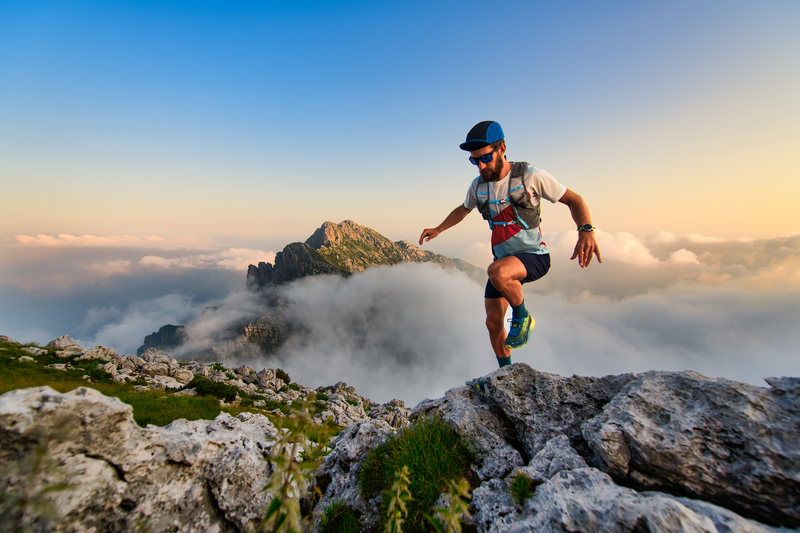
94% of researchers rate our articles as excellent or good
Learn more about the work of our research integrity team to safeguard the quality of each article we publish.
Find out more
ORIGINAL RESEARCH article
Front. Genet. , 17 August 2022
Sec. Cancer Genetics and Oncogenomics
Volume 13 - 2022 | https://doi.org/10.3389/fgene.2022.953580
This article is part of the Research Topic The Involvement of Systemic Homeostasis in Tumour Biology View all 21 articles
To determine the relationship between glioma and muscle aging and to predict prognosis by screening for co-expressed genes, this study examined the relationship between glioma and sarcopenia. The study identified eight co-downregulated miRNAs, three co-upregulated miRNAs, and seven genes associated with overall glioma survival, namely, KRAS, IFNB1, ALCAM, ERBB2, STAT3, FOSL1, and EN2. With a multi-factor Cox regression model incorporating FOSL1 and EN2, we obtained ROC curves of 0.702 and 0.709, respectively, suggesting that glioma prognosis can be predicted by FOSL1 and EN2, which are differentially expressed in both cancer and aged muscle. FOSL1 and EN2 were analyzed using Gene Set Enrichment Analysis to identify possible functional pathways. RT-qPCR and a dual-luciferase reporter gene system verified that hsa-miR-33a targets FOSL1 and EN2. We found that hsa-mir-33a co-targeting FOSL1 and EN2 has a good predictive value for glioblastoma and skeletal muscle reduction.
Gliomas are the most common primary tumors of the central nervous system (CNS), arising from glial cells or supporting cells (Davis, 2018; Ostrom et al., 2018). The World Health Organization updated their classification system for gliomas in May 2016, taking advantage of advances in molecular genetics and epigenetic research. The revised guidelines aim to allow oncologists to better diagnose, predict treatment outcomes, and improve individualized treatment plans for patients (Louis et al., 2016; Wesseling and Capper, 2018). About 49% of malignant tumors of the CNS are gliomas, which can be found anywhere in the CNS but are most frequently in the frontal and temporal lobes (Schmoldt et al., 1975; Aldape et al., 2015; Ostrom et al., 2018; Miller et al., 2021). Early symptoms of glioma, similar to other benign neurological disorders, include headache, vomiting, loss of vision, seizures, weakness, confusion, and other signs of increased intracranial pressure (Rasmussen et al., 2017; Ozawa et al., 2019). The most common treatment for glioma is surgical resection followed by radiotherapy and chemotherapy (Wen et al., 2020). However, the blood–brain barrier prevents most drugs from reaching the tumor site, so even with combination therapy, the overall survival rate of patients with gliomas is still low (Stummer et al., 2012; Kim et al., 2019; Tan et al., 2020; Miller et al., 2021). Therefore, for early diagnosis and risk assessment of glioma, transcriptomic and epigenomic screening of neuroblastoma-related biomarkers is critical to facilitate early targeted interventions to improve survival.
Sarcopenia is an age-related syndrome of progressive decline in skeletal muscle mass and function, associated with adverse outcomes such as decreased body function, impaired quality of life, physical disability, and death (Cruz-Jentoft et al., 2019). In patients with chronic diseases, malnutrition, and malignancies, sarcopenia is associated with poor prognosis, increasing the risk of recurrence and death (Xia et al., 2020; Williams et al., 2021). Previous studies suggested that sarcopenia and loss of temporal muscle thickness may be associated with lower overall survival (OS) in glioma patients (An et al., 2021; Guven et al., 2021; Huq et al., 2021). There are, however, relatively few studies investigating the association between glioma prognosis and sarcopenia expression profiles.
Typically, sarcopenia is characterized by muscle aging and loss of function and mass in the elderly (Larsson et al., 2019). Muscle loss and sarcopenia are regulated by microRNAs (miRNAs) (Wang et al., 2020; Javanmardifard et al., 2021). MicroRNAs) are endogenous, small RNAs that have a variety of regulatory functions in living organisms (Sun et al., 2017; Chen et al., 2019; Hill and Tran, 2021; Chen et al., 2022). Glioma miRNAs are known to be potential diagnostic markers (Zhou et al., 2018). In previous studies, miRNAs were differentially expressed in the skeletal muscles of elderly people (Larsson et al., 2019). MiRNA-1245a, for instance, has been identified as a potential key molecule for treating glioma-related sarcopenia (An and Wang, 2021). The regulation of miRNAs in the skeletal muscle is influenced by systemic homeostasis (Kim et al., 2020; Podkalicka et al., 2022). The development of sarcopenia and poor outcome for glioma patients are likely to occur as a result of gliomas deteriorating the body’s microenvironmental homeostasis (Magnus et al., 2014; Chen and Kang, 2015). Gliomas may cause sarcopenia by affecting miRNA expression in skeletal muscle.
This study examined miRNAs associated with glioma and sarcopenia and their potentially regulated mRNAs. We screened for co-expressed genes to determine the relationship between glioma and sarcopenia and predict prognosis.
