- 1Department of Plant Breeding and Genetics, Punjab Agricultural University, Ludhiana, Punjab, India
- 2Center of Excellence in Genomics and Systems Biology, International Crops Research Institute for the Semi-Arid Tropics (ICRISAT), Hyderabad, India
- 3Regional Research Station, Punjab Agricultural University, Faridkot, India
- 4Division of Genetics, ICAR-Indian Agricultural Research Institute, New Delhi, India
- 5Crop Improvement Division, ICAR- Indian Institute of Pulses Research, Kanpur, India
- 6International Center for Agricultural Research in the Dry Areas (ICARDA), Rabat Office, Rabat, Morocco
- 7Murdoch’s Centre for Crop and Food Innovation, State Agricultural Biotechnology Centre, Food Futures Institute, Murdoch University, Murdoch, WA, Australia
Chickpea yield is severely affected by drought stress, which is a complex quantitative trait regulated by multiple small-effect genes. Identifying genomic regions associated with drought tolerance component traits may increase our understanding of drought tolerance mechanisms and assist in the development of drought-tolerant varieties. Here, a total of 187 F8 recombinant inbred lines (RILs) developed from an interspecific cross between drought-tolerant genotype GPF 2 (Cicer arietinum) and drought-sensitive accession ILWC 292 (C. reticulatum) were evaluated to identify quantitative trait loci (QTLs) associated with drought tolerance component traits. A total of 21 traits, including 12 morpho-physiological traits and nine root-related traits, were studied under rainfed and irrigated conditions. Composite interval mapping identified 31 QTLs at Ludhiana and 23 QTLs at Faridkot locations for morphological and physiological traits, and seven QTLs were identified for root-related traits. QTL analysis identified eight consensus QTLs for six traits and five QTL clusters containing QTLs for multiple traits on linkage groups CaLG04 and CaLG06. The identified major QTLs and genomic regions associated with drought tolerance component traits can be introgressed into elite cultivars using genomics-assisted breeding to enhance drought tolerance in chickpea.
Introduction
Chickpea (C. arietinum L.) is an important cool-season legume crop cultivated largely in the semi-arid and arid regions of Asia and sub-Saharan Africa (Gaur et al., 2012). It is a diploid (2n = 2x = 16) and self-pollinated crop with a genome size of ∼738 Mb (Varshney et al., 2013a) and serves as a rich source of nutrients such as proteins (23%), carbohydrates (40%), vitamins, essential amino acids, and free from anti-nutritional factors (Jukanti et al., 2012; Roorkiwal et al., 2021). In spite of its economic importance and its role in improving human health, chickpea production is falling short of meeting the dietary needs of the burgeoning human population, mainly because of low productivity due to biotic and abiotic constraints (Thudi et al., 2014; Roorkiwal et al., 2020; Kushwah et al., 2021a). Among the abiotic stresses, drought alone causes up to 60% of annual yield losses in chickpea (Sabaghpour et al., 2006; Toker et al., 2007; Varshney et al., 2010; Hajjarpoor et al., 2018; Barmukh et al., 2022). The impact of global warming and climate change has emphasized researchers’ need to study the effect of drought stress on crop development and yield. Thus, it has become imperative to develop cultivars, which can attain their maximum potential under drought stress or rainfed environments.
Usually, drought stress adversely affects the plants through a transient or terminal drought (Li et al., 2018; Varshney et al., 2021). In general, it is a terminal drought that can terminate or reduce the reproductive phase to drastically reduce the crop yield. Drought tolerance is a complex quantitative trait affected by significant genotype by environment (G × E) interactions (Kashiwagi et al., 2008; Varshney et al., 2014; Barmukh et al., 2022), which hampers direct selection of genotypes with higher yield under drought conditions. Drought stress is well-known for reducing crop growth, thus affecting yield components, such as total biomass, pod number, seed number, seed weight, seed quality, and yield per plant (Toker et al., 2007; Krishnamurthy et al., 2013). Understanding the genetic basis of drought tolerance is difficult due to multiple underlying mechanisms used by plants to survive, such as drought escape, drought avoidance, and drought tolerance (Tuberosa & Salvi, 2006). The close association between several morphological and physiological traits (e.g., crop growth rate, leaf area index, canopy temperature depression, shoot biomass, phenology, etc.) with grain yield under drought was revealed in a previous study (Purushothaman et al., 2016). Early flowering in chickpea can be advantageous for enhancing the seed yield by shortening the vegetative phase and completing the crop life cycle prior to the onset of terminal drought stress (Kushwah et al., 2020b). Several other physiological traits, such as membrane permeability index, photosynthetic efficiency, relative leaf water content, chlorophyll content, proline accumulation, and ABA content, and morphological traits, such as days to germination, days to flowering, plant height, biomass, and 100-seed weight, have been proposed for the selection of drought-tolerant chickpea genotypes (Gaur et al., 2008; Kashiwagi et al., 2013; Purushothaman et al., 2016; Maqbool et al., 2017).
To further understand the drought tolerance mechanisms, screening of chickpea germplasm has led to the selection of genotypes with extensive root systems and better productivity under drought stress (Kashiwagi et al., 2005). Phenotypic attributes of the root system have gained more importance as they are expected to have a direct effect on transpiration in plants under drought stress (Ye et al., 2018). A profuse root system is expected to extract more soil water than a less extensive root system under drought stress (Zaman-Allah et al., 2011). Detailed studies on various root traits are difficult due to low heritability and complex mechanisms of these traits, variable expression across diverse soil environments, and the labor-intensive nature of the studies (Gaur et al., 2008; Varshney et al., 2011). Association studies have revealed positive associations (Kell, 2011; Bishopp & Lynch, 2015) and negative or neutral associations (Zaman-Allah et al., 2011; Schoppach et al., 2013) of profuse root systems with grain yield.
Considering the challenges associated with breeding drought-tolerant varieties, the identification of quantitative trait loci (QTLs) for drought tolerance component traits can be a judicious approach in a chickpea breeding program. For instance, QTLs identified in previous studies have helped in dissecting the genetic basis of drought tolerance-related traits in chickpea (Varshney et al., 2014; Sivasakthi et al., 2018). Furthermore, during the last decade, chickpea researchers have deciphered the chickpea genome (Varshney et al., 2013a; Jain et al., 2013) and developed several genomic (Roorkiwal et al., 2017; Roorkiwal et al., 2020) and transcriptomic resources (Hiremath et al., 2011; Kudapa et al., 2014; Mashaki et al., 2018) that have transformed chickpea from an “orphan crop” to a “genomics resource-rich crop” for faster genetic gains in chickpea (Varshney, 2016). Rapid advances in next-generation sequencing technologies have enabled the use of sequencing-based genotyping platforms for unraveling the genetic basis of several complex traits. The double digestion restriction site-associated DNA sequencing (ddRAD-seq) approach, developed by Peterson et al. (2012), can adjust the number of fragments by utilizing two different restriction enzymes (Puritz et al., 2014) and exclusively uses size selection for recovering the appropriate number of regions, which are arbitrarily distributed throughout the genome and maximizes the ability of multiplexing of numerous samples.
Due to the comparatively narrow genetic base of chickpea (Stephens et al., 2014) and relatively low levels of polymorphism, interspecific RIL populations from C. arietinum and C. reticulatum have been used efficiently for genetic studies (Singh et al., 2008; Barmukh et al., 2021a). The amount of polymorphism varied from 16% to 36% in an interspecific mapping population compared to only 9.5% in an intraspecific mapping population (Nayak et al., 2010). Interspecific populations have been proven to be useful for the construction of high-density genetic maps as well as trait dissection (Thudi et al., 2011; Roorkiwal et al., 2017; Barmukh et al., 2021a).
In the present study, an interspecific mapping population derived from a cross between GPF 2 (C. arietinum) and ILWC 292 (C. reticulatum) was used to identify key genomic regions associated with drought tolerance component traits. Some promising RILs were also detected for yield, morpho-physiological, and root-related traits under rainfed conditions for use in breeding programs. Identification and development of markers for drought tolerance component traits will be useful for deploying genomics-assisted breeding for the development of drought-tolerant improved chickpea varieties.
Materials and methods
Plant material and experimental sites
A set of 187 RILs (F8 generation) segregating for drought tolerance component traits was developed from an interspecific cross of drought-tolerant genotype GPF 2 (C. arietinum) with drought-sensitive accession ILWC 292 (C. reticulatum) using a single seed descent method as described in Kushwah et al. (2021b). Chickpea cultivar GPF2 is a semi-erect, medium-tall cultivar recommended for cultivation in Punjab state and in the North-Western Plains Zone of India. Apart from its drought tolerance characteristics, GPF2 is also resistant to Fusarium wilt and Ascochyta blight (Kushwah et al., 2021c). The accession ILWC 292 (C. reticulatum) has a semi-prostrate growth habit. It is sensitive to drought and susceptible to Ascochyta blight but resistant to botrytis grey mold and chickpea cyst nematode. Although ILWC 292 is a drought-sensitive genotype, it possesses some desirable drought tolerance-related traits such as higher root length density, root to shoot ratio, and membrane permeability index (Singh et al., 1996).
The RIL population and the parental genotypes were grown during the 2017–18 crop season, in an alpha lattice design (17 × 12) under irrigated (non-stress) and rainfed (drought-stress) conditions at two locations in India (Ludhiana and Faridkot), with three replications. Each RIL was planted in 2 m long paired rows with 30 cm × 10 cm spacing. The Ludhiana (30.9010°N, 75.8573°E) and Faridkot (30.6769°N, 74.7583°E) sites are categorized as semi-arid sub-tropical and semi-arid dry regions, respectively. Both sites consist of loamy sand with 59.8% sand and 16.5% clay (Typic Ustorthents). The average annual rainfall is 700 mm at Ludhiana and 450 mm at Faridkot, of which more than 70% occurs from July to September (Yadav et al., 2007; Kang et al., 2009).
Phenotyping for morphological and physiological traits under field conditions
Sowing under field conditions was performed during November–April of the 2017–18 crop season on the residual soil moisture, which was sufficient for good germination, as recommended for chickpea sowing in this region. Soil moisture was measured at the time of sowing, after 70, 90, 110, and 130 days of sowing, and at the time of maturity in irrigated and rainfed conditions at both the locations. In the case of rainfed plots, the soil moisture was ideal for drought conditions for chickpea crop. As a result of the drastic reduction in soil moisture content at 90, 110, and 130 days after sowing in rainfed plots as compared to irrigated plots, sufficient drought stress was induced at the reproductive (flowering and pod formation) and pod development stages at both the locations.
