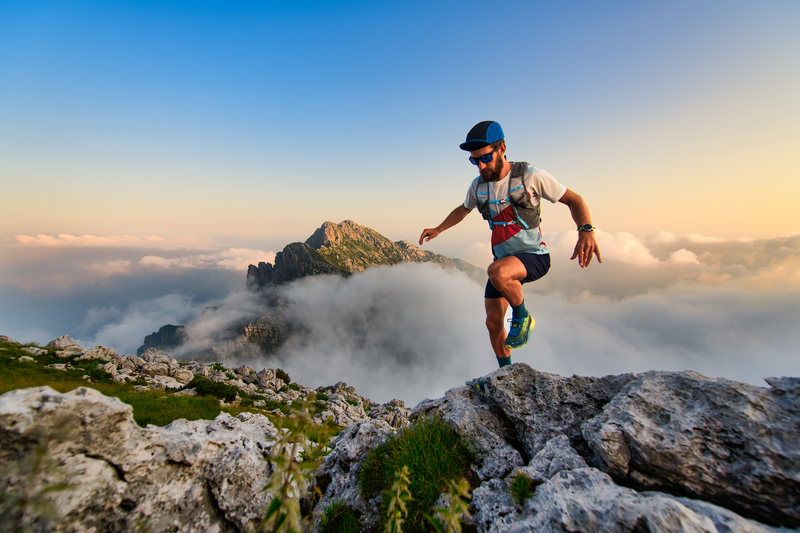
94% of researchers rate our articles as excellent or good
Learn more about the work of our research integrity team to safeguard the quality of each article we publish.
Find out more
BRIEF RESEARCH REPORT article
Front. Genet. , 09 November 2022
Sec. Computational Genomics
Volume 13 - 2022 | https://doi.org/10.3389/fgene.2022.944837
This article is part of the Research Topic Identifying Genetics-Based Mechanisms and Treatments for Neurodevelopmental and Psychiatric Disorders Through Data Integration View all 6 articles
At present, the neuronal mechanisms underlying the diagnosis of autism spectrum disorder (ASD) have not been established. However, studies from human postmortem ASD brains have consistently revealed disruptions in cerebellar circuitry, specifically reductions in Purkinje cell (PC) number and size. Alterations in cerebellar circuitry would have important implications for information processing within the cerebellum and affect a wide range of human motor and non-motor behaviors. Laser capture microdissection was performed to obtain pure PC populations from a cohort of postmortem control and ASD cases and transcriptional profiles were compared. The 427 differentially expressed genes were enriched for gene ontology biological processes related to developmental organization/connectivity, extracellular matrix organization, calcium ion response, immune function and PC signaling alterations. Given the complexity of PCs and their far-ranging roles in response to sensory stimuli and motor function regulation, understanding transcriptional differences in this subset of cerebellar cells in ASD may inform on convergent pathways that impact neuronal function.
A diagnosis of autism spectrum disorder (ASD) is accompanied by both gifts and challenges and the description of ASD as a “spectrum” acknowledges the heterogeneous presentation of symptoms across individuals. The American Psychiatric Association’s Diagnostic and Statistical Manual (DSM-5) provides standardized criteria that outline typical differences in behaviors that fall into the broad categories of social communication and interaction and restricted and repetitive behaviors (American Psychiatric Association, 2013). Within the category of restricted and repetitive behavior, sensorimotor challenges play a prominent role in diagnosis. For example, many individuals display stereotyped and repetitive motor movements that include hand flapping, rocking, pacing, spinning objects, lining up toys, echolalia, etc. that is often accompanied by hyper- or hyporeactivity to sensory input. Although attention is usually focused on the differences in complex behaviors of social communication, there is a large body of research that implicates alterations in fundamental motor patterns in ASD. Basic motor differences during development may translate into challenges with complex behaviors that rely on the acquisition of fundamental motor skills (for review, see: Subramanian et al., 2017). The underlying circuitry within the brain that is responsible for mediating such a diverse set of motor and cognitive behaviors has not been fully defined, however, there is growing consensus that the cerebellum plays a major role in ASD (Fatemi et al., 2012; Becker and Stoodley, 2013; Rogers et al., 2013; Wang et al., 2014; Hampson and Blatt, 2015). As knowledge of the cerebellum expands to include functional roles in non-motor domains as well as the typical motor-domains (Schmahmann, 2019), it is now clear that the cerebellum influences a wide range of behaviors relevant to the ASD phenotype.
Aside from the many cerebellar disorders that have comorbid ASD associated symptoms (Becker and Stoodley, 2013; Wang et al., 2014), alteration of cerebellar circuitry early in development is correlated with ASD (Hashimoto et al., 1995; Courchesne et al., 2001; Beversdorf et al., 2005; Limperopoulos et al., 2007). Cerebellar insult (prenatal stressors, infection, etc.) during development has an ASD risk ratio comparable to the risk seen with twin studies and the strongest genetic associations (i.e. highest-risk single genetic variants) (Wang et al., 2014). Furthermore, imaging studies repeatedly implicate the cerebellum in structural differences in ASD, where volumetric differences emerge in the early years of life, persist into adulthood and have been associated with language deficits (Scott et al., 2009; Becker and Stoodley, 2013; Wang et al., 2014; Hampson and Blatt, 2015).
Importantly, when unbiasedly assessing the resting-state functional connectome in ASD across the entire brain, the cerebellum emerged as the only region meeting stringent criteria for significant abnormal connectivity (Arnold Anteraper et al., 2019). These converging lines of evidence from behavioral, lesion and imaging studies implicate the cerebellum in the development of ASD and highlight the need for a deeper understanding of how the cerebellum mediates sensorimotor and cognitive behavior. This will likely be a crucial step toward developing support for ASD individuals and points to the need for understanding the specific cerebellar circuitry deficits that lead to the various reported differences.
Purkinje cells (PCs) are central to cerebellar function as the only output cells of the cerebellar cortex. Human postmortem studies have shown differences in PC number (Bauman and Kemper 1985; Bauman et al., 1995; Bailey et al., 1998; Whitney et al., 2008; Skefos et al., 2014), size (Fatemi et al., 2002) and gene expression (Yip et al., 2007; Yip et al., 2008; Soghomonian et al., 2017) in the ASD brain. PC dysfunction is the most consistent neuropathological finding to date, with as many as 75% of cases showing reductions in number (Schumann and Nordahl, 2011; Hampson and Blatt, 2015).
In ASD, many of the genes implicated by genome-wide association studies (GWAS) affect synaptic stability and adhesion molecules (Hussman et al., 2011; Lin et al., 2016). Since PCs have hundreds of thousands of synapses, making their dendritic branching the most complex in the brain, mutations in synaptic proteins and adhesion molecules may be particularly detrimental to PC signaling and survival. The abundant spines on PCs modify their shape and function in response to stimuli and have been shown to be involved in cerebellar motor learning (Kleim et al., 1998; Kim et al., 2002; Federmeier et al., 2002; Lee et al., 2005). Therefore, it’s possible their disruption can provide a mechanism behind the decreased numbers of PCs and alterations reported in human postmortem ASD studies.
An important study (Parikshak et al., 2016) compared transcriptome alterations between brain regions in ASD, concluding that the cortex is more vulnerable to transcript alterations than the cerebellum. However, it is possible that the heavily skewed proportion toward granule cell number as compared to PC number represented in bulk cerebellar tissue obscures differences in PC transcriptome profiles, as it is surprising there would be few transcriptional differences in a brain region as heavily implicated in ASD as the cerebellum. Until recently, most studies investigating altered gene expression in human postmortem brain tissue have been limited to heterogeneous cell populations acquired from whole tissues. However, laser capture microdissection has emerged as a viable method to extract single cell types from the brain (Murray, 2007; Chu et al., 2009; Pietersen et al., 2009; Cunnea et al., 2010; Greene et al., 2010; Pietersen et al., 2011; Simpson et al., 2011; Waller et al., 2012).