The Gene Expression Omnibus (GEO) database provided glioma-derived exosome miRNA transcript datasets (GSE122488), which included 16 standard samples and 22 glioma samples. The miRNAs that did not appear in more than 10 samples were removed. Additionally, 17 old Basal samples and 19 young Basal samples were obtained from skeletal muscle-derived miRNA transcript datasets (GSE23527). For 143 glioma samples, transcriptomic FPKM expression data were obtained from the UCSC Xena website (https://xenabrowser.net/). Expression data were transformed into log2 (x+1) when multiple probes corresponded to the same gene.
GSE122488 and GSE23527 datasets were screened for differentially expressed miRNAs associated with GBM and aging, respectively. Differentially expressed miRNAs were those with p-values less than 0.05. Differentially expressed miRNA volcanoes were mapped using the ggplot2 package (Version 3.3.5) and the ggrepel package (Version 0.9.1), and differentially expressed miRNA heatmaps were mapped using the pheatmap package (Version 1.0.12).
The miRTarBase database contains a large number of experimentally validated miRNA target gene regulatory pairs, ensuring the reliability of the data (Huang et al., 2019). Based on miRTarBase, co-differentially expressed miRNA target genes were examined, and pairs with at least two validated results in Western blot, qPCR, or CLIPseq were retained. Cytoscape (Version 3.8.0) was used to map the miRNA-target regulatory network.
For all target genes, the clusterProfiler (Version 4.0.5) package and org. Hs.eg.db (Version 3.13.0) package were used to perform GO and KEGG enrichment analyses, retaining all enriched entries with FDR <0.05 as in previous research studies (Fabris et al., 2020; Huang et al., 2021; Li et al., 2022a; Zhang et al., 2022a; Zhang et al., 2022b; Wang et al., 2022).
Training (70%, n = 100) and validation (30%, n = 43) sets of The Cancer Genome Atlas (TCGA) glioblastoma dataset were randomly divided. Based on the training set samples, a survival risk prediction model was constructed and its performance was then validated using the validation set. To identify the miRNA targets associated with overall survival, a univariate Cox proportional risk regression analysis was conducted on all target genes of common differential miRNAs. The Least absolute shrinkage and selection operator (LASSO) Cox model in the glmnet package (Version 4.1-3) was further used to screen genes with one-way Cox p < 0.05 and multicollinearity between the genes was removed. The gene screened by the LASSO regression analysis was then used to construct a multifactorial Cox proportional risk regression model based on the survival package (version 3.2-13) and the survminer package (version 0.4.9). The risk score for each patient was calculated by entering the expression values of the characteristic genes screened by multifactorial Cox regression analysis into the formula. Patients in the training set were segregated into low- and high-risk groups, the relationship between risk score levels was analyzed, and Kaplan–Meier curves were used for prognosis. In addition, the survivalROC (Version 1.0.3) package was used to evaluate the time-dependent subject operating characteristic curve (ROC) of the prediction model over multiple years.
TCGA-glioma samples were classified into high-expression and low-expression groups by median gene expression values for the signature genes screened. The Gene Set Enrichment Analysis (GSEA) was performed on the Kyoto Encyclopedia of Genes and Genomes (KEGG) pathway using the clusterProfiler package (Version 4.0.5), with the nPermSimple parameter set to 10,000.
The human glioma cell lines U251 and 293T cells were purchased from the Institute of Cell Research, Chinese Academy of Sciences, Shanghai, and cultured in a constant humidity incubator with 5% CO2 in DMEM+10% FBS+1% double-antibody.
The total RNA was extracted using Trizol (Beyotime, Shanghai, China) according to the instructions and reverse-transcribed into cDNA using the GoScript ™ Reverse Transcription Kit (Promega, Wisconsin, United States), followed by TB Green Premix Ex Taq (Takara, Japan) to determine the mRNA expression levels. The mRNA expression levels were calculated as 2-ΔΔCt, and GAPDH was used as an internal reference.
The pmirGLO-FOSL1-3′ UTR-WT and pmirGLO-EN2-3′ UTR-WT vector plasmids were synthesized by Shanghai Biotech, and the corresponding pmirGLO-FOSL1-3′ UTR-MUT and pmirGLO-EN2-3′ UTR-MUT were used as controls. A total of 500 ng of vector plasmid and 100 pmol of hsa-miR-33a mimics were transfected into 293 T cells, and the fluorescence was detected by using a Dual-Luciferase Assay Kit from Promega (United States) after 24 h.
All data from at least three independent experiments are expressed as mean ± standard deviation. A t-test was used to analyze the statistical differences between two independent samples. p < 0.05 was considered to be statistically significant.
The GSE122488 dataset was screened using differential expression analysis to identify 105 downregulated miRNAs and 62 upregulated miRNAs in glioma (Figure 1A). Aged muscle GSE23527 was also screened for 40 downregulated and 15 upregulated miRNAs (Figure 1B). Furthermore, we obtained eight co-downregulated miRNAs (hsa-miR-512, hsa-miR-660, hsa-miR-1304, hsa-miR-30d, hsa-miR-33a, hsa-miR-337, hsa-miR-1277, and hsa-miR-758) and three co-upregulated miRNAs (hsa-miR-25, hsa-let-7b, and hsa-miR-215) (Figures 1C,D). Different subgroups of the samples displayed different expression patterns of differentially expressed miRNAs, as demonstrated by the expression heatmap (Figures 1E,F). The development of glioma with muscle aging may be closely related to those miRNAs.
FIGURE 1. Differentially expressed miRNAs in glioma and muscle. (A) Volcano plot of differentially expressed miRNAs in the GSE122488 dataset. Green dots indicate downregulated miRNAs and red dots indicate upregulated differential miRNAs. Common differential miRNAs of both datasets are marked on the plot. (B) Volcano plot of differentially expressed miRNAs in the GSE23527 dataset (glioma vs. healthy). Green dots indicate downregulated miRNAs and red dots indicate upregulated differential miRNAs. Common differential miRNAs of both datasets are marked on the plot. (C,D) Venn diagram of differential downregulated and upregulated miRNAs in the two datasets. (E) Expression heatmap of differentially expressed miRNAs in the GSE23527 dataset. MiRNA expression values were Z-score transformed. (F) Expression heatmap of differentially expressed miRNAs in the GSE122488 dataset; miRNA expression values were transformed by the Z-score.
