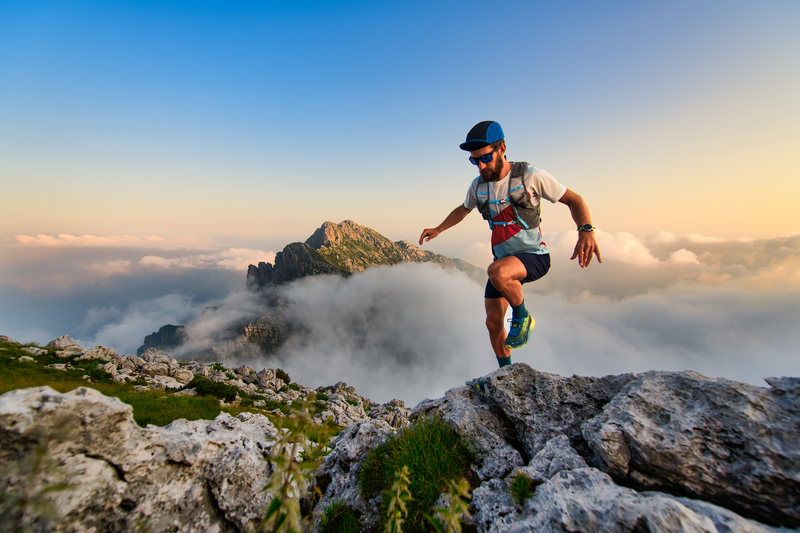
94% of researchers rate our articles as excellent or good
Learn more about the work of our research integrity team to safeguard the quality of each article we publish.
Find out more
ORIGINAL RESEARCH article
Front. Genet. , 23 August 2022
Sec. Human and Medical Genomics
Volume 13 - 2022 | https://doi.org/10.3389/fgene.2022.951939
This article is part of the Research Topic Adoption of Artificial Intelligence in Human and Clinical Genomics View all 11 articles
Introduction: Deep learning technology has been widely used in genetic research because of its characteristics of computability, statistical analysis, and predictability. Herein, we aimed to summarize standardized knowledge and potentially innovative approaches for deep learning applications of genetics by evaluating publications to encourage more research.
Methods: The Science Citation Index Expanded TM (SCIE) database was searched for deep learning applications for genomics-related publications. Original articles and reviews were considered. In this study, we derived a clustered network from 69,806 references that were cited by the 1,754 related manuscripts identified. We used CiteSpace and VOSviewer to identify countries, institutions, journals, co-cited references, keywords, subject evolution, path, current characteristics, and emerging topics.
Results: We assessed the rapidly increasing publications concerned about deep learning applications of genomics approaches and identified 1,754 articles that published reports focusing on this subject. Among these, a total of 101 countries and 2,487 institutes contributed publications, The United States of America had the most publications (728/1754) and the highest h-index, and the US has been in close collaborations with China and Germany. The reference clusters of SCI articles were clustered into seven categories: deep learning, logic regression, variant prioritization, random forests, scRNA-seq (single-cell RNA-seq), genomic regulation, and recombination. The keywords representing the research frontiers by year were prediction (2016–2021), sequence (2017–2021), mutation (2017–2021), and cancer (2019–2021).
Conclusion: Here, we summarized the current literature related to the status of deep learning for genetics applications and analyzed the current research characteristics and future trajectories in this field. This work aims to provide resources for possible further intensive exploration and encourages more researchers to overcome the research of deep learning applications in genetics.
Deep learning (DL) is a subfield of machine learning (ML) that aims to avoid extensive manual processing in traditional methods Wu et al., (2020). Different from machine learning, deep learning is a form of representation learning in which a machine is fed with raw data and develops its own representations needed for pattern recognition—which is composed of multiple layers of representations Esteva et al., (2019). The application of DL in medical healthcare has been widely reported. For example, DL has been reported to be successful in identifying a variety of histopathological features and detecting the biomarkers (Berrar and Dubitzky, 2021). DL has also been applied to predict diagnosis, prognosis, and treatment response in certain cancers Tran et al., (2021). This information could prove valuable in clinical decision-making for cancer treatment and triage for in-depth sequencing.
Genomic data had served as a biomarker for the onset and progression of the disease. Various deep learning applications in genomics had been reported, such as predicting gene expression from genotype data and studying the splicing-code model and the identification of long noncoding RNAs (Tripathi et al., 2016; Xie et al., 2017; Vellido, 2020; Tang et al., 2021). Recent advances in deep learning have emerged in several applications, ranging from natural language to vision processing Zou et al., (2019). Bibliometric approaches have generated a considerable impact on the deep learning research field, such as deep learning networks in identifying medical images and histopathology images for breast cancer classification (Khairi et al., 2021; Wang et al., 2022). However, gaps exist for deep learning in genetics research, and there is a dearth of information on associated bibliometric development trends. Therefore, based on deep learning technology advancements in genetics, a comprehensive bibliometric overview is required to provide researchers with new future research directions.