Given the numerous studies implicating PC pathophysiology in ASD, we used laser capture microdissection to isolate and extract PCs from the billions of other cells in the cerebellum and compared the transcriptional profile of PCs in ASD cases to controls. We identified 427 differentially expressed genes, which were enriched for gene ontology (GO) biological processes related to nervous system development, synaptic and cellular signaling, immune responses, extracellular matrix organization and MAPK signaling. The number of DEGs is consistent with those found in cortical studies (Parikshak et al., 2016), which highlights the importance of single-cell analysis in complex disorders. Similarly, single nucleus RNA sequencing of postmortem ASD brains in cortical regions resulted in 513 differentially expressed genes, in which transcripts of neurons were more impacted than that of glial cells (Velmeshev et al., 2019), however, metanalysis of single cell RNA sequencing data suggests glia may also be impacted in ASD (Nassir et al., 2021). The data presented provides evidence for disrupted neuronal function in mature cells while also suggesting that developmental processes and early connectivity mechanisms are impacted.
Human postmortem brain tissue was obtained from the University of Maryland Brain and Tissue Bank, a brain and tissue repository of the NIH Neurobiobank. Case demographics are provided in Table 1. All ASD cases had confirmed diagnoses through Autism Diagnostic Interview-Revised (ADI-R) scores and/or received a clinical diagnosis of autism from a licensed psychiatrist. Cases were age- and PMI-matched, but different race/ethnic backgrounds were taken as available. As this research did not involve live human subjects, Institutional Review Board approval and informed consent were not necessary. However, the University of Maryland Brain and Tissue Bank (NIH Neurobiobank) is overseen by Institutional Review Board protocol number HM-HP-00042077 and de-identifies all cases before distribution to researchers.
Overall, 39 samples were used for this analysis. This number was primarily dictated by the paucity of autopsy samples available from younger individuals and secondarily by the amount of time taken for the extraction of single cells from each sample by laser capture microdissection. Twenty ASD and nineteen control fresh frozen age, gender and postmortem interval (PMI) matched human lateral hemisphere (crus II) cerebellar blocks were dissected in a consistent anatomical location across cases and cut at 8 µm onto membrane slides (Zeiss MembraneSlide 1.0 PEN 41590-9041-000) with a cryostat (Leica CM 1950) and kept frozen at −80°C.
Sections (8 µm) on membrane slides were briefly fixed in 75% ethanol, dipped into RNase-free water, exposed to a rapid Cresyl violet solution and placed in a series of alcohols for dehydration as described (41). A Zeiss PALM MicroBeam laser microdissection system was used to manually outline PCs, as identified by morphology and cerebellar architecture, which were then lifted into an adhesive cap, effectively isolating approximately 1,500 PCs per case from the surrounding cerebellar tissue. The cells were lysed, and RNA extraction was performed with a Qiagen RNeasy Micro Kit (74004).
RNA sequencing was performed at the John P. Hussman Institute for Human Genomics Center for Genome Technology at the University of Miami. RNA integrity (RIN) scores and RNA concentration were determined for the extracted RNA using the 2100 BioAnalyzer (Agilent Technologies, Santa Clara, CA) and the Qubit RNA High Sensitivity Assay Kit (Thermo Fisher Scientific, Waltham, MA). Samples with a RIN ≥4 were included for library preparation and sequencing. Total RNA was prepared using the Ovation SoLo RNA-Seq Library Preparation Kit (Tecan Life Science, Mannedorf, Switzerland). Sequencing was performed on the Illumina NovaSeq 6,000 (Illumina, San Diego, CA) with single end 100bp reads targeting 25 million reads per sample.
Raw FASTQs were processed through a bioinformatics pipeline including adapter trimming by TrimGalore (v0.6.1) (https://github.com/FelixKrueger/TrimGalore), alignment with the STAR aligner 2.5.0a{{43692 Dobin, A. 2013}} to the GRCh38 human reference genome, and gene counts quantified against the GENCODE v35 human gene release using the GeneCounts function in STAR. Alignment quality control was performed with the CollectRnaSeqMetrics module implemented in Picard v1.103 (http://broadinstitute.github.io/picard). Protein coding genes that had a raw read count <10 in 25% or more of samples were filtered out from further analysis.
The gene counts for each sample were transformed and normalized using the variance-stabilizing transformation method implemented in the Biocinductor package DESeq2 (v2.2){{47440 Love,M.I. 2014}} run with R software v3.4.3. Principle linear regression models within DESeq2 were used to test differentially expressed genes between individuals with ASD and controls, correcting for covariates of age, self-reported ethnicity, sex and post-mortem interval. We analyzed the differentially expressed genes for enriched pathways using the GO Biological Processes and KEGG pathway databases using the DAVID analysis tool (Dennis et al., 2003) with the background set to only those genes analyzed in this experiment. To identify potential protein interaction networks between differentially expressed genes, we utilized the STRINGdb database (Snel et al., 2000; Szklarczyk et al., 2019) accessed at https://string-db.org/with parameters of high confidence interaction (0.7) and MCL using a stringent inflation parameter of 1.5. Bioinformatic analysis on the defined clusters was performed to identify GO processes that are overrepresented in the genes that make up each cluster.
Each 8 µm section was stained with a rapid Cresyl violet stain to visualize cell morphology and outline PC bodies (Figures 1A,B). Upon removal from the tissue (Figure 1C), 1,500 PCs, on average, were pooled and the RNA was immediately extracted. RNAseq data was of high quality and there were no significant difference between the ASD and the control samples in QC metrics, including number of total reads, percentage of uniquely mapping reads, and percentage of reads mapping to the transcriptome (Supplementary Material S1, Table 1). Each sample generated on average 34.6 ± 6.9 million reads with no significant difference in the number of reads between groups (Student’s t-test, p = 0.15). An average of 61.0 ± 9.8% of total reads mapped uniquely to the reference genome and of those 65.1 ± 5.5% mapped to annotated genes, again with no significant differences between ASD and control samples (Student’s t-test, p = 0.58 and p = 0.33, respectively). Of the 19,954 protein coding genes in the GENCODE v35, 13,702 were detected with at least ten reads in at least 25% of the samples. Principal component analysis (PCA) of the variance stabilized transformed values indicated a clear separation of sex based on gene expression, but no outlier samples beyond 2.5 standard deviations from the mean nor distinction of samples by status or self-reported ethnicity (Supplementary Figure S1). Since PCs are the only cell type within the PC layer and the sections were thin, it is unlikely that other cell types were included in extracted cells. To confirm this, we compared the average normalized expression of genes associated with cerebellar PCs versus those associated with cerebellar astrocytes or oligodendrocytes (Kuhn et al., 2012) (Supplementary Figure S2). The average expression of the PC associated genes was significantly higher than those associated with astrocytes or oligodendrocytes (Kruskal-Wallis p-value < 0.0001).
FIGURE 1. Laser capture microdissection and differential expression analysis. Tissue is stained with a rapid Nissl protocol, which clearly delineates Purkinje cells based on the consistent architecture of the cerebellar cortex and large cell bodies (A). The Zeiss RoboPalm software is used to manually outline individual thinly sliced (8 µm) Purkinje cells (B) and specifically removes the cell body, leaving the other cells and tissue behind (C). After RNA extraction and sequencing, Principle Linear regression models within DESeq2 were used to test differentially expressed genes between individuals with ASD and controls, correcting for covariates of age, self-reported ethnicity, sex, and post-mortem interval (D). The top ten significant up- and down-regulated genes are provided (E).
Using the criteria of nominal p-value ≤ 0.05 and a fold change of >1.5, a total of 427 protein coding genes were found to be differentially-expressed between the ASD and control samples (Figure 1D). The 10 most significantly differentially up and down-regulated genes, as determined by p-value are listed in Figure 1E (and Supplementary Figure S3). Of these 427 differentially expressed genes, 360 were down-regulated and 67 were up-regulated in ASD compared to controls (Figure 2A). A complete list of all genes tested and all genes that are significantly differentially expressed is provided in Supplementary Tables S2,3, respectively. These include differential expressed genes (followed by their ASD gene score)—ADCY3(2), AGAP2(2), APBA2(2), BCAS1(2), CACNA2D1(2), CADPS2(2), CDH10(2), CDKL5(1), DLG1(2), GLRA2(2), GRID1(2), GRM5(2), KCND2(2), LRRC4(2), NAV2(2), NR4A2(1), NTNG1(2S), P2RX5(2), PCDH19(1), PCDHA2(2), PRUNE2(2), RANBP17(2), RELN(1), and RIMS1(1)—that are among the autism candidate genes list in the human gene module of SFARI Gene (https://gene.sfari.org/) with a score of 1 (High Evidence) or 2 (Strong Candidate), with S in each category indicating genes classified as syndromic. SFARI curates lists of all genes implicated in ASD from GWAS and other genetic studies.