Phenotypic data were collected for 21 traits including 12 morphological and physiological traits, namely, days to germination (DG), days to flowering initiation (DFI), days to 50% flowering (DFF), days to 100% flowering (DHF), plant height (PH), number of pods per plant (NPP), biomass (BIO), yield (YLD), 100-seed weight (HSW), harvest index (HI), membrane permeability index (MPI), relative leaf water content (RLWC), and nine root-related traits. Five randomly selected plants were used to record observations on PH, NPP, BIO, and YLD in each plot. The data for DG, DFI, DFF, DHF, and HSW were recorded on a plot basis. HI was calculated as follows:
MPI was determined according to the method of Premchandra et al. (1990), and modified by Sairam (1994), as follows:
where C1 is the initial electrical conductivity at 40°C and C2 is the final electrical conductivity at 100°C.
RLWC was calculated using the formula (Slavik, 1974):
where FW is the fresh weight, DW is the dry weight, and TW is the turgid weight.
Phenotyping for root-related traits
A total of nine traits, i.e., root length (RL), shoot length (SL), root to shoot ratio (RSR), root length density (RLD), fresh root weight (FRW), fresh shoot weight (FSW), root dry weight (RDW), shoot dry weight (SDW), and the ratio of root dry weight to total plant dry weight (RDW/TDW) were recorded in the present study. A polyvinyl chloride (PVC) pipe-based cylinder culture approach was used for phenotyping root-related traits. Chickpea plants were grown in PVC cylinders (18 cm in diameter and 120 cm in height) with three replications. The PVC cylinders, except for the top 15 cm, were filled with an equi-mixture (w/w) of vertisol and sand, mixed with 0.07 g·kg−1 diammonium phosphate. The soil water content of the mixture was equilibrated to 70% field capacity to create conditions similar to those in the field at sowing when the soil is not fully saturated with water. A mixture of soil and sand was used to decrease the soil bulk density and facilitate root growth and extraction. Sampling was carried out at 35 days after sowing (DAS), avoiding physically damaged plants. This is because maximum variations in root-related traits among genotypes were best detected at 35 DAS and variation decreased after 41 DAS (Krishnamurthy et al., 1996).
Root samples were collected using steel soil-coring tubes (50 mm in diameter) to a depth of 120 cm at the flowering stage. Each RIL sample comprised three soil cores, which were pooled to increase the sample size. The soil cores for each sample were soaked overnight in water, and the roots were recovered by passing the suspension through a 2 mm wire mesh sieve. Chickpea roots were separated manually from the debris and weed roots. Total RL and FRW were measured, and roots were then oven-dried at 70°C for 72 h before measuring RDW. Likewise, FSW was measured, and then shoots were oven-dried at 70°C before measuring SDW. The RLD (cm−3) was measured as root length (cm)/volume of soil core (cm3), while the root to shoot ratio (RSR) was calculated using root and shoot lengths.
Statistical analysis
Analysis of variance (ANOVA) was computed for individual environments using mixed model analysis to estimate the contribution made by each factor to the total variation using SAS software version 9.3 (SAS Institute, Cary, NC). The data from irrigated and rainfed conditions were used to estimate the best linear unbiased predictors (BLUPs) using the residual maximum likelihood algorithm (ReML) in the R package lmer (Bates et al., 2015). BLUPs were estimated for all 21 morpho-physiological traits, and scatter plots were drawn for all the traits using BLUPs to find the correlation between the two locations evaluated, i.e., Ludhiana and Faridkot.
QTL analysis
The RIL population was genotyped with ddRAD-seq (Peterson et al., 2012), which uses PstI and MspI restriction enzymes (Thermo Fisher Scientific, MA, United States). The ddRAD-seq data analysis of RILs for SNP discovery and development of linkage map was performed as described previously (Kushwah et al., 2021c).
QTL analysis was performed with the composite interval mapping (CIM) method executed in the Windows QTL Cartographer V2.5 software package (Wang et al., 2007). The CIM analysis was conducted using forward and backward stepwise regression. For each trait, experiment-wise significance thresholds (p ≤ 0.05) were determined with 1,000 permutations for QTL detection. The position of the QTLs was identified on the basis of its logarithm of odds (LOD) peak location with a 95% confidence interval. The percentage of phenotypic variance and additive effect described by QTLs was also estimated. QTLs explaining total phenotypic variance (PVE) >10% were classified as major-effect QTLs, whereas those with PVE <10% were regarded as minor-effect QTLs (Varshney et al., 2014). The phenotypic contribution (R2) was estimated as the percentage of variance explained by each QTL in proportion to the total phenotypic variance, while the additive effect was estimated to find the positive or negative effect for the respective trait.
Results
Phenotypic variation among the RILs and parental genotypes
The RILs along with parent genotypes were evaluated under irrigated (non-stress) and rainfed (drought-stress) conditions at Ludhiana and Faridkot locations in India. Significant variation was observed among the RILs and their parents for multiple morphological, physiological, and root-related traits under irrigated and rainfed conditions (Tables 1, 2). Phenotyping data analysis for all the traits in parental lines depicted highly significant differences under irrigated and rainfed conditions. All of the measured morphological, physiological, and root-related traits were significantly affected by drought stress, except for HI, RL, RLD, and FRW. Under rainfed conditions, the RILs had slightly higher mean values (8.68%) for DG, while values for DFI, DFF, and DHF decreased by 16.11, 14.48, and 13.76%, respectively, when compared to irrigated conditions. Similarly, RILs possessed significantly lower mean values for PH (30.91%), NPP (42.50%), BIO (40.15%), and YLD (44.18%) under rainfed conditions. A moderate reduction in the mean performance of HSW (18.28%) and RLWC (19.53%) was observed under rainfed conditions relative to irrigated conditions. The rainfed conditions significantly increased the mean values of RILs for MPI (16.34%), while HI was least affected by water deficit with only a 0.09% reduction, relative to the irrigated conditions (Table 1). In the case of root-related traits, water deficit significantly reduced the mean values of RILs for SL (13.93%), FSW (25.40%), and SDW (14.05%), and slightly reduced for FRW (0.82%), relative to the irrigated conditions. A significant increase in the mean performance of RILs for RSR (18.40%), a moderate increase for RDW (6.09%) and RRDWTDW (9.30%), and a slight increase for RL (1.39%) and RLD (0.21%) were observed under rainfed conditions relative to the irrigated conditions (Table 2).
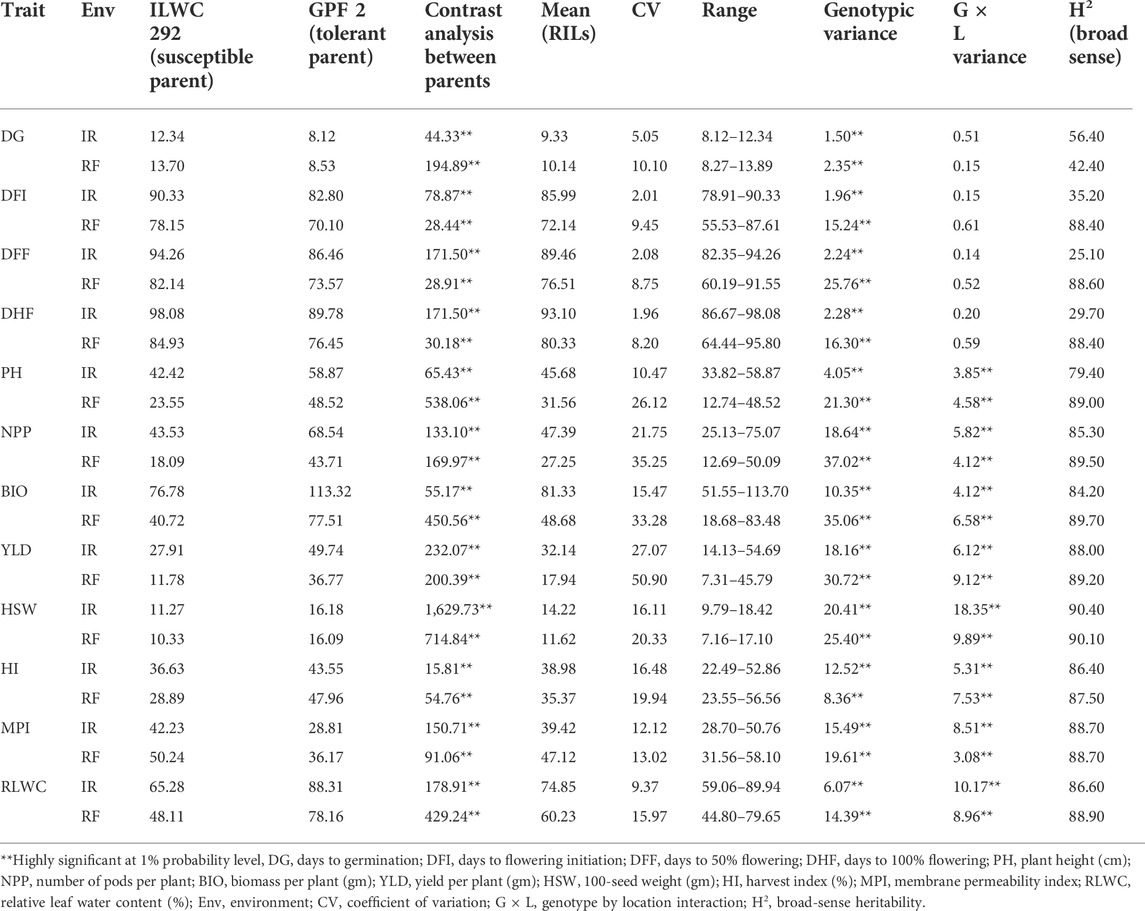
TABLE 1. Mean performance of chickpea RIL population for various morphological and physiological traits under irrigated (IR) and rainfed (RF) conditions (Ludhiana and Faridkot pooled).