To identify co-differentially expressed miRNAs, we used the miRTarBase database to predict the target genes and screened for miRNA-gene pairs with at least two validation records in a reporter assay, Western blot, qPCR, and CLIPseq. The results showed that miR-1277 and miR-1304 had no target genes; miR-758 and miR512 both had two target genes; miR-660 had one target gene; miR-337 had three target genes; miR-215 had eight target genes; miR-30d had 11 target genes; miR-25 had 18 target genes; miR-33a had 21 target genes; and let-7b had 32 target genes (Figure 2A). The miRNA–target interaction network showed that HMGA2, IRS2, CASP3, TP53, EZH2, and SMAD7 were regulated by two miRNAs, and there was no common target gene between most of the miRNAs (Figure 2A). To further investigate the biological processes affected by these differential miRNAs (hsa-let7b, hsa-miR-25, hsa-miR-30d, hsa-miR-33a, hsa-miR-337, hsa-miR-512, hsa-miR-660, and hsa-miR-758), GO and KEGG enrichment analyses were performed for all common differential miRNAs, involving 1,173 GO pathways (including 1083 BP, 12 CC, and 78 MF) and 35 KEGG pathways. The enrichment results indicated that these miRNAs might be involved in the cell cycle, gene transcription, and cancer-related pathways (Figures 2B,C). Accordingly, these miRNAs may play an essential role in cancer development by targeting specific mRNAs.
FIGURE 2. Target gene prediction of common differential miRNAs and their functional enrichment analysis. (A) Differential regulation of miRNAs. The diamond-shaped dots indicate miRNAs. Green and red dots indicate downregulation and upregulation, respectively, and blue dots indicate miRNA regulatory targets. (B) GO enrichment results of miRNA target genes, highlighting the top 10 GO terms of BP, CC, and MF. (C) KEGG enrichment results of miRNA target genes show the top 10 enrichment pathways.
TCGA training set samples were used to construct a model of glioma-related survival risk prediction. KRAS, IFNB1, ALCAM, ERBB2, STAT3, FOSL1, and EN2 were screened by univariate Cox proportional risk regression (Figure 3A). ALCAM was a protective factor for patient survival, whereas the others were risk factors. The Cox regression analysis revealed an association between high IFNB1 expression and poor prognosis. We then used LASSO Cox regression to further remove the multicollinearity between genes, and LASSO regression did not reject any genes (Figure 3C). Finally, we obtained a convergent patient survival risk regression model using multifactorial Cox proportional risk regression and stepwise regression with two characteristic genes, FOSL1 (hazard ratio, 1.357; 95% CI, 1.077 to 1.711; p = 0.010), and EN2 (hazard ratio, 1.415; 95% CI, 1.090 to 1.837; p = 0.009) (Figure 3E). The formula for predicting the survival risk is risk score = (FOSL1 expression × 0.3056) + (EN2 expression × 0.3471). FOSL1 and EN2 may affect the prognosis of gliomas independently. The Kaplan–Meier survival analysis was used to compare the survival differences between the high- and low-risk groups based on the median values of the risk scores of the training set samples. The high-risk group’s prognosis was worse than the low-risk group’s (p = 0.011) (Figure 3D). Therefore, the multifactorial Cox model developed by FOSL1 and EN2 can better predict glioma prognoses.
FIGURE 3. Prognostic value of miRNA target genes. (A) Genes targeted by significant miRNAs were analyzed by univariate Cox proportional risk regression. (B,C) Lasso regression analysis was conducted on the seven target genes screened by univariate Cox proportional risk regression analysis, and the seven best genes were screened again by Lasso regression. (D) K–M plots show the survival differences between high- and low-risk groups in the training set samples. (E) Survival risk regression model was constructed using multi-factor Cox proportional risk regression and stepwise regression, and two genes were retained, FOSL1 and EN2.
Based on the prediction model, we constructed a nomogram (Figure 4A) and plotted the calibration curves for the model predicting overall survival at 1 and 2 years (Figures 4C,D). To evaluate the model’s predictive performance, we plotted time-dependent ROC curves. AUCs for the training sets 1, 2, 3, 4, and 5 years were 0.678, 0.748, 0.881, 0.979, and 0.957, respectively (Figure 4B). These results suggest that this nomogram model has good predictive power.
FIGURE 4. Constructing a nomogram prediction model. (A) Nomogram prediction model for prognostic risk. (B) Time-dependent ROC curves for the overall survival of the training set. During the first, second, third, and fourth years, AUC values were evaluated. (C,D) Calibration curves for predicting the overall survival of the training set at 1 and 2 years.
We calculated the survival scores of the validation set samples and compared the survival differences between the high-risk and low-risk groups to further validate the performance of the survival risk prediction model. The high-risk group had a poor prognosis in the validation set (Figure 5A). Additionally, we plotted time-dependent ROC curves of the risk model to predict overall survival at 1 and 2 years for the validation set samples with AUC values of 0.702 and 0.709, respectively (Figure 5B). To investigate whether FOSL1 and EN2 are independent prognostic factors, we divided all TCGA-glioma samples into high- and low-expression groups based on median gene expression values. The Kaplan–Meier survival analysis was then used to compare the survival differences between the high- and low-expression groups. The results showed that both the high-expression groups of FOSL1 and EN2 had poor prognosis (Figures 6A,B).
FIGURE 5. Nomogram prediction model evaluation. (A) Difference in the overall survival between high-risk and low-risk groups. (B)We evaluated the AUC values at 1 and 2 years based on time-dependent ROC curves for the overall survival of the validation set. (C,D) Calibration curves for predicting the overall survival in the validation set at 1 and 2 years.
FIGURE 6. Prognostic model signature genes analyzed by GSEA. (A,B) Overall survival differences among FOSL1 and EN2 high- and low-expression groups (grouped by median expression values). (C) GSEA enrichment of KEGG pathways in FOSL1 high- and low-groups. (D) GSEA significantly enriched the KEGG pathway in the high- and low-EN2 expression groups.
In comparing high- and low-expression groups of FOSL1 and EN2, we examined the functional pathways involved with these proteins. FOSL1-related GSEA analysis significantly enriched 23 KEGG pathways, including 22 activated pathways (NES >0), such as leukocyte transendothelial migration, VEGF signaling pathway, and apoptotic cell death. FOSL1-associated top five pathways were plotted using GSEA (Figure 6C). Based on the EN2-related GSEA analysis, only two functional pathways were enriched, proteasome and ribosome (Figure 6D).