In the present study, we accessed the Web of Science Core Collection (WoSCC) using bibliometric methods to review and select deep learning studies in genetics research from 2000 to 2021. Specifically, co-word biclustering analysis was utilized to identify the research hot spots of the application of DL in genomics research. We hope this article can provide some reference for future research on deep learning and genomics research.
On 22 December 2021, we downloaded data from the Web of Science Core Collection (WoSCC); two authors independently verified citations and retrieved studies. The WoSCC is a frequently used authoritative database for scientific information, from which we generated a clustered network of 69,806 references cited by 1,754 studies. Between the publication years 2000 and 2021, literature searches were performed using the search terms: [TS = (“deep learning” OR “machine learning” OR “convolutional neural network*” OR CNN* OR RNN OR “Recurrent neural network*” OR “Fully Convolutional Network*” OR FCN*)], and The literature type = “Article OR Review OR Opening Online”, WoS category = Genetic heredity. Information on the following topics was collected: title, abstract, authors, institution, country/region, journal, keywords, and references. Articles were indexed in the WoSCC and excluded meeting articles, repeated articles, proceedings articles, book chapters, and unpublished documents without enough information for further analysis at the same time.
We described publication characteristics, including institutes, countries, journals, and keywords. The Journal of Citation Reports (JCR, 2021 version) was accessed to identify impact factors that reflected the scientific value of research (Eyre-Walker and Stoletzki, 2013). Retrieved data were analyzed in VOS viewer (Leiden University, Leiden, Holland) and CiteSpace V (Drexel University, Philadelphia, PA, United States), which facilitated collaborative network analyses connecting different publication characteristics (Chen, 2006; Chen, 2017). From the analysis and measures above, we obtain the current characteristics, research hotspot, subject evolution path, and future trajectories in deep learning applications of genetics.
A total of 1,754 articles from 2000 to 2021 were published. As shown in Figure 1, the line with points denoted by square shows the trend of publications from 2000 to 2021, and the line with points denoted by circles shows the number of articles published each year. The number of published articles showed a rapid increase since 2018, and more than 70% of the total articles were published in the last 4 years. This suggests that the studies of deep learning applied in genetics research were new research hot points in recent years.
FIGURE 1. Number of published articles about deep learning application in genetics research from 2000 to 2021.
A total of 101 countries and 2,487 institutes contributed publications. The top 10 countries, institutions, and cited journals are listed in Table 1. 728 (41.5%) articles published in the United States ranked first place, which was 18.3% higher than those in China, whose publication number was 407 (23.2%), thereby ranking second. However, it is worth noting that the research institution with the largest number (41 articles) of published articles was the Chinese Academy of Sciences, which indicated this institution had powerful scientific research ability in the field of deep learning application in genetics research. The collaborations between different countries and institutions are shown in Figures 2, 3. The bigger size of the circle represents the larger number of articles published by this country. The shorter the distance between two circles, the better the cooperation between the two countries. As shown in Figure 2, the biggest circle belongs to the United States of America which had close cooperation with Germany, England, and France. Although the Chinese Academy of Sciences published the largest number of articles, it was lack of cooperation with other institutions. Harvard Medical School was in a key position in this study field, which kept close cooperation with multiple institutions, such as Columbia University and Stanford University (shown in Figure 3).
FIGURE 2. Cooperation of countries in the field of Deep Learning application in genetics research from 2000 to 2021.
FIGURE 3. Cooperation of institutions contributed to publications for Deep Learning applications in genetic research.
A total of 151 cited journals published publications related to deep learning in genetics research. The top 10 cited journals are presented in Table 1(with green background). The highest cited count belonged to the BIOINFORMATICS (1,175 times), followed by NATURE (1,082 times). Among these journals, NATURE had the highest impact factor (49.962). Collaborations among these cited journals are shown in Figure 4.
FIGURE 4. Network map of cited journals in the field of Deep Learning application in genetics research from 2000 to 2021.
In academic journals, referential relationships facilitate knowledge exchange within the research field, where citing articles form the knowledge frontier and cited articles form the knowledge base. A journal dual-map overlay is shown in Figure 5. The cluster analysis of citing articles (the left side) belongs to journals focusing on the field of molecular/biology/immunology research. Also, the cluster analysis of cited articles (the right side) belongs to journals focusing on the field of molecular/biology/genetics research. The primary citation path colored orange represents the citation relationship between the two clusters, which indicated that based on genetics research, deep learning tends to be applied to immunology.
FIGURE 5. Dual-map overlay of journals in the field of Deep Learning application in genetics research from 2000 to 2021.