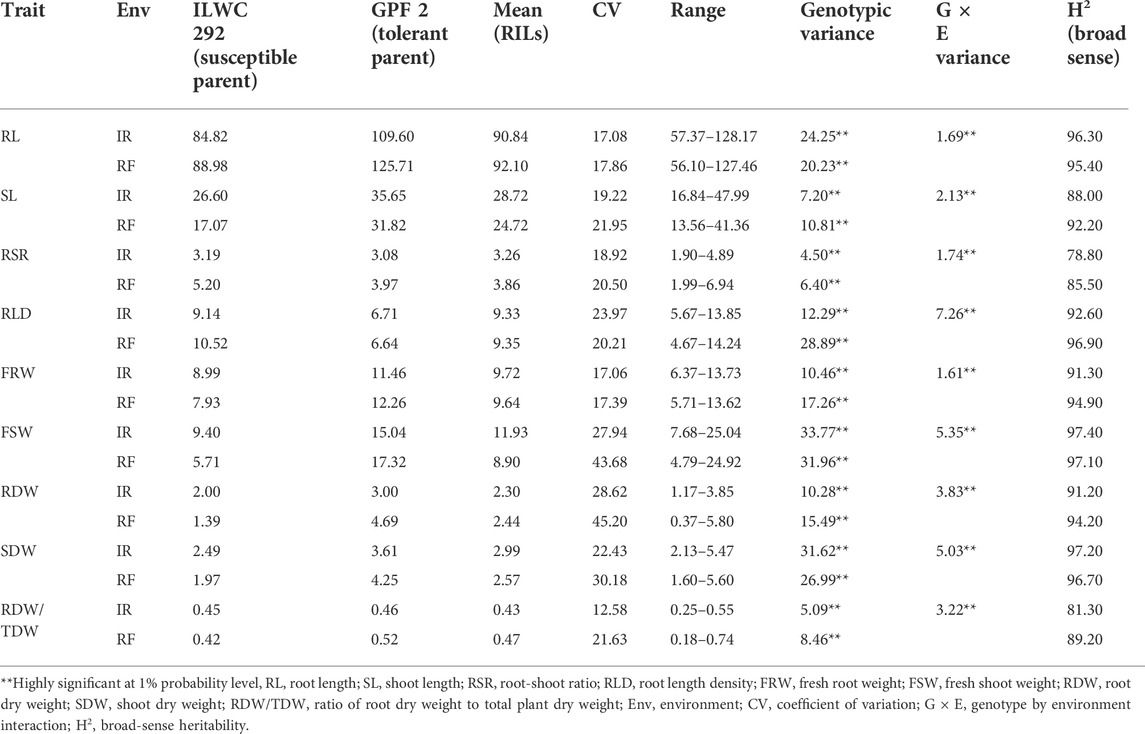
TABLE 2. Mean performance of chickpea RIL population for root-related traits under irrigated (IR) and rainfed (RF) conditions.
The pooled ANOVA for all the morphological and physiological traits showed highly significant differences between genotypes at both locations in the irrigated and rainfed conditions. Significant differences were also observed for genotype × environment (G × E) interactions for these traits, except for DG, DFI, DFF, and DHF (Table 1). The scatter plots showed a highly significant relationship between locations for most of the traits except PH, HI, and RLWC which showed a moderately high correlation coefficient (Supplementary Figure S1). In the case of root-related traits, pooled ANOVA for variation in genotypes and G × E interactions showed highly significant differences for the RIL population under irrigated and rainfed conditions at both locations (Table 2). Under irrigated conditions, PH, NPP, BIO, YLD, HSW, HI, MPI, and RLWC had higher broad-sense heritability (79.40–90.40%), while low to moderate heritability (25.10–56.40%) was observed for DG, DFI, DFF, and DHF. By contrast, under rainfed conditions, all traits had high heritability (87.50–90.10%), except for DG (42.40%) (Table 1). The root-related traits had high broad-sense heritability under both irrigated (78.80–97.40%) and rainfed conditions (85.50–97.10%) (Table 2). A significantly high correlation coefficient between the irrigated and rainfed conditions was observed for all root-related traits, except RLD (Supplementary Figure S2).
Selection of promising lines
A total of 17 out of 187 RILs were found to be highly promising for yield, morpho-physiological, and root-related traits under rainfed conditions (Tables 3, 4). Of these 17 lines, five lines showing early flowering were also promising for yield-related traits (Table 3).
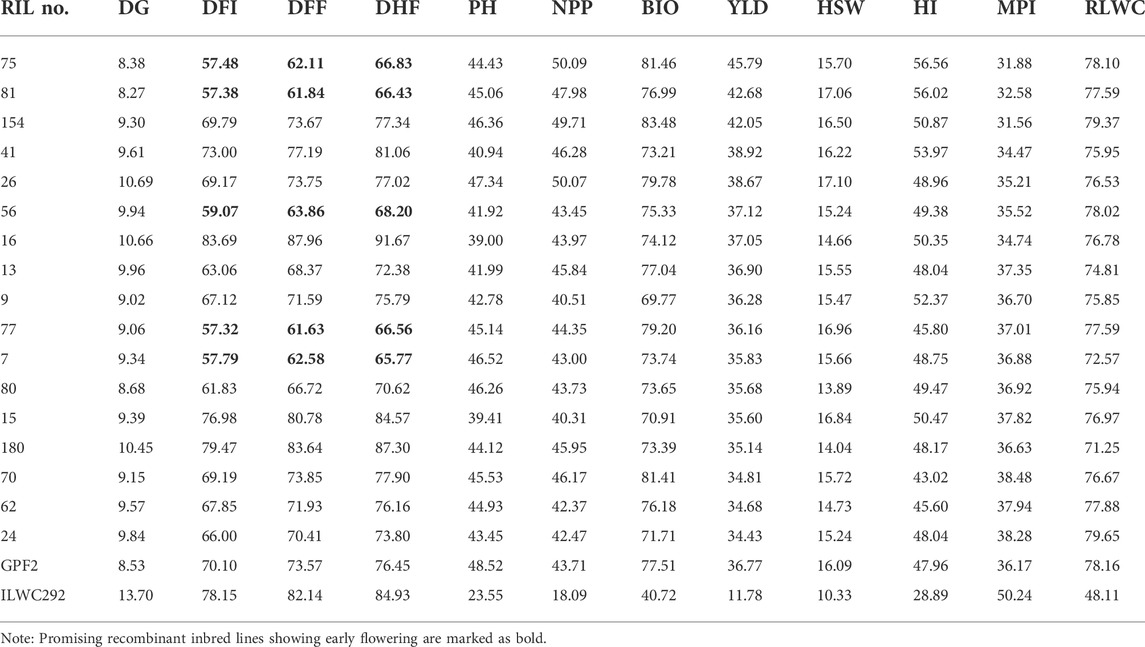
TABLE 3. List of promising recombinant inbred lines for various morphological and physiological traits under rainfed conditions (Ludhiana and Faridkot pooled).
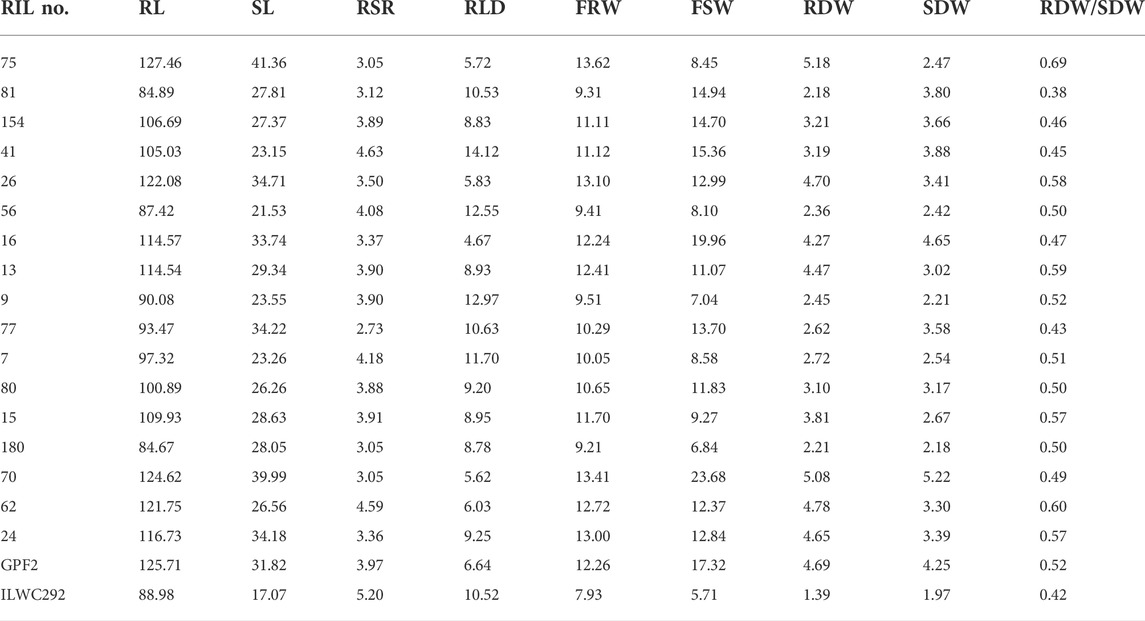
TABLE 4. List of promising recombinant inbred lines for various root-related traits under rainfed conditions at Ludhiana.
QTLs for drought tolerance component traits
A total of 1,365 polymorphic SNPs were used for linkage map construction (Kushwah et al., 2021c). Genotypic data on 1,365 informative SNPs, linkage map distances, and BLUP values for 21 traits evaluated at two locations were used to identify QTLs for drought tolerance component traits. A total of 31 QTLs at Ludhiana and 23 QTLs at Faridkot were identified for 12 morphological and physiological traits, except root-related traits, evaluated under irrigated and rainfed conditions (Tables 5, 6; Figure 1; Supplementary Figure S3). Out of 31 QTLs identified at Ludhiana, 14 were classified as major-effect QTLs and 17 as minor-effect QTLs. Likewise, out of 23 QTLs identified at Faridkot, 15 were major-effect QTLs and seven were minor-effect QTLs.