FIGURE 2. (A) Heat map of differentially expressed genes. Of the total 427 differently expressed genes, 360 were down-regulated and 67 were up-regulated in ASD cases. Both the genes (rows) and cases (columns) were clustered using dendrograms constructed using Euclidean distances and hierarchical clustering and grouped by case demographics. (B) STRINGdb analysis of the differentially expressed genes that were decreased in the cases compared to the controls. The network was divided into clusters using the MCL inflation parameter and the top 4 clusters were analyzed for enrichment of Gene Ontology processes and the top 10 processes for each cluster are represented.
To better understand how these differentially expressed genes impact PC function, GO term and KEGG pathway enrichment analyses were performed (Table 2 and Supplementary Figure S4). The differentially expressed genes were enriched for GO biological processes broadly falling into categories of neuron development, signal transduction, extracellular matrix organization and synaptic function. The KEGG pathways were enriched for MAPK signaling (Table 2). In order to provide more functional context to these altered genes, we performed protein-protein interaction analyses using STRINGdb. STRINGdb analysis was performed on the differentially expressed genes of cases compared to controls as two separate analyses for increased or decreased genes. 352 of the 427 differentially expressed genes were down-regulated and produced a protein-protein interaction cluster with a PPI enrichment p-value of 1.1 × 10-14 (Supplementary Figure S5). This parent cluster was enriched for 216 GO processes (false discovery rate (FDR) < 0.05 (Supplementary Material S1). These included processes related to nervous system development (neurogenesis), cell communication, cell migration, cell adhesion, and axon development. This parental PPI cluster could be broken down into 26 subclusters that had a PPI enrichment p-value < 0.005. The clusters which involved >10 proteins are shown in Figure 2B along with the top 10 GO processes enriched in each cluster. Cluster one was enriched for 137 processes which included multiple processes related to immune responses (such as cytokine-mediated signaling pathways, innate immune response, and type I interferon pathway), cell metabolism and response to growth factors. Cluster two showed an enrichment for 43 processes, including cell migration, tissue/organ development, neurogenesis, cell-substrate adhesion and regulation of dendrite development. Cluster three was enriched in 68 GO processes which involved functions such as chemotaxis, Semaphorin-plexin signaling pathway involved in axon guidance, cell migration, signal transduction and cell development. Cluster four was enriched for 26 GO processes, including regulation of ion transmembrane transport, regulation of membrane potential, chemical synaptic transmission, and action potential. Other clusters were enriched for GO processes, such as apoptosis (Cluster 5), cAMP metabolism (Cluster 6), phospholipase C-activating G protein-coupled receptor signaling pathway (Cluster 7), GABA-A receptor activity (Cluster 8), neuron fate specification (Cluster 11) and negative regulation of sodium ion transmembrane transporter activity (Cluster 15). All of the GO processes enriched for each of the 26 clusters is shown in Supplementary Material S1.75 of the 427 differentially expressed genes were up-regulated in the cases compared to the control samples. However, STRINGdb analysis of the up-regulated genes showed no enrichment for GO processes (data not shown).
There are a number of limitations inherent to current methods in postmortem studies. PMI has been extensively reported on as a factor in postmortem RNA degradation, however, a recent report (K. White et al., 2018) on tissue collected from the NIH Neurobiobank concludes that RIN numbers are a more reliable indicator of RNA quality than PMI and that a RIN number of six or above is acceptable for postmortem RNA research. However, our tissue was stained and ran through alcohol dehydration for the laser capture procedure, therefore, a few cases dropped to a RIN of four or lower and we chose to include cases as low as 4, although most were above 6. Our RNA-seq kit was specifically chosen for use with lower quality samples. Although samples with a RIN score close to four show some degradation, the use of short read next generation sequencing still facilitates the identification and quantification of gene expression. A recent comparative study showed robust gene expression analysis even in lower quality and low input samples using ribodepletion library preparation and short read NGS (Schuierer et al., 2017). Cause of death may also influence RNA degradation, but would be represented in both ASD cases and controls. Limitations in the sample number due to the paucity of postmortem samples in ASD coupled with the work intensive isolation of individual PCs have made it difficult to analyze differences in age, sex and cause of death. With regards to age at donation and PMI, the two groups were matched as best as possible based on the available tissues. Overall, the age and PMI between the ASD cases and controls were not significantly different.
The data suggest that PCs are neurons of interest in ASD with the number of differentially expressed genes on par with that reported in cortical cells. More cases with a method conducive to gathering larger quantities of isolated PCs would be important future studies to elucidate developmental and gender differences. Additionally, larger sample sizes will allow for multiple testing correction to identify differentially expressed genes. Here, we used a p-value of <0.05 as a cutoff, which clinical trials have suggested as an appropriate cutoff for pilot studies (Lee et al., 2014) and focused on the pathway enrichment, which inputs nominally significant results. When using a false discovery rate with a threshold of 0.2, four genes were differentially expressed: CCL2, ZFP36, ADAMTS1 and GALNT17. Future studies should compare transcriptional profiles to the genetic mutations implicated in each sample and it would be useful to compare the PC transcriptional profile to other cell types in ASD tissue, such as granule cells, to determine whether PCs are more heavily impacted. Substantial literature exists relating to PCS and the potential function of many of the implicated genes, particularly from rodent studies, however, a full discussion is outside the scope of this study. Additionally, validation of gene targets of interest with immunohistochemistry, in situ hybridization or qRT-PCR would be valuable. Genes implicated in ASD are highly heterogeneous, often having significant statistical association but unclear functional impact. Therefore, we chose to base our analyses and discussion on shared pathways through which these genes may participate in the etiology of ASD.
Social interaction, language and coordinated sensorimotor behaviors depend on the integration of information across brain areas. In ASD, hyper- and hypo-connectivity between regions have been extensively reported in neuroimaging studies (Belmonte et al., 2004; Just et al., 2004; Just et al., 2007; Minshew and Williams, 2007; Mostofsky and Ewen, 2011; Wass, 2011; Mak-Fan et al., 2013; Nair et al., 2014; Ray et al., 2014; Dean et al., 2016; Jahedi et al., 2017). The cerebellum in particular has been reported to have volumetric differences based on connectivity trajectories during development (Becker and Stoodley, 2013; Hampson and Blatt, 2015; Wang et al., 2014; Scott et al., 2009; Arnold Anteraper et al., 2019). These findings suggest the possibility of a disrupted developmental trajectory of white matter development in ASD.
The transcriptome findings presented here suggest that PCs specifically may be contributing to altered connectivity in ASD. This implication is consistent with large-scale GWAS and gene pathway enrichment findings implicating alterations in neurite outgrowth and guidance in ASD (Hussman et al., 2011). PCs are the only output neurons of the cerebellar cortex, sending their axons to the deep cerebellar nuclei to coordinate information to the rest of the brain. Downregulation of genes in pathways such as cell migration, axon guidance, nervous system development and neuron projection/extension cell proliferation pathways could have significant consequences for the functional organization of the cerebellum. During development, cell-adhesion molecules and extracellular matrix organization are critical for patterning the intricate zones and stripes of the cerebellar cortex (Sotelo, 2020; Apps et al., 2018; Sillitoe and Joyner 2007; White and Sillitoe, 2013). How these downregulated genes impact the early development of PC patterning and their potential long-term impact on functional connectivity in the adult brain will be important to determine. In an analysis of PC gene expression in the developing mouse brain (Clifford et al., 2019), identified a cluster of genes enriched for genes associated with ASD whose expression was positively regulated during PC development. Gene ontology analysis showed this cluster of genes was enriched for those involved in cell-cell signaling, synaptic transmission, cerebellar PC layer development, cellular localization and developmental maturation. Although this analysis was performed in mice at varying developmental stages, we identify gene enrichment in similar pathways, supporting the validity of our findings in human PCs.