References are key bibliometric indicators as frequently cited documents can greatly influence their research areas (Table 2). The article was published on Nature which was cited 89 times, ranking first. Summarizing the highly cited topics, the result indicated that deep learning methods such as deep convolutional nets and recurrent nets have dramatically improved drug discovery and genomics research.
In network research, betweenness centrality is a major indicator to determine the importance of nodes in the network, and a higher betweenness centrality means that the literature is more important Synnestvedt et al., (2005). Table 2 also shows the betweenness centrality of these works of literature.
In this article, a co-cited document-based clustering analysis can be used to generate sub-fields and connect nodes in the research. We constructed a network of co-cited references to test the scientific relevance of related publications (Figure 6). Cluster setting parameters were Top N% = 0.5, #Years Per Slice = 3, and the pruning algorithm was chosen. The Modularity Q score = 0.7197, which was > 0.5, indicated the network adopted loosely coupled clusters. The Weighted Mean silhouette score = 0.9185, which was > 0.5, indicated acceptable cluster homogeneity. From the literature, we used index items as cluster markers (#0–#6); the largest cluster (#0) was “deep learning”, #1 was “logic regression”, #2 was “variant prioritization”, #3 was “random forests”, #4 was “scRNA-seq”, #5 was “genomic regulation”, and #6 was “recombination”.
We extracted and analyzed keyword co-occurrence in related publications. The top 20, with highly linked strengths, are shown in Table 3. The co-occurrence of any two terms indicates their presence in the same publication. While identifying thematics in research areas, keyword analyses of articles 1754) identified 27 keywords with a minimum of 40 occurrences (Figure 7). The co-occurrence analysis based on author keywords was built with occurrence times as a threshold. There are several distinct clusters with different colors. The co-occurrence network map of keywords is shown in Figure 7, given that the larger the size of the circle, the higher the co-occurrence of keywords. Furthermore, having closer keywords together shows a stronger relationship. The average year of publication of the keywords was determined using colors. Machine learning, deep learning, and genetics constitute the largest circle of all keywords that are identified through co-occurrence analysis. Our study also investigated temporal trends in hotspot shifts using the top 19 keywords having the strongest citation bursts. These included prediction (2016–2021), sequence (2017–2021), mutation (2017–2021), and cancer (2019-2021) (Figure 8).
Between the publication years 2000–2021, we selected and investigated 1754 SCIE articles related to deep learning in genetics. Since 2015 with the development of gene sequencing technology, biological genetic data have exploded. The number of published articles showed a rapid increase. Another growth time node is 2018, more than 70% of the total articles were published in the last 4 years. This suggests that as deep learning technology enters its mature stage, it has attracted widespread attention. The highest number of studies (728) was generated by the United States, with China in second place at 407. The top ten institutions included seven in the United States and two in China. According to our data, most of the research in deep learning in genetics was produced by institutions and countries in developed countries, such as United States, Germany, and France. The reason for this trend is that better socioeconomic development can be the premise of ensuring adequate funding, resources, and human input to explore brand new scientific research. Socioeconomic factors such as GDP, GDP per capita, research and development funding, number of researchers, number of physicians, or international collaboration are important decisive factors of scientific productivity. There are many reasons for this trend, such as GDP level Nature Genetics was the most frequently used publishing journal; therefore, it significantly contributed to research in this area. Additionally, we investigated the top 10 cited publications; the top-cited article was published by Lec et al. on Nature and was cited 89 times. These high cited articles will shed some light to this research field.
In previous studies, different deep learning research applications have been investigated in genetics and generated significant results. As indicated (Figure 6), after clustering co-cited references, key clustering nodes successfully identified knowledge bases, namely: #0 “deep learning”, #1 “logic regression”, #2 was marked as “variant prioritization”, #3 was marked as “random forests”, cluster #4 was marked as “scrna-seq”, cluster #5 was marked as “genomic regulation”, and cluster #6 was marked as “recombination”. We described the knowledge base according to different clusters with time characteristics.
In the #0 “deep learning” cluster, applications of deep learning methods show cutting-edge performance in a variety of complex prediction tasks and large datasets in natural images. Scientists propose a deep-learning framework for genetic research events, e.g., distant metastasis in cancer, protein subcellular localization, genome recombination map of African Drosophila melanogaster, and DNA transcription factor binding; the abovementioned aspects show the advantages (Xiao et al., 2019; Adrion et al., 2020; Zhang et al., 2021a; Chereda et al., 2021).
In the #1 “logic regression” cluster, Liu et al. proposed a logic regression-based approach that was used to analyze the gene–gene interaction of eight genes involved in cell adhesion in 806 NSCL/P Chinese case-parent triad recruited to explore the risk of non-syndromic cleft lip Liu et al., (2019). Nicodemus KK’s team tested and discussed the interactions between these susceptibility genes using four machine learning algorithms (including random forest, generalized enhanced regression, and Monte Carlo logistic regression) in a case-control study of schizophrenia Nicodemus et al., (2010). Dasgupta et al. reviewed machine learning and regression-based methods in 200 common or rare genetic variants from exome sequencing data and discussed cross-validation for model assessment and selection Dasgupta et al., (2011).