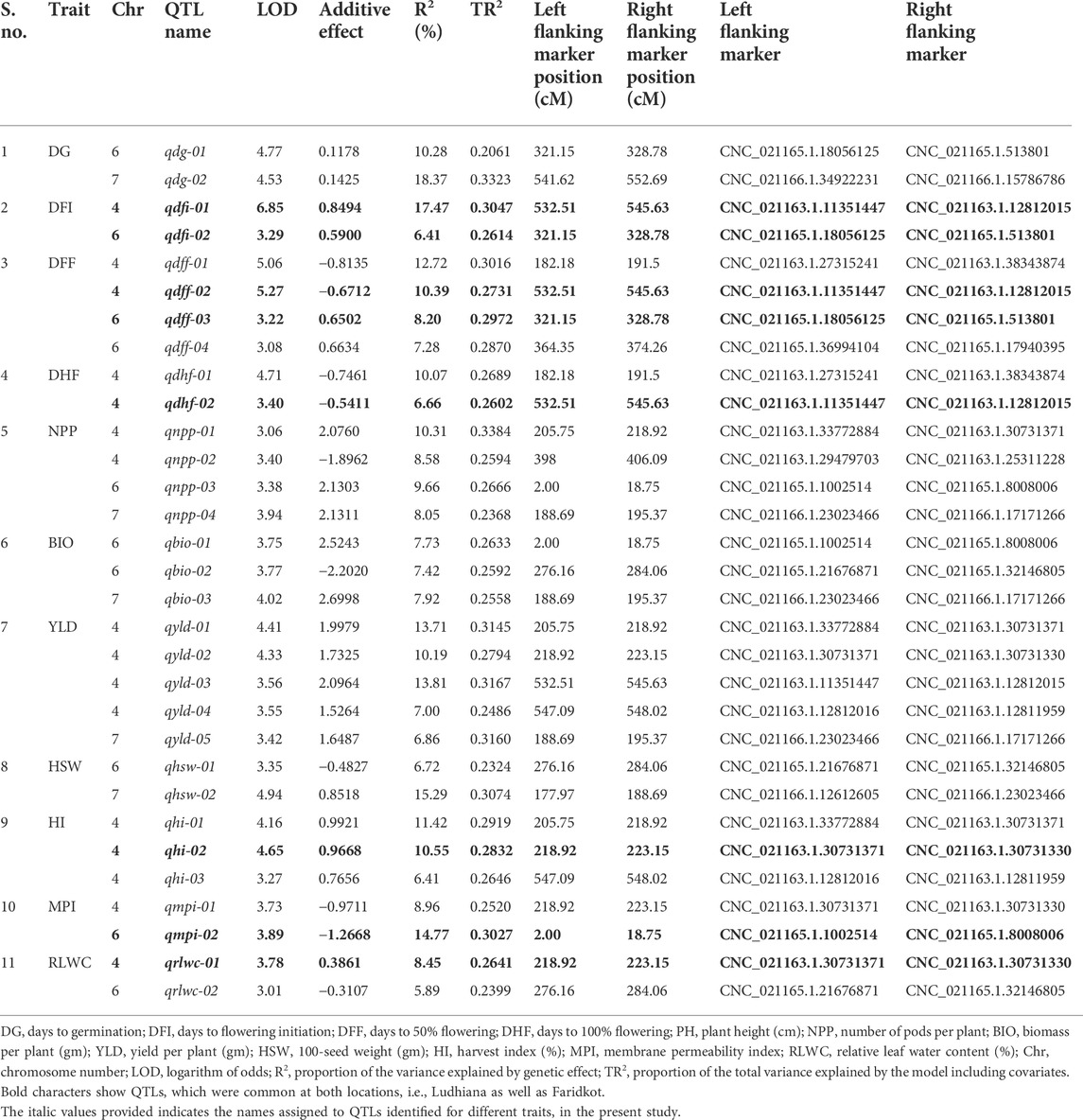
TABLE 5. Summary of QTLs associated various morphological and physiological traits evaluated at Ludhiana.
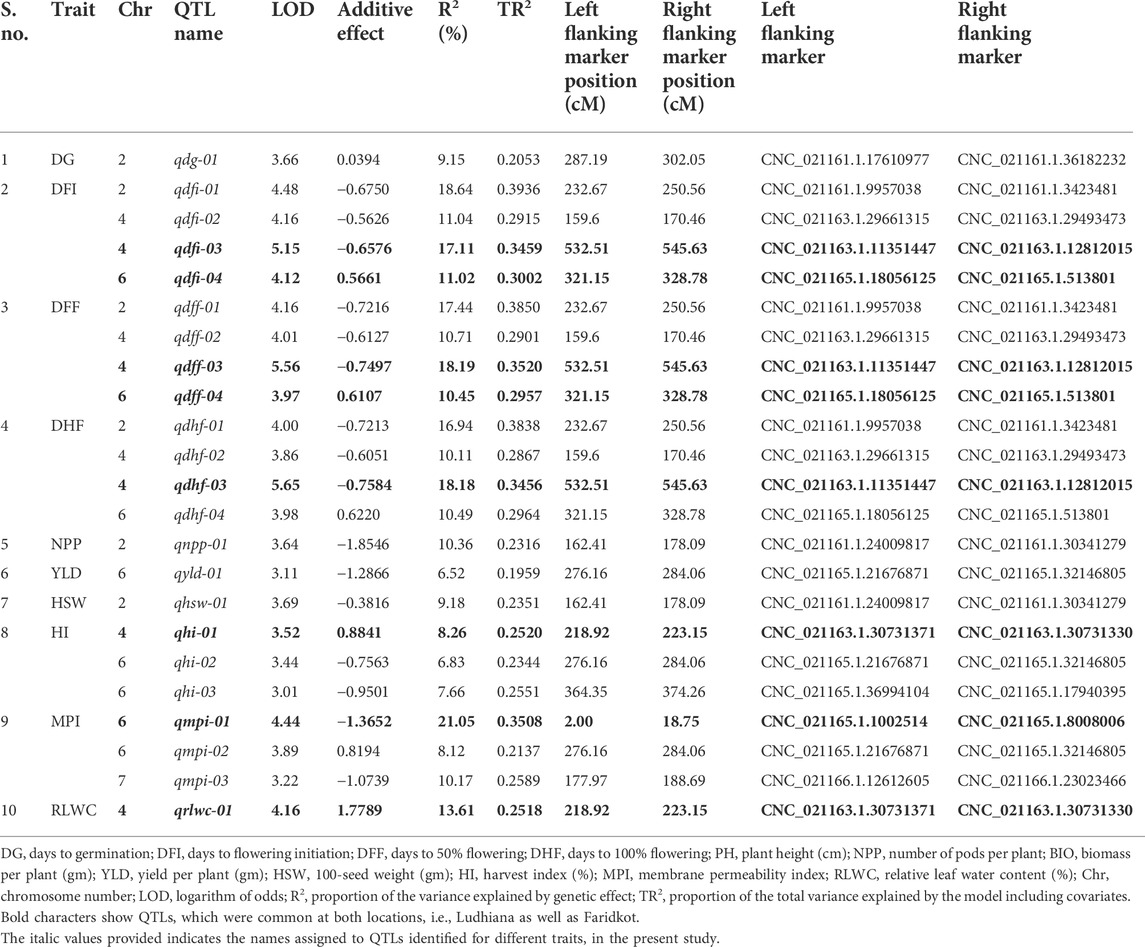
TABLE 6. Summary of QTLs associated with various morphological and physiological traits evaluated Faridkot.
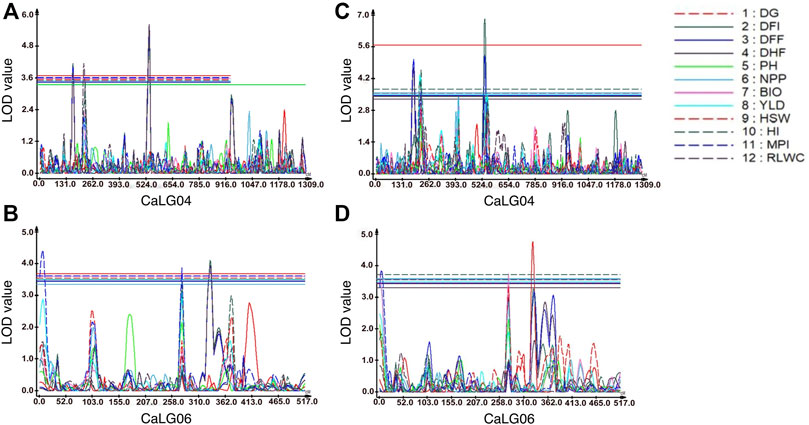
FIGURE 1. Genomic regions with QTLs for morphological and physiological traits. For traits evaluated at Faridkot, (A) QTLs for days to flowering initiation (DFI), days to 50% flowering (DFF), days to 100% flowering (DHF), harvest index (HI), and relative leaf water content (RLWC) were mapped on CaLG04; while (B) QTLs for days to flowering initiation (DFI), days to 50% flowering (DFF), days to 100% flowering (DHF), yield (YLD), harvest index (HI), and membrane permeability index (MPI) were mapped on CaLG06. For traits evaluated at Ludhiana, (C) QTLs for days to flowering initiation (DFI), days to 50% flowering (DFF), days to 100% flowering (DHF), yield (YLD), harvest index (HI), membrane permeability index (MPI), and relative leaf water content (RLWC) were mapped on CaLG04; whereas (D) QTLs for days to germination (DG), days to flowering initiation (DFI), days to 50% flowering (DFF), number of pods per plant (NPP), biomass per plant (BIO), 100-seed weight (HSW), membrane permeability index (MPI), and relative leaf water content (RLWC) were mapped on CaLG06.
A total of eight consensus QTLs were identified for DFI, DFF, DHF, HI, MPI, and RLWC at both the locations evaluated. Nine QTL clusters containing QTLs for DG, DFI, DFF, DHF, NPP, BIO, YLD, HSW, HI, MPI, and RLWC traits evaluated at Ludhiana were identified on linkage groups CaLG04, CaLG06, and CaLG07. Furthermore, seven QTL clusters were identified containing QTLs for DFI, DFF, DHF, NPP, YLD, HSW, HI, MPI, and RLWC traits evaluated at Faridkot on linkage groups CaLG02, CaLG04, and CaLG06. Four QTL clusters were identified on linkage groups CaLG04 and CaLG06 for traits evaluated at both the locations and contained QTLs for DG, DFI, DFF, DHF, BIO, YLD, HSW, HI, MPI, and RLWC traits. All of these QTLs were distributed on linkage groups CaLG02, CaLG04, CaLG06, and CaLG07, while linkage groups CaLG01, CaLG03, CaLG05, and CaLG08 did not contain any QTL for any location. Maximum QTLs were present on linkage group CaLG04 followed by linkage group CaLG06. The highest phenotypic variation was observed for DG (18.37%) at Ludhiana and MPI (21.05%) at Faridkot. The highest LOD value was observed for DFI (6.85) at Ludhiana and DFF (5.56) at Faridkot. The QTLs having positive or negative additive effects for a particular trait implied that an increase in the proportion of the phenotypic variation of that particular trait was contributed by the allele from GPF 2 or ILWC 292, respectively.
In the case of root-related traits, a total of seven QTLs were identified for four traits, namely, RSR, RLD, RDW, and RDW/TDW, evaluated under irrigated and rainfed conditions (Table 7; Figure 2; Supplementary Figure S4). Of these, two were major-effect QTLs and five were minor-effect QTLs. One QTL cluster located on linkage group CaLG04 for RDW also contained QTLs for YLD, HI, MPI, and RLWC on the same genomic position. A maximum number of QTLs (four QTLs) for root-related traits were observed for RSR on four different linkage groups. All of these QTLs linked with root-related traits were distributed on linkage groups CaLG02, CaLG04, CaLG05, CaLG06, and CaLG07, while linkage groups CaLG01, CaLG03, and CaLG08 harbored no QTL. The highest phenotypic variation was observed for RDW (11.56%), and the highest LOD value was observed for RLD (5.13).
