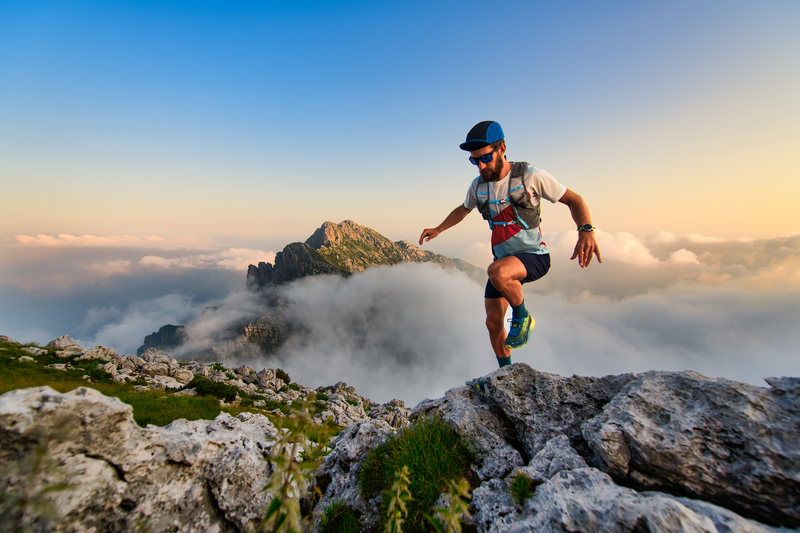
94% of researchers rate our articles as excellent or good
Learn more about the work of our research integrity team to safeguard the quality of each article we publish.
Find out more
ORIGINAL RESEARCH article
Front. Genet. , 10 January 2023
Sec. Neurogenomics
Volume 13 - 2022 | https://doi.org/10.3389/fgene.2022.967730
This article is part of the Research Topic Bioinformatics Applied to Neuroscience View all 16 articles
Chaperonin containing TCP1 subunit 2 (CCT2) is essential in various neurodegenerative diseases, albeit its role in the pathogenesis of Alzheimer’s disease (AD) remains elusive. This study aimed to evaluate the role of CCT2 in Alzheimer’s disease. First, bioinformatics database analysis revealed that CCT2 was significantly downregulated in patients with Alzheimer’s disease and associated with autophagic clearance of β-amyloid. The 789 differentially expressed genes overlapped in AD-group and CCT2-low/high group, and the CCT2-high-associated genes screened by Pearson coefficients were enriched in protein folding, autophagy, and messenger RNA stability regulation pathways. These results suggest that CCT2 is significantly and positively associated with multiple pathways linked to autophagy and negatively associated with neuronal death. The logistic prediction model with 13 key genes, such as CCT2, screened in this study better predicts Alzheimer’s disease occurrence (AUC = 0.9671) and is a favorable candidate for predicting potential biological targets of Alzheimer’s disease. Additionally, this study predicts reciprocal micro RNAs and small molecule drugs for hub genes. Our findings suggest that low CCT2 expression may be responsible for the autophagy suppression in Alzheimer’s disease, providing an accurate explanation for its pathogenesis and new targets and small molecule inhibitors for its treatment.
Alzheimer’s disease (AD) is a neurodegenerative disease responsible for 60–80% of dementia cases, which is characterized by memory loss and reduced cognitive function (Liu et al., 2020). This report indicates that neuronal fibrous tangles caused by Tau hyperphosphorylation in neurons, sedimentation of amyloid beta (Aβ) plaques (Ma et al., 2022), apoptosis of numerous neurons, and loss of neural synapses all contribute to AD. Drugs approved by the FDA for AD are designed to improve the quality of life of patients with the disease albeit may not play an effective therapeutic role in the treatment of AD (2020 AD facts and figures, 2020). AD-related therapeutic drugs based on Aβ starch spot and Tau protein have not made significant progress (Kopeikina et al., 2011); thus, the development of the specific pathogenesis of AD requires further research and exploration. It has been reported that the chaperonin containing TCP1 subunit 2 (CCT2) is poorly expressed in AD; however, the relationship between CCT2 and AD remains elusive (Yuan et al., 2019), implying that there is some link between CCT2 gene expression and the occurrence of AD.
Aggrephagy, a process in which autophagy selectively degrades protein aggregates, is important for removing intracellular toxic protein aggregates and is a key target for the treatment of aggregate-related diseases such as neurodegenerative diseases. Several studies have reported that autophagy deficiency occurs in the early stages of AD (Vaillant-Beuchot L et al., 2021; Roca-Agujetas V et al., 2021). Autophagy is important in the production and metabolism of Aβ, and its dysfunction may contribute to the progression of AD (Li et al., 2017). Traditional ubiquitin-binding receptors (P62, NBR1, and TAX1BP1) can mediate aggrephagy and other types of ubiquitin-related selective autophagy (Zellner S et al., 2021). The novel ubiquitin-binding receptor, CCT2, promotes autophagic clearance of various toxic protein aggregates associated with neurodegenerative diseases (Zhang and Klionsky., 2022). Similar to the conventional ubiquitin-binding receptors, CCT2 binds to LC3 and protein aggregates. CCT2 binds protein aggregates in a ubiquitin-independent manner through its apical domain, laying the groundwork for CCT2-specific aggregate recognition. Research has indicated that conventional autophagy receptors degrade liquid aggregates whereas CCT2 degrades solid aggregates (Ma et al., 2022). Consequently, CCT2 is more likely than autophagy receptors to function and become an AD drug target in pathological states. CCT2 mediates aggrephagy as a monomer, exposing the VLIR domain of the binding site to LC3. The presence of aggregates inhibits the formation of the Chaperonin complex, thus, releasing more CCT2 monomers to promote aggregate clearance (Khaminets et al., 2016; Johansen and Lamark, 2020; Gatica et al., 2018). Full-length tau protein has been reported to preferentially be degraded by macrophage whereas caspase-cleaved tau, tauΔC, which is more likely than natural proteins to aggregate and cause neurotoxicity, is preferentially degraded by autophagy and can turnover faster than the full-length tau. Thus, the autophagy degradation pathway is important in inhibiting the formation of pathological manifestations of AD and has the potential to be a novel target for its treatment (Zare-Shahabadi et al., 2015).
Therefore, this study aimed to investigate the changes in the expression level of CCT2 in patients with AD and its possible pathway involved in autophagy and predict the possible micro RNA targets. Our study may help researchers investigate how CCT2 affects AD via autophagy, contributing to the understanding of disease causes, mechanisms, and treatments.