FOSL1 and EN2, which are target mRNAs of hsa-miR-33a, can be used to predict the prognosis of glioma based on the results of the bioinformatics analysis. As risk factors for glioma, both FOSL1 and EN2 can be considered and further verified to be regulated and targeted by hsa-miR-33a. Glioma cell lines were treated with mimics of hsa-miR-33a. As shown in Figures 7A,B RT-qPCR analysis of mimics-treated glioma cell lines showed decreased expression of FOSL1 and EN2. We then used the dual-luciferase reporter gene system to detect the regulation of miRNAs and target genes (Figure 7C,D). The results indicate that miR-33a targets and regulates FOSL1 and EN2. In summary, miR-33a with differential expression in glioma and sarcopenia may affect the prognosis of glioma by targeting and regulating FOSL1 and EN2.
FIGURE 7. Hsa-miR-33a targets and regulates the expression of FOSL1 and EN2. (A) RT-qPCR results indicate that FOSL1 expression is lower in miR-33a-treated glioma cell lines than in the control. (B) RT-qPCR results indicated that EN2 expression was lower in miR-33a-treated glioma cell lines than in the control. (C) Dual-luciferase reporter gene system indicates the targeting relationship between FOSL1 and hsa-miR-33a. (D) Dual-luciferase reporter gene system indicates the targeting relationship between EN2 and hsa-miR-33a. *- p < 0.05 and **- p < 0.01.
We identified co-expressed differential miRNA genes in glioma and skeletal muscle miRNA datasets. Target genes were predicted using the miRTarBase database after functional annotation according to GO and KEGG. ROC curves were plotted to evaluate the prognostic value of the aforementioned target genes in glioma. Multivariate Cox regression was used to construct a model of prognosis prediction for glioma. The model was evaluated by plotting ROC curves and K–M curves. This model accurately predicted the prognosis for glioblastoma based on correlation analysis, providing an exciting pathway for clinical applications.
Glioma is the most common type of malignant tumor in the central nervous system, and its pathogenesis is complex, involving genetic background, gene mutation, tumor microenvironment, and other aspects (McKinnon et al., 2021). However, most GBMs have no identifiable risk factors for tumor development. Some rare familial cancer syndromes such as Lynch syndrome, neurofibromatosis type 1, and tuberous sclerosis have increased the risk of developing glioma (Ostrom et al., 2014; Wen et al., 2020). Glioma is characterized by aggressiveness and poor prognosis of the disease. In addition, the early diagnosis of glioblastoma is often tricky due to the lack of specificity of early clinical symptoms and the lack of early diagnostic tools. With the improvement of microsurgery technology, the application of various new radiotherapy techniques, and the introduction of chemotherapy and targeted drugs, the clinical prognosis of glioma has been improved to some extent. However, the overall survival rate of patients is still extremely poor, with a median survival time of only about 1 year, and almost all patients tend to relapse (Jhanwar-Uniyal et al., 2015; Kim et al., 2019). Therefore, developing a validated risk assessment model is essential to guide early clinical diagnosis, prognostic assessment, and individualized treatment.
Sarcopenia is an age-related syndrome of progressive decline in skeletal muscle mass and function, commonly seen in patients with malignancies and associated with poor prognosis in cancer patients (Williams et al., 2021). Several studies have shown that temporalis muscle thickness can be used as a surrogate marker for sarcopenia (Huq et al., 2021). Temporalis muscle thickness was also significantly associated with the expected survival of treated glioma patients with recurrent or concomitant brain metastases (Muglia et al., 2021). Quantifying temporalis muscle thickness has clinical value in the prognostic assessment of glioma (Guven et al., 2021; Mi et al., 2022). This study obtained co-expressed differential miRNA genes by analyzing glioma and skeletal muscle miRNA datasets, followed by multifactorial Cox regression analysis and stepwise regression analysis to obtain a patient survival risk regression model, which included FOSL1 and EN2.
FOSL1 belongs to the Fos gene family and encodes FOS-associated antigen 1 (FRA1), which is involved in forming a transcription factor complex AP-1 (Matsuo et al., 2000). In addition, as a proto-oncogene, FOSL1 also plays a vital role in tumorigenesis and can promote tumor cell metastasis through epithelial–mesenchymal transition (EMT). FOS1 also has prognostic value in various epithelial tumors, and its overexpression is associated with tumor aggressiveness, chemotherapy resistance, tumor progression, and poor survival prognosis (Yu et al., 2013; Gao et al., 2017; Vallejo et al., 2017; Xu et al., 2017; Sobolev et al., 2022). It has been shown that FOSL1 can promote the development and invasion of colorectal cancer through the Smurf1-mediated FBXL2/Wnt/β-catenin axis and the migration, invasion, and proliferation of breast, head, and neck squamous cell carcinoma, pancreatic cancer, bladder cancer, and prostate cancer (Elangovan et al., 2018; Luo et al., 2018; Cui et al., 2020; Dai et al., 2021; Hyakusoku et al., 2021; Liu et al., 2021). In addition, FOSL1 also mediates the dephosphorylation of proliferation and apoptosis bridging protein 15 (PEA15) by upregulating dual-specificity phosphatase 7 (DUSP7), which increases drug resistance in breast cancer (Li et al., 2022b). It is to be noted that FOSL1 was also associated with glioma growth and invasion and was a poor prognostic factor for GBM (Guo et al., 2022). Gliomas overexpress FRA1, which regulates the malignancy of gliomas, including morphology, growth pattern, and tumorigenic potential (Debinski and Gibo, 2005). The engrailed-2 (EN2) gene encodes a transcription factor containing a homology cassette involved in regionalization, patterning, and cellular differentiation in early brain development. It plays an essential role during nervous system development. In addition, disorders of EN2 regulation can lead to abnormal cell proliferation, leading to tumorigenesis. Studies show that EN2 plays an important role in the proliferation, migration, and invasion of various tumor cells, including prostate cancer, colorectal cancer, esophageal squamous cell carcinoma, lung cancer, and bladder cancer, and is closely related to the poor prognosis of tumor patients (Zhou et al., 2017; Lin et al., 2018; Cao et al., 2020; Li et al., 2020; Li et al., 2021). EN2 promotes the proliferation and invasion of colorectal cancer cells by regulating CCL20, thus promoting the progression of colorectal cancer. EN2 also promotes the invasion and metastasis of esophageal squamous cell carcinoma by upregulating SPARC expression. It promotes the proliferation, invasion, and metastasis of bladder cancer cells by activating the PI3K/Akt pathway and inhibiting the PTEN gene (Cao et al., 2020; Li et al., 2020) (Li et al., 2021). In addition, it was found that EN2 expression levels are also correlated with the degree of malignancy of gliomas and promoted the malignant progression of glioma (Zeng et al., 2020). These lines of evidence indicate that FOSL1 and EN2, which are involved in our risk assessment model, have prognostic value in various tumors, including glioma.