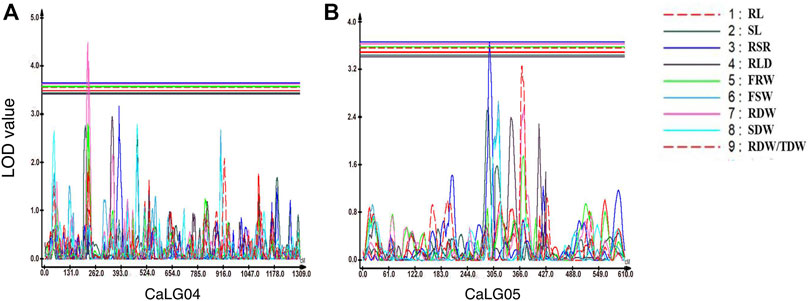
FIGURE 2. Genomic regions with major-effect QTLs for root-related traits. (A) QTLs for root to shoot ratio (RSR) and root dry weight (RDW) were mapped on CaLG04. (B) QTLs for root to shoot ratio (RSR) and the ratio of root dry weight to total plant dry weight (RDW/TDW) were mapped on CaLG05.
QTLs for phenological traits
In the case of phenological traits, two QTLs each were detected for DG, DFI, and DHF, while four QTLs were identified for DFF at Ludhiana, having PVE in the range of 6.41–18.37%. Likewise, one QTL for DG and four QTLs each for DFI, DFF, and DHF were identified at Faridkot, having 9.15–18.64% PVE.
QTLs for physiological traits
In the case of physiological traits, two QTLs each were identified for MPI and RLWC traits evaluated at Ludhiana, with a PVE of 5.89–14.71%. In addition, three QTLs were detected for MPI and one QTL for RLWC at Faridkot location and had PVE in the range of 8.12–21.05%.
QTLs for yield and yield-related traits
Yield and yield-related traits contained two QTLs for HSW, three QTLs each for BIO and HI, four QTLs for NPP, and five QTLs for YLD evaluated at Ludhiana, with a PVE ranging from 6.41% to 15.29%. In addition, one QTL each for NPP, HSW, and YLD and three QTLs for HI were identified for phenotypic data collected at Faridkot, which possessed PVE in the range of 6.52–10.36%.
QTLs for root-related traits
In the case of root-related traits, one QTL each for RLD, RDW, and RDW/TDW and four QTLs for RSR were identified, which had PVE ranging from 6.17% to 11.56%.
Discussion
Drought represents one of the most significant abiotic stresses that causes up to a 60% reduction in chickpea yields (Kumar et al., 2015; Hajjarpoor et al., 2018; Barmukh et al., 2022). Drought tolerance is highly influenced by several parameters such as days to germination, days to 50% flowering, days to maturity, biomass, and yield as morphological traits; transpiration efficiency, membrane permeability, and relative leaf water content as physiological traits; and root depth, root length density, root weight, and root to shoot ratio as root-related traits (Varshney et al., 2011). Due to these factors and unknown mechanisms underlying drought tolerance, molecular mapping for drought tolerance is complicated. Therefore, for genetic dissection of drought tolerance, evaluation of drought tolerance component traits and identification of molecular markers tightly linked to these traits will facilitate the selection of drought-tolerant genotypes and eventually introgression of these traits into elite cultivars through genomics-assisted breeding programs. Thus, it is imperative to study the complex nature of drought stress for identifying key genomic regions associated with drought tolerance component traits for the selection of drought-tolerant genotypes.
In this study, drought stress significantly affected all of the measured morphological, physiological, and root-related traits, except HI, RL, RLD, and FRW. A total of 17 lines were found to be promising for yield and yield-related traits as well as root-related traits under rainfed conditions. Out of these 17 lines, five lines showing early flowering and better yield contributing traits are being evaluated under multi-location trials in India. It has been found in earlier studies that traits such as early flowering, phenological plasticity, and a profuse and deep root system could be beneficial under drought stress (Saxena, 2003; Berger et al., 2006), which is in accordance with our results. Early flowering can be a good option as more pods are set before the occurrence of drought stress, and thus genotypes can escape drought stress (Kushwah et al., 2020b). Breeding programs for developing drought-tolerant genotypes in chickpea were focused on accelerating flowering and maturity to escape terminal drought stress (Upadhyaya et al., 2012). The primary adaptive strategy identified by several chickpea breeding programs for tolerance to terminal drought stress is drought escape via early flowering (Kumar & Abbo, 2001; Berger et al., 2006; Gaur et al., 2008; Purushothaman et al., 2014) in spite of the fact that selection pressure for drought tolerance and drought escape per se are different from each other (Berger et al., 2016). However, some previous studies have observed that early flowering is negatively correlated with seed yield under drought stress (Kumar et al., 2005; Yucel et al., 2006). Hence, there is a need to develop drought-tolerant, high-yielding chickpea genotypes with early maturity.
Previous studies show that root traits, such as root length density, root depth, and root dry weight, could be promising for improving drought tolerance in chickpea (Kashiwagi et al., 2006; Zaman-Allah et al., 2011; Purushothaman et al., 2017; Barmukh et al., 2022). The present study also showed that root length density was non-significantly affected by drought stress. Prolific root systems are likely to influence transpiration, biomass, and harvest index under drought conditions through the utilization of deep soil moisture (Zaman-Allah et al., 2011; Kashiwagi et al., 2013; Purushothaman et al., 2016). Despite the significance of prolific root systems for drought stress, only a few advances have been made in this direction, mainly because root studies are laborious and time consuming. Shoot and root vigor are reciprocally advantageous as the production of shoot biomass depends on the exploitation of soil moisture by the root system (Pinheiro et al., 2005; Purushothaman et al., 2017; Sivasakthi et al., 2018) and root vigor depends on the production of photo-assimilates by shoots (Wasson et al., 2014). This suggests that further improvements in root-related traits could advance drought stress tolerance in chickpeas as higher yields and harvest index can be attained with a strong root system. A high correlation coefficient was observed between the irrigated and rainfed conditions for all root-related traits except RLD. Our results are in accordance with a previous study (Zaman-Allah et al., 2011) in which RLD did not differ between sensitive and tolerant chickpea genotypes under drought stress and had no correlation with seed yield.
In the present study, the pooled ANOVA for all measured traits, including root-related traits, showed highly significant differences between genotypes under irrigated and rainfed conditions at both locations. Combined ANOVA in several studies also showed significant differences for various morphological and physiological traits (Hamwieh et al., 2013; Pang et al., 2017; Purushothaman et al., 2017; Sachdeva et al., 2018) and for root-related traits (Varshney et al., 2014; Purushothaman et al., 2017). Significant differences were also observed for genotype × environment (G × E) interactions for almost all the measured traits including root-related traits. Both the locations have almost similar trends of rainfall patterns under rainfed conditions with little differences. Thus, significant G × E interaction could be due to other factors other than the available soil moisture. To encounter these differences, BLUP values for genotypes for both the locations were also estimated taking location as a random effect. BLUP values of the RIL population for both locations showed high correlations with each, thus showing that these can be used for further QTL analysis to find consistent QTLs at both the locations. BLUPs were also estimated from phenotypic data across the years and locations by several researchers for statistical analysis and QTL mapping (Thudi et al., 2014; Li et al., 2018; Sivasakthi et al., 2018).
Due to polygenic control and high G × E interaction, quantitative traits like drought tolerance are complex in nature. Because of this, little progress could be made to breed cultivars harboring these traits through conventional breeding approaches. Thus, identifying the QTLs for complex drought tolerance component traits is an important requirement for understanding their genetic architecture and precise transfer in the background of elite cultivars. In the present study, QTLs having a positive additive effect indicated that the donor parent allele (GPF 2) contributed toward increasing the trait value; while those with a negative additive effect indicated that the recipient parent allele (ILWC 292) conferred a higher trait value. A total of 31 QTLs at Ludhiana and 23 QTLs at Faridkot were identified for the 12 morphological and physiological traits excluding root-related traits using BLUPs in the RIL population evaluated under irrigated and rainfed conditions. Out of these, eight consensus QTLs for DFI, DFF, DHF, HI, MPI, and RLWC were identified at both locations. QTLs responsible for early flowering have an advantage of more pod setting before the occurrence of drought stress due to a comparatively longer period of reproductive growth. Early flowering genotypes follow the mechanism of drought escape by reducing the duration of crop maturity under drought stress (Krishnamurthy et al., 2010; Purushothaman et al., 2016), which indicated that this trait is a useful selection criterion for drought tolerance. The consensus QTLs for DFI, DFF, and DHF harbor on linkage groups 4 and 6, suggesting that these loci confer flowering time in chickpea.
A total of three QTLs and two QTLs for seed yield were reported earlier by Jingade and Ravikumar (2015) and Rehman et al. (2011), respectively, on CaLG01. Likewise, one QTL on CaLG04 (Cobos et al., 2007) and four QTLs on linkage groups CaLG04, CaLG06, CaLG07, and CaLG08 (Verma et al., 2015) for seed yield were also identified. Some QTLs for seed yield were also mapped in the present study, which were located on linkage groups CaLG04, CaLG06, and CaLG07. Similarly, two QTLs on CaLG04 and CaLG08 (Cobos et al., 2009), two QTLs on CaLG06 and CaLG07 (Gupta et al., 2015), and one QTL on CaLG04 (Jamalabadi et al., 2013) for seed weight were identified. Recently, major-effect QTLs for seed weight were identified on CaLG06 and CaLG04 in chickpea (Barmukh et al., 2021b). In the present study, three QTLs were identified for seed weight on linkage groups CaLG02, CaLG06, and CaLG07. Several QTLs for plant height, number of pods per plant, 100-seed weight, biomass, harvest index, and yield were also identified in some previous studies (Gowda et al., 2011; Varshney et al., 2014) which were also at the same locus as identified in our study.
Solute leakage from the cell membrane is used to estimate the damage caused by drought stress (ElBasyoni et al., 2017). Drought-tolerant genotypes show less cell membrane damage, and thus, the membrane permeability index can be used as an effective selection criterion against drought stress. Likewise, RLWC indicates the equilibrium between water content in the leaf tissues and transpiration rate (Lugojan & Ciulca, 2011). Thus, RLWC is also a key indicator of water status present in plants and helps in the efficient selection of drought-tolerant genotypes. Consequently, QTLs representing genotypic differences in the RIL population for both MPI and RLWC traits can be used in genomics-assisted breeding programs for improving drought tolerance in chickpea.
While roots represent the first plant parts to be exposed to drought conditions in the soil, improvement of root-related traits holds the potential to enhance soil water extraction under drought scenarios. Breeding strategies should concentrate on the improvement of root-related traits for the efficient utilization of available soil water. In the present study, a total of seven QTLs were identified for four root-related traits, namely, RSR, RLD, RDW, and RDW/TDW, in the RIL population evaluated under irrigated and rainfed conditions. In the past, Varshney and colleagues (2014) identified robust main-effect QTLs for root traits such as root length density, root surface area, and root dry weight/total plant dry weight ratio, explaining up to 16.67% PVE. Furthermore, Thudi et al. (2014) identified 15 significant molecular markers closely associated with root length density, root dry weight, rooting depth, root surface area, and root volume. Due to the lack of precise and high-throughput phenotyping for root-related traits, breeding for the advancement of drought tolerance appears to be a difficult task. In the present study, molecular mapping of root-related traits will be helpful for the introgression of the genomic regions associated with root traits into elite cultivars by using genomics-assisted breeding approaches (Varshney et al., 2021).
