- 1Oncopathology Research Center, Iran University of Medical Sciences (IUMS), Tehran, Iran
- 2Department of Embryology, Reproductive Biomedicine Research Center, Royan Institute for Reproductive Biomedicine, ACECR, Tehran, Iran
- 3School of Biomedical Engineering, University of Technology Sydney, Sydney, NSW, Australia
- 4Department of Genetics, Reproductive Biomedicine Research Center, Royan Institute for Reproductive Biomedicine, ACECR, Tehran, Iran
- 5Department of Gynecologic Oncology, Vali-Asr Hospital, Tehran University of Medical Sciences, Tehran, Iran
- 6Department of Stem Cells and Developmental Biology, Cell Science Research Center, Royan Institute for Stem Cell Biology and Technology, ACECR, Tehran, Iran
- 7Department of Epidemiology, School of Public Health, Iran University of Medical Sciences, Tehran, Iran
- 8Department of Molecular Medicine, Faculty of Advanced Technologies in Medicine, Iran University of Medical Sciences, Tehran, Iran
Analysis of circulating tumor DNA (ctDNA) can be used to characterize and monitor cancers. Recently, non-invasive prenatal testing (NIPT) as a new next-generation sequencing (NGS)-based approach has been applied for detecting ctDNA. This study aimed to investigate the copy number variations (CNVs) utilizing the non-invasive prenatal testing in plasma ctDNA from ovarian cancer (OC) patients who were treated with neoadjuvant chemotherapy (NAC). The plasma samples of six patients, including stages II–IV, were collected during the pre- and post-NAC treatment that were divided into NAC-sensitive and NAC-resistant groups during the follow-up time. CNV analysis was performed using the NIPT via two methods “an open-source algorithm WISECONDORX and NextGENe software.” Results of these methods were compared in pre- and post-NAC of OC patients. Finally, bioinformatics tools were used for data mining from The Cancer Genome Atlas (TCGA) to investigate CNVs in OC patients. WISECONDORX analysis indicated fewer CNV changes on chromosomes before treatment in the NAC-sensitive rather than NAC-resistant patients. NextGENe data indicated that CNVs are not only observed in the coding genes but also in non-coding genes. CNVs in six genes were identified, including HSF1, TMEM249, MROH1, GSTT2B, ABR, and NOMO2, only in NAC-resistant patients. The comparison of these six genes in NAC-resistant patients with The Cancer Genome Atlas data illustrated that the total alteration frequency is amplification, and the highest incidence of the CNVs (≥35% based on TCGA data) is found in MROH1, TMEM249, and HSF1 genes on the chromosome (Chr) 8. Based on TCGA data, survival analysis showed a significant reduction in the overall survival among chemotherapy-resistant patients as well as a high expression level of these three genes compared to that of sensitive samples (all, p < 0.0001). The continued Chr8 study using WISECONDORX revealed CNV modifications in NAC-resistant patients prior to NAC therapy, but no CNV changes were observed in NAC-sensitive individuals. Our findings showed that low coverage whole-genome sequencing analysis used for NIPT could identify CNVs in ctDNA of OC patients before and after chemotherapy. These CNVs are different in NAC-sensitive and -resistant patients highlighting the potential application of this approach in cancer patient management.