MiR-33a plays a variety of physiological roles in tumor microenvironments, and it has been proposed as a potential target for cancer prevention and therapy (Gao et al., 2020). By inhibiting phosphorylation of JAK2 and STAT3, which are highly activated in many malignant cells, including glioma cells, miR-33a inhibits the growth, invasion, and EMT of tumor cells (Feng et al., 2016; Chang et al., 2017; Liu et al., 2019a; Liu et al., 2019b). Aside from targeting PDE8A and UVRAG, miR-33a also targets cAMP/PKA and NOTCH signaling pathways in glioma cancer cells (Wang et al., 2014). It is to be noted that both signaling pathways promote self-renewal of glioma-initiating cells only when they are activated simultaneously (Jiang et al., 2019). MiR-33a may exert oncogenic effects by regulating JAK2/STAT3, cAMP/PKA, and NOTCH signaling pathways in gliomas. Further investigation is needed to determine whether miR-33a overexpression affects glioma cell proliferation, invasion, and other oncogenic features.
In this study, hsa-miR-33a was found to regulate FOSL1 and EN2 and affect the prognosis of gliomas. Furthermore, hsa-miR-33a appeared to be involved in sarcopenia and gliomas. However, a larger sample of data is needed to validate the findings conclusively, and further experimental studies are needed to help understand the specific regulatory mechanisms. Because this study only has data on skeletal muscle age, sarcopenia cannot be fully simulated. Furthermore, miR-33a downregulation caused by glioma and muscle aging may also be caused by sarcopenia, but this remains unclear as we did not investigate which causes miR-33a downregulation.
In conclusion, this study’s risk model provides good clinical application and predictive value for assessing the risk and prognosis of glioblastoma and a potential therapeutic target. Moreover, hsa-mir-33a co-targeting FOSL1 and EN2 has a good predictive value for glioma and skeletal muscle reduction.
The original contributions presented in the study are publicly available. This data can be found here: https://www.ncbi.nlm.nih.gov/geo/GSE122488; GSE23527.Transcriptomic FPKM expression data was accessed from the UCSC Xena website (https://xenabrowser.net/).
Conceptualization: HJ and WW; methodology: WW and WL; software: WW; validation: WW and JX; writing—original draft preparation: WW, WL, JX, and HJ; writing—review and editing: WW; all authors contributed to the manuscript and approved the submitted version.
The authors declare that the research was conducted in the absence of any commercial or financial relationships that could be construed as a potential conflict of interest.
All claims expressed in this article are solely those of the authors and do not necessarily represent those of their affiliated organizations, or those of the publisher, the editors, and the reviewers. Any product that may be evaluated in this article, or claim that may be made by its manufacturer, is not guaranteed or endorsed by the publisher.
Aldape, K., Zadeh, G., Mansouri, S., Reifenberger, G., and von Deimling, A. (2015). Glioblastoma: Pathology, molecular mechanisms and markers. Acta Neuropathol. 129, 829–848. doi:10.1007/s00401-015-1432-1
An, G., Ahn, S., Park, J-S., Jeun, S-S., and Hong, Y-K. (2021). Association between temporal muscle thickness and clinical outcomes in patients with newly diagnosed glioblastoma. J. Cancer Res. Clin. Oncol. 147, 901–909. doi:10.1007/s00432-020-03386-5
An, L., and Wang, Y. (2021). Potential roles of miRNA-1245a regulatory networks in sarcopenia. Int. J. Gen. Med. 14, 6807–6813. doi:10.2147/IJGM.S334501
Cao, Y., Wang, X., Tang, L., Li, Y., Song, X., Liu, X., et al. (2020). Engrailed-2 promotes a malignant phenotype of esophageal squamous cell carcinoma through upregulating the expression of pro-oncogenic genes. PeerJ 8, e8662. doi:10.7717/peerj.8662
Chang, M., Qiao, L., Li, B., Wang, J., Zhang, G., Shi, W., et al. (2017). Suppression of SIRT6 by miR-33a facilitates tumor growth of glioma through apoptosis and oxidative stress resistance. Oncol. Rep. 38, 1251–1258. doi:10.3892/or.2017.5780
Chen, L., Heikkinen, L., Wang, C., Yang, Y., Sun, H., and Wong, G. (2019). Trends in the development of miRNA bioinformatics tools. Brief. Bioinform. 20, 1836–1852. doi:10.1093/bib/bby054
Chen, L., and Kang, C. (2015). miRNA interventions serve as ‘magic bullets’ in the reversal of glioblastoma hallmarks. Oncotarget 6, 38628–38642. doi:10.18632/oncotarget.5926
Chen, Y., Sun, Y., Luo, Z., Lin, J., Qi, B., Kang, X., et al. (2022). Potential mechanism underlying exercise upregulated circulating blood exosome miR-215-5p to prevent necroptosis of neuronal cells and a model for early diagnosis of alzheimer’s disease. Front. Aging Neurosci. 14, 860364. doi:10.3389/fnagi.2022.860364
Cruz-Jentoft, A. J., Bahat, G., Bauer, J., Boirie, Y., Bruyère, O., Cederholm, T., et al. (2019). Sarcopenia: Revised European consensus on definition and diagnosis. Age Ageing 48, 16–31. doi:10.1093/ageing/afy169
Cui, Y-P., Xie, M., Pan, W-X., Zhang, Z-Y., and Li, W-F. (2020). HOXA10 promotes the development of bladder cancer through regulating FOSL1. Eur. Rev. Med. Pharmacol. Sci. 24, 2945–2954. doi:10.26355/eurrev_202003_20659