Four QTL clusters were consistently identified at both Ludhiana and Faridkot locations, containing QTLs for DG, DFI, DFF, DHF, BIO, YLD, HSW, HI, MPI, and RLWC at the same genomic position on CaLG04 and CaLG06. One QTL cluster located on CaLG04 possessed QTLs for RDW, YLD, HI, MPI, and RLWC traits. Several genomic regions having co-localized/pleiotropic QTLs can be scrutinized to identify closely linked molecular markers, which will be helpful for introgressing this region into elite cultivars through marker-assisted breeding programs. Previously, Varshney et al. (2014) identified a total of nine QTL clusters for drought tolerance-related traits, out of which one major cluster on CaLG04 was referred to as a “QTL hotspot”. Importantly, the “QTL-hotspot” region was found to contain 13 major QTLs for 12 drought tolerance component traits and explained up to 58.20% PVE (Varshney et al., 2014). The estimated size of this “QTL hotspot” was refined from 29 cM to 14 cM using a genotyping-by-sequencing approach (Jaganathan et al., 2015) and then to ∼300 kb using a bin mapping approach (Kale et al., 2015). Notably, fine mapping of the “QTL-hotspot” region led to the identification of CaTIFY4b as the candidate gene regulating drought responses in chickpea (Barmukh et al., 2022). In the recent past, introgression of the “QTL-hotspot” region into different genetic backgrounds of elite cultivars led to the development and release of molecular breeding lines with enhanced drought tolerance (Varshney et al., 2013b; Bharadwaj et al., 2021). In a similar way, the QTL clusters identified in the present study can be targeted for introgression into elite cultivars for enhancing drought stress tolerance. These identified QTLs will serve as a potential tool for detecting candidate genes with the recent advances in genomics and transcriptomics resources in chickpea.
Data availability statement
The raw sequencing reads of RIL population and its parents has been deposited in NCBI-SRA and is accessible publically through the BioProject ID: PRJNA530673 and the submission ID: SUB5404800.
Author contributions
SS and RKV conceived the idea and provided critical inputs to the concept. AK, SB, SV, IS, and SS designed and conducted the experiments. AK, SB, SV, and GS performed the phenotyping. DB, AK, CB, and AP performed the data analysis. AK, DB, RB, MR, SK, SS, and RKV wrote the manuscript. All authors read and approved the manuscript.
Funding
RKV acknowledges the financial support from Bill and Melinda Gates Foundation through the Tropical Legumes III project. The INSPIRE research grant was provided to AK by the Department of Science and Technology (DST), New Delhi, India, and research grants were provided under the project “Incentivizing Research in Agriculture” and Consortia Research Platform on Molecular Breeding by the Indian Council of Agricultural Research, New Delhi to SS for carrying out the research are highly acknowledged. RB acknowledges funding support from the Council of Scientific and Industrial Research (CSIR), Government of India, for the award of a research fellowship.
Acknowledgments
The authors thank all cooperating partners and employees who contributed to this study.
Conflict of interest
The authors declare that the research was conducted in the absence of any commercial or financial relationships that could be construed as a potential conflict of interest.
Publisher’s note
All claims expressed in this article are solely those of the authors and do not necessarily represent those of their affiliated organizations, or those of the publisher, the editors, and the reviewers. Any product that may be evaluated in this article, or claim that may be made by its manufacturer, is not guaranteed or endorsed by the publisher.
Supplementary material
The Supplementary Material for this article can be found online at: https://www.frontiersin.org/articles/10.3389/fgene.2022.953898/full#supplementary-material
Supplementary Figure S1 | Scatter plots of drought tolerance-related traits showing a relationship between Ludhiana and Faridkot locations. FDK, Faridkot; LDH, Ludhiana.
Supplementary Figure S2 | Scatter plots of root-related traits showing a relationship between Ludhiana and Faridkot locations. FDK, Faridkot; LDH, Ludhiana.
Supplementary Figure S3 | Genomic regions with QTLs for morphological and physiological traits. For traits evaluated at Faridkot, (A) QTLs for days to germination (DG), days to flowering initiation (DFI), days to 50% flowering (DFF), days to 100% flowering (DHF), number of pods per plant (NPP), and 100-seed weight (HSW) were mapped on CaLG02; while (B) QTL for membrane permeability index (MPI) was mapped on CaLG07. (C) QTLs for days to germination (DG), number of pods per plant (NPP), biomass per plant (BIO), yield per plant (YLD), and 100-seed weight (HSW) were mapped on CaLG07 for Ludhiana.
Supplementary Figure S4 | Genomic regions with QTLs for root-related traits. QTLs for root to shoot ratio (RSR) mapped on (A) CaLG02 and (B) CaLG06. (C) QTL for root length density (RLD) mapped on CaLG07.
References
Barmukh, R., Roorkiwal, M., Garg, V., Khan, A. W., German, L., Jaganathan, D., et al. (2022). Genetic variation in CaTIFY4b contributes to drought adaptation in chickpea. Plant Biotechnol. J., 1–15. doi:10.1111/pbi.13840
Barmukh, R., Roorkiwal, M., Jaba, J., Chitikineni, A., Mishra, S., Sagurthi, S. R., et al. (2021b). Development of a dense genetic map and QTL analysis for pod borer Helicoverpa armigera (Hübner) resistance component traits in chickpea (Cicer arietinum L.). Plant Genome 14, e20071–48. doi:10.18637/jss.v067.i01
Barmukh, R., Soren, K. R., Madugula, P., Gangwar, P., Shanmugavadivel, P. S., Bharadwaj, C., et al. (2021a). Construction of a high-density genetic map and QTL analysis for yield, yield components and agronomic traits in chickpea (Cicer arietinum L.). PLoS ONE 16, e0251669. doi:10.1371/journal.pone.0251669
Bates, D., Mächler, M., Bolker, B., and Walker, S. (2015). Fitting linear mixed-effects models using lme4. J. Stat. Softw. 67, 1–48.
Berger, J. D., Ali, M., Basu, P. S., Chaudhary, B. D., Chaturvedi, S. K., Deshmukh, P. S., et al. (2006). Genotype by environment studies demonstrate the critical role of phenology in adaptation of chickpea (Cicer arietinum L.) to high and low yielding environments of India. Field Crops Res. 98, 230–244. doi:10.1016/j.fcr.2006.02.007
Berger, J., Palta, J., and Vadez, V. (2016). Review: An integrated framework for crop adaptation to dry environments: Responses to transient and terminal drought. Plant Sci. 253, 58–67. doi:10.1016/j.plantsci.2016.09.007
Bharadwaj, C., Tripathi, S., Soren, K. R., Thudi, M., Singh, R. K., Sheoran, S., et al. (2020). Introgression of “QTL-hotspot” region enhances drought tolerance and grain yield in three elite chickpea cultivars. Plant Genome 14, e20076. doi:10.1002/tpg2.20076
Bharadwaj, C., Tripathi, S., Soren, K. R., Thudi, M., Singh, R. K., Sheoran, S., et al. (2021). Introgression of "QTL-hotspot" region enhances drought tolerance and grain yield in three elite chickpea cultivars. Plant Genome 14, e20076.
Bishopp, A., and Lynch, J. P. (2015). The hidden half of crop yields. Nat. Plants 1, 15117. doi:10.1038/nplants.2015.117
Cobos, M. J., Rubio, J., Fernández-Romero, M. D., Garza, R., Moreno, M. T., Millian, T., et al. (2007). Genetic analysis of seed size, yield and days to flowering in a chickpea recombinant inbred line population derived from a Kabuli × Desi cross. Ann. Appl. Biol. 151, 33–42. doi:10.1111/j.1744-7348.2007.00152.x
Cobos, M. J., Winter, P., Kharrat, M., Cubero, J. I., Gil, J., Millan, T., et al. (2009). Genetic analysis of agronomic traits in a wide cross of chickpea. Field Crops Res. 111, 130–136. doi:10.1016/j.fcr.2008.11.006
ElBasyoni, I., Saadalla, M., Baenziger, S., Bockelman, H., and Morsy, S. (2017). Cell membrane stability and association mapping for drought and heat tolerance in a worldwide wheat collection. Sustainability 9, 1606. doi:10.3390/su9091606
Gaur, P. M., Jukanti, A. K., and Varshney, R. K. (2012). Impact of genomic technologies on chickpea breeding strategies. Agronomy 2, 199–221. doi:10.3390/agronomy2030199
Gaur, P. M., Krishnamurthy, L., and Kashiwagi, J. (2008). Improving drought-avoidance root traits in chickpea (Cicer arietinum L.): Current status of research at ICRISAT. Plant Prod. Sci. 11, 3–11. doi:10.1626/pps.11.3
Gowda, S. J. M., Radhika, P., Mhase, L. B., Jamadagni, B. M., Gupta, V. S., Kadoo, N. Y., et al. (2011). Mapping of QTLs governing agronomic and yield traits in chickpea. J. Appl. Genet. 52, 9–21. doi:10.1007/s13353-010-0016-y
Gupta, S., Kumar, T., Verma, S., Bharadwaj, C., and Bhatia, S. (2015). Development of gene-based markers for use in construction of the chickpea (Cicer arietinum L.) genetic linkage map and identification of QTLs associated with seed weight and plant height.Cicer arietinum L.). Mol. Biol. Rep. 42, 1571–1580. doi:10.1007/s11033-015-3925-3
Hajjarpoor, A., Vadez, V., Soltani, A., Gaur, P., Whitbread, A., Babu, D. S., et al. (2018). Characterization of the main chickpea cropping systems in India using a yield gap analysis approach. Field Crops Res. 223, 93–104. doi:10.1016/j.fcr.2018.03.023
Hamwieh, A., Imtiaz, M., and Malhotra, R. S. (2013). Multi-environment QTL analyses for drought-related traits in a recombinant inbred population of chickpea (Cicer arientinum L.). Theor. Appl. Genet. 126, 1025–1038.