Introduction
Ovarian cancer (OC) is a tumor with the worst prognosis among female malignancies, and most cases are diagnosed in the advanced stage with peritoneal metastases (Bray et al., 2018). Neoadjuvant chemotherapy (NAC) is the gold standard treatment for OC patients with a high perioperative risk profile and/or a poor chance of effective debulking surgery (Elies et al., 2018), although a considerable percentage of patients demonstrate resistance to NAC treatment (Sato and Itamochi, 2014). Considering that the administration of proper chemotherapy regimens is dependent on imaging tests, cytology, and laparoscopic biopsy (Lee et al., 2018), inadequate tumor specimens are a flaw and difficulty in cytology or laparoscopic biopsy prior to the onset of NAC (Sharbatoghli et al., 2020). Biomarkers related to drug resistance and treatment, including prognostic and predictive molecules, play a key role in selecting appropriate treatment protocols and improving survival rates (Le Page et al., 2010). In OC, several studies have demonstrated changes in the serum levels of cancer antigen 125 (CA125) which may serve as a predictor of monitoring the response to NAC (Pelissier et al., 2014; Zeng et al., 2016), but its utility is often limited (Sharbatoghli et al., 2020). Serum tumor markers do not exhibit a significant increase in some histological types of OC (Sharbatoghli et al., 2020). Since gene amplification and deletion are common in cancer cells and contribute to cancer cell growth, angiogenesis, and drug resistance (Matsui et al., 2013; Rahimi et al., 2020), recently, copy number variants (CNVs) have been reported as potential biomarkers in cancer management (Pan et al., 2019; Yu et al., 2019; Jin et al., 2021). Moreover, comparing gene expression, CNV is considerably more stable and robust (Pan et al., 2019; Shao et al., 2019) and the gain or loss of gene copies often correlates with a corresponding increase or decrease in the amount of RNA and protein encoded by the gene (Hastings et al., 2009). It is critical to accurately measure copy number alterations in tumor samples in order to enable translational research and precision medicine. The majority of the CNV investigations were conducted on tumor tissue biopsy samples (Vives-Usano et al., 2021). A fraction of cell-free DNA (cfDNA) in cancer patients originates from tumor cells, known as circulating tumor DNA (ctDNA), which is obtained through liquid biopsy. Liquid biopsy as a semi-invasive diagnostic and prognostic tool has the advantage of being less invasive than tumor biopsy, and specimens can be frequently checked in real-time (Mathai et al., 2019). It seems that the molecular alterations identified in ctDNA might mirror molecular heterogeneity of tumor compared to those reflected by tumor biopsy (Sharbatoghli et al., 2020). Therefore, ctDNA analysis was applied to detect various types of genomic alterations, such as CNVs, mutation, and nucleosome positioning variation in cancers (Molparia et al., 2017; Huang et al., 2019; Noguchi et al., 2020).
In recent years, cancer detection by non-invasive prenatal testing (NIPT) as a new next-generation sequencing (NGS)-based approach is used to detect ctDNA (Cohen et al., 2016; Nakabayashi et al., 2018). The low coverage of whole-genome sequencing of cfDNA from maternal plasma is the basis for prenatal screening of common fetal autosomal aneuploidies and trisomy of chromosomes 21, 18, and 13 utilizing NIPT. One of the most often publicized benefits of the NIPT for chromosomal abnormality screening in pregnant women is its low false-positive rate (1–3%) (Filoche et al., 2017). Regarding fast advances in the NIPT, analysis of tumor CNV changes in ctDNA using NIPT was introduced as a potential cancer screening tool. In this context, Amant et al. (2015) discovered cancer in three pregnant women who had NIPT and indicated that CNVs may be used as a cancer screening tool. Furthermore, the implications of the whole-genome NIPT platform for cancer screening were shown in OC patients (Cohen et al., 2016). Furthermore, CNV analysis in cell-free DNA by low coverage whole-genome sequencing was used as a biomarker for the diagnosis of OC (Vanderstichele et al., 2017). For the first time, we applied the NIPT as a non-invasive test platform to compare the tumor-derived CNV in ctDNA measured pre- and post-NAC in plasma samples obtained from OC patients. This is proof of the concept that NIPT might be useful for predicting responsive and resistant patients to chemotherapy.