A consistent finding in genome-wide association studies (GWAS) is genetic susceptibility in ECM-associated genes (Weiss and Arking, 2009; Hussman et al., 2011; Wang et al., 2009; Anney et al., 2010). Among these genes, Reelin, a disintegrin and metalloproteinase with thrombospondin motifs (ADAMTS) and semaphorins provide the strongest evidence linking ASD to disruption of the ECM (for review see: Pantazopoulos and Berretta, 2016; Sorg et al., 2016). Considering that these GWAS studies typically analyze DNA from whole blood, it is encouraging that mRNA expression data from a specific neuron subtype can recapitulate these findings and show differential mRNA expression of previously implicated genes. Notably, RELN, as well as multiple ADAMTS and semaphorin genes were differentially expressed in ASD compared to neurotypical individuals in our PC transcriptome analysis.
Components of a condensed form of the ECM, perineuronal nets (PNNs), are highly enriched in the deep cerebellar nuclei (DCN), surrounding synapses from PCs onto DCN neurons and have recently been shown to be important for cerebellar plasticity and the formation of associative memories (Carulli et al., 2020). PCs produce various components of PNNs and are themselves surrounded by a loose (semi-organized) form of PNN (Carulli et al., 2006). PNNs are typically associated with fast-spiking neurons, have numerous functional roles in development, plasticity and neuroprotection and can be modified by numerous factors including metalloproteinases (for review see: Fawcett et al., 2019). ADAMTS are a type of metalloproteinase shown to degrade PNN components and are associated with remodeling and excitotoxicity. The differential expression of several ADAMTS in ASD PCs, suggests that both PC function and input to the DCN may be impacted.
PNNs have gained attention due to their dysregulation in multiple psychiatric conditions (Berretta et al., 2015; Sorg et al., 2016; Wen et al., 2018) and they have known roles in modulating parvalbumin interneuron function and gamma oscillations. As there is increasing evidence of association between parvalbumin interneuron dysfunction and ASD, the study of PNNs in the context of ASD will be important for future research (Burket et al., 2017; Brandenburg and Blatt 2022).
Parvalbumin, a calcium-binding protein, has been reported to be dysregulated in multiple mouse models of ASD (Gogolla et al., 2009; Wöhr et al., 2015) as well as in human postmortem studies (Hashemi et al., 2017; Brandenburg and Blatt 2022). Notably, while PC loss is commonly observed in ASD, the remaining PCs in postmortem ASD cases have shown reduced mRNA expression for parvalbumin using in situ hybridization histochemistry (Soghomonian et al., 2017). This finding suggests brain-wide changes in inhibitory network function. However, PCs have an exceptionally high concentration of several different calcium-binding proteins that are critical for PC calcium homeostasis (Schwaller et al., 2002). Although our cases did not show significant differences in parvalbumin expression, calcium ion response was an enriched pathway for downregulated genes.
A potential relationship exists between calcium ion response and the ECM pathways. The physiochemical properties of ECM/PNNs can regulate concentration gradients by acting as a reservoir for extracellular ions and modifying their diffusion properties (Morawski et al., 2015). If ECM/PNNs are indeed dysregulated around PCs in ASD, they could potentially impact ion homeostasis and produce differences in calcium ion response and neuronal signaling. As calcium homeostasis is an important aspect of PC function, the possibility of ECM/PNN disruption may be among important pathways to consider in ASD.
Several pathways related to functional properties of PCs were enriched, including signal transduction, regulation of membrane potential, positive regulation of phosphatidylinositol 3-kinase (PI3K) signaling and the mitogen-activated protein kinase (MAPK) signaling pathway. The interplay of these various pathways and the functional outcomes of their disruption may provide clues for treatment strategies, especially in the context of sensorimotor challenges. The MAPK signaling pathway was identified in the KEGG pathway enrichment analysis. Alterations in MAPK signaling have been associated with altered brain cytoarchitecture (Pucilowska et al., 2015; Pucilowska et al., 2018) and can result in social deficits and cognitive dysfunction reminiscent of ASD (Faridar et al., 2014; Yufune et al., 2015). Likewise, PI3K signaling plays an important role in neuronal guidance and circuit formation (Hussman et al., 2011). Among the genes in this pathway, loss of PDGFRB expression has been associated with social interaction deficits (a core feature of ASD) in mouse models (Nguyen et al., 2011). Additionally, loss of CACNA2D1 (another gene differentially expressed between the ASD and control PCs) expression altered the morphology and functionality of cerebellar PCs (Barclay et al., 2001; Brodbeck et al., 2002). (Velmeshev et al., 2019) performed single nuclei RNAseq from individuals with ASD (15 individuals) and control (16 individuals) and showed an enrichment in differential gene expression associated with chemical synaptic transmission, axon guidance and neuronal migration. Although they performed their analysis on cells from the prefrontal cortex and anterior cingulate cortex, they identified similar GO categories as we identified here.
As reviewed in (Meltzer and Van De Water, 2017), immune pathways are commonly altered in ASD GWAS and transcriptome studies. However, the transcriptome studies reported typically use bulk tissue so have representation of neurons, microglia and astrocytes. In the present study, we isolated PCs from the surrounding tissue and found down-regulated genes in a variety of immune related pathways. Among these genes, CCL2 has been previously suggested to act as a modulator of neuronal activity, neuroendocrine functions and neuronal inflammatory processes (Banisadr et al., 2005). It has also been suggested to support the maturation of PCs (Meng et al., 1999)by promoting dendrite outgrowth and synapse maturation, GO processes that were shown to be enriched in the STRINGdb analysis. Experiments in mice have shown that IRF7 (interferon regulatory factor 7) was involved in toll like receptor-mediated neuronal protection from stressors, such as ischemia (Stevens et al., 2011). Additionally, the deletion of IRF-1 in mice lead to elevated levels of cognitive impairment, supporting a role for IRF1 in cognitive function (Mogi et al., 2018). Mouse knock-out studies have shown that Interleukin one receptor 1 (IL1R1) has also been associated with social interactivity and working memory/cognition in mice (DiSabato et al., 2021). Myeloid differentiation primary response protein 88 (MyD88) knockout in mice resulted in decreased motor activity and elevated levels of anxiety-like behavior. In addition, the MyD88−/− mice showed decreased neuronal arborization (Schroeder et al., 2021). Myd88 −/− neurons were unable to respond to Toll-like receptor 3 (TLR3) activation leading to an inhibition of dendritic growth (Chen et al., 2017). These results and others show that immune genes play key roles in neuronal development and maturation leading to phenotypes that are consistent with ASD.
Immune related genes may also have a potential relationship with ECM pathway dysregulation, as matrix metalloproteinases (MMPs) can induce neuroimmune responses by triggering microglial activation and cytokine release (Bitanihirwe et al., 2016). It is possible that dysregulation of ECM/PNNs, consistent with the pathway findings reported here, could interact with immune pathways in ASD, with a combination of environmental factors and genetic susceptibility contributing to an altered transcriptional profile of ECM/PNN genes.
Our discussion centers on the top enriched GO pathways and potential evidence for their interaction with other implicated pathways, but a definitive link to any particular pathway is speculative in ASD cases. We hope future studies will validate individual genes from this pilot study and examine their participation in the broad pathways hypothesized. It is worth noting that specific sets of genes are often dysregulated in ASD (Cell, 2013, 155 (5), 1,008–1,021;, Cell, 2013, 155 (5), 997–1,007; Velmeshev et al., Science (New York, N.Y.), 2019, 364 (6,441), 685–89), suggesting that gene regulatory mechanisms are impacted. Chromatin modifiers are capable of regulating ASD risk genes (Murgatroyd and Spengler, 2012; Krumm et al., 2014; Hoffmann and Spengler, 2021) and their contribution to the differential expression of genes in PCs will be important to uncover. Although the individuals in this cohort were adolescents or adults at the time of death, it is noteworthy that many of the enriched pathways suggest altered PC developmental trajectories and connectivity in ASD cases. The impact of early developmental transcript differences on PC specification within the complex landscape of cerebellar microcircuitry maturation may therefore be a worthwhile avenue of exploration. Other enriched pathways that impact mature PCs, such as signal transduction, regulation of membrane potential, cellular response to calcium ions and positive regulation of phosphatidylinositol three kinase signaling may be amenable to treatment strategies. Identification of altered gene expression in PCs is a step toward understanding the complex molecular and physiological mechanisms underlying ASD.