Hiremath, P. J., Farmer, A., Cannon, S. B., Woodward, J., Kudapa, H., Tuteja, R., et al. (2011). Large-scale transcriptome analysis in chickpea (Cicer arietinum L.), an orphan legume crop of the semi-arid tropics of Asia and Africa. Plant Biotechnol. J. 9, 922–931. doi:10.1111/j.1467-7652.2011.00625.x
Jaganathan, D., Thudi, M., Kale, S., Azam, S., Roorkiwal, M., Gaur, P. M., et al. (2015). Genotyping-by-sequencing based intra-specific genetic map refines a ‘‘QTL-hotspot” region for drought tolerance in chickpea. Mol. Genet. Genomics 290, 559–571. doi:10.1007/s00438-014-0932-3
Jain, M., Misra, G., Patel, R. K., Priya, P., Jhanwar, S., Khan, A. W., et al. (2013). A draft genome sequence of the pulse crop chickpea (Cicer arietinum L.). Plant J. 74, 715–729. doi:10.1111/tpj.12173
Jamalabadi, J. G., Saidi, A., Karami, E., Kharkesh, M., and Talebi, R. (2013). Molecular mapping and characterization of genes governing time to flowering, seed weight, and plant height in an intraspecific genetic linkage map of chickpea (Cicer arietinum L.). Biochem. Genet. 51, 387–397. doi:10.1007/s10528-013-9571-3
Jingade, P., and Ravikumar, R. L. (2015). Development of molecular map and identification of QTLs linked to Fusarium wilt resistance in chickpea. J. Genet. 94, 723–729. doi:10.1007/s12041-015-0589-7
Jukanti, A. K., Gaur, P. M., Gowda, C. L. L., and Chibbar, R. N. (2012). Nutritional quality and health benefits of chickpea (cicer arietinum L.): A review. Br. J. Nutr. 108, 11–26. doi:10.1017/S0007114512000797
Kale, S. M., Jaganathan, D., Ruperao, P., Chen, C., Punna, R., Kudapa, H., et al. (2015). Prioritization of candidate genes in "QTL-hotspot" region for drought tolerance in chickpea (Cicer arietinum L.). Sci. Rep. 19, 15296. doi:10.1038/srep15296
Kang, B. S., Singh, K., Singh, D., Garg, B. R., Lal, R., Velayutham, M., et al. (2009). Viable alternatives to the rice-wheat cropping system in Punjab. J. Crop Improv. 23 (3), 300–318. doi:10.1080/15427520902809912
Kashiwagi, J., Krishnamurthy, L., Crouch, J. H., and Serraj, R. (2006). Variability of root length density and its contributions to seed yield in chickpea (Cicer arietinum L.) under terminal drought stress. Field Crops Res. 95, 171–181. doi:10.1016/j.fcr.2005.02.012
Kashiwagi, J., Krishnamurthy, L., Gaur, P. M., Upadhyaya, H. D., Varshney, R. K., Tobita, S., et al. (2013). Traits of relevance to improve yield under terminal drought stress in chickpea (C. arietinum L.). Field Crops Res. 145, 88–95. doi:10.1016/j.fcr.2013.02.011
Kashiwagi, J., Krishnamurthy, L., Upadhyaya, H. D., and Gaur, P. M. (2008). Rapid screening technique for canopy temperature status and its relevance to drought tolerance improvement in chickpea. J. Sat. Agric. Res. 6, 1–4.
Kashiwagi, J., Krishnamurthy, L., Upadhyaya, H. D., Krishna, H., Chandra, S., Vadez, V., et al. (2005). Genetic variability of drought-avoidance root traits in the mini-core germplasm collection of chickpea (Cicer arietinum L.). Euphytica 146, 213–222. doi:10.1007/s10681-005-9007-1
Kell, D. B. (2011). Breeding crop plants with deep roots: Their role in sustainable carbon, nutrient and water sequestration. Ann. Bot. 108, 407–418. doi:10.1093/aob/mcr175
Krishnamurthy, L., Ito, O., and Johansen, C. (1996). Dynamics of Roots and Nitrogen in Cropping Systems of the Semi-arid Tropics. Tsukuba, Japan: Japan International Research Center for Agricultural Sciences (235–250.Genotypic differences in root growth dynamics and its implications for drought resistance chickpea
Krishnamurthy, L., Kashiwagi, J., Gaur, P. M., Upadhyaya, H. D., and Vadez, V. (2010). Sources of tolerance to terminal drought in the chickpea (Cicer arietinum L.) mini core germplasm. Field Crops Res. 119, 322–330. doi:10.1016/j.fcr.2010.08.002
Krishnamurthy, L., Kashiwagi, J., Upadhyaya, H. D., Gowda, C. L. L., Gaur, P. M., Singh, S., et al. (2013). Partitioning coefficient—A trait that contributes to drought tolerance in chickpea. Field Crops Res. 149, 354–365. doi:10.1016/j.fcr.2013.05.022
Kudapa, K., Azam, S., Sharpe, A. G., Taran, B., Li, R., Deonovic, B., et al. (2014). Comprehensive transcriptome assembly of chickpea (Cicer arietinum L.) using sanger and next generation sequencing platforms: Development and applications. PLoS ONE 9, e86039. doi:10.1371/journal.pone.0086039
Kumar, J., and Abbo, S. (2001). Genetics of flowering time in chickpea and its bearing on productivity in semi-arid environments. Adv. Agron. 72, 107–138. doi:10.1016/S0065-2113(01)72012-3
Kumar, S., Mittal, R. K., Gupta, D., and Katna, G. (2005). Correlation among some morpho-physiological characters associated with drought tolerance in wheat. Ann. Agri. Bio. Res. 10, 129–134.
Kumar, T., Bharadwaj, C., Rizvi, A. H., Sarker, A., Tripathi, S., Alam, A., et al. (2015). Chickpea landraces: A valuable and divergent source for drought tolerance. Int. J. Trop. Agric. 33, 633–638.
Kushwah, A., Bhatia, D., Rani, U., Yadav, I. S., Singh, I., Bharadwaj, C., et al. (2021c). Molecular mapping of quantitative trait loci for ascochyta blight and botrytis grey mould resistance in an inter-specific cross in chickpea (Cicer arietinum L.) using genotyping by sequencing. Breed. Sci. 71, 229–239. doi:10.1270/jsbbs.20085
Kushwah, A., Bhatia, D., Singh, G., Singh, I., Bindra, S., Vij, S., et al. (2021a). Phenotypic evaluation of genetic variability and selection of yield contributing traits in chickpea recombinant inbred line population under high temperature stress. Physiol. Mol. Biol. Plants. 27, 747–767. doi:10.1007/s12298-021-00977-5
Kushwah, A., Bhatia, D., Singh, I., Thudi, M., Singh, G., Bindra, S., et al. (2021b). Identification of stable heat tolerance QTLs using inter-specific recombinant inbred line population derived from GPF 2 and ILWC 292. PLoS ONE 16, e0254957. doi:10.1371/journal.pone.0254957
Kushwah, A., Bindra, S., Singh, I., Dixit, G. P., Sharma, P., Srinivasan, S., et al. (2020b). Advances in chickpea breeding and genomics for varietal development and trait improvement in India. Accel. Plant Breed. 3, 31–66. Springer. doi:10.1007/978-3-030-47306-8
Kushwah, A., Gupta, S., Bindra, S., Johal, N., Singh, I., Bharadwaj, C., et al. (2020a). Chickpea: Crop Wild Relatives for Enhancing Genetic Gains. Massachusetts: Elsevier Academic Press, 131–165.Gene pyramiding and multiple character breeding
Li, Y., Ruperao, P., Batley, J., Edwards, D., Khan, T., Colmer, T. D., et al. (2018). Investigating drought tolerance in chickpea using genome-wide association mapping and genomic selection based on whole-genome resequencing data. Front. Plant Sci. 9, 190. doi:10.3389/fpls.2018.00190
Lugojan, C., and Ciulca, S. (2011). Evaluation of relative water content in winter wheat. J. Hortic. For. Biotechnol. 15, 173–177.
Maqbool, M. A., Aslam, M., and Ali, H. (2017). Breeding for improved drought tolerance in Chickpea (Cicer arietinum L.). Plant Breed. 136, 300–318. doi:10.1111/pbr.12477
Mashaki, K. M., Garg, V., Ghomi, A. A. N., Kudapa, H., Chitikineni, A., Nezhad, K. Z., et al. (2018). RNA-Seq analysis revealed genes associated with drought stress response in kabuli chickpea (Cicer arietinum L.). PLoS ONE 13, e0199774. doi:10.1371/journal.pone.0199774
Nayak, S. N., Zhu, H., Varghese, N., Datta, S., Choi, H. K., Horres, R., et al. (2010). Integration of novel SSR and gene-based SNP marker loci in the chickpea genetic map and establishment of new anchor points with Medicago truncatula genome.Medicago truncatula genome. Theor. Appl. Genet. 120, 1415–1441. doi:10.1007/s00122-010-1265-1
Pang, J., Turner, N. C., Khan, T., Du, Y-L., Xiong, J-L., Colmer, T. D., et al. (2017). Response of chickpea (Cicer arietinum L.) to terminal drought: Leaf stomatal conductance, pod abscisic acid concentration, and seed set. J. Exp. Bot. 68, 1973–1985. doi:10.1093/jxb/erw153
Peterson, B. K., Weber, J. N., Kay, E. H., Fisher, H. S., and Hoekstra, H. E. (2012). Double Digest RADseq: An inexpensive method for de novo SNP discovery and genotyping in model and non-model species. PLoS ONE 7, e37135. doi:10.1371/journal.pone.0037135
Pinheiro, H. A., DaMatta, F. M., Chaves, A. R. M., Loureiro, M. E., and Ducatti, C. (2005). Drought tolerance is associated with rooting depth and stomatal control of water use in clones of Coffeacanephora. Ann. Bot. 96, 101–108. doi:10.1093/aob/mci154
Premachandra, G. S., Sangroka, H., and Ogata, S. (1990). Cell membrane stability, an indicator of drought tolerance as affected by applied nitrogen in soybean. J. Agric. Sci. 115, 63–66.