All the datasets used in this study were obtained from the Gene Expression Omnibus database (https://www.ncbi.nlm.nih.gov/geo/) (Barrett et al., 2013). The AD transcriptome datasets screened from the database included brain tissue sequencing samples, GSE33000, GSE44768, GSE44770, and GSE44771, based on the GPL 4372 platform, peripheral blood samples of patients with AD, GSE140829, based on GPL5988 platform, and serum microRNA (miRNA) sequencing samples, GSE120584, based on the GPL21263 platform. GSE33000, which included 310 patients with AD and 157 controls, was used to explore the potential role of CCT2 in AD. GSE44768, GSE44770, and GSE44771 were obtained from the cerebellum, frontal cortex, and visual cortex, respectively, and included 129 patients with AD and 101 controls. GSE140829 included 204 patients with AD and 249 controls to validate the model and explore CCT2 expression in different tissues. GSE120584 included 1,021 patients with AD and 288 controls and was used to probe the possible messenger RNA (mRNA)-miRNA interaction networks. Component differences were observed using principal component analysis (PCA) plots drawn by the FactoMineR and factoextra packages. The data in GSE33000 was normalized using the normalizeBetweenArrays function in the Limma package (Ritchie et al., 2015), and the first group was retained for duplicated genes in the sequencing data.
LmFit, eBayes from the limma package, and the topTable function were used to identify differentially expressed genes DEGs between AD-con and CCT2-low/high expression groups. According to the false discovery rate (FDR), p ≤ 0.05 was statistically significant, and log2fold change (FC) was used to comprehensively analyze the upregulated and downregulated genes.
For the AD-con group, we selected the first 30% genes with larger |logFC| under the p ≤ 0.05 condition as the DEGs. Further, we divided all patients with AD into high-and low-expression groups based on the median of CCT2, and under the p ≤ 0.05 condition, |logFC| the larger top 10% genes were selected as DEGs in the CCT2-low/high group. DEGs were intersected between AD-con and CCT2-low/high groups for further analysis.
Cor function was used for the raw data, and Pearson correlation analysis was performed between CCT2 and other genes. If p-value was ≤0.05 and the gene was positively associated with CCT2, it was selected as the related gene.
CCT2 and 460 genes with the strongest positive correlation with CCT2 were uploaded to the online Fdatabase—Database for Annotation, Visualization, and Integrated Discovery, 2021 (Sherman et al., 2022; Huang et al., 2009)—for analysis. The official gene symbol was selected as the identifier, and the species was Homo sapiens. This was followed by Gene Ontology (GO) and Kyoto Encyclopedia of Genes and Genomes (KEGG) pathway enrichment analysis. The top eight pathway are displayed in ascending order of p-value (p ≤ 0.05).
The differential expression analysis results of the limma package were analyzed by GSEA using the gseKEGG and gseGO functions of the clusterProfiler package in R (Wu et al., 2021), and biological process (BP) GO terms and KEGG pathways that may be related to AD and CCT2 expression were explored. p ≤ 0.05 and |NES| >1 indicated significant differences.
Gene sets related to autophagy and protein folding were obtained from the GSEA website (http://www.gsea-msigdb.org/gsea/index.jsp) (Subramanian et al., 2005; Mootha et al., 2003). Before standardization, the GSVA package in R was used to calculate the functional enrichment scores of all AD groups in the GSE33000 dataset, and the parameters were set as default (Hänzelmann et al., 2013). Results were visualized by drawing heatmaps using the pheatmap package in R, and Pearson correlation analysis was used to determine the correlation between CCT2 and autophagy and protein folding processes. Further, the top ten genes with the strongest positive and negative correlation with CCT2 were drawn to exhibit their correlation with CCT2 using data from the HADb database (http://www.autophagy.lu/index.Autopophagy-related gene sets of html) (Moussay et al., 2011) and circos package (Krzywinski et al., 2009). Relevant gene sets from different stages of autophagy were selected for GSVA analysis; Pearson correlation analysis was used to calculate its correlation coefficient; the corrgram package was used to construct matrix plots.
The DEGs from the AD-con groups intersected with the CCT2 low-high groups, and 295 upregulated and 494 downregulated genes were removed as co-DEGs and uploaded to the online database (STRING version 11.0, https://cn.string-db.org/) (Szklarczyk et al., 2021) to predict the PPI network, with the default parameters. The PPI interaction network was further drawn using Cytoscape, and 36 hub genes associated with CCT2 were removed using the MCODE plugin.
The least absolute shrinkage and selection operator (LASSO) is a compression estimation method that has a strong factor screening ability (Tibshirani,1997; Zou et al., 2019). The hub genes were intersected using Pearson’s analysis results (|r|≥0.65, p ≤0.05) to obtain 26 genes, and the expression profiles of these genes were used to construct the LASSO model, with 13 genes whose regression coefficient was not zero. These genes were used to construct a logistic regression model using the glmnet package. This model had the following formula: index = EXGene1×Coef1 + EXGene2×Coef2 + EXGene3×Coef3+…… (Coef was the regression coefficient, derived from the logistic regression (Domínguez-Almendros et al., 2011); EXGene was the gene expression level).
Further, data from the GSE33000 dataset were randomly assigned to the test set (30%) and validated with those of the GSE44768, GSE44770, GSE44771, and GSE140829 datasets, and the ROC curve was drawn using the pROC package.
Using the online database, AlzDate (http://www.alzdata.org/) (Xu et al., 2018; Zhang et al., 2019) and the Single Cell Expression tool, CCT2 expression in single cells was obtained. Using the Differential Expression tool, the differential expression of CCT2 in multiple databases was obtained.
MicroRNA is a type of single-stranded RNA molecule that is encoded by endogenous genes and binds to mRNA inside cells to inhibit protein translation. Exploring the interaction between miRNA and its target genes can provide a reference for investigating the disease causes and therapeutic methods. Databases for predicting gene-miRNA interactions include MiRDB, miRWalk, RNA22, and RNAInter (Chen and Wang, 2020; Dweep et al., 2011; Miranda et al., 2006; Lin et al., 2020). They were used to predict the miRNA interactions with hub genes, and the results were cross-checked to improve prediction accuracy. Simultaneously, serum miRNA sequencing samples (GSE120584) were analyzed for differential expression using the limma package, and miRNAs with p ≤ 0.05 were considered differentially expressed. Additionally, the differentially expressed miRNAs that interacted with hub genes were visualized using the Cytoscape software.
Drug development has always prioritized research on drugs for AD. Numerous effective drugs are ineffective in AD treatment as they cannot cross the blood-brain barrier (BBB) whereas small-molecule drugs have natural advantages in crossing the BBB.
CCT2 has been reported to be used as a target of small-molecule drugs in the treatment of neurodegenerative diseases. Consequently, the prediction of CCT2-related DEGs serves as a reference for AD therapy. The Drug Signatures database (DSigDB) on the Enrichr website was used in this study to identify relevant targeted drugs for DEGs (Chen EY et al., 2013; Kuleshov et al.,., 2016; Xie Z et al., 2021). The results were reviewed and displayed (Kuleshov et al., 2016).
To investigate the differences in gene transcriptome between AD and normal controls, we conducted the following analysis. First, the PCA chart demonstrated that there are significant differences between AD and con groups in GSE33000, allowing for subsequent analysis (Figure 1A). Boxchart displayed standardized data, eliminating intra-group differences (Figure 1B). Second, we observed that CCT2 was significantly downregulated in AD, p = -1.26e-22 and logFC = -0.07632 (Figure 1C), which suggested that the low CCT2 expression is associated with AD. There were 4,381 DEGs in AD, with 2,152 upregulated and 2,229 downregulated genes (Figure 1E), among which the top 50 upregulated and 50 downregulated genes are indicated in the heatmap (Figure 1D). However, the CCT2-low group had 1,273 DEGs compared with the CCT2-high group, of which 561 were upregulated and 712 were downregulated (Figure 1F). A total of 789 genes were either upregulated or downregulated in the AD-con and CCT2 low-high groups, which may be associated with both CCT2 expression and AD.