In the #2 “variant prioritization” cluster, key challenges in genomics research are variant prioritization methods. Huang et al. identified a deep learning framework, which was evolution-based, for unified variant and gene prioritization. The authors integrated constraints predicting missense variants and protein-coding genes associated with dominant disorders and estimated fitness effects for potential single-nucleotide variants, which outperformed current methods Huang, (2020). Zhang et al. formulated a disease-specific variant classifier that assessed discriminate pathogenic variants from benign variants and prioritized disease-associated variants Zhang et al., (2021b). In their study, Mattia et al. proposed an automated computational framework that identified causal genetic variants (small insertions and deletions and coding/splicing single-nucleotide variants) to improve causal variant prioritization methods and variant pathogenicity classifications Bosio et al., (2019).
For the #3 “random forest” cluster, as a standard regression model, which has been widely used in the machine learning (ML) application, Jian Y’s team applied a random forest machine learning algorithm to purity pediatric children central nervous system tumor analysis, which helps with the clinical management of pediatrics Yang et al., ( 2021). Chen Z constructed a deep learning network model based on the random forest classifier, and it can easily identify malonylation sites, for predicting sites shows high confidence Chen et al., (2018). Nicholls et al. reviewed ML model (gradient boosting and random forests) applications, dissected variant and gene signal heterogeneity, prioritized complex disease-associated loci, and critically evaluated prioritization issues for genome-wide association investigations Nicholls et al., (2020).
For the #4 “scRNA-seq” cluster, as single-cell RNA-sequencing (scRNA-seq) is used to analyze gene expression with high resolution, scientists have comprehensively exploited this area to dissect individual cell types in several diseases. For example, Carlos et al. generated a Deep Neural Network (DNN) model which quantified immune infiltration levels in breast and colorectal cancer bulk RNA-seq samples and identified improved and accurate survival prediction and quantification data (Torroja and Sanchez-CaboDigitaldlsorter, 2019). Cédric et al., in an effort to accommodate increasing levels of scRNA-seq data, designed a deep neural network–based imputation algorithm that is more suitable for the ever-increasing scRNA-seq data (Arisdakessian et al., 2019). Additionally, Yao and Nelson’s teams generated unsupervised deep learning methods for improved data integration which showed improved performances in scRNA-seq datasets (Johansen and Quon, 2019; He et al., 2020).
For the #5 “gene regulation” cluster, an ML modality was adapted by Colbran et al. to impute gene regulation information from genotype data and investigate 490 ancient Eurasian human DNA samples and explore divergent gene regulation mechanisms which contributed to skin pigmentation and metabolic and immune functions. The authors identified gene regulation roles in adaptation and associations between complex traits and genetic diversity Colbran et al., (2021). Atak et al. devised a deep learning approach and integrative genomics strategy to analyze functional enhancer mutations with allelic imbalance of gene expression and chromatin accessibility and successfully interpreted and predicted the impact of a mutation on gene regulation Atak et al., (2021). Godwin et al. devised a deep learning–based model to predict the gene regulatory effects of low-molecular-weight compounds; the model potentially identified drug candidates inducing particular gene responses, without prior interactional information on protein targets Woo et al., (2020).
For the #6 “recombination” cluster, a central tenet of genomics is the accurate assessment of genome-wide recombination rates in natural populations. Andrew et al. used ML algorithms to examine if DNA motifs across the genome could be used to predict crossover variation and identify genetic factors influencing variation in recombination rates Adrian et al., (2016). Kha F proposed a DL intelligent computational predictor based on the deep neural network (DNN) as a classification engine for the identification of recombination spots through an experimental benchmark dataset with 10-fold cross-validation which achieved the 95.81% highest accuracy Khan et al., (2020).
Keywords concentrate on contemporary research issues or concepts, while burst keywords represent emerging trends and frontiers in research. In our work, we used CiteSpace to capture burst keywords, and four related research frontiers were identified: four keywords with the strongest citation bursts, such as prediction (2016–2021), sequence (2017–2021), mutation (2017–2021), cancer (2019–2021), and these key words cover the research frontier of the current topic.
Large-scale genetic datasets and deep-learning approaches are increasingly exploited by bioinformatics approaches to model protein structures and complexes. Zhao et al., using sequence information, devised a deep forest-based protein location algorithm to accurately predict protein subcellular locations using only protein sequences, which outperformed contemporary state-of-art algorithms Zhao et al., (2018). Cui et al. analyzed the main methods used to represent protein sequence data, theoretically reviewed the architecture of different embedding models, and investigated the development of these sequence-embedding approaches Cui et al., (2021). Braberg et al. analyzed the emergence of large-scale genetic datasets and deep learning approaches which modeled protein structures and associated interactions (deep mutational scanning, genome-scale genetic or chemical-genetic interaction mapping, and coevolution) and discussed structural data integration from different sources Braberg et al., (2022).
