365. doi:10.1001/archneur.1992.00530280040020
Schaffner, S. L., Wassouf, Z., Lazaro, D. F., Xylaki, M., Gladish, N., Lin, D. T. S., et al. (2022). Alpha-synuclein overexpression induces epigenomic dysregulation of glutamate signaling and locomotor pathways. Hum. Mol. Genet., ddac104. doi:10.1093/hmg/ddac104
Schmitt, I., Kaut, O., Khazneh, H., deBoni, L., Ahmad, A., Berg, D., et al. (2015). L-dopa increases α -synuclein DNA methylation in Parkinson's disease patients in vivo and in vitro. Mov. Disord. 30, 1794–1801. doi:10.1002/mds.26319
Scott, W. K., Stajich, J. M., Yamaoka, L. H., Speer, M. C., Vance, J. M., Roses, A. D., et al. (1997). Genetic complexity and Parkinson's disease. Science 277, 387–390. doi:10.1126/science.277.5324.387
Scott, W. K., Yamaoka, L. H., Stajich, J. M., Scott, B. L., Vance, J. M., Roses, A. D., et al. (1999). The α-synuclein gene is not a major risk factor in familial Parkinson disease. Neurogenetics 2, 191–192. doi:10.1007/s100480050083
Shi, X., Radhakrishnan, S., Wen, J., Chen, J. Y., Chen, J., Lam, B. A., et al. (2020). Association of CNVs with methylation variation. NPJ Genom. Med. 5, 41. doi:10.1038/s41525-020-00145-w
Singh, A., Shannon, C. P., Gautier, B., Rohart, F., Vacher, M., Tebbutt, S. J., et al. (2019). Diablo: An integrative approach for identifying key molecular drivers from multi-omics assays. Bioinformatics 35, 3055–3062. doi:10.1093/bioinformatics/bty1054
Skvortsova, K., Zotenko, E., Luu, P.-L., Gould, C. M., Nair, S. S., Clark, S. J., et al. (2017). Comprehensive evaluation of genome-wide 5-hydroxymethylcytosine profiling approaches in human DNA. Epigenetics Chromatin 10, 16. doi:10.1186/s13072-017-0123-7
Slieker, R. C., van Iterson, M., van Iterson, R., Luijk, M., Beekman, D. V., Zhernakova, M. H., et al. (2016). Age-related accrual of methylomic variability is linked to fundamental ageing mechanisms. Genome Biol. 17, 191. doi:10.1186/s13059-016-1053-6
Smith, A. K., Kilaru, V., Kocak, M., Almli, L. M., Mercer, K. B., Ressler, K. J., et al. (2014). Methylation quantitative trait loci (meQTLs) are consistently detected across ancestry, developmental stage, and tissue type. BMC Genomics 15, 145. doi:10.1186/1471-2164-15-145
Spiers, H., Hannon, E., Schalkwyk, L. C., Bray, N. J., and Mill, J. (2017). 5-hydroxymethylcytosine is highly dynamic across human fetal brain development. BMC Genomics 18, 738. doi:10.1186/s12864-017-4091-x
Stingo, F. C., Chen, Y. A., Tadesse, M. G., and Vannucci, M. (2011). Incorporating biological information into linear models: A bayesian approach to the selection of pathways and genes. Ann. Appl. Stat. 5, 463. doi:10.1214/11-AOAS46310.1214/11-AOAS463
Stricker, S. H., and Gotz, M. (2018). DNA-Methylation: Master or slave of neural fate decisions? Front. Neurosci. 12, 5. doi:10.3389/fnins.2018.00005
Sudlow, C., Gallacher, J., Allen, N., Beral, V., Burton, P., Danesh, J., et al. (2015). UK Biobank: An open access resource for identifying the causes of a wide range of complex diseases of middle and old age. PLoS Med. 12, e1001779. doi:10.1371/journal.pmed.1001779
Sujkowski, A., Hong, L., Wessells, R. J., and Todi, S. V. (2022). The protective role of exercise against age-related neurodegeneration. Ageing Res. Rev. 74, 101543. doi:10.1016/j.arr.2021.101543
Szulwach, K. E., Li, X., Li, Y., Song, C.-X., Wu, H., Dai, Q., et al. (2011). 5-hmC-mediated epigenetic dynamics during postnatal neurodevelopment and aging. Nat. Neurosci. 14, 1607–1616. doi:10.1038/nn.2959
Tanner, C. M., Kamel, F., Ross, G. W., Hoppin, J. A., Goldman, S. M., Korell, M., et al. (2011). Rotenone, paraquat, and Parkinson's disease. Environ. Health Perspect. 119, 866–872. doi:10.1289/ehp.1002839