FIGURE 1. Differential expression analysis. (A) Principal component analysis (PCA) plot demonstrating differences among groups, with Alzheimer’s disease (AD) in red and con in green. (B) Boxchart before and after standardization—the upper figure is before standardization; the following figure is after standardization. (C) Chaperonin containing TCP1 subunit 2 (CCT2) was downregulated in AD (p = 1.26e-22, logFC = -0.07632). (D) Heatmap of the top 50 upregulated and downregulated genes between AD and control. (E) Volcano plot of AD-con, with upregulated genes in red and downregulated genes in blue. (F) Volcano plot of CCT2-low/high, with upregulated genes in red and downregulated genes in blue.
Four hundred and sixty genes with the strongest positive correlation with CCT2 were selected using Pearson correlation analysis to explore the relevant biological functions of CCT2. GO and KEGG analyses were performed according to the above-mentioned gene sets. Genes associated with CCT2 in biological processes (BP) are primarily enriched in protein folding pathways, regulation of telomere protein localization related to the Cajal body, and regulation of mRNA stability (Figure 2A). Additionally, the most relevant cellular components (CC) of CCT2 and its related genes included cell cytoplasm and T complex proteins (Figure 2B) and were related to exosomes (Figure 2C), whose molecular function (MF) was protein binding and folding, RNA binding, and ribosome composition. Moreover, the most related signaling pathway (KEGG) was mainly associated with various neurodegenerative diseases, including AD, and autophagy (Figure 2D). These findings indicated that downregulating CCT2 in patients with AD may be significant for snRNP formation, mRNA splicing, protein folding, and clearance of misfolded proteins by autophagy. Thus, CCT2 was associated with the production and clearance of amyloid proteins, and a possible cause of AD was CCT2 downregulation.
FIGURE 2. Chaperonin containing TCP1 subunit 2 (CCT2) was closely related to the process of β-amyloid formation and clearance in AD. (A–C) Biological processes (BP), cellular components (CC), and molecular functions (MF) were enriched in gene sets that were positively associated with CCT2 in Pearson’s test, whose credibility gradually increases from blue to red, and the size of the circle exhibits the number of genes contained in the corresponding pathway. (D) The signaling pathways (Kyoto Encyclopedia of Genes and Genomes (KEGG)) were enriched in the gene set that was positively associated with CCT2 in Pearson’s test, whose credibility gradually increased from blue to red, and the size of the circle exhibits the number of genes contained in the corresponding pathway.
The transcriptomic data was analyzed using GSEA and GSVA. In GSEA, the Janus kinase (JAK)-signal transducer and activator of transcription (STAT) signaling pathway and the Notch signaling pathway were significantly enriched in the AD group compared to the con group; however, there was a contrasting observation in proteasome and animal autophagy (Figure 3A). Meanwhile, there was a similar trend in the CCT2-low/high group (Figure 3B). Additionally, compared with the con group, glial cell development and differentiation and angiogenesis-related pathways were significantly enriched in AD, opposing the observation in protein catabolism and neuronal development (Figure 3C), with a similar trend in the CCT2-low/high group (Figure 3D). This suggests that the downregulation of CCT2 may be a cause of AD. It has been proven that the accumulation of amyloid protein can affect the production of angiogenic factors (Skaaraas et al., 2021).
FIGURE 3. Correlation of Chaperonin containing TCP1 subunit 2 (CCT2) expression with autophagy and other gene sets. (A) Biological processes (BP) of Alzheimer’s disease (AD) enrichment. (B) BP of CCT2-low enrichment. (C) The Kyoto Encyclopedia of Genes and Genomes (KEGG) pathways enriched in AD. (D) The KEGG pathway enriched in CCT2-low. (E) Heatmaps display the enrichment scores for CCT2 expression and related pathways in GSE33000; samples are arranged in CCT2 ascending order, and bar and line plots on the right indicate the analyzed R and p-values. (F) Autophagy-related genes positively associated with CCT2, as indicated in red. (G) Autophagy-related genes negatively associated with CCT2, as indicated in blue. (H) Correlation of CCT2 with autophagy-related gene sets, the correlation coefficient is presented in the lower left and simultaneously in the upper right, red for positive correlation and green for the negative correlation.
In AD progression, CCT2 may alleviate amyloid aggregation by promoting normal protein folding and autophagy. Thus, the method used by the study was GSVA for calculating the enrichment score of CCT2 expression levels for several pathway and BP. These include neuronal death regulation, mitochondrial autophagy, chaperone-mediated autophagy, peroxisomal autophagy, and other related pathway. BP such as protein folding and its decomposition. The enrichment score indicated that CCT2 was positively correlated with most autophagy-related BP and protein folding; however, it was reversed in the negative regulation of neuronal death and the negative regulation of mitochondrial autophagy. (Figure 3E). These results indicate that high CCT2 expression can inhibit neuronal death while enhancing mitophagy, which is considered to be the target of AD treatment (Xie et al., 2022).
Since CCT2 was observed to be involved in AD, we investigated its relationship with the expression levels of some important autophagy-related genes. The results indicated that CCT2 expression was positively correlated with MAPK8, HSPA8, NCKAP1, RAB11A, and RAB1A (Figure 3F), and negatively associated with BAX, MAPK3, ITGB4, ATG16L2, and ERBB2 (Figure 3G). Using the Pearson matrix diagram (Figure 3H), high CCT2 expression revealed a significant and positive correlation with autophagy formation, macroautophagy, and autophagy mediated by molecular chaperones and a negative correlation with the mTOR pathway. This validates that CCT2 downregulation affects normal autophagy for clearing Tau and Aβ, thus, causing AD.
Through ppi network analysis, we screened 36 hub genes from co-DEGs, including CCT2, ACTR2, CLTA et al. Using the results obtained from the PPI network analysis (Supplementary Figure one to two), we extracted the expression profiles of the hub genes to construct a predictive model. Using LASSO regression, 12 genes were selected with non-zero regression coefficients and value of lambda. min = 0.003690707 (Figures 4A,B). CCT2 was further used to construct logistic regression prediction models as follows: risk score = (4.0041× ARAF- 1.9746× ACTR2- 5.8043× ATP5F1+ 15.7535× ATP6V1A+ 9.4168× ATP6V1C1- 15.9159× CA10–1.8964× GNG11 + 4.0073× NRXN1- 10.1124× PPFIA2+ 4.2734× PPP1R1B- 11.3482× PPP2CA- 4.1251× RAN+ 2.2023× CCT2). The heatmap indicated the relationship between prediction score and disease, age, and related genes (Figure 4C). The ROC curve indicates that the area under curve (AUC) is 0.9671 and 0.9700 (Figure 4D,E) in the training and validation sets, respectively. In the external validation set (GSE44768, GSE44770, GSE44771, and GSE140829), AUC values were 0.9681, 0.9724, 0.923, and 0.6342 for prefrontal samples, hippocampal samples, cerebellar samples, and whole blood samples, respectively (Figure 4F), indicating that the model has high accuracy in AD prediction.