Originally used for image processing and pattern recognition methods, deep learning models are now used to detect genetic alterations in cancer and determine cancer patient prognoses. The framework by Mallik et al. integrated linear regression, differential expression, and deep learning and facilitated the robust interpretation of DNA methylation signatures and gene expression data for cervical cancer Mallik et al., (2020). Poirion et al., using multi-omics data, established a deep learning ensemble network that predicted patient survival subtypes Poirion et al., (2021). In order to predict survival outcomes in cancer patients, Huang et al. broadly analyzed The Cancer Genome Atlas cancers using several deep learning–based models Huang et al., (2020). Tran et al. reviewed emerging deep learning approaches and how they were applied to precision oncology. The authors not only exemplified how deep learning was used for cancer diagnostics, prognostics, and treatment management strategies, but they also reviewed the current limitations and challenges of deep learning in this area Vaernet, (1972).
With considerable high-throughput technology advancements, somatic mutations in their millions have been reported, but critically, the identification of specific driver genes expressing oncogenic mutations is highly challenging and complex. In their study, Luo et al. used “deep drive” to predict driver genes by combining similarity networks with features that characterize the functional impact of mutations. They use AUC scores to evaluate predictive efficiency. DeepDriver achieved AUC scores of 0.984 and 0.976 on breast cancer and colorectal cancer, respectively, which were better than those of the competing algorithms. Luo et al., (2019) Sahraeian et al. inaugurated a deep convolutional neural network–based somatic mutation detection strategy using high-confidence somatic mutations in a cancer cell line. The authors generated comprehensive models using multiple datasets and highly robust and significantly superior methods when compared with traditional detection strategies Sahraeian et al., (2022).
Ding YL provided a comprehensive review of ML-based approaches for predicting disease–biomolecule associations with multi-view data sources. They discussed feature representation methods and provided some perspectives for further improving biomolecule-disease prediction methods (Ding et al., 2021). Groschel MI presented a translational genomics platform for tuberculosis application to predict antibiotic resistance from next-generation sequence data. After benchmarking, it can rapidly and accurately predict resistance to anti-tuberculosis drugs Gröschel et al., (2021). Majumdar A et al. developed a novelty ensemble support vector regression to predict each drug response value for a single patient based on cell-line gene expression data. This can be used to develop a robust drug response prediction system for cancer patients using cancer cell lines guidance and multi-omics data (Majumdar et al., 2021).
Our study still has some limitations to be addressed. First, we choose the SCIE database as the collection, while a few studies not included in the core collection were missed. Second, this study includes two types of publication (article and review), and the uneven quality of the collected publications may reduce the credibility of the mapping analysis. However, the visualized analysis based on bibliometric analysis undoubtedly lays a foundation for readers to quickly understand the research subjects, hotspots, and development trends in an unfamiliar research field.
Using bibliometrics, we systematically, comprehensively, and objectively investigated the literature related to deep learning applications in genetics research. Importantly, we identified research bases, current hotspots, and future trends in this area. The knowledge bases were “deep learning,” logic regression,” “variant prioritization,” “random forests,” “scRNA-seq,” “genomic regulation,” and “recombination”. We also provided hotspot and frontier guidance for researchers wishing to conduct advanced genetics research in the future. We identified research frontiers and emerging trends topics that incorporated prediction, sequence, mutation, and cancer. Finally, some studies selected for this research were not comprehensive and may have generated publication bias, thereby potentially affecting the study outcomes of this bibliometric review.
The original contributions presented in the study are included in the article/Supplementary Material; further inquiries can be directed to the corresponding author.
Author TF and BZ make the conception and design of this work. Author BZ was responsible for making the acquisition, analysis, interpretation of the data, and drafting the manuscript. TF designed the study and revised the article. All the authors read and approved the final manuscript.
This study was supported by the 345 Talent Project of Shengjing Hospital (M0347) and “ Research on the application of genetic big data analysis in the prevention and control of newborn birth defects” (2900021013-CMU-013).
The authors declare that the research was conducted in the absence of any commercial or financial relationships that could be construed as a potential conflict of interest.
All claims expressed in this article are solely those of the authors and do not necessarily represent those of their affiliated organizations, or those of the publisher, the editors, and the reviewers. Any product that may be evaluated in this article, or claim that may be made by its manufacturer, is not guaranteed or endorsed by the publisher.