Puritz, J. B., Matz, M. V., Toonen, R. J., Weber, J. N., Bolnick, D. I., Bird, C. E., et al. (2014). Demystifying the RAD fad. Mol. Ecol. 23, 5937–5942. doi:10.1111/mec.12965
Purushothaman, R., Krishnamurthy, L., Upadhyaya, H. D., Vadez, V., and Varshney, R. K. (2017). Genotypic variation in soil water use and root distribution and their implications for drought tolerance in chickpea. Funct. Plant Biol. 44, 235–252. doi:10.1071/FP16154
Purushothaman, R., Krishnamurthy, L., Upadhyaya, H. D., Vadez, V., and Varshney, R. K. (2016). Shoot traits and their relevance in terminal drought tolerance of chickpea (Cicer arietinum L.). Field Crops Res. 197, 10–27. doi:10.1016/j.fcr.2016.07.016
Purushothaman, R., Upadhyaya, H. D., Gaur, P. M., Gowda, C. L. L., and Krishnamurthy, L. (2014). Kabuli and desi chickpeas differ in their requirement for reproductive duration. Field Crops Res. 163, 24–31. doi:10.1016/j.fcr.2014.04.006
Rehman, A. U., Malhotra, R. S., Bett, K., Tar’an, B., Bueckert, R., Warkentin, T. D., et al. (2011). Mapping QTL associated with traits affecting grain yield in chickpea (Cicer arietinum L.) under terminal drought stress. Crop Sci. 51, 450–463. doi:10.2135/cropsci2010.03.0129
Roorkiwal, M., Bharadwaj, C., Barmukh, R., Dixit, G. P., Thudi, M., Gaur, P. M., et al. (2020). Integrating genomics for chickpea improvement: Achievements and opportunities. Theor. Appl. Genet. 133, 1703–1720. doi:10.1007/s00122-020-03584-2
Roorkiwal, M., Jain, A., Thudi, M., and Varshney, R. K. (2017). The chickpea genome. New York: Springer, 53–67.Advances in chickpea genomic resources for accelerating the crop improvement
Roorkiwal, M., Pandey, S., Thavarajah, D., Hemalatha, R., and Varshney, R. K. (2021). Molecular mechanisms and biochemical pathways for micronutrient acquisition and storage in legumes to support biofortification for nutritional security. Front. Plant Sci. 12, 682842. doi:10.3389/fpls.2021.682842
Sabaghpour, S. H., Mahmoudi, A. A., Saeed, A., and Iraj, K. (2006). Study of chickpea drought tolerance lines under dryland conditions of Iran. Indian J. Crop Sci. 1, 70–73.
Sachdeva, S., Bharadwaj, C., Sharma, V., Patil, B. S., Soren, K. R., Roorkiwal, M., et al. (2018). Molecular and phenotypic diversity among chickpea (Cicer arietinumL.) genotypes as a function of drought tolerance. Crop Pasture Sci. 69, 142. doi:10.1071/cp17284
Sairam, R. K. (1994). Effect of moisture stress on physiological activities of two contrasting wheat genotypes. Indian J. Exp. Biol. 32, 584–593.
Saxena, N. P. (2003). Management of agricultural drought-agronomic and genetic options. New Delhi, India: Oxford and IBH publishing, 103–122.Management of drought in chickpea-holistic approach
Schoppach, R. M., Wauthelet, D., Jeanguenin, L., and Sadok, W. (2013). Conservative water use under high evaporative demand associated with smaller root metaxylem and limited trans-membrane water transport in wheat. Funct. Plant Biol. 41, 257–269. doi:10.1071/FP13211
Singh, K. B., Di Vito, M., Greco, N., and Saxena, M. C. (1996). Registration of ILWC292, a chickpea cyst nematode resistant germplasm of Cicer reticulatum Ladiz. Crop Sci. 36, 1421–1422. doi:10.2135/cropsci1996.0011183x003600050076x
Singh, R., Sharma, P., Varshney, R. K., Sharma, S. K., and Singh, N. K. (2008). Chickpea improvement: Role of wild species and genetic markers. Biotechnol. Genet. Eng. Rev. 25, 267–313. doi:10.5661/bger-25-267
Sivashakthi, S., Thudi, M., Tharanya, M., Kale, S. M., Kholova, J., Halime, M. H., et al. (2018). Plant vigour QTLs co-map with an earlier reported QTL-hotspot for drought tolerance while water saving QTLs map in other regions of the chickpea genome. BMC Plant Biol. 18, 29. doi:10.1186/s12870-018-1245-1
Stephens, A., Lombardi, M., Cogan, N. O. I., Forster, J. W., Hobson, K., Materne, M., et al. (2014). Genetic marker discovery, intraspecific linkage map construction and quantitative trait locus analysis of ascochyta blight resistance in chickpea (Cicer arietinumL.). Mol. Breed. 33, 297–313. doi:10.1007/s11032-013-9950-9
Thudi, M., Bohra, A., Nayak, S. N., Varghese, N., Shah, T. M., Penmetsa, R. V., et al. (2011). Novel SSR markers from BAC-end sequences, DArT arrays and a comprehensive genetic map with 1291 marker loci for chickpea (Cicer arietinum L.). PLoS ONE 6, e27275. doi:10.1371/journal.pone.0027275
Thudi, M., Upadhyaya, H. D., Rathore, A., Gaur, P. M., Krishnamurthy, L., Roorkiwal, M., et al. (2014). Genetic dissection of drought and heat tolerance in chickpea through genome-wide and candidate gene-based association mapping approaches. PLoS ONE 9, e96758. doi:10.1371/journal.pone.0096758
Toker, C., Lluch, C., Tejera, N. A., Serraj, R., and Siddique, K. H. M. (2007). Chickpea breeding and management. Wallingford: CAB International, 474–496.Abiotic stresses
Tuberosa, R., and Salvi, S. (2006). Genomics-based approaches to improve drought tolerance of crops. Trends Plant Sci. 11, 405–412. doi:10.1016/j.tplants.2006.06.003
Upadhyaya, H. D., Kashiwagi, J., Varshney, R. K., Gaur, P. M., Saxena, K. B., Krishnamurthy, L., et al. (2012). Phenotyping chickpeas and pigeonpeas for adaptation to drought. Front. Physiol. 3, 179. doi:10.3389/fphys.2012.00179
Varshney, R. K., Barmukh, R., Roorkiwal, M., Qi, Y., Kholova, J., Tuberosa, R., et al. (2021). Breeding custom-designed crops for improved drought adaptation. Adv. Genet. Hob. 2, e202100017. doi:10.1002/ggn2.202100017
Varshney, R. K. (2016). Exciting journey of 10 years from genomes to fields and markets: Some success stories of genomics-assisted breeding in chickpea, pigeonpea and groundnut. Plant Sci. 242, 98–107. doi:10.1016/j.plantsci.2015.09.009
Varshney, R. K., Gaur, P. M., Chamarthi, S. K., Krishnamurthy, L., Tripathi, S., Kashiwagi, J., et al. (2013b). Fast-track introgression of “QTL-hotspot” for root traits and other drought tolerance traits in JG 11, an elite and leading variety of chickpea. Plant Genome 6, 1–9. doi:10.3835/plantgenome2013.07.0022
Varshney, R. K., Pazhamala, L., Kashiwagi, J., Gaur, P. M., Krishnamurthy, L., and Hoisington, D. (2011). Genomics and physiological approaches for root trait breeding to improve drought tolerance in chickpea (Cicer arietinum L.). Root Genomics, 233–250. doi:10.1007/978-3-540-85546-0Springer: Germany
Varshney, R. K., Song, C., Saxena, R. K., Azam, S., Yu, S., Sharpe, A., et al. (2013a). Draft genome sequence of chickpea (Cicer arietinum) provides a resource for trait improvement. Nat. Biotechnol. 31, 240–246. doi:10.1038/nbt.2491
Varshney, R. K., Thudi, M., May, G. D., and Jackson, S. A. (2010). Plant breeding reviews. USA: Wiley, 257–304.Legume genomics and breeding
Varshney, R. K., Thudi, M., Nayak, S. N., Gaur, P. M., Kashiwagi, J., Krishnamurthy, L., et al. (2014). Genetic dissection of drought tolerance in chickpea (Cicer arietinum L.). Theor. Appl. Genet. 127, 445–462. doi:10.1007/s00122-013-2230-6
Verma, S., Gupta, S., Bandhiwal, N., Kumar, T., Bharadwaj, C., Bhatia, S., et al. (2015). High-density linkage map construction and mapping of seed trait QTLs in chickpea (Cicer arietinum L) using genotyping-by-sequencing (GBS). Sci. Rep. 5, 17512. doi:10.1038/srep17512
Wang, S., Basten, C. J., and Zeng, Z. B. (2007). Windows QTL cartographer 2.5. Raleigh, NC: Department of Statistics, North Carolina State University.
Wasson, A. P., Rebetzke, G. J., Kirkegaard, J. A., Christopher, J., Richards, R. A., Watt, M., et al. (2014). Soil coring at multiple field environments can directly quantify variation in deep root traits to select wheat genotypes for breeding. J. Exp. Bot. 65, 6231–6249. doi:10.1093/jxb/eru250
Yadav, S., Gill, M. S., and Kukal, S. S. (2007). Performance of direct-seeded basmati rice in loamy sand in semi-arid sub-tropical India. Soil Tillage Res. 97 (2), 229–238. doi:10.1016/j.still.2007.09.019
Ye, H., Roorkiwal, M., Valliyodan, B., Zhou, L., Chen, P., Varshney, R. K., et al. (2018). Genetic diversity of root system architecture in response to drought stress in grain legumes. J. Exp. Bot. 69, 3267–3277. doi:10.1093/jxb/ery082
Yucel, D. O., Anlarsal, A. E., and Yucel, C. (2006). Genetic variability, correlation and path analysis of yield, and yield components in chickpea (Cicer arietinum L.). Turk. J. Agric. For. 30, 183–188.
Keywords: genetic mapping, ddRAD-seq, single nucleotide polymorphism (SNP), quantitative trait locus (QTL), root system architecture
Citation: Kushwah A, Bhatia D, Barmukh R, Singh I, Singh G, Bindra S, Vij S, Chellapilla B, Pratap A, Roorkiwal M, Kumar S, Varshney RK and Singh S (2022) Genetic mapping of QTLs for drought tolerance in chickpea (Cicer arietinum L.). Front. Genet. 13:953898. doi: 10.3389/fgene.2022.953898
Received: 26 May 2022; Accepted: 05 July 2022;
Published: 19 August 2022.
Edited by:
Karansher Singh Sandhu, Bayer Crop Science, United StatesReviewed by:
Mangesh Pralhad Jadhav, National Institute of Plant Genome Research (NIPGR), IndiaJagmohan Singh, Guru Angad Dev Veterinary and Animal Sciences University, India
Vishal Dinkar, Central Institute of Temperate Horticulture (ICAR), India
Kajal Samantara, Centurion University of Technology and Management, India
Copyright © 2022 Kushwah, Bhatia, Barmukh, Singh, Singh, Bindra, Vij, Chellapilla, Pratap, Roorkiwal, Kumar, Varshney and Singh. This is an open-access article distributed under the terms of the Creative Commons Attribution License (CC BY). The use, distribution or reproduction in other forums is permitted, provided the original author(s) and the copyright owner(s) are credited and that the original publication in this journal is cited, in accordance with accepted academic practice. No use, distribution or reproduction is permitted which does not comply with these terms.
*Correspondence: Rajeev K. Varshney, cmFqZWV2LnZhcnNobmV5QG11cmRvY2guZWR1LmF1; Sarvjeet Singh, c2FydmplZXQ2MkBwYXUuZWR1
†Present address:Manish Roorkiwal, Khalifa Center for Genetic Engineering and Biotechnology, United Arab Emirates University, Al-Ain, United Arab Emirates