FIGURE 4. A logistic model for identifying Alzheimer’s disease (AD) and the expression of Chaperonin containing TCP1 subunit 2 (CCT2) in different tissues and cells. (A–B) Least absolute shrinkage and selection operator (LASSO) model. (C) Heatmap demonstrates changes in category, age, and gene expression as predictive scores rise. (D) Receiver operating characteristic (ROC) curve analysis of the training set (GSE33000). (E) ROC curve analysis of the validation set (GSE33000). (F) The ROC curve analysis of the external validation set (GSE44768, GSE44770, GSE44771, and GSE140829). (G) CCT2 expression observed in various cells of the brain. (H) CCT2 was significantly downregulated in various brain regions of patients with AD.
Additionally, CCT2 was expressed in all cell types in the human brain (Figure 4G) and significantly downregulated in various brain regions (Figure 4H), indicating that CCT2 and its related genes are significantly correlated with AD and have broad prospects as a biomarker.
There are several studies suggesting that miRNA acts on target genes through exosomes and thus affects neurodegenerative diseases (Lydie et al., 2013; Jiang et al., 2019). We used four miRNA databases for joint prediction (Figure 5A), among which three were predicted for CCT2, including miR-196b-3p, miR-4778–3p, and miR-6740–3p, where miR-6740–3p was significantly different in blood samples (Figure 5B). Thus, miR-6740–3p may inhibit CCT2 translation by binding to its transcript, which may be a potential cause of AD. We analyzed all 36 hub genes in the same way, and the mRNA-miRNA interaction network revealed that the majority of the miRNAs corresponding to the downregulated genes in AD were upregulated, confirming that the interaction network had a good predictive value (Figure 5C).
FIGURE 5. The mRNA-miRNA interaction network. (A) Four databases—miRDB, miRWalk, RNA22, and RNAInter—were combined for CCT2-targeting miRNA prediction. (B) The boxplot demonstrates the expression level of miR-6740–3p in the serum samples (GSE120584) (logFC = 0.38540845, p = 2.34E-16). (C) The interaction network presents the hub genes and their corresponding miRNA, with octagon nodes representing genes and other shapes representing miRNA. Upregulation is indicated in red; downregulation is indicated in green.
CCT2 may be a novel target for AD therapy, thus, the protein-drug interactions must be predicted. Since macromolecular drugs are difficult to cross the blood-brain barrier, we used the DSingDB database for model gene prediction to identify ten viable small molecule drugs. Additionally, they were sorted and displayed based on the p-value (Table 1).
Some studies have shown that Amantadine may have a new beneficial effect on axial symptoms of Parkinson’s disease (PD) patients with subthalamic nucleus deep brain stimulation (Chan et al., 2013). Additionally, Reserpine is an antihypertensive agent whose action is attributed to its ability to inhibit the vesicle monoamine transporter VMAT, thereby reducing the level of bioamine neurotransmitters in synaptic vesicles. One study found that reserpine improves Aβ toxicity in caenorhabditis elegans model of AD (Arya et al., 2009). Similarly, several small-molecule drugs seem to be associated with AD. Elevated enkephalins cause neuronal and behavioral disorders in transgenic mouse models of AD (Meilandt et al., 2008). Caffeic acid slows the development of AD by increasing cognitive function, alleviating brain damage, and inhibiting the AD-induced increase in AChE activity and nitrite production (Wang et al., 2016).
AD has gradually grown to be one of the most significant diseases of this century as a typical neurodegenerative condition affecting the elderly. Over 50 million people worldwide currently suffer from dementia, with AD accounting for 60–80% of all dementia cases (Porsteinsson et al., 2021). Acetylcholinesterase inhibitor (AChEI) is a common drug for the treatment of AD; however, it can only treat its symptoms and have some side effects (Lane et al., 2018). Studies have reported that AD is caused by abnormal folding of Aβ protein, and the neurofibrillary tangles are caused by excessive phosphorylation of Tau (Kozlov et al., 2017), which is accompanied by neuronal apoptosis, which is irreversible. Therefore, the onset of AD is usually irreversible (Ganzer, 2007).
Previous studies have demonstrated the eukaryotic chaperone tailless complex polypeptide one ring complex and its eight subunits can prevent the formation of protein aggregates (Behrends et al., 2006; Noormohammadi et al., 2016). A recently published paper has demonstrated that CCT2 binds to protein aggregates, recruits autophagosomes to endocytose, and degrades Tau (Ma et al., 2022). Therefore, our study used the transcriptome sequencing dataset of AD prefrontal cells to explore the regulatory mechanism of CCT2 in AD. We observed that CCT2 was significantly downregulated in patients with AD, suggesting that the CCT2 downregulation may be a contributing factor for AD. By considering the intersection of AD-con and CCT2-low/high, we identified the common DEGs. Further, using PPI network analysis, 36 hub genes of co-DEGs were screened out, including CCT2, ACTR2, and TCIRG1. Among them, MYT1L was observed to induce cell reprogramming into cholinergic neurons and provide a strategy for treating AD (Liang et al., 2018). ATP6V1A is involved in AD via the synaptic vesicle cycle, phagosome, and oxidative phosphorylation downregulation (Zhou et al., 2021). Additionally, VDAC1 has been observed to impact AD occurrence by regulating mitochondrial function (Shoshan-Barmatz et al., 2018). This indicates a strong correlation between hub genes and AD occurrence. Since CCT2 is also closely related to these genes, it may play an important role in the regulation of hub genes, providing a reference for future research.
However, functional enrichment analysis revealed that the genes positively correlated with CCT2, selected by Pearson correlation coefficient, were associated with telomerase, Cajal body positioning, mRNA splicing, protein folding, and MAPK activity, indicating that the genes highly associated with CCT2 are involved in the entire process of transcription and translation. This is also consistent with the mechanism of co-regulation of protein homeostasis by a molecular chaperone and aggregate autophagy reported in a study by Ma et al. (Ma et al., 2022). Meanwhile, the MAPK signaling pathway is believed to function during the early stages of AD (Johnson and Bailey, 2003), and the pathway enrichment analysis revealed that it is related to multiple neurodegenerative diseases and autophagy in animals whereas autophagic deficiency is a widely recognized cause of AD (Reddy and Oliver, 2019; Fang et al., 2019).