DL, deep learning; ML, machine learning; CNNs, convolutional neural networks; DNN, deep neural network; scRNA-Seq, single-cell RNA sequencing; TCGA, The Cancer Genome Atlas; NSCL/P, nonsyndromic cleft lip with or without cleft palate.
Adrian, A. B., Corchado, J. C., and Comeron, J. M. (2016). Predictive models of recombination rate variation across the Drosophila melanogaster genome. Genome Biol. Evol. 8, 2597–2612. doi:10.1093/gbe/evw181
Adrion, J. R., Galloway, J. G., and Kern, A. D. (2020). Predicting the landscape of recombination using deep learning. Mol. Biol. Evol. 37, 1790–1808. doi:10.1093/molbev/msaa038
Arisdakessian, C., Poirion, O., Yunits, B., Zhu, X., and Garmire, L. X. (2019). DeepImpute: An accurate, fast, and scalable deep neural network method to impute single-cell RNA-seq data. Genome Biol. 20, 211. doi:10.1186/s13059-019-1837-6
Atak, Z. K., Taskiran, I. I., Demeulemeester, J., Flerin, C., Mauduit, D., Minnoye, L., et al. (2021). Interpretation of allele-specific chromatin accessibility using cell state-aware deep learning. Genome Res. 31, 1082–1096. doi:10.1101/gr.260851.120
Berrar, D., and Dubitzky, W. (2021). Deep learning in bioinformatics and biomedicine. Brief. Bioinform. 22 (2), 1513–1514. doi:10.1093/bib/bbab087
Bosio, M., Drechsel, O., Rahman, R., Muyas, F., Rabionet, R., Bezdan, D., et al. (2019). eDiVA-Classification and prioritization of pathogenic variants for clinical diagnostics. Hum. Mutat. 40, 865–878. doi:10.1002/humu.23772
Braberg, H., Echeverria, I., Kaake, R. M., Sali, A., and Krogan, N. J. (2022). From systems to structure - using genetic data to model protein structures. Nat. Rev. Genet. 23 (6), 342–354. doi:10.1038/s41576-021-00441-w
Chen, C. (2006). CiteSpace II: Detecting and visualizing emerging trends and transient patterns in scientific literature. J. Am. Soc. Inf. Sci. Technol. 57 (3), 359–377. doi:10.1002/asi.20317
Chen, C. (2017). Science mapping: A systematic review of the literature. J. Data Inf. Sci. 2, 1–40. doi:10.1515/jdis-2017-0006
Chen, Z., He, N., Huang, Y., Qin, W. T., Liu, X., Li, L., et al. (2018). Integration of A Deep learning classifier with A random forest approach for predicting malonylation sites. Genomics Proteomics Bioinforma. 16, 451–459. doi:10.1016/j.gpb.2018.08.004
Chereda, H., Bleckmann, A., Menck, K., Perera-Bel, J., Stegmaier, P., Auer, F., et al. (2021). Explaining decisions of graph convolutional neural networks: Patient-specific molecular subnetworks responsible for metastasis prediction in breast cancer. Genome Med. 13, 42. doi:10.1186/s13073-021-00845-7
Colbran, L. L., Johnson, M. R., Mathieson, I., and Capra, J. A. (2021). Tracing the evolution of human gene regulation and its association with shifts in environment. Genome Biol. Evol. 13 (11), evab237. doi:10.1093/gbe/evab237
Cui, F., Zhang, Z., and Zou, Q. (2021). Sequence representation approaches for sequence-based protein prediction tasks that use deep learning. Brief. Funct. Genomics 20, 61–73. doi:10.1093/bfgp/elaa030
Dasgupta, A., Sun, Y. V., König, I. R., Bailey-Wilson, J. E., and Malley, J. D. (2011). Brief review of regression-based and machine learning methods in genetic epidemiology: The genetic analysis workshop 17 experience. Genet. Epidemiol. 35 (1), S5–S11. doi:10.1002/gepi.20642
Ding, Y., Lei, X., Liao, B., and Wu, F. X. (2021). Machine learning approaches for predicting biomolecule-disease associations. Brief. Funct. Genomics 20 (4), 273–287. doi:10.1093/bfgp/elab002
Esteva, A., Robicquet, A., Ramsundar, B., Kuleshov, V., DePristo, M., Chou, K., et al. (2019). A guide to deep learning in healthcare. Nat. Med. 25 (1), 24–29. doi:10.1038/s41591-018-0316-z
Eyre-Walker, A., and Stoletzki, N. (2013). The assessment of science: The relative merits of post-publication review, the impact factor, and the number of citations. PLoS Biol. 11, e1001675. doi:10.1371/journal.pbio.1001675