Dai, C., Rennhack, J. P., Arnoff, T. E., Thaker, M., Younger, S. T., Doench, J. G., et al. (2021). SMAD4 represses FOSL1 expression and pancreatic cancer metastatic colonization. Cell Rep. 36, 109443. doi:10.1016/j.celrep.2021.109443
Davis, M. E. (2018). Epidemiology and overview of gliomas. Semin. Oncol. Nurs. 34, 420–429. doi:10.1016/j.soncn.2018.10.001
Debinski, W., and Gibo, D. M. (2005). Fos-related antigen 1 modulates malignant features of glioma cells. Mol. Cancer Res. 3, 237–249. doi:10.1158/1541-7786.MCR-05-0004
Elangovan, I. M., Vaz, M., Tamatam, C. R., Potteti, H. R., Reddy, N. M., and Reddy, S. P. (2018). FOSL1 promotes kras-induced lung cancer through amphiregulin and cell survival gene regulation. Am. J. Respir. Cell Mol. Biol. 58, 625–635. doi:10.1165/rcmb.2017-0164OC
Fabris, F., Palmer, D., de Magalhães, J. P., and Freitas, A. A. (2020). Comparing enrichment analysis and machine learning for identifying gene properties that discriminate between gene classes. Brief. Bioinform. 21, 803–814. doi:10.1093/bib/bbz028
Feng, J., Yan, P-F., Zhao, H-Y., Zhang, F-C., Zhao, W-H., and Feng, M. (2016). SIRT6 suppresses glioma cell growth via induction of apoptosis, inhibition of oxidative stress and suppression of JAK2/STAT3 signaling pathway activation. Oncol. Rep. 35, 1395–1402. doi:10.3892/or.2015.4477
Gao, C., Wei, J., Tang, T., and Huang, Z. (2020). Role of microRNA-33a in malignant cells. Oncol. Lett. 20, 2537–2556. doi:10.3892/ol.2020.11835
Gao, X-Q., Ge, Y-S., Shu, Q-H., and Ma, H-X. (2017). Expression of Fra-1 in human hepatocellular carcinoma and its prognostic significance. Tumour Biol. 39, 1010428317709635. doi:10.1177/1010428317709635
Guo, S., King, P., Liang, E., Guo, A. A., and Liu, M. (2022). LncRNA HOTAIR sponges miR-301a-3p to promote glioblastoma proliferation and invasion through upregulating FOSL1. Cell. Signal. 94, 110306. doi:10.1016/j.cellsig.2022.110306
Guven, D. C., Aksun, M. S., Cakir, I. Y., Kilickap, S., and Kertmen, N. (2021). The association of BMI and sarcopenia with survival in patients with glioblastoma multiforme. Future Oncol. 17, 4405–4413. doi:10.2217/fon-2021-0681
Hill, M., and Tran, N. (2021). miRNA interplay: Mechanisms and consequences in cancer. Dis. Model. Mech. 14, dmm047662. doi:10.1242/dmm.047662
Huang, H-Y., Lin, Y-C-D., Li, J., Huang, K-Y., Shrestha, S., Hong, H-C., et al. (2019). miRTarBase 2020: updates to the experimentally validated microRNA–target interaction database. Nucleic Acids Res. 48, D148–D154. doi:10.1093/nar/gkz896
Huang, J., Liang, X., and Cai, Z. (2021). A potential ceRNA network for neurological damage in preterm infants. Biomed. Res. Int. 2021, 2628824. doi:10.1155/2021/2628824
Huq, S., Khalafallah, A. M., Ruiz-Cardozo, M. A., Botros, D., Oliveira, L. A. P., Dux, H., et al. (2021). A novel radiographic marker of sarcopenia with prognostic value in glioblastoma. Clin. Neurol. Neurosurg. 207, 106782. doi:10.1016/j.clineuro.2021.106782
Hyakusoku, H., Sawakuma, K., Sano, D., Takahashi, H., Hatano, T., Sato, K., et al. (2021). FosL1 regulates regional metastasis of head and neck squamous cell carcinoma by promoting cell migration, invasion, and proliferation. Anticancer Res. 41, 3317–3326. doi:10.21873/anticanres.15119
Javanmardifard, Z., Shahrbanian, S., and Mowla, S. J. (2021). MicroRNAs associated with signaling pathways and exercise adaptation in sarcopenia. Life Sci. 285, 119926. doi:10.1016/j.lfs.2021.119926
Jhanwar-Uniyal, M., Labagnara, M., Friedman, M., Kwasnicki, A., and Murali, R. (2015). Glioblastoma: Molecular pathways, stem cells and therapeutic targets. Cancers 7, 538–555. doi:10.3390/cancers7020538
Jiang, K., Sun, F., Zhu, J., Luo, G., Ban, Y., and Zhang, P. (2019). miR-33a inhibits cell growth in renal cancer by downregulation of MDM4 expression. Mol. Genet. Genomic Med. 7, e833. doi:10.1002/mgg3.833
Kim, J., Aydemir, T. B., Jimenez-Rondan, F. R., Ruggiero, C. H., Kim, M-H., and Cousins, R. J. (2020). Deletion of metal transporter Zip14 (Slc39a14) produces skeletal muscle wasting, endotoxemia, Mef2c activation and induction of miR-675 and Hspb7. Sci. Rep. 10, 4050. doi:10.1038/s41598-020-61059-2
Kim, J-Y., Jackman, J. G., Woodring, S., McSherry, F., Herndon, J. E., Desjardins, A., et al. (2019). Second primary cancers in long-term survivors of glioblastoma. Neurooncol. Pract. 6, 386–391. doi:10.1093/nop/npz001
Larsson, L., Degens, H., Li, M., Salviati, L., Lee, Y., Thompson, W., et al. (2019). Sarcopenia: Aging-Related loss of muscle mass and function. Physiol. Rev. 99, 427–511. doi:10.1152/physrev.00061.2017
Li, L., Wang, N., Xiong, Y., Guo, G., Zhu, M., and Gu, Y. (2022). Transcription factor FOSL1 enhances drug resistance of breast cancer through DUSP7-mediated dephosphorylation of PEA15. Mol. Cancer Res. 20, 515–526. doi:10.1158/1541-7786.MCR-21-0658
Li, L., Xiao, F., Liu, G., and Chen, Y. (2022). Serum exosomal lncRNA AC007099.1 regulates the expression of neuropeptide-related FAP, as a potential biomarker for hepatocarcinogenesis. Dis. Markers 9501008, 1–13. doi:10.1155/2022/9501008
Li, Y., Duan, Q., Gan, L., Li, W., Yang, J., and Huang, G. (2021). microRNA-27b inhibits cell proliferation and invasion in bladder cancer by targeting engrailed-2. Biosci. Rep. 41, BSR20201000. doi:10.1042/BSR20201000