Meanwhile, GSEA analysis of the DEGs and GSVA analysis of the CCT2-related genes revealed that CCT2 affects autophagy. GSEA analysis demonstrated that the JAK-STAT pathway, the Notch signaling pathway, angiogenesis, and development and differentiation of glial cells were enriched in AD-con. At the same time, CCT2-low/high groups whereas proteasome, animal autophagy, protein breakdown, and neuronal development were not enriched. Several pathways have been reported to correlate with AD occurrence. For example, the JAK-STAT signaling pathway has been reported to play a potential role in the molecular mechanism regulating cellular autophagy (Chen et al., 2021). The Notch pathway is linked to autophagy (Ko et al., 2020), and angiogenesis is also believed to promote AD (Vagnucci and Li, 2003). Additionally, there is evidence that the AD and the CCT2-low expression groups can clear Aβ and Tau by inhibiting proteasomes and autophagy (Bonet-Costa et al., 2016). In contrast, GSVA revealed that the expression level of CCT2 was negatively associated with neuronal death, suggesting that high CCT2 expression can inhibit neuronal death. Furthermore, the CCT2 expression level was significantly and positively correlated with mitophagy, macroautophagy, pexophagy, protein folding, and protein metabolism; however, the R-value for mitophagy was relatively low, possibly since CCT2 does not directly affect mitophagy. This is consistent with the first report of CCT2-mediated aggregate elimination and indicates that CCT2 can regulate the levels of Aβ and Tau by regulating protein folding and promoting autophagy. Increasing evidence suggests that autophagy plays a role in scavenging abnormal proteins, thus, affecting the clearance of Aβ and Tau when autophagy activation is hampered (Dou et al., 2020). Hence, autophagy dysfunction plays a crucial role in the pathological process of AD (Li et al., 2010).
We also analyzed the correlation between CCT2 and autophagy-related genes, and top ten autophagy genes with the strongest positive correlation included BNIP3, HSPA8, and MAPK8 etc. Whereas those with the strongest negative correlation included ATG16L2, ATG9B, and BAX etc. This indicates that CCT2 may affect autophagy by interacting with genes that are highly associated with autophagy. The autophagy gene set correlation analysis revealed that CCT2 is positively correlated with the initiation stage of autophagy, macroautophagy, and chaperone-mediated autophagy, which also suggested that CCT2 may affect the occurrence of autophagy via some mechanism. We also observed the inhibition of the mammalian target of the rapamycin (mTOR) pathway. Additionally, high expression of mTOR-inhibiting autophagy has been demonstrated in neurodegenerative diseases (Zhu et al., 2019).
During the construction of the clinical prediction model, we used LASSO regression to screen for genes with regression coefficients greater than zero and then combined these genes with CCT2 to build the logistic model. The model performed well with high AUC values in the prefrontal cortex, visual cortex, and hippocampus; however, it performed poorly in whole blood samples, which could be attributed to the fact that the brain tissue samples were used to construct the model. Some of the genes involved in the model have been observed to be involved in AD, of which CCT2 is significantly downregulated in AD, PPP1R1B can regulate cAMP response element-binding protein (CREB) phosphorylation, and CREB dysfunction is one of the causes of AD (Cho et al., 2015) whereas NRXN1 is involved in memory recovery in rats by affecting synaptic plasticity (Zhang et al., 2021). The ROC curve revealed that the model had high AUC values in the training, test, and external validation sets; thus, the expression of these genes can be used as a biomarker for AD. Online database analysis also demonstrated that CCT2 is expressed in various brain cells, with neuronal cells having the highest levels of expression. CCT2 expression, in contrast, was significantly reduced in various brain regions, including the entorhinal cortex, hippocampus, frontal cortex, and frontal cortex, providing further evidence that low CCT2 expression is one of the mechanisms of AD pathogenesis.
The study also investigated the causes of low CCT2 expression and observed that gene mutations, DNA methylation, and miRNA may cause changes in gene expression, causing AD (Qin et al., 2020; De Jager et al., 2014; Akhter and Bekris, 2019). Thus, we aimed to explore the miRNA interacting with hub genes, most of which had the opposite expression profile of their target genes in AD. For example, miR-6740–3p, which interacts with CCT2, is significantly upregulated in AD (logFC = 0.38540845, p = 2.34E-16) and miR-661, which interacts with HPCAL4, NECAP1, CLTA, and GNAI2, has been observed to be involved in AD via metabolic and stress pathways (Hojati et al., 2021). The miR-501–3p, which interacts with CADM2, may impact AD by regulating cell division (Hara et al., 2017); hsa-miR-107, which interacts with ACTR2, AMPH, and RAN, targets Aβ precursor protein (APP) and influences AD (Hébert et al., 2008). This study can assist researchers in screening for appropriate miRNA and validating their biological functions to obtain effective biological results. (The specific pathways by which most miRNAs affect AD are currently unknown, albeit the mRNA-miRNA interaction network serves as a reference for AD diagnosis and treatment. Similarly, the prediction of the last small-molecule drugs provides a point of reference for targeting CCT2 to treat AD.
Using bioinformatic analysis, this study used multiple datasets and revealed that the low expression of CCT2 in AD may be responsible for the inhibition of autophagy in AD. The PPI network was used to screen out potential AD biomarkers with diagnostic value, and the mRNA-miRNA interaction network was constructed to predict the potential miRNA. These findings contribute to our understanding of the pathogenesis of AD and provide new guidelines for the treatment and diagnosis of the disease.
Publicly available datasets were analyzed in this study. The names of the repository/repositories and accession number(s) can be found in the article/Supplementary Material.
Z-LL provided the experimental idea of this study and reviewed and modified the manuscript. M-XT and F-YX designed the experiments and collected the data; M-XT verified the experiments, analyzed the data, and visualized the results; G-BY and Q-XY edited pictures and tables; M-XT, F-YX, and Q-XY wrote the initial manuscript, with F-XQ and L-GD providing amendments and financial assistance. All authors contributed to this article and validated the final version to be submitted.
This work was supported by the National Key Research and Development Program of China [Grant Number 2021YFA1500400]. Science and Technology Department of Jilin Province [Grant Number 20200201386JC]. Science and Technology Department of Jilin Province [Grant Number 20190701037 GH]. Education department of Jilin Province [Grant Number JJKH20200948KJ]. The funding bodies played no role in study design, in the collection, analysis, and interpretation of data, in the report’s writing, and in the decision to submit the article for publication.
The authors declare that the research was conducted in the absence of any commercial or financial relationships that could be construed as a potential conflict of interest.
All claims expressed in this article are solely those of the authors and do not necessarily represent those of their affiliated organizations, or those of the publisher, the editors and the reviewers. Any product that may be evaluated in this article, or claim that may be made by its manufacturer, is not guaranteed or endorsed by the publisher.