Materials and Methods
Collection of Samples and Blood Processing
This study was conducted as a prospective study with 10 plasma samples derived from 6 OC patients of the Cancer Institute of Imam Khomeini Hospital (Tehran, Iran) between December 2018 and October 2019. This study was performed with the approval of the Ethics Committee of Iran University of Medical Sciences (authorization no: IR.IUMS.REC 1397.32825). Each participating hospital’s ethical norms required that written informed permission has to be acquired. The FIGO (International Federation of Gynecology and Obstetrics) stage (Bhatla and Denny, 2018) was used to histologically confirm the patients’ diagnosis. Blood samples were obtained in pre- and post-NAC treatment from OC patients that received platinum-based chemotherapy as an NAC regimen, at the first-line treatment. A week before the first dose of chemotherapy, baseline blood samples were collected, and post-NAC samples were taken after the first course (six cycles) of chemotherapy. The patients with complete response were defined as NAC-sensitive, while those with stable disease and progressive disease were defined as NAC-resistant (Noguchi et al., 2020). A total volume of 10 ml of whole blood was collected in K2EDTA-coated tubes (REF: CDLP 029, C.D. RICH®, Romania) from each patient. Then, the blood was centrifuged at 1,600×g for 15 min at 25°C, and the plasma fraction was collected and centrifuged for a second time at 2,500×g for 10 min at 25°C. After the second spin, the plasma was transferred into barcoded tubes and immediately stored at ≤-70°C. The cell-free DNA was extracted from 3 ml of patient plasma using the QIAamp Circulating Nucleic Acid Kit (Qiagen, Gaithersburg, MD, United States) (Diefenbach et al., 2018).
Library Preparation, Sequencing, and Data Analysis
DNA libraries were prepared from 2 ng of cell-free DNA extracted from 3 ml of plasma using the VeriSeq NIPT Solution v2 according to the manufacturer’s instructions for 75 bp single-end high via sequencing. All libraries were normalized to 1.6 nM, multiplexed, and sequenced on HiSeq: 4000 with 27 sequencing cycles of the cell-free DNA insert and an additional eight sequencing cycles for the index barcodes. Each research sample was sequenced alongside 12 clinical samples, with 36-cycle single-end sequencing on an Illumina NextSeq550. The read depth was low coverage at 0.2× to 0.3× based on the amount of sequencing data. The open-source algorithm WISECONDORX (WIthin-SamplE COpy Number Aberration DetectOR X) and NextGENe (Next GENeration sequencing software for biologists) were used for data analysis (Faircloth and Glenn, 2012).
Copy Number Variation Call Using WISECONDORX and NextGENe
WISECONDORX was used to identify whole chromosome (Chr) and subchromosomal abnormalities that the standard NIPT pipeline failed to detect (Raman et al., 2019). Segmental alterations of less than 0.05 Mb were prespecified as abnormal (“positive cancer screen”). FASTQC (Andrews, 2010) was used to do quality control on the raw single-end sequencing data. The BBduk tool from the BBmap toolbox (Bushnell, 2014) was used to trim and adjust the fastq files as needed.
Reads were mapped to the human reference genome (hg38) using bwa samse algorithm (Li and Durbin, 2009). The resulting sam files were subjected to corrections and converted to bam via samtools (Li et al., 2009). CNVs were called by WISECONDORX run on default settings suggested by the developer (Raman et al., 2018). NextGene software version 2.4.1 (SoftGenetics, LLC) was used for CNV analysis according to the Kerkhof et al. (2017) method.
After obtaining WISECONDORX and NextGENe data, an investigation of these CNV results in pre-treatment was performed on all patients. Moreover, a comparison of these data for patients within the NAC-sensitive and NAC-resistant groups were performed separately to obtain common alterations in chromosomes and gene levels for each group. Then, the data of WISECONDORX and NextGENe software from NAC-resistant were compared to NAC-sensitive patients in order to receive alteration genes detected in NAC resistance. Similarly, post-treatment results were compared in patients with OC in order to detect CNVs.