Teh, A. L., Pan, H., Chen, L., Ong, M.-L., Dogra, S., Wong, J., et al. (2014). The effect of genotype and in utero environment on interindividual variation in neonate DNA methylomes. Genome Res. 24, 1064–1074. doi:10.1101/gr.171439.113
Tiili, E. M., Antikainen, M. S. H., Mitiushkina, N. V., Sukhovskaya, O. A., Imyanitov, E. N., and Hirvonen, A. P. (2015). Effect of genotype and methylation of CYP2D6 on smoking behaviour. Pharmacogenet. Genomics 25, 531–540. doi:10.1097/FPC.0000000000000166
Vallerga, C. L., Zhang, F., Fowdar, J., McRae, A. F., Qi, T., Nabais, M. F., et al. (2020). Analysis of DNA methylation associates the cystine-glutamate antiporter SLC7A11 with risk of Parkinson's disease. Nat. Commun. 11, 1238. doi:10.1038/s41467-020-15065-7
van der Plaat, D. A., de Jong, K., de Vries, M., van Diemen, C. C., Nedeljkovic, I., Amin, N., et al. (2018). Occupational exposure to pesticides is associated with differential DNA methylation. Occup. Environ. Med. 75, 427–435. doi:10.1136/oemed-2017-104787
Villar-Piqué, A., Lopes da Fonseca, T., and Outeiro, T. F. (2016). Structure, function and toxicity of alpha-synuclein: The Bermuda triangle in synucleinopathies. J. Neurochem. 139, 240–255. doi:10.1111/jnc.13249
Villicana, S., and Bell, J. T. (2021). Genetic impacts on DNA methylation: Research findings and future perspectives. Genome Biol. 22, 127. doi:10.1186/s13059-021-02347-6
Voutsinas, G. E., Stavrou, E. F., Karousos, G., Dasoula, A., Papachatzopoulou, A., Syrrou, M., et al. (2010). Allelic imbalance of expression and epigenetic regulation within the alpha-synuclein wild-type and p.Ala53Thr alleles in Parkinson disease. Hum. Mutat. 31, 685–691. doi:10.1002/humu.21248
Wang, C., Chen, L., Yang, Y., Zhang, M., and Wong, G. (2019b). Identification of potential blood biomarkers for Parkinson's disease by gene expression and DNA methylation data integration analysis. Clin. Epigenet. 11, 1–15. doi:10.1186/s13148-019-0621-5
Wang, H., Lou, D., and Wang, Z. (2019a). Crosstalk of genetic variants, allele-specific DNA methylation, and environmental factors for complex disease risk. Front. Genet. 9, 1–15. doi:10.3389/fgene.2018.00695
Wassouf, Z., Hentrich, T., Samer, S., Rotermund, C., Kahle, P. J., Ehrlich, I., et al. (2018). Environmental enrichment prevents transcriptional disturbances induced by alpha-synuclein overexpression. Front. Cell. Neurosci. 12, 112. doi:10.3389/fncel.2018.00112
Wassouf, Z., and Schulze-Hentrich, J. M. (2019). Alpha-synuclein at the nexus of genes and environment: The impact of environmental enrichment and stress on brain health and disease. J. Neurochem. 150, 591–604. doi:10.1111/jnc.14787
Wen, L., Li, X., Yan, L., Tan, Y., Li, R., Zhao, Y., et al. (2014). Whole-genome analysis of 5-hydroxymethylcytosine and 5-methylcytosine at base resolution in the human brain. Genome Biol. 15, R49. doi:10.1186/gb-2014-15-3-r49
Wen, L., and Tang, F. (2014). Genomic distribution and possible functions of DNA hydroxymethylation in the brain. Genomics 104, 341–346. doi:10.1016/j.ygeno.2014.08.020
Wijetunga, N. A., Johnston, A. D., Maekawa, R., Delahaye, F., Ulahannan, N., Kim, K., et al. (2017). Smite: An R/bioconductor package that identifies network modules by integrating genomic and epigenomic information. BMC Bioinforma. 18, 41. doi:10.1186/s12859-017-1477-3
Wnuk, A., Rzemieniec, J., Litwa, E., Lasoń, W., Krzeptowski, W., Wójtowicz, A. K., et al. (2016). The crucial involvement of retinoid X receptors in DDE neurotoxicity. Neurotox. Res. 29, 155–172. doi:10.1007/s12640-015-9572-6
Wu, H., and Zhang, Y. (2011). Mechanisms and functions of Tet protein-mediated 5-methylcytosine oxidation. Genes Dev. 25, 2436–2452. doi:10.1101/gad.179184.111