The data presented in the study are deposited in the GEO repository, accession number GEO GSE211154, https://www.ncbi.nlm.nih.gov/geo/query/acc.cgi?acc=GSE211154
CB: conceptualization; CB, AG, DB, DD: investigation; CB, AG, DB, MK, JF, MN, DD: methodology; CB, AJ, DD: writing—original draft. CB, AG, DB, MK, JF, MN, DD, MP-V, GB: review and editing. MP-V, GB: supervision.
This work was supported by the Hussman Foundation.
The authors gratefully thank the families that donated brain tissue to the University of Maryland Brain and Tissue Bank (NIH NeuroBioBank) that make this and other research possible. The authors are grateful for technical support from Djeneba Sidibe, Brittany White and Charity Ensor. Finally, the authors also acknowledge Dr. John Hussman, whose support made this research project possible.
The authors declare that the research was conducted in the absence of any commercial or financial relationships that could be construed as a potential conflict of interest.
All claims expressed in this article are solely those of the authors and do not necessarily represent those of their affiliated organizations, or those of the publisher, the editors and the reviewers. Any product that may be evaluated in this article, or claim that may be made by its manufacturer, is not guaranteed or endorsed by the publisher.
The Supplementary Material for this article can be found online at: https://www.frontiersin.org/articles/10.3389/fgene.2022.944837/full#supplementary-material
American Psychiatric Association (2013). Diagnostic and statistical manual of mental disorders: DSM-5. Arlington, VA: American Psychiatric Association.
Anney, R., Klei, L., Pinto, D., Regan, R., Conroy, J., Magalhaes, T. R., et al. (2010). A genome-wide scan for common alleles affecting risk for autism. Hum. Mol. Genet. 19 (20), 4072–4082. doi:10.1093/hmg/ddq307
Apps, R., Hawkes, R., Aoki, S., Bengtsson, F., Brown, A. M., Chen, G., et al. (2018). Cerebellar modules and their role as operational cerebellar processing units: A consensus paper [corrected]. Cerebellum 17 (5), 654–682. doi:10.1007/s12311-018-0952-3
Arnold Anteraper, S., Guell, X., D’Mello, A., Joshi, N., Whitfield-Gabrieli, S., and Joshi, G. (2019). Disrupted cerebrocerebellar intrinsic functional connectivity in young adults with high-functioning autism spectrum disorder: A data-driven, whole-brain, high-temporal resolution functional magnetic resonance imaging study. Brain Connect. 9 (1), 48–59. doi:10.1089/brain.2018.0581
Bailey, A., Luthert, P., Dean, A., Harding, B., Janota, I., Montgomery, M., et al. (1998). A clinicopathological study of autism. Brain 121 (5), 889–905. doi:10.1093/brain/121.5.889
Banisadr, G., Gosselin, R.-D., Mechighel, P., Kitabgi, P., Rostène, W., and Parsadaniantz, S. M. (2005). Highly regionalized neuronal expression of monocyte chemoattractant protein-1 (MCP-1/CCL2) in rat brain: Evidence for its colocalization with neurotransmitters and neuropeptides. J. Comp. Neurol. 489 (3), 275–292. doi:10.1002/cne.20598
Barclay, J., Balaguero, N., Mione, M., Ackerman, S. L., Letts, V. A., Brodbeck, J., et al. (2001). Ducky mouse phenotype of epilepsy and ataxia is associated with mutations in the Cacna2d2 gene and decreased calcium channel current in cerebellar Purkinje cells. J. Neurosci. 21, 6095. doi:10.1523/JNEUROSCI.21-16-06095.2001
Bauman, M., and Kemper, T. L. (1985). Histoanatomic observations of the brain in early infantile autism. Neurology 35 (6), 866–874. doi:10.1212/wnl.35.6.866
Bauman, M. L., Kemper, T. L., and Arin, D. M. (1995). Pervasive neuroanatomic abnormalities of the brain in three cases of rett’s syndrome. Neurology 45 (8), 1581–1586. doi:10.1212/wnl.45.8.1581
Becker, E. B. E., and Stoodley, C. J. (2013). Autism spectrum disorder and the cerebellum. Int. Rev. Neurobiol. 113, 1–34. doi:10.1016/B978-0-12-418700-9.00001-0
Belmonte, M. K., Allen, G., Beckel-Mitchener, A., Boulanger, L. M., Carper, R. A., and Webb, S. J. (2004). Autism and abnormal development of brain connectivity. J. Neurosci. 24, 9228–9231. Society for Neuroscience. doi:10.1523/JNEUROSCI.3340-04.2004
Berretta, S., Pantazopoulos, H., Markota, M., Brown, C., and Batzianouli, E. T., (2015). Losing the sugar coating: Potential impact of perineuronal net abnormalities on interneurons in schizophrenia. Schizophrenia Res. 167, 18. Elsevier B.V. doi:10.1016/j.schres.2014.12.040
Beversdorf, D. Q., Manning, S. E., Hillier, A., Anderson, S. L., Nordgren, R. E., Walters, S. E., et al. (2005). Timing of prenatal stressors and autism. J. Autism Dev. Disord. 35 (4), 471–478. doi:10.1007/s10803-005-5037-8
Bitanihirwe, B. K. Y., Mauney, S. A., and Woo, T.-U. W. (2016). Weaving a net of neurobiological mechanisms in schizophrenia and unraveling the underlying pathophysiology. Biol. Psychiatry 80 (8), 589–598. doi:10.1016/j.biopsych.2016.03.1047
Brandenburg, C., and Blatt, G. J. (2022). Region-specific alterations of perineuronal net expression in postmortem autism brain tissue. Front. Mol. Neurosci. 15, 838918–839010. doi:10.3389/fnmol.2022.838918
Brodbeck, J., Davies, A., Courtney, J.-M., Meir, A., Balaguero, N., Canti, C., et al. (2002). The ducky mutation in Cacna2d2 results in altered Purkinje cell morphology and is associated with the expression of a truncated a2δ-2 protein with abnormal function. J. Biol. Chem. 277 (10), 7684–7693. doi:10.1074/JBC.M109404200
Burket, J. A., Urbano, M. R., and Deutsch, S. I. (2017). Sugarcoated perineuronal nets regulate ‘GABAergic’ transmission: Bittersweet hypothesis in autism spectrum disorder. Clin. Neuropharmacol. 40 (3), 120–130. doi:10.1097/WNF.0000000000000209
Carulli, D., Broersen, R., De Winter, F., Muir, E. M., Meškovi, M., De Waal, M., et al. (2006). Composition of perineuronal nets in the adult rat cerebellum and the cellular origin of their components. J. Comp. Neurol. 494 (4), 559–577. doi:10.1002/cne.20822
Carulli, D., Rhodes, K. E., Brown, D. J., Bonnert, T. P., Pollack, S. J., Oliver, K., et al. (2020). Composition of perineuronal nets in the adult rat cerebellum and the cellular origin of their components. Biol. Sci. 117, 6855. Accessed February 14, 2021. doi:10.1073/pnas.1916163117/-/DCSupplemental
Chen, C.-Y., Liu, H.-Y., and Hsueh, Y.-P. (2017). TLR3 downregulates expression of schizophrenia gene Disc1 via MYD88 to control neuronal morphology. EMBO Rep. 18 (1), 169–183. doi:10.15252/embr.201642586
Chu, T. T., Liu, Y., and Kemether, E. (2009). Thalamic transcriptome screening in three psychiatric states. J. Hum. Genet. 54 (11), 665–675. doi:10.1038/jhg.2009.93
Clifford, H., Dulneva, A., Ponting, C. P., Haerty, W., and Becker, E. B. E. (2019). A gene expression signature in developing Purkinje cells predicts autism and intellectual disability Co-morbidity status. Sci. Rep. 9 (1), 485–510. doi:10.1038/s41598-018-37284-1