Li, Y., Liu, J., Xiao, Q., Tian, R., Zhou, Z., Gan, Y., et al. (2020). EN2 as an oncogene promotes tumor progression via regulating CCL20 in colorectal cancer. Cell Death Dis. 11, 604. doi:10.1038/s41419-020-02804-3
Lin, X., Liu, X., and Gong, C. (2018). Expression of engrailed homeobox 2 regulates the proliferation, migration and invasion of non-small cell lung cancer cells. Oncol. Lett. 16, 536–542. doi:10.3892/ol.2018.8693
Liu, K., Tian, T., Zheng, Y., Zhou, L., Dai, C., Wang, M., et al. (2019). Scutellarin inhibits proliferation and invasion of hepatocellular carcinoma cells via down-regulation of JAK2/STAT3 pathway. J. Cell. Mol. Med. 23, 3040–3044. doi:10.1111/jcmm.14169
Liu, S-C., Huang, C-M., Bamodu, O. A., Lin, C-S., Liu, B-L., Tzeng, Y-M., et al. (2019). Ovatodiolide suppresses nasopharyngeal cancer by targeting stem cell-like population, inducing apoptosis, inhibiting EMT and dysregulating JAK/STAT signaling pathway. Phytomedicine 56, 269–278. doi:10.1016/j.phymed.2018.05.007
Liu, Y., Yue, M., and Li, Z. (2021). FOSL1 promotes tumorigenesis in colorectal carcinoma by mediating the FBXL2/Wnt/β-catenin axis via Smurf1. Pharmacol. Res. 165, 105405. doi:10.1016/j.phrs.2020.105405
Louis, D. N., Perry, A., Reifenberger, G., von Deimling, A., Figarella-Branger, D., Cavenee, W. K., et al. (2016). The 2016 world health organization classification of tumors of the central nervous system: A summary. Acta Neuropathol. 131, 803–820. doi:10.1007/s00401-016-1545-1
Luo, Y-Z., He, P., and Qiu, M-X. (2018). FOSL1 enhances growth and metastasis of human prostate cancer cells through epithelial mesenchymal transition pathway. Eur. Rev. Med. Pharmacol. Sci. 22, 8609–8615. doi:10.26355/eurrev_201812_16624
Magnus, N., D’Asti, E., Meehan, B., Garnier, D., and Rak, J. (2014). Oncogenes and the coagulation system – forces that modulate dormant and aggressive states in cancer. Thromb. Res. 133, S1–S9. doi:10.1016/S0049-3848(14)50001-1
Matsuo, K., Owens, J. M., Tonko, M., Elliott, C., Chambers, T. J., and Wagner, E. F. (2000). Fosl1 is a transcriptional target of c-Fos during osteoclast differentiation. Nat. Genet. 24, 184–187. doi:10.1038/72855
McKinnon, C., Nandhabalan, M., Murray, S. A., and Plaha, P. (2021). Glioblastoma: Clinical presentation, diagnosis, and management. BMJ 374, n1560. doi:10.1136/bmj.n1560
Mi, E., Mauricaite, R., Pakzad-Shahabi, L., Chen, J., Ho, A., and Williams, M. (2022). Deep learning-based quantification of temporalis muscle has prognostic value in patients with glioblastoma. Br. J. Cancer 126, 196–203. doi:10.1038/s41416-021-01590-9
Miller, K. D., Ostrom, Q. T., Kruchko, C., Patil, N., Tihan, T., Cioffi, G., et al. (2021). Brain and other central nervous system tumor statistics, 2021. Ca. Cancer J. Clin. 71, 381–406. doi:10.3322/caac.21693
Muglia, R., Simonelli, M., Pessina, F., Morenghi, E., Navarria, P., Persico, P., et al. (2021). Prognostic relevance of temporal muscle thickness as a marker of sarcopenia in patients with glioblastoma at diagnosis. Eur. Radiol. 31, 4079–4086. doi:10.1007/s00330-020-07471-8
Ostrom, Q. T., Bauchet, L., Davis, F. G., Deltour, I., Fisher, J. L., Langer, C. E., et al. (2014). The epidemiology of glioma in adults: A “state of the science” review. Neuro. Oncol. 16, 896–913. doi:10.1093/neuonc/nou087
Ostrom, Q. T., Gittleman, H., Truitt, G., Boscia, A., Kruchko, C., and Barnholtz-Sloan, J. S. (2018). CBTRUS Statistical Report: Primary Brain and Other Central Nervous System Tumors Diagnosed in the United States in 2011–2015, 20, iv1–iv86. doi:10.1093/neuonc/noy131Neuro-Oncology
Ozawa, M., Brennan, P. M., Zienius, K., Kurian, K. M., Hollingworth, W., Weller, D., et al. (2019). The usefulness of symptoms alone or combined for general practitioners in considering the diagnosis of a brain tumour: A case-control study using the clinical practice research database (CPRD) (2000-2014). BMJ Open 9, e029686. doi:10.1136/bmjopen-2019-029686
Podkalicka, P., Mucha, O., Kaziród, K., Szade, K., Stępniewski, J., Ivanishchuk, L., et al. (2022). miR-378 affects metabolic disturbances in the mdx model of Duchenne muscular dystrophy. Sci. Rep. 12, 3945. doi:10.1038/s41598-022-07868-z
Rasmussen, B. K., Hansen, S., Laursen, R. J., Kosteljanetz, M., Schultz, H., Nørgård, B. M., et al. (2017). Epidemiology of glioma: Clinical characteristics, symptoms, and predictors of glioma patients grade I–iv in the the Danish neuro-oncology registry. J. Neurooncol. 135, 571–579. doi:10.1007/s11060-017-2607-5
Schmoldt, A., Benthe, H. F., and Haberland, G. (1975). Digitoxin metabolism by rat liver microsomes. Biochem. Pharmacol. 24, 1639–1641. doi:10.1016/0006-2952(75)90094-5
Sobolev, V. V., Khashukoeva, A. Z., Evina, O. E., Geppe, N. A., Chebysheva, S. N., Korsunskaya, I. M., et al. (2022). Role of the transcription factor FOSL1 in organ development and tumorigenesis. Int. J. Mol. Sci. 23, 1521. doi:10.3390/ijms23031521
Stummer, W., Meinel, T., Ewelt, C., Martus, P., Jakobs, O., Felsberg, J., et al. (2012). Prospective cohort study of radiotherapy with concomitant and adjuvant temozolomide chemotherapy for glioblastoma patients with no or minimal residual enhancing tumor load after surgery. J. Neurooncol. 108, 89–97. doi:10.1007/s11060-012-0798-3