The Supplementary Material for this article can be found online at: https://www.frontiersin.org/articles/10.3389/fgene.2022.967730/full#supplementary-material
Alzheimer's Association (2020). 2020 alzheimer's disease facts and figures. Alzheimer's Dementia 16 (3), 391–460. doi:10.1002/alz.12068
Akhter, R., and Bekris, L. M. (2019). Potential role of miRNA-140 in alzheimer's disease. Aging (Albany, NY.) 11 (4), 1087–1088. doi:10.18632/aging.101827
Arya, U., Dwivedi, H., and Subramaniam, J. R. (2009). Reserpine ameliorates Abeta toxicity in the Alzheimer's disease model in Caenorhabditis elegans. Exp. Gerontol. 44 (6-7), 462–466. doi:10.1016/j.exger.2009.02.010
Barrett, T., Wilhite, S. E., Ledoux, P., Evangelista, C., Kim, I. F., Tomashevsky, M., et al. (2013). NCBI geo: Archive for functional genomics data sets-update. Nucleic Acids Res. 41, D991–D995. doi:10.1093/nar/gks1193
Behrends, C., Langer, C. A., Boteva, R., Böttcher, U. M., Stemp, M. J., Schaffar, G., et al. (2006). Chaperonin TRiC promotes the assembly of polyQ expansion proteins into nontoxic oligomers. Mol. Cell. 23 (6), 887–897. doi:10.1016/j.molcel.2006.08.017
Bonet-Costa, V., Pomatto, L. C., and Davies, K. J. (2016). The proteasome and oxidative stress in alzheimer's disease. Antioxid. Redox Signal. 25 (16), 886–901. doi:10.1089/ars.2016.6802
Chan, H. F., Kukkle, P. L., Merello, M., Lim, S. Y., Poon, Y. Y., and Moro, E. (2013). Amantadine improves gait in PD patients with STN stimulation. Park. Relat. Disord. 19 (3), 316–319. doi:10.1016/j.parkreldis.2012.11.005
Chen, D., Liu, Y., Chen, J., Lin, H., Guo, H., Wu, Y., et al. (2021). JAK/STAT pathway promotes the progression of diabetic kidney disease via autophagy in podocytes. Eur. J. Pharmacol. 902, 174121. doi:10.1016/j.ejphar.2021.174121
Chen, E. Y., Tan, C. M., Kou, Y., Duan, Q., Wang, Z., Meirelles, G. V., et al. (2013). Enrichr: Interactive and collaborative HTML5 gene list enrichment analysis tool. BMC Bioinforma. 14, 128. doi:10.1186/1471-2105-14-128
Chen, Y., and Wang, X. (2020). miRDB: an online database for prediction of functional microRNA targets. Nucleic Acids Res. 48 (D1), D127-D131–D131. doi:10.1093/nar/gkz757
Cho, K., Cho, M. H., Seo, J. H., Peak, J., Kong, K. H., Yoon, S. Y., et al. (2015). Calpain-mediated cleavage of DARPP-32 in Alzheimer's disease. Aging Cell. 14 (5), 878–886. doi:10.1111/acel.12374
De Jager, P. L., Srivastava, G., Lunnon, K., Burgess, J., Schalkwyk, L. C., Yu, L., et al. (2014). Alzheimer's disease: Early alterations in brain DNA methylation at ANK1, BIN1, RHBDF2 and other loci. Nat. Neurosci. 17 (9), 1156–1163. doi:10.1038/nn.3786
Domínguez-Almendros, S., Benítez-Parejo, N., and Gonzalez-Ramirez, A. R. (2011). Logistic regression models. Allergol. Immunopathol. 39 (5), 295–305. doi:10.1016/j.aller.2011.05.002
Dou, J., Su, P., Xu, C., Wen, Z., Mao, Z., and Li, W. (2020). Targeting Hsc70-based autophagy to eliminate amyloid β oligomers. Biochem. Biophys. Res. Commun. 524 (4), 923–928. doi:10.1016/j.bbrc.2020.02.016
Dweep, H., Sticht, C., Pandey, P., and Gretz, N. (2011). miRWalk-database: prediction of possible miRNA binding sites by "walking" the genes of three genomes. J. Biomed. Inf. 44 (5), 839–847. doi:10.1016/j.jbi.2011.05.002
Fang, E. F., Hou, Y., Palikaras, K., Adriaanse, B. A., Kerr, J. S., Yang, B., et al. (2019). Mitophagy inhibits amyloid-β and tau pathology and reverses cognitive deficits in models of Alzheimer's disease. Nat. Neurosci. 22 (3), 401–412. doi:10.1038/s41593-018-0332-9
Ganzer, C. A. (2007). Assessing alzheimer's disease and dementia: Best practices in nursing care. Geriatr. Nurs. 28 (6), 358–365. doi:10.1016/j.gerinurse.2007.10.008
Gatica, D., Lahiri, V., and Klionsky, D. J. (2018). Cargo recognition and degradation by selective autophagy. Nat. Cell. Biol. 20 (3), 233–242. doi:10.1038/s41556-018-0037-z
Hänzelmann, S., Castelo, R., and Guinney, J. (2013). Gsva: Gene set variation analysis for microarray and RNA-seq data. BMC Bioinforma. 14, 7. doi:10.1186/1471-2105-14-7
Hara, N., Kikuchi, M., Miyashita, A., Hatsuta, H., Saito, Y., Kasuga, K., et al. (2017). Serum microRNA miR-501-3p as a potential biomarker related to the progression of Alzheimer's disease. Acta Neuropathol. Commun. 5 (1), 10. doi:10.1186/s40478-017-0414-z
Hébert, S. S., Horré, K., Nicolaï, L., Papadopoulou, A. S., Mandemakers, W., Silahtaroglu, A. N., et al. (2008). Loss of microRNA cluster miR-29a/b-1 in sporadic Alzheimer's disease correlates with increased BACE1/beta-secretase expression. Proc. Natl. Acad. Sci. U. S. A. 105 (17), 6415–6420. doi:10.1073/pnas.0710263105
Hojati, Z., Omidi, F., Dehbashi, M., and Mohammad Soltani, B. (2021). The highlighted roles of metabolic and cellular response to stress pathways engaged in circulating hsa-miR-494-3p and hsa-miR-661 in alzheimer's disease. Iran. Biomed. J. 25 (1), 62–67. doi:10.29252/ibj.25.1.62
Huang, d., Sherman, B. T., and Lempicki, R. A. (2009). Systematic and integrative analysis of large gene lists using DAVID bioinformatics resources. Nat. Protoc. 4 (1), 44–57. doi:10.1038/nprot.2008.211
Jiang, Y., Liu, J., Chen, L., Jin, Y., Zhang, G., Lin, Z., et al. (2019). Serum secreted miR-137-containing exosomes affects oxidative stress of neurons by regulating OXR1 in Parkinson’s disease. Brain Res. 1722, 146331. doi:10.1016/j.brainres.2019.146331
Johansen, T., and Lamark, T. (2020). Selective autophagy: ATG8 family proteins, LIR motifs and cargo receptors. J. Mol. Biol. 432 (1), 80–103. doi:10.1016/j.jmb.2019.07.016
Johnson, G. V., and Bailey, C. D. (2003). The p38 MAP kinase signaling pathway in Alzheimer's disease. Exp. Neurol. 183 (2), 263–268. doi:10.1016/s0014-4886(03)00268-1
Khaminets, A., Behl, C., and Dikic, I. (2016). Ubiquitin-Dependent and independent signals in selective autophagy. Trends Cell. Biol. 26 (1), 6–16. doi:10.1016/j.tcb.2015.08.010