Gröschel, M. I., Owens, M., Freschi, L., Vargas, R., Marin, M. G., Phelan, J., et al. (2021). GenTB: A user-friendly genome-based predictor for tuberculosis resistance powered by machine learning. Genome Med. 13 (1), 138. doi:10.1186/s13073-021-00953-4
He, Y., Yuan, H., Wu, C., and Xie, Z. (2020). DISC: A highly scalable and accurate inference of gene expression and structure for single-cell transcriptomes using semi-supervised deep learning. Genome Biol. 21, 170. doi:10.1186/s13059-020-02083-3
Huang, Y. F. (2020). Unified inference of missense variant effects and gene constraints in the human genome. PLoS Genet. 16, e1008922. doi:10.1371/journal.pgen.1008922
Huang, Z., Johnson, T. S., Han, Z., Helm, B., Cao, S., Zhang, C., et al. (2020). Deep learning-based cancer survival prognosis from RNA-seq data: Approaches and evaluations. BMC Med. Genomics 13, 41. doi:10.1186/s12920-020-0686-1
Johansen, N., and Quon, G. (2019). scAlign: a tool for alignment, integration, and rare cell identification from scRNA-seq data. Genome Biol. 20, 166. doi:10.1186/s13059-019-1766-4
Khairi, S. S. M., Bakar, M. A. A., Alias, M. A., Bakar, S. A., Liong, C.-Y., Rosli, N., et al. (2021). Deep learning on histopathology images for breast cancer classification: A bibliometric analysis. Healthcare 10, 10. doi:10.3390/healthcare10010010
Khan, F., Khan, M., Iqbal, N., Khan, S., Muhammad Khan, D., Khan, A., et al. (2020). Prediction of recombination spots using novel hybrid feature extraction method via deep learning approach. Front. Genet. 11, 539227. doi:10.3389/fgene.2020.539227
Liu, D., Wang, M., Yuan, Y., Schwender, H., Wang, H., Wang, P., et al. (2019). Gene-gene interaction among cell adhesion genes and risk of nonsyndromic cleft lip with or without cleft palate in Chinese case-parent trios. Mol. Genet. Genomic Med. 7, e00872. doi:10.1002/mgg3.872
Luo, P., Ding, Y., Lei, X., and Wu, F. X. (2019). deepDriver: Predicting cancer driver genes based on somatic mutations using deep convolutional neural networks. Front. Genet. 10, 13. doi:10.3389/fgene.2019.00013
Majumdar, A., Liu, Y., Lu, Y., Wu, S., and Cheng, L. (2021). kESVR: An ensemble model for drug response prediction in precision medicine using cancer cell lines gene expression. Genes. 12 (6), 844. doi:10.3390/genes12060844
Mallik, S., Seth, S., Bhadra, T., and Zhao, Z. (2020). A linear regression and deep learning approach for detecting reliable genetic alterations in cancer using DNA methylation and gene expression data. Genes. (Basel), 11: 931. doi:10.3390/genes11080931
Nicholls, H. L., John, C. R., Watson, D. S., Munroe, P. B., Barnes, M. R., Cabrera, C. P., et al. (2020). Reaching the end-game for GWAS: Machine learning approaches for the prioritization of complex disease loci. Front. Genet. 11, 350. doi:10.3389/fgene.2020.00350
Nicodemus, K. K., Callicott, J. H., Higier, R. G., Luna, A., Nixon, D. C., Lipska, B. K., et al. (2010). Evidence of statistical epistasis between DISC1, CIT and NDEL1 impacting risk for schizophrenia: Biological validation with functional neuroimaging. Hum. Genet. 127, 441–452. doi:10.1007/s00439-009-0782-y
Poirion, O. B., Jing, Z., Chaudhary, K., Huang, S., and Garmire, L. X. (2021). DeepProg: An ensemble of deep-learning and machine-learning models for prognosis prediction using multi-omics data. Genome Med. 13, 112. doi:10.1186/s13073-021-00930-x
Sahraeian, S., Fang, L. T., Karagiannis, K., Moos, M., Smith, S., Santana-Quintero, L., et al. (2022). Achieving robust somatic mutation detection with deep learning models derived from reference data sets of a cancer sample. Genome Biol. 23 (1), 12. doi:10.1186/s13059-021-02592-9
Synnestvedt, M. B., Chen, C., and Holmes, J. H. (2005). CiteSpace II: Visualization and knowledge discovery in bibliographic databases. AMIA Annu. Symp. Proc. 2005, 724–728.