Courchesne, E., Karns, C. M., Davis, H. R., Ziccardi, R., Carper, R. A., Tigue, Z. D., et al. (2001). Unusual brain growth patterns in early life in patients with autistic disorder: An MRI study. Neurology 57 (2), 245–254. doi:10.1212/wnl.57.2.245
Cunnea, P., McMahon, J., O’Connell, E., Mashayekhi, K., Fitzgerald, U., and McQuaid, S. (2010). Gene expression analysis of the microvascular compartment in multiple sclerosis using laser microdissected blood vessels. Acta Neuropathol. 119 (5), 601–615. doi:10.1007/s00401-009-0618-9
Dean, D. C., Travers, B. G., Adluru, N., Tromp, D. P. M., Destiche, D. J., Samsin, D., et al. (2016). Investigating the microstructural correlation of white matter in autism spectrum disorder. Brain Connect. 6 (5), 415–433. doi:10.1089/brain.2015.0385
Dennis, G., Sherman, B. T., Hosack, D. A., Yang, J., Gao, W., Lane, H. C., et al. (2003). David: Database for annotation, visualization, and integrated discovery. Genome Biol. 4 (5), R60. doi:10.1186/gb-2003-4-9-r60
DiSabato, D. J., Nemeth, D. P., Liu, X., Witcher, K. G., O’Neil, S. M., Oliver, B., et al. (2021). Interleukin-1 receptor on hippocampal neurons drives social withdrawal and cognitive deficits after chronic social stress. Mol. Psychiatry 26 (9), 4770–4782. doi:10.1038/s41380-020-0788-3
Faridar, A., Jones-Davis, D., Rider, E., Li, J., Gobius, I., Morcom, L., et al. (2014). Mapk/erk activation in an animal model of social deficits shows a possible link to autism. Mol. Autism 5 (1), 57. doi:10.1186/2040-2392-5-57
Fatemi, S. H., Aldinger, K. A., Ashwood, P., Bauman, M. L., Blaha, C. D., Blatt, G. J., et al. (2002). Purkinje cell size is reduced in cerebellum of patients with autism. Cell. Mol. Neurobiol. 22 (2), 171–175. doi:10.1023/a:1019861721160
Fatemi, S. H., Aldinger, K. A., Ashwood, P., Bauman, M. L., Blaha, C. D., Blatt, G. J., et al. (2012). Consensus paper: Pathological role of the cerebellum in autism. Cerebellum Lond. Engl. 11 (3), 777–807. doi:10.1007/s12311-012-0355-9
Fawcett, J. W., Oohashi, T., and Pizzorusso, T. (2019). The roles of perineuronal nets and the perinodal extracellular matrix in neuronal function. Nat. Rev. Neurosci. 20, 451. Nature Publishing Group. doi:10.1038/s41583-019-0196-3
Federmeier, K. D., Kleim, J. A., and Greenough, W. T. (2002). Learning-induced multiple synapse formation in rat cerebellar cortex. Neurosci. Lett. 332 (3), 180–184. doi:10.1016/s0304-3940(02)00759-0
Gogolla, N., Leblanc, J. J., Quast, K. B., Südhof, T. C., Fagiolini, M., and Hensch, T. K. (2009). Common circuit defect of excitatory-inhibitory balance in mouse models of autism. J. Neurodev. Disord. 1 (2), 172–181. doi:10.1007/s11689-009-9023-x
Greene, J. G., Dingledine, R., and Greenamyre, J. T. (2010). Neuron-selective changes in RNA transcripts related to energy metabolism in toxic models of parkinsonism in rodents. Neurobiol. Dis. 38 (3), 476–481. doi:10.1016/j.nbd.2010.03.014
Hampson, D. R., and Blatt, G. J. (2015). Autism spectrum disorders and neuropathology of the cerebellum. Front. Neurosci. 9, 420. doi:10.3389/fnins.2015.00420
Hashemi, E., Ariza, J., Rogers, H., Noctor, S. C., and Martínez-Cerdeño, V. (2017). The number of parvalbumin-expressing interneurons is decreased in the prefrontal cortex in autism. Cereb. Cortex 1991 27 (3), 1931–1943. doi:10.1093/cercor/bhw021
Hashimoto, T., Tayama, M., Murakawa, K., Yoshimoto, T., Miyazaki, M., Harada, M., et al. (1995). Development of the brainstem and cerebellum in autistic patients. J. Autism Dev. Disord. 25 (1), 1–18. doi:10.1007/BF02178163
Hoffmann, A., and Spengler, D. (2021). Clinical medicine chromatin remodeler CHD8 in autism and brain development. J. Clin. Med. 10, 366. doi:10.3390/jcm10020366
Hussman, J. P., Chung, R.-H., Griswold, A. J., Jaworski, J. M., Salyakina, D., Ma, D., et al. (2011). A noise-reduction GWAS analysis implicates altered regulation of neurite outgrowth and guidance in autism. Mol. Autism 2 (1), 1. doi:10.1186/2040-2392-2-1
Jahedi, A., Nasamran, C. A., Faires, B., Fan, J., and Müller, R.-A. (2017). Distributed intrinsic functional connectivity patterns predict diagnostic status in large autism cohort. Brain Connect. 7 (8), 515–525. doi:10.1089/brain.2017.0496
Just, M. A., Cherkassky, V. L., Keller, T. A., Kana, R. K., and Minshew, N. J. (2007). Functional and anatomical cortical underconnectivity in autism: Evidence from an fmri study of an executive function task and corpus callosum morphometry. Cereb. Cortex 17 (4), 951–961. doi:10.1093/cercor/bhl006
Just, M. A., Cherkassky, V. L., Keller, T. A., and Minshew, N. J. (2004). Cortical activation and synchronization during sentence comprehension in high-functioning autism: Evidence of underconnectivity. Brain 127 (8), 1811–1821. doi:10.1093/brain/awh199
Kim, H. T., Kim, I. H., Lee, K. J., Lee, J. R., Park, S. K., Chun, Y.-H., et al. (2002). Specific plasticity of parallel fiber/purkinje cell spine synapses by motor skill learning. Neuroreport 13 (13), 1607–1610. doi:10.1097/00001756-200209160-00007
Kleim, J. A., Swain, R. A., Armstrong, K. A., Napper, R. M. A., Jones, T. A., and Greenough, W. T. (1998). Selective synaptic plasticity within the cerebellar cortex following complex motor skill learning. Neurobiol. Learn. Mem. 69 (3), 274–289. doi:10.1006/nlme.1998.3827
Krumm, N., O’Roak, B. J., Shendure, J., and Eichler, E. E. (2014). A de Novo convergence of autism genetics and molecular neuroscience. Trends Neurosci. 37 (2), 95–105. doi:10.1016/J.TINS.2013.11.005
Kuhn, A., Kumar, A., Beilina, A., Dillman, A., Cookson, M. R., and Singleton, A. B. (2012). Cell population-specific expression analysis of human cerebellum. BMC Genomics 13, 610. doi:10.1186/1471-2164-13-610
Lee, K. J., Kim, H., and Rhyu, I. J. (2005). The roles of dendritic spine shapes in Purkinje cells. Cerebellum Lond. Engl. 4 (2), 97–104. doi:10.1080/14734220510007842
Lee, E. C., Whitehead, A. L., Jacques, R. M., and Julious, S. A. (2014). The statistical interpretation of pilot trials: Should significance thresholds Be reconsidered? BMC Med. Res. Methodol. 14, 41. doi:10.1186/1471-2288-14-41
Limperopoulos, C., Bassan, H., Gauvreau, K., Robertson, R. L., Sullivan, N. R., Benson, C. B., et al. (2007). Does cerebellar injury in premature infants contribute to the high prevalence of long-term cognitive, learning, and behavioral disability in survivors? Pediatrics 120 (3), 584–593. doi:10.1542/peds.2007-1041
Lin, Y.-C., Frei, J. A., Kilander, M. B. C., Shen, W., and Blatt, G. J. (2016). A subset of autism-associated genes regulate the structural stability of neurons. Front. Cell. Neurosci. 10, 263–335. doi:10.3389/fncel.2016.00263