Xu, Z., Taylor, J. A., Leung, Y.-K., Ho, S.-M., and Niu, L. (2016). oxBS-MLE: an efficient method to estimate 5-methylcytosine and 5-hydroxymethylcytosine in paired bisulfite and oxidative bisulfite treated DNA. Bioinformatics 32, btw527–3669. doi:10.1093/bioinformatics/btw527
Yu, M., Han, D., Hon, G. C., and He, C. (2018). “Tet-assisted bisulfite sequencing (TAB-seq),” in DNA methylation protocols methods in molecular biology. Editor J. Tost (New York, NY: Springer), 645–663. doi:10.1007/978-1-4939-7481-8_33
Zhang, F., Chen, W., Zhu, Z., Zhang, Q., Nabais, M. F., Qi, T., et al. (2019). Osca: A tool for omic-data-based complex trait analysis. Genome Biol. 20, 107. doi:10.1186/s13059-019-1718-z
Zhang, H.-Q., Wang, J.-Y., Li, Z.-F., Cui, L., Huang, S.-S., Zhu, L.-B., et al. (2021). DNA methyltransferase 1 is dysregulated in Parkinson's disease via mediation of miR-17. Mol. Neurobiol. 58, 2620–2633. doi:10.1007/s12035-021-02298-w
Zhang, T.-Y., Keown, C. L., Wen, X., Li, J., Vousden, D. A., Anacker, C., et al. (2018). Environmental enrichment increases transcriptional and epigenetic differentiation between mouse dorsal and ventral dentate gyrus. Nat. Commun. 9, 298. doi:10.1038/s41467-017-02748-x
Zhang, Y., Xu, S., Qian, Y., He, X., Mo, C., Yang, X., et al. (2022a). Sodium butyrate attenuates rotenone-induced toxicity by activation of autophagy through epigenetically regulating PGC-1α expression in PC12 cells. Brain Res. 1776, 147749. doi:10.1016/j.brainres.2021.147749
Zhang, Z., Lee, M. K., Perreard, L., Kelsey, K. T., Christensen, B. C., and Salas, L. A. (2022b). Navigating the hydroxymethylome: Experimental biases and quality control tools for the tandem bisulfite and oxidative bisulfite illumina microarrays. Epigenomics 14, 139–152. doi:10.2217/epi-2021-0490
Zhao, Q., Liu, H., Cheng, J., Zhu, Y., Xiao, Q., Bai, Y., et al. (2019). Neuroprotective effects of lithium on a chronic MPTP mouse model of Parkinson's disease via regulation of α-synuclein methylation. Mol. Med. Rep. 19, 4989–4997. doi:10.3892/mmr.2019.10152
Zhou, J., Broe, M., Huang, Y., Anderson, J. P., Gai, W.-P., Milward, E. A., et al. (2011). Changes in the solubility and phosphorylation of α-synuclein over the course of Parkinson's disease. Acta Neuropathol. 121, 695–704. doi:10.1007/s00401-011-0815-1
Zhou, W., Barkow, J. C., and Freed, C. R. (2017). Running wheel exercise reduces α-synuclein aggregation and improves motor and cognitive function in a transgenic mouse model of Parkinson's disease. PLoS ONE 12, e0190160. doi:10.1371/journal.pone.0190160
Keywords: Parkinson’s disease, neurodegeneration, epigenetics, DNA methylation, gene-environment interaction
Citation: Schaffner SL and Kobor MS (2022) DNA methylation as a mediator of genetic and environmental influences on Parkinson’s disease susceptibility: Impacts of alpha-Synuclein, physical activity, and pesticide exposure on the epigenome. Front. Genet. 13:971298. doi: 10.3389/fgene.2022.971298
Received: 16 June 2022; Accepted: 25 July 2022;
Published: 19 August 2022.
Edited by:
Katherine Athayde Teixeira De Carvalho, Pelé Pequeno Príncipe Research Institute, BrazilReviewed by:
Lucas A. Salas, Dartmouth College, United StatesCláudia Sirlene Oliveira, Instituto de Pesquisa Pelé Pequeno Príncipe, Brazil
Copyright © 2022 Schaffner and Kobor. This is an open-access article distributed under the terms of the Creative Commons Attribution License (CC BY). The use, distribution or reproduction in other forums is permitted, provided the original author(s) and the copyright owner(s) are credited and that the original publication in this journal is cited, in accordance with accepted academic practice. No use, distribution or reproduction is permitted which does not comply with these terms.
*Correspondence: Michael S. Kobor, michael.kobor@ubc.ca