Data Mining for Genes Detected in NAC-Resistant Patients
To obtain a more comprehensive and deep understanding of the biological process and molecular function of genes detected in NAC resistance, Gene Ontology (GO) term enrichment analysis was performed (Ashburner et al., 2000). Furthermore, KEGG (Kanehisa and Goto, 2000), Reactome (Sidiropoulos et al., 2017), and WikiPathways (Slenter et al., 2018) were investigated to examine the biological pathways in which these genes are involved. GO enrichment and pathway analysis of the genes detected in NAC-resistant patients was revealed using the ClueGO plug-in using Cytoscape (Bindea et al., 2009). Furthermore, genes detected in NAC resistance were investigated in the cBio Cancer Genomics Portal (cBioPortal) database in order to further evaluate the alterations of these genes in OC tissue samples. cBioPortal is an open-access database providing visualization and analyzing tools for multidimensional cancer genomics data, such as The Cancer Genome Atlas (TCGA) (Cerami et al., 2012). The genes detected in NAC resistance with more copy number alteration frequency on CBioPortal were selected to evaluate the mRNA expression levels on Gene Expression Profiling Interactive Analysis (GEPIA2) for OC tissue data compared to normal tissues (Tang et al., 2019). GEPIA2 is an online database, including RNA sequence expression data based on tumor and normal samples from TCGA and the GTEx (Tang et al., 2019). Gene Set Cancer Analysis (GSCALite) was applied to investigate the correlation between mRNA expression and CNV in OC patients. Spearman correlation coefficients were reported using GSCALite software, a user-friendly web server for dynamic analysis and visualization of gene sets in cancer which will be of broad utility to cancer researchers (Liu et al., 2018) from TCGA (Liu et al., 2018). The Cancer Virtual Cohort Discovery Analyses Platform (CVCDAP) portal was also utilized to compare the overall survival (OS) analyses between chemo-resistant and chemo-sensitive patients based on mRNA expression levels of these genes (Guan et al., 2020). CVCDAP is a web-based platform to deliver an interactive and customizable toolbox off the shelf for cohort-level analysis of TCGA and CPTAC public datasets (Guan et al., 2020). Finally, a volcano plot was applied to evaluate the protein expression in OC chemotherapy-resistant patients compared to that in chemotherapy-sensitive patients by TCGAbiolinks, an R/Bioconductor package for integrative analysis of TCGA data (Colaprico et al., 2016).
Results
Patient Characteristics
The clinicopathological characteristics and response to NAC of the six OC patients are summarized in Table 1. The mean age of the patients was 54.3 years (38–78 years), and they included stages II, III, and IV high-grade serous ovarian carcinoma (HGSOC). Two of the six patients died undergoing chemotherapy. As a result, these two patients were unable to provide post-NAC plasma samples. Two patients were NAC-sensitive, while two cases were NAC-resistant and did not respond appropriately to chemotherapy treatment.

TABLE 1. Clinicopathological characteristics of six ovarian cancer patients that were treated with neoadjuvant chemotherapy.
Investigation of CNV From ctDNA in NAC-Resistant and NAC-Sensitive Patients With OC
We detected 6/6 HGSOC cases including early-stage (II) to late-stage (IV)—using the WISECONDORX (Table 2) and NextGENe analysis (Supplementary Tables S1–S3). WISECONDORX results indicated that most patients (at least 5 cases) have abnormality (gain and/or loss) on chromosomes, 4, 9, 18, and 22 before NAC treatment (Table 2). NextGENe data illustrated CNVs not only in coding genes but also alterations in duplication and deletion in non-coding genes (Supplementary Tables S1–S3). Chr changes detected by WISECONDORX software indicated that NAC-sensitive patients have fewer chromosomes’ CNV changes before treatment rather than NAC-resistant patients as shown in Figure 1; Table 2.