Sun, Y., Li, Y., Wang, H., Li, H., Liu, S., Chen, J., et al. (2017). miR-146a-5p acts as a negative regulator of TGF-β signaling in skeletal muscle after acute contusion. Acta Biochim. Biophys. Sin. 49, 628–634. doi:10.1093/abbs/gmx052
Tan, A. C., Ashley, D. M., López, G. Y., Malinzak, M., Friedman, H. S., and Khasraw, M. (2020). Management of glioblastoma: State of the art and future directions. Ca. Cancer J. Clin. 70, 299–312. doi:10.3322/caac.21613
Vallejo, A., Perurena, N., Guruceaga, E., Mazur, P. K., Martinez-Canarias, S., Zandueta, C., et al. (2017). An integrative approach unveils FOSL1 as an oncogene vulnerability in KRAS-driven lung and pancreatic cancer. Nat. Commun. 8, 14294. doi:10.1038/ncomms14294
Wang, H., Sun, T., Hu, J., Zhang, R., Rao, Y., Wang, S., et al. (2014). miR-33a promotes glioma-initiating cell self-renewal via PKA and NOTCH pathways. J. Clin. Invest. 124, 4489–4502. doi:10.1172/JCI75284
Wang, T., Liu, G., Guo, X., and Ji, W. (2022). Single-cell analysis reveals the role of the neuropeptide receptor FPR2 in monocytes in kawasaki disease: A bioinformatic study. Dis. Markers 1666240, 1–15. doi:10.1155/2022/1666240
Wang, Y., Zhao, Z-J., Kang, X-R., Bian, T., Shen, Z-M., Jiang, Y., et al. (2020). lncRNA DLEU2 acts as a miR-181a sponge to regulate SEPP1 and inhibit skeletal muscle differentiation and regeneration. Aging (Albany NY) 12, 24033–24056. doi:10.18632/aging.104095
Wen, P. Y., Weller, M., Lee, E. Q., Alexander, B. M., Barnholtz-Sloan, J. S., Barthel, F. P., et al. (2020). Glioblastoma in adults: A society for neuro-oncology (SNO) and European society of neuro-oncology (EANO) consensus review on current management and future directions. Neuro. Oncol. 22, 1073–1113. doi:10.1093/neuonc/noaa106
Wesseling, P., and Capper, D. (2018). WHO 2016 Classification of gliomas. Neuropathol. Appl. Neurobiol. 44, 139–150. doi:10.1111/nan.12432
Williams, G. R., Dunne, R. F., Giri, S., Shachar, S. S., and Caan, B. J. (2021). Sarcopenia in the older adult with cancer. J. Clin. Oncol. 39, 2068–2078. doi:10.1200/JCO.21.00102
Xia, L., Zhao, R., Wan, Q., Wu, Y., Zhou, Y., Wang, Y., et al. (2020). Sarcopenia and adverse health-related outcomes: An umbrella review of meta-analyses of observational studies. Cancer Med. 9, 7964–7978. doi:10.1002/cam4.3428
Xu, H., Jin, X., Yuan, Y., Deng, P., Jiang, L., Zeng, X., et al. (2017). Prognostic value from integrative analysis of transcription factors c-jun and fra-1 in oral squamous cell carcinoma: A multicenter cohort study. Sci. Rep. 7, 7522. doi:10.1038/s41598-017-05106-5
Yu, M., Bardia, A., Wittner, B. S., Stott, S. L., Smas, M. E., Ting, D. T., et al. (2013). Circulating breast tumor cells exhibit dynamic changes in epithelial and mesenchymal composition. Science 339, 580–584. doi:10.1126/science.1228522
Zeng, S., Zhou, C., Yang, D-H., Xu, L-S., Yang, H-J., Xu, M-H., et al. (2020). LEF1-AS1 is implicated in the malignant development of glioblastoma via sponging miR-543 to upregulate EN2. Brain Res. 1736, 146781. doi:10.1016/j.brainres.2020.146781
Zhang, Y., Kong, X., Zhang, J., and Wang, X. (2022). Functional analysis of bronchopulmonary dysplasia-related neuropeptides in preterm infants and miRNA-based diagnostic model construction. Comput. Math. Methods Med. 5682599, 1–17. doi:10.1155/2022/5682599
Zhang, Y., Zhang, J., Sun, C., and Wu, F. (2022). Identification of the occurrence and potential mechanisms of heterotopic ossification associated with 17-beta-estradiol targeting MKX by bioinformatics analysis and cellular experiments. PeerJ 9, e12696. doi:10.7717/peerj.12696
Zhou, Q., Liu, J., Quan, J., Liu, W., Tan, H., and Li, W. (2018). MicroRNAs as potential biomarkers for the diagnosis of glioma: A systematic review and meta-analysis. Cancer Sci. 109, 2651–2659. doi:10.1111/cas.13714
Keywords: FOSL1, EN2, mir-33a, glioma, sarcopenia, muscle, systemic homeostasis-related genes
Citation: Wang W, Liu W, Xu J and Jin H (2022) MiR-33a targets FOSL1 and EN2 as a clinical prognostic marker for sarcopenia by glioma. Front. Genet. 13:953580. doi: 10.3389/fgene.2022.953580
Received: 26 May 2022; Accepted: 25 July 2022;
Published: 17 August 2022.
Edited by:
Qian Wang, Tai’an City Central Hospital, ChinaReviewed by:
Zhi-jie Zhao, Shanghai Jiao Tong University, ChinaCopyright © 2022 Wang, Liu, Xu and Jin. This is an open-access article distributed under the terms of the Creative Commons Attribution License (CC BY). The use, distribution or reproduction in other forums is permitted, provided the original author(s) and the copyright owner(s) are credited and that the original publication in this journal is cited, in accordance with accepted academic practice. No use, distribution or reproduction is permitted which does not comply with these terms.
*Correspondence: Hongze Jin, aHY4d2wzekAxNjMuY29t
†These authors have contributed equally to this work
Disclaimer: All claims expressed in this article are solely those of the authors and do not necessarily represent those of their affiliated organizations, or those of the publisher, the editors and the reviewers. Any product that may be evaluated in this article or claim that may be made by its manufacturer is not guaranteed or endorsed by the publisher.
Research integrity at Frontiers
Learn more about the work of our research integrity team to safeguard the quality of each article we publish.