Mak-Fan, K. M., Morris, D., Vidal, J., Anagnostou, E., Roberts, W., and Taylor, M. J. (2013). White matter and development in children with an autism spectrum disorder. Autism 17 (5), 541–557. doi:10.1177/1362361312442596
Meltzer, A., and Van De Water, J. (2017). The role of the immune system in autism spectrum disorder. Neuropsychopharmacology 42, 284. Nature Publishing Group. doi:10.1038/npp.2016.158
Meng, S. Z., Oka, A., and Takashima, S. (1999). Developmental expression of monocyte chemoattractant protein-1 in the human cerebellum and brainstem. Brain Dev. 21 (1), 30–35. doi:10.1016/s0387-7604(98)00065-5
Minshew, N. J., and Williams, D. L. (2007). The new neurobiology of autism: Cortex, connectivity, and neuronal organization.” Archives of neurology. Arch. Neurol. 64, 945–950. doi:10.1001/archneur.64.7.945
Mogi, M., Iwanami, J., Wang, X.-L., Tsukuda, K., Kan-No, H., Bai, H., et al. (2018). Deletion of interferon-regulatory factor-1 results in cognitive impairment. Hypertens. Res. 41 (10), 809–816. doi:10.1038/s41440-018-0080-y
Morawski, M., Reinert, T., Meyer-Klaucke, W., Wagner, F. E., Tröger, W., Reinert, A., et al. (2015). Ion exchanger in the brain: Quantitative analysis of perineuronally fixed anionic binding sites suggests diffusion barriers with ion sorting properties. Sci. Rep. 5, 16471. doi:10.1038/srep16471
Mostofsky, S. H., and Ewen, J. B. (2011). Altered connectivity and action model formation in autism is autism. Neuroscientist 17, 437–448. doi:10.1177/1073858410392381
Murgatroyd, C., and Spengler, D. (2012). Genetic variation in the epigenetic machinery and mental health. Curr. Psychiatry Rep. 14, 138–149. doi:10.1007/s11920-012-0255-1
Murray, G. I. (2007). An overview of laser microdissection Technologies. Acta Histochem. 109, 171. Elsevier GmbH. doi:10.1016/j.acthis.2007.02.001
Nair, A., Keown, C. L., Datko, M., Shih, P., Keehn, B., and Müller, R.-A. (2014). Impact of methodological variables on functional connectivity findings in autism spectrum disorders. Hum. Brain Mapp. 35 (8), 4035–4048. doi:10.1002/hbm.22456
Nassir, N., Bankapur, A., Samara, B., Ali, A., Ahmed, A., Inuwa, I. M., et al. (2021). Single-cell transcriptome identifies molecular subtype of autism spectrum disorder impacted by de Novo loss-of-function variants regulating glial cells. Hum. Genomics 15 (1), 68. doi:10.1186/s40246-021-00368-7
Nguyen, P. T. H., Nakamura, T., Hori, E., Urakawa, S., Uwano, T., Zhao, J., et al. (2011). Cognitive and socio-emotional deficits in platelet-derived growth factor receptor-β gene knockout mice. PLoS ONE 6 (3), e18004. doi:10.1371/JOURNAL.PONE.0018004
Pantazopoulos, H., and Berretta, S. (2016). In sickness and in health: Perineuronal nets and synaptic plasticity in psychiatric disorders. Neural Plast. 2016 2016, 9847696. doi:10.1155/2016/9847696
Parikshak, N. N., Luo, R., Zhang, A., Won, H., Lowe, J. K., Chandran, V., et al. (2013). Integrative functional genomic analyses implicate specific molecular pathways and circuits in autism. Cell 155 (5), 1008–1021. doi:10.1016/j.cell.2013.10.031
Parikshak, N. N., Swarup, V., Belgard, T. G., Irimia, M., Ramaswami, G., Gandal, M. J., et al. (2016). Vivek Swarup, T. Grant Belgard, Manuel Irimia, Gokul Ramaswami, Michael J. Gandal, Christopher Hartl, et alGenome-Wide Changes in LncRNA, Splicing, and Regional Gene Expression Patterns in Autism. Nature 540 (7633), 423–427. doi:10.1038/nature20612
Pietersen, C. Y., Lim, M. P., and Woo, T.-U. W. (2009). Obtaining high quality RNA from single cell populations in human postmortem brain tissue. J. Vis. Exp. 30, 1444–1449. doi:10.3791/1444
Pietersen, C. Y., Lim, M. P., Macey, L., Woo, T.-U. W., and Sonntag, K. C. (2011). Neuronal type-specific gene expression profiling and laser-capture microdissection. Methods Mol. Biol. 755, 327–343. doi:10.1007/978-1-61779-163-5_28
Pucilowska, J., Vithayathil, J., Tavares, E. J., Kelly, C., Karlo, J. C., and Landreth, G. E. (2015). The 16p11.2 deletion mouse model of autism exhibits altered cortical progenitor proliferation and brain cytoarchitecture linked to the ERK MAPK pathway. J. Neurosci. 35 (7), 3190–3200. doi:10.1523/JNEUROSCI.4864-13.2015
Pucilowska, J., Vithayathil, J., Pagani, M., Kelly, C., Karlo, J. C., Robol, C., et al. (2018). Pharmacological inhibition of ERK signaling rescues pathophysiology and behavioral phenotype Associated with 16p11.2 chromosomal deletion in mice. J. Neurosci. 38 (30), 6640–6652. doi:10.1523/JNEUROSCI.0515-17.2018
Ray, S., Miller, M., Karalunas, S., Robertson, C., Grayson, D. S., Cary, R. P., et al. (2014). Structural and functional connectivity of the human brain in autism spectrum disorders and attention-deficit/hyperactivity disorder: A rich club-organization study. Hum. Brain Mapp. 35 (12), 6032–6048. doi:10.1002/hbm.22603
Rogers, T. D., McKimm, E., Dickson, P. E., Goldowitz, D., Blaha, C. D., and Mittleman, G. (2013). Is autism a disease of the cerebellum? An integration of clinical and pre-clinical research. Front. Syst. Neurosci. 7, 15. doi:10.3389/fnsys.2013.00015
Schmahmann, J. D. (2019). The cerebellum and cognition. Neurosci. Lett. 688, 62–75. doi:10.1016/j.neulet.2018.07.005
Schroeder, P., Rivalan, M., Zaqout, S., Krüger, C., Schüler, J., Long, M., Meisel, A., et al. (2021). Abnormal brain structure and behavior in MyD88-deficient mice. Brain Behav. Immun. 91, 181–193. doi:10.1016/j.bbi.2020.09.024
Schuierer, S., Carbone, W., Knehr, J., Petitjean, V., Fernandez, A., Sultan, M., et al. (2017). A comprehensive assessment of RNA-seq protocols for degraded and low-quantity samples. BMC Genomics 18 (1), 442. doi:10.1186/s12864-017-3827-y
Schumann, C. M., and Nordahl, C. W. (2011). Bridging the gap between MRI and postmortem research in autism. Brain Res. 1380, 175–186. doi:10.1016/j.brainres.2010.09.061
Schwaller, B., Meyer, M., and Schiffmann, S. (2002). ‘New’ functions for ‘old’ proteins: The role of the calcium-binding proteins calbindin D-28k, calretinin and parvalbumin, in cerebellar physiology. Studies with knockout mice. Cerebellum 1 (4), 241–258. doi:10.1080/147342202320883551
Scott, J. A., Schumann, C. M., Goodlin-Jones, B. L., and Amaral, D. G. (2009). A comprehensive volumetric analysis of the cerebellum in children and adolescents with autism spectrum disorder. Autism Res. 2 (5), 246–257. doi:10.1002/aur.97
Sillitoe, R. V., and Joyner, A. L. (2007). Morphology, molecular codes, and circuitry produce the three-dimensional complexity of the cerebellum. Annu. Rev. Cell Dev. Biol. 23, 549–577. doi:10.1146/annurev.cellbio.23.090506.123237
Simpson, J. E., Ince, P. G., Shaw, P. J., Heath, P. R., Raman, R., Garwood, C. J., et al. (2011). Microarray analysis of the astrocyte transcriptome in the aging brain: Relationship to alzheimer’s pathology and APOE genotype. Neurobiol. Aging 32 (10), 1795–1807. doi:10.1016/j.neurobiolaging.2011.04.013