Ko, S. H., Apple, E. C., Liu, Z., and Chen, L. (2020). Age-dependent autophagy induction after injury promotes axon regeneration by limiting NOTCH. Autophagy 16 (11), 2052–2068. doi:10.1080/15548627.2020.1713645
Kopeikina, K. J., Carlson, G. A., Pitstick, R., Ludvigson, A. E., Peters, A., Luebke, J. I., et al. (2011). Tau accumulation causes mitochondrial distribution deficits in neurons in a mouse model of tauopathy and in human alzheimer's disease brain. Am. J. Pathol. 179 (4), 2071–2082. doi:10.1016/j.ajpath.2011.07.004
Kozlov, S., Afonin, A., Evsyukov, I., and Bondarenko, A. (2017). Alzheimer's disease: As it was in the beginning. Rev. Neurosci. 28 (8), 825–843. doi:10.1515/revneuro-2017-0006
Krzywinski, M., Schein, J., Birol, I., Connors, J., Gascoyne, R., Horsman, D., et al. (2009). Circos: An information aesthetic for comparative genomics. Genome Res. 19 (9), 1639–1645. doi:10.1101/gr.092759.109
Kuleshov, M. V., Jones, M. R., Rouillard, A. D., Fernandez, N. F., Duan, Q., Wang, Z., et al. (2016). Enrichr: A comprehensive gene set enrichment analysis web server 2016 update. Nucleic Acids Res. 44 (W1), W90–W97. doi:10.1093/nar/gkw377
Lane, C. A., Hardy, J., and Schott, J. M. (2018). Alzheimer's disease. Eur. J. Neurol. 25 (1), 59–70. doi:10.1111/ene.13439
Li, L., Zhang, X., and Le, W. (2010). Autophagy dysfunction in Alzheimer's disease. Neurodegener. Dis. 7 (4), 265–271. doi:10.1159/000276710
Li, Q., Liu, Y., and Sun, M. (2017). Autophagy and alzheimer's disease. Cell. Mol. Neurobiol. 37 (3), 377–388. doi:10.1007/s10571-016-0386-8
Liang, X. G., Tan, C., Wang, C. K., Tao, R. R., Huang, Y. J., Ma, K. F., et al. (2018). Myt1l induced direct reprogramming of pericytes into cholinergic neurons. CNS Neurosci. Ther. 24 (9), 801–809. doi:10.1111/cns.12821
Lin, Y., Liu, T., Cui, T., Wang, Z., Zhang, Y., Tan, P., et al. (2020). RNAInter in 2020: RNA interactome repository with increased coverage and annotation. Nucleic Acids Res. 48 (D1), D189-D197–D197. doi:10.1093/nar/gkz804
Liu, L., Wu, Q., Zhong, W., Chen, Y., Zhang, W., Ren, H., et al. (2020). Microarray analysis of differential gene expression in alzheimer's disease identifies potential biomarkers with diagnostic value. Med. Sci. Monit. Int. Med. J. Exp. Clin. Res. 26, e919249. doi:10.12659/MSM.919249
Lydie, M., Melissa, R., Haruki, H., Seng, K. N., Christine, E., Svetlana, V., et al. (2013). Neuronal exosomal miRNA-dependent translational regulation of astroglial glutamate transporter GLT1. J. Biol. Chem. 288 (10), 7105–7116. doi:10.1074/jbc.M112.410944
Ma, X., Lu, C., Chen, Y., Li, S., Ma, N., Tao, X., et al. (2022). CCT2 is an aggrephagy receptor for clearance of solid protein aggregates. Cell. 185 (8), 1325–1345. e22. doi:10.1016/j.cell.2022.03.005
Meilandt, W. J., Yu, G. Q., Chin, J., Roberson, E. D., Palop, J. J., Wu, T., et al. (2008). Enkephalin elevations contribute to neuronal and behavioral impairments in a transgenic mouse model of Alzheimer's disease. J. Neurosci. 28 (19), 5007–5017. doi:10.1523/JNEUROSCI.0590-08.2008
Miranda, K. C., Huynh, T., Tay, Y., Ang, Y. S., Tam, W. L., Thomson, A. M., et al. (2006). A pattern-based method for the identification of MicroRNA binding sites and their corresponding heteroduplexes. Cell. 126 (6), 1203–1217. doi:10.1016/j.cell.2006.07.031
Mootha, V. K., Lindgren, C. M., Eriksson, K. F., Subramanian, A., Sihag, S., Lehar, J., et al. (2003). PGC-1alpha-responsive genes involved in oxidative phosphorylation are coordinately downregulated in human diabetes. Nat. Genet. 34 (3), 267–273. doi:10.1038/ng1180
Moussay, E., Kaoma, T., Baginska, J., Muller, A., Van Moer, K., Nicot, N., et al. (2011). The acquisition of resistance to TNFα in breast cancer cells is associated with constitutive activation of autophagy as revealed by a transcriptome analysis using a custom microarray. Autophagy 7 (7), 760–770. doi:10.4161/auto.7.7.15454
Noormohammadi, A., Khodakarami, A., Gutierrez-Garcia, R., Lee, H. J., Koyuncu, S., König, T., et al. (2016). Somatic increase of CCT8 mimics proteostasis of human pluripotent stem cells and extends C. elegans lifespan. Nat. Commun. 7, 13649. doi:10.1038/ncomms13649
Porsteinsson, A. P., Isaacson, R. S., Knox, S., Sabbagh, M. N., and Rubino, I. (2021). Diagnosis of early alzheimer's disease: Clinical practice in 2021. J. Prev. Alzheimers Dis. 8 (3), 371–386. doi:10.14283/jpad.2021.23
Qin, Q., Yin, Y., Wang, Y., Lu, Y., Tang, Y., and Jia, J. (2020). Gene mutations associated with early onset familial Alzheimer's disease in China: An overview and current status. Mol. Genet. Genomic Med. 8 (10), e1443. doi:10.1002/mgg3.1443
Reddy, P. H., and Oliver, D. M. (2019). Amyloid beta and phosphorylated tau-induced defective autophagy and mitophagy in alzheimer's disease. Cells 8 (5), 488. doi:10.3390/cells8050488
Ritchie, M. E., Phipson, B., Wu, D., Hu, Y., Law, C. W., Shi, W., et al. (2015). Limma powers differential expression analyses for RNA-sequencing and microarray studies. Nucleic Acids Res. 43 (7), e47. doi:10.1093/nar/gkv007
Roca-Agujetas, V., de Dios, C., Abadin, X., and Colell, A. (2021). Upregulation of brain cholesterol levels inhibits mitophagy in Alzheimer disease. Autophagy 17 (6), 1555–1557. doi:10.1080/15548627.2021.1920814
Sherman, B. T., Hao, M., Qiu, J., Jiao, X., Baseler, M. W., Lane, H. C., et al. (2022). David: A web server for functional enrichment analysis and functional annotation of gene lists (2021 update). Nucleic acids Res., 194. doi:10.1093/nar/gkac194
Shoshan-Barmatz, V., Nahon-Crystal, E., Shteinfer-Kuzmine, A., and Gupta, R. (2018). VDAC1, mitochondrial dysfunction, and Alzheimer's disease. Pharmacol. Res. 131, 87–101. doi:10.1016/j.phrs.2018.03.010
Skaaraas, G., Melbye, C., Puchades, M. A., Leung, D., Jacobsen, Ø., Rao, S. B., et al. (2021). Cerebral amyloid angiopathy in a mouse model of alzheimer's disease associates with upregulated angiopoietin and downregulated hypoxia-inducible factor. J. Alzheimers Dis. 83 (4), 1651–1663. doi:10.3233/JAD-210571