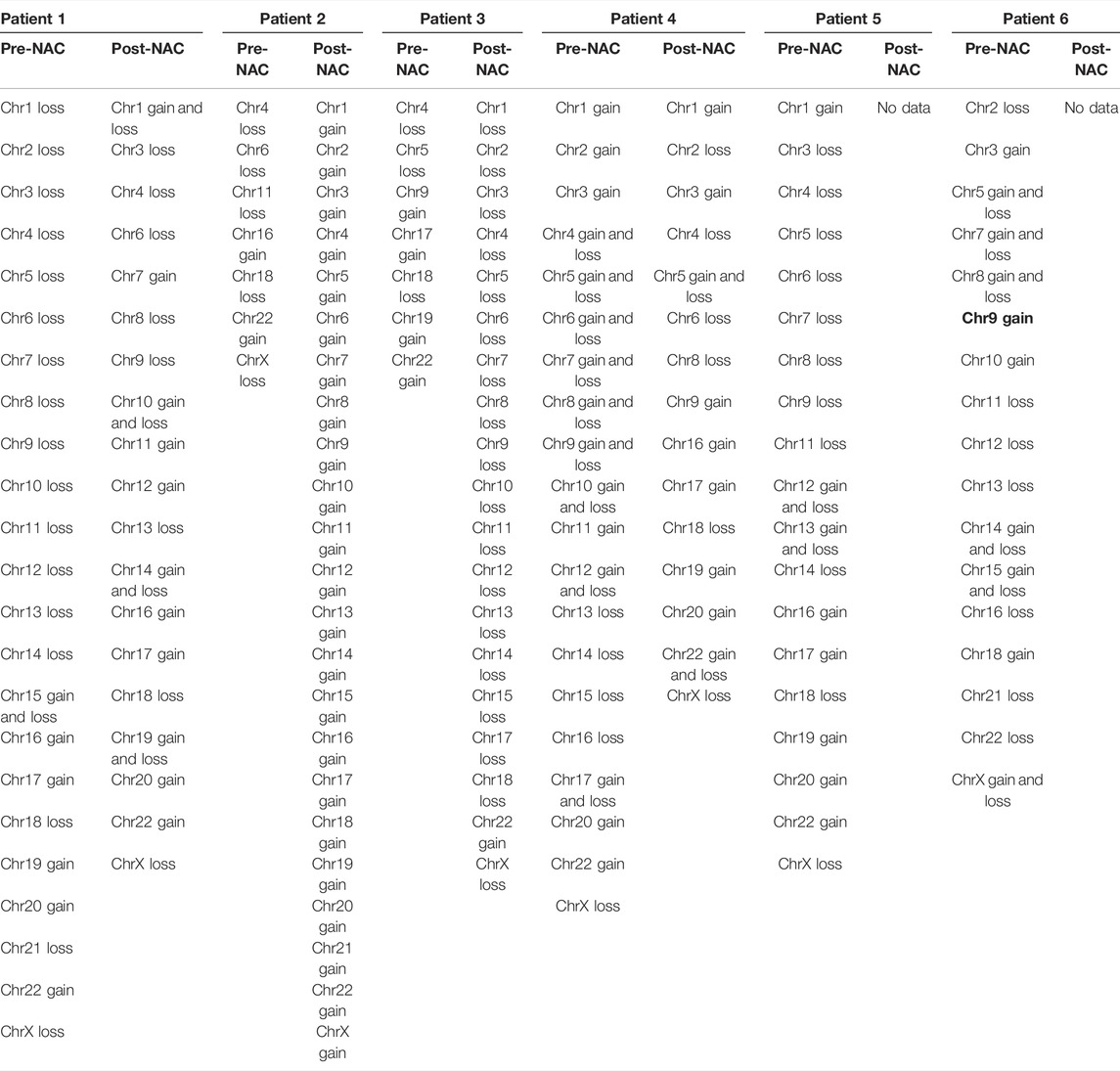
TABLE 2. Copy number variations (CNVs) in six ovarian cancer cases which were reported based on gains and losses of chromosomes by the WISECONDORX analysis.