Skefos, J., Cummings, C., Enzer, K., Holiday, J., Weed, K., Levy, E., et al. (2014). Regional alterations in Purkinje cell density in patients with autism. PloS One 9 (2), e81255. doi:10.1371/journal.pone.0081255
Snel, B., Lehmann, G., Bork, P., and Huynen, M. A. (2000). String: A web-server to retrieve and display the repeatedly occurring neighbourhood of a gene. Nucleic Acids Res. 28 (18), 3442–3444. doi:10.1093/nar/28.18.3442
Soghomonian, J.-J., Zhang, K., Reprakash, S., and Blatt, G. J. (2017). Decreased parvalbumin MRNA levels in cerebellar Purkinje cells in autism. Autism Res. 10 (11), 1787–1796. doi:10.1002/aur.1835
Sorg, B. A., Berretta, S., Blacktop, J. M., Fawcett, J. W., Kitagawa, H., Kwok, J. C. F., et al. (2016). Mini-symposium casting a wide net: Role of perineuronal nets in neural plasticity. J. Neurosci. 36, 11459. doi:10.1523/JNEUROSCI.2351-16.2016
Sotelo, C. (2020). Cellular mechanisms involved in cerebellar microzonation. Neuroscience 462, 56–69. doi:10.1016/j.neuroscience.2020.01.019
Stevens, S. L., Leung, P. Y., Vartanian, K. B., Gopalan, B., Yang, T., Simon, R. P., et al. (2011). Multiple preconditioning paradigms converge on interferon regulatory factor-dependent signaling to promote tolerance to ischemic brain injury. J. Neurosci. 31 (23), 8456–8463. doi:10.1523/JNEUROSCI.0821-11.2011
Subramanian, K., Brandenburg, C., Orsati, F., Soghomonian, J.-J., Hussman, J. P., and Blatt, G. J. (2017). Basal ganglia and autism - a translational perspective. Autism Res. 10 (11), 1751–1775. doi:10.1002/aur.1837
Szklarczyk, D., Gable, A. L., Lyon, D., Junge, A., Wyder, S., Huerta-Cepas, J., et al. (2019). String V11: Protein-protein association networks with increased coverage, supporting functional discovery in genome-wide experimental datasets. Nucleic Acids Res. 47 (1), D607–D613. doi:10.1093/nar/gky1131
Velmeshev, D., Schirmer, L., Jung, D., Haeussler, M., Perez, Y., Mayer, S., et al. (2019). Single-cell Genomics identifies cell type-specific molecular changes in autism. Sci. (New York, N.Y.) 364 (6441), 685–689. doi:10.1126/science.aav8130
Waller, R., Woodroofe, M. N., Francese, S., Heath, P. R., Wharton, S. B., Ince, P. G., et al. (2012). Isolation of enriched glial populations from post-mortem human CNS material by immuno-laser capture microdissection. J. Neurosci. Methods 208 (2), 108–113. doi:10.1016/j.jneumeth.2012.04.014
Wang, K., Zhang, H., Ma, D., Bucan, M., Glessner, J. T., Abrahams, B. S., et al. (2009). Common genetic variants on 5p14.1 associate with autism spectrum disorders. Nature 459 (7246), 528–533. doi:10.1038/nature07999
Wang, S. S.-H., Kloth, A. D., and Badura, A. (2014). The cerebellum, sensitive periods, and autism. Neuron 83 (3), 518–532. doi:10.1016/j.neuron.2014.07.016
Wass, S. (2011). Distortions and disconnections: Disrupted brain connectivity in autism. Brain Cogn. 75 (1), 18–28. doi:10.1016/j.bandc.2010.10.005
Weiss, L. A., and Arking, D. E. (2009). A genome-wide linkage and association scan reveals novel loci for autism. Nature 461, 802. doi:10.1038/nature08490
Wen, T. H., Binder, D. K., Ethell, I. M., and Razak, K. A. (2018). The perineuronal ‘safety’ net? Perineuronal net abnormalities in neurological disorders. Front. Mol. Neurosci. 11, 270. doi:10.3389/fnmol.2018.00270
White, J. J., and Sillitoe, R. V. (2013). Development of the cerebellum: From gene expression patterns to circuit maps. Wiley Interdiscip. Rev. Dev. Biol. 2 (1), 149–164. doi:10.1002/wdev.65
White, K., Yang, P., Li, L., Farshori, A., Medina, A. E., and Zielke, H. R. (2018). Effect of postmortem interval and years in storage on RNA quality of tissue at a repository of the NIH NeuroBioBank. Biopreserv. Biobank. 16 (2), 148–157. doi:10.1089/bio.2017.0099
Whitney, E. R., Kemper, T. L., Bauman, M. L., Rosene, D. L., and Blatt, G. J. (2008). Cerebellar Purkinje cells are reduced in a subpopulation of autistic brains: A stereological experiment using calbindin-d28k. Cerebellum Lond. Engl. 7 (3), 406–416. doi:10.1007/s12311-008-0043-y
Willsey, A. J., Sanders, S. J., Li, M., Dong, S., Tebbenkamp, A. T., Muhle, R. A., et al. (2013). Coexpression networks implicate human midfetal deep cortical projection neurons in the pathogenesis of autism. Cell 155 (5), 997–1007. doi:10.1016/j.cell.2013.10.020
Wöhr, M., Orduz, D., Gregory, P., Moreno, H., Khan, U., Vörckel, K. J., et al. (2015). Lack of parvalbumin in mice leads to behavioral deficits relevant to all human autism core symptoms and related neural morphofunctional abnormalities. Transl. Psychiatry 5 (3), e525. doi:10.1038/tp.2015.19
Yip, J., Soghomonian, J.-J., and Blatt, G. J. (2007). Decreased GAD67 MRNA levels in cerebellar Purkinje cells in autism: Pathophysiological implications. Acta Neuropathol. 113 (5), 559–568. doi:10.1007/s00401-006-0176-3
Yip, J., Soghomonian, J.-J., and Blatt, G. J. (2008). Increased GAD67 MRNA expression in cerebellar interneurons in autism: Implications for Purkinje cell dysfunction. J. Neurosci. Res. 86 (3), 525–530. doi:10.1002/jnr.21520
Keywords: autism, cerebellum, purkinje cells, extracellular matrix, nervous system development, cell migration, axon guidance, immune function
Citation: Brandenburg C, Griswold AJ, Van Booven DJ, Kilander MBC, Frei JA, Nestor MW, Dykxhoorn DM, Pericak-Vance MA and Blatt GJ (2022) Transcriptomic analysis of isolated and pooled human postmortem cerebellar Purkinje cells in autism spectrum disorders. Front. Genet. 13:944837. doi: 10.3389/fgene.2022.944837
Received: 15 May 2022; Accepted: 11 August 2022;
Published: 09 November 2022.
Edited by:
Li Wang, University of California, San Francisco, United StatesReviewed by:
Xiameng Chen, Sichuan University, ChinaCopyright © 2022 Brandenburg, Griswold, Van Booven, Kilander, Frei, Nestor, Dykxhoorn, Pericak-Vance and Blatt. This is an open-access article distributed under the terms of the Creative Commons Attribution License (CC BY). The use, distribution or reproduction in other forums is permitted, provided the original author(s) and the copyright owner(s) are credited and that the original publication in this journal is cited, in accordance with accepted academic practice. No use, distribution or reproduction is permitted which does not comply with these terms.
*Correspondence: Cheryl Brandenburg, Y2JyYW5kZW5idXJnQHNvbS51bWFyeWxhbmQuZWR1
Disclaimer: All claims expressed in this article are solely those of the authors and do not necessarily represent those of their affiliated organizations, or those of the publisher, the editors and the reviewers. Any product that may be evaluated in this article or claim that may be made by its manufacturer is not guaranteed or endorsed by the publisher.
Research integrity at Frontiers
Learn more about the work of our research integrity team to safeguard the quality of each article we publish.