Subramanian, A., Tamayo, P., Mootha, V. K., Mukherjee, S., Ebert, B. L., Gillette, M. A., et al. (2005). Gene set enrichment analysis: A knowledge-based approach for interpreting genome-wide expression profiles. Proc. Natl. Acad. Sci. U. S. A. 102 (43), 15545–15550. doi:10.1073/pnas.0506580102
Szklarczyk, D., Gable, A. L., Nastou, K. C., Lyon, D., Kirsch, R., Pyysalo, S., et al. (2021). The STRING database in 2021: Customizable protein-protein networks, and functional characterization of user-uploaded gene/measurement sets. Nucleic Acids Res. 49 (D1), D605–D612. doi:10.1093/nar/gkaa1074
Tibshirani, R. (1997). The lasso method for variable selection in the Cox model. Stat. Med. 16 (4), 385–395. doi:10.1002/(sici)1097-0258(19970228)16:4<385:aid-sim380>3.0.co;2-3
Vagnucci, A. H., and Li, W. W. (2003). Alzheimer's disease and angiogenesis. Lancet (London, Engl. 361 (9357), 605–608. doi:10.1016/S0140-6736(03)12521-4
Vaillant-Beuchot, L., Mary, A., Pardossi-Piquard, R., Bourgeois, A., Lauritzen, I., Eysert, F., et al. (2021). Accumulation of amyloid precursor protein C-terminal fragments triggers mitochondrial structure, function, and mitophagy defects in Alzheimer's disease models and human brains. Acta Neuropathol. 141 (1), 39–65. doi:10.1007/s00401-020-02234-7
Wang, Y., Wang, Y., Li, J., Hua, L., Han, B., Zhang, Y., et al. (2016). Effects of caffeic acid on learning deficits in a model of Alzheimer's disease. Int. J. Mol. Med. 38 (3), 869–875. doi:10.3892/ijmm.2016.2683
Wu, T., Hu, E., Xu, S., Chen, M., Guo, P., Dai, Z., et al. (2021). clusterProfiler 4.0: A universal enrichment tool for interpreting omics data. Innovation. 2 (3), 100141. doi:10.1016/j.xinn.2021.100141
Xie, C., Zhuang, X. X., Niu, Z., Ai, R., Lautrup, S., Zheng, S., et al. (2022). Amelioration of Alzheimer's disease pathology by mitophagy inducers identified via machine learning and a cross-species workflow. Nat. Biomed. Eng. 6 (1), 76–93. doi:10.1038/s41551-021-00819-5
Xie, Z., Bailey, A., Kuleshov, M. V., Clarke, D. J. B., Evangelista, J. E., Jenkins, S. L., et al. (2021). Gene set knowledge discovery with Enrichr. Curr. Protoc. 1 (3), e90. doi:10.1002/cpz1.90
Xu, M., Zhang, D. F., Luo, R., Wu, Y., Zhou, H., Kong, L. L., et al. (2018). A systematic integrated analysis of brain expression profiles reveals YAP1 and other prioritized hub genes as important upstream regulators in Alzheimer's disease. Alzheimers Dement. 14 (2), 215–229. doi:10.1016/j.jalz.2017.08.012
Yuan, O., Lin, C., Wagner, J., Archard, J. A., Deng, P., Halmai, J., et al. (2019). Exosomes derived from human primed mesenchymal stem cells induce mitosis and potentiate growth factor secretion. Stem Cells Dev. 28 (6), 398–409. doi:10.1089/scd.2018.0200
Zare-Shahabadi, A., Masliah, E., Johnson, G. V., and Rezaei, N. (2015). Autophagy in Alzheimer's disease. Rev. Neurosci. 26 (4), 385–395. doi:10.1515/revneuro-2014-0076
Zellner, S., Schifferer, M., and Behrends, C. (2021). Systematically defining selective autophagy receptor-specific cargo using autophagosome content profiling. Mol. Cell. 81 (6), 1337–1354.e8. e8. doi:10.1016/j.molcel.2021.01.009
Zhang, D. F., Fan, Y., Xu, M., Wang, G., Wang, D., Li, J., et al. (2019). Complement C7 is a novel risk gene for Alzheimer's disease in Han Chinese. Natl. Sci. Rev. 6, 257–274. doi:10.1093/nsr/nwy127
Zhang, S., Wu, D., Xu, Q., You, L., Zhu, J., Wang, J., et al. (2021). The protective effect and potential mechanism of NRXN1 on learning and memory in ADHD rat models. Exp. Neurol. 344, 113806. doi:10.1016/j.expneurol.2021.113806
Zhang, Z., and Klionsky, D. J. (2022). CCT2, a newly identified aggrephagy receptor in mammals, specifically mediates the autophagic clearance of solid protein aggregates. Autophagy 18 (7), 1483–1485. doi:10.1080/15548627.2022.2083305
Zhou, Z., Bai, J., Zhong, S., Zhang, R., Kang, K., Zhang, X., et al. (2021). Downregulation of ATP6V1A involved in alzheimer's disease via synaptic vesicle cycle, phagosome, and oxidative phosphorylation. Oxid. Med. Cell. Longev. 2021, 5555634. doi:10.1155/2021/5555634
Zhu, Z., Yang, C., Iyaswamy, A., Krishnamoorthi, S., Sreenivasmurthy, S. G., Liu, J., et al. (2019). Balancing mTOR signaling and autophagy in the treatment of Parkinson’s disease. Int. J. Mol. Sci. 20 (3), 728. doi:10.3390/ijms20030728
Keywords: alzheimer’s disease, autophagy, CCT2, microRNA, logistic model
Citation: Ma X, Feng Y, Quan X, Geng B, Li G, Fu X and Zeng L (2023) Multi-omics analysis revealed the role of CCT2 in the induction of autophagy in Alzheimer’s disease. Front. Genet. 13:967730. doi: 10.3389/fgene.2022.967730
Received: 13 June 2022; Accepted: 07 December 2022;
Published: 10 January 2023.
Edited by:
Clévia Rosset, Clinical Hospital of Porto Alegre, BrazilReviewed by:
Yizhuo Wang, University of Texas MD Anderson Cancer Center, United StatesCopyright © 2023 Ma, Feng, Quan, Geng, Li, Fu and Zeng. This is an open-access article distributed under the terms of the Creative Commons Attribution License (CC BY). The use, distribution or reproduction in other forums is permitted, provided the original author(s) and the copyright owner(s) are credited and that the original publication in this journal is cited, in accordance with accepted academic practice. No use, distribution or reproduction is permitted which does not comply with these terms.
*Correspondence: Linlin Zeng, emVuZ2xpbmxpbkBqbHUuZWR1LmNu
Disclaimer: All claims expressed in this article are solely those of the authors and do not necessarily represent those of their affiliated organizations, or those of the publisher, the editors and the reviewers. Any product that may be evaluated in this article or claim that may be made by its manufacturer is not guaranteed or endorsed by the publisher.
Research integrity at Frontiers
Learn more about the work of our research integrity team to safeguard the quality of each article we publish.