Tang, X., Shi, Z., and Jin, M. (2021). Multi-category multi-state information ensemble-based classification method for precise diagnosis of three cancers. Neural Comput. Appl. 33, 15901–15917. doi:10.1007/s00521-021-06211-3
Torroja, C., Sanchez-Cabo, , and Digitaldlsorter, F. (2019). Digitaldlsorter: Deep-Learning on scRNA-seq to deconvolute gene expression data. Front. Genet. 10, 978. doi:10.3389/fgene.2019.00978
Tran, K. A., Kondrashova, O., Bradley, A., Williams, E. D., Pearson, J. V., Waddell, N., et al. (2021). Deep learning in cancer diagnosis, prognosis and treatment selection. Genome Med. 13 (1), 152. doi:10.1186/s13073-021-00968-x
Tripathi, R., Patel, S., Kumari, V., Chakraborty, P., and Varadwaj, P. K. (2016). DeepLNC, a long non-coding RNA prediction tool using deep neural network. Netw. Model. Anal. Health Inf. Bioinforma. 5, 21. doi:10.1007/s13721-016-0129-2
Vaernet, K. (1972). Stereotaxic amygdalotomy in temporal lobe epilepsy. Stereotact. Funct. Neurosurg. 34, 176–183. doi:10.1159/000103055
Vellido, A. (2020). The importance of interpretability and visualization in machine learning for applications in medicine and health care. Neural Comput. Appl. 32, 18069–18083. doi:10.1007/s00521-019-04051-w
Wang, L., Wang, H., Huang, Y., Yan, B., Chang, Z., and Liu, Z. (2022) Trends in the application of deep learning networks in medical image. Eur. J. Radiology 146, 110069. doi:10.1016/j.ejrad.2021.110069
Woo, G., Fernandez, M., Hsing, M., Lack, N. A., Cavga, A. D., Cherkasov, A. DeepC. O. P., et al. (2020). DeepCOP: Deep learning-based approach to predict gene regulating effects of small molecules. Bioinformatics 36, 813–818. doi:10.1093/bioinformatics/btz645
Wu, S., Roberts, K., Datta, S., Du, J., Ji, Z., Si, Y., et al. (2020). Deep learning in clinical natural language processing: A methodical review. J. Am. Med. Inf. Assoc. 27 (3), 457–470. doi:10.1093/jamia/ocz200
Xiao, M., Shen, X., and Pan, W. (2019). Application of deep convolutional neural networks in classification of protein subcellular localization with microscopy images. Genet. Epidemiol. 43, 330–341. doi:10.1002/gepi.22182
Xie, R., Wen, J., Quitadamo, A., Cheng, J., and Shi, X. (2017). A deep auto-encoder model for gene expression prediction. BMC Genomics 18 (9), 845. doi:10.1186/s12864-017-4226-0
Yang, J., Wang, J., Tian, S., Wang, Q., Zhao, Y., Wang, B., et al. (2021). An integrated analysis of tumor purity of common central nervous system tumors in children based on machine learning methods. Front. Genet. 12, 707802. doi:10.3389/fgene.2021.707802
Zhang, X., Walsh, R., Whiffin, N., Buchan, R., Midwinter, W., Wilk, A., et al. (2021). Disease-specific variant pathogenicity prediction significantly improves variant interpretation in inherited cardiac conditions. Genet. Med. 23, 69–79. doi:10.1038/s41436-020-00972-3
Zhang, Y., Mo, Q., Xue, L., and Luo, J. (2021). Evaluation of deep learning approaches for modeling transcription factor sequence specificity. Genomics 113, 3774–3781. doi:10.1016/j.ygeno.2021.09.009
Zhao, L., Wang, J., Nabil, M. M., and Zhang, J. (2018). Deep forest-based prediction of protein subcellular localization. Curr. Gene Ther. 18, 268–274. doi:10.2174/1566523218666180913110949
Keywords: deep learning, machine learning, genetics, bibliometric, knowledge graph
Citation: Zhang B and Fan T (2022) Knowledge structure and emerging trends in the application of deep learning in genetics research: A bibliometric analysis [2000–2021]. Front. Genet. 13:951939. doi: 10.3389/fgene.2022.951939
Received: 24 May 2022; Accepted: 13 July 2022;
Published: 23 August 2022.
Edited by:
Deepak Kumar Jain, Chongqing University of Posts and Telecommunications, ChinaReviewed by:
Ourlad Alzeus Gaddi Tantengco, University of the Philippines Manila, PhilippinesCopyright © 2022 Zhang and Fan. This is an open-access article distributed under the terms of the Creative Commons Attribution License (CC BY). The use, distribution or reproduction in other forums is permitted, provided the original author(s) and the copyright owner(s) are credited and that the original publication in this journal is cited, in accordance with accepted academic practice. No use, distribution or reproduction is permitted which does not comply with these terms.
*Correspondence: Ting Fan, dGZhbkBjbXUuZWR1LmNu
Disclaimer: All claims expressed in this article are solely those of the authors and do not necessarily represent those of their affiliated organizations, or those of the publisher, the editors and the reviewers. Any product that may be evaluated in this article or claim that may be made by its manufacturer is not guaranteed or endorsed by the publisher.
Research integrity at Frontiers
Learn more about the work of our research integrity team to safeguard the quality of each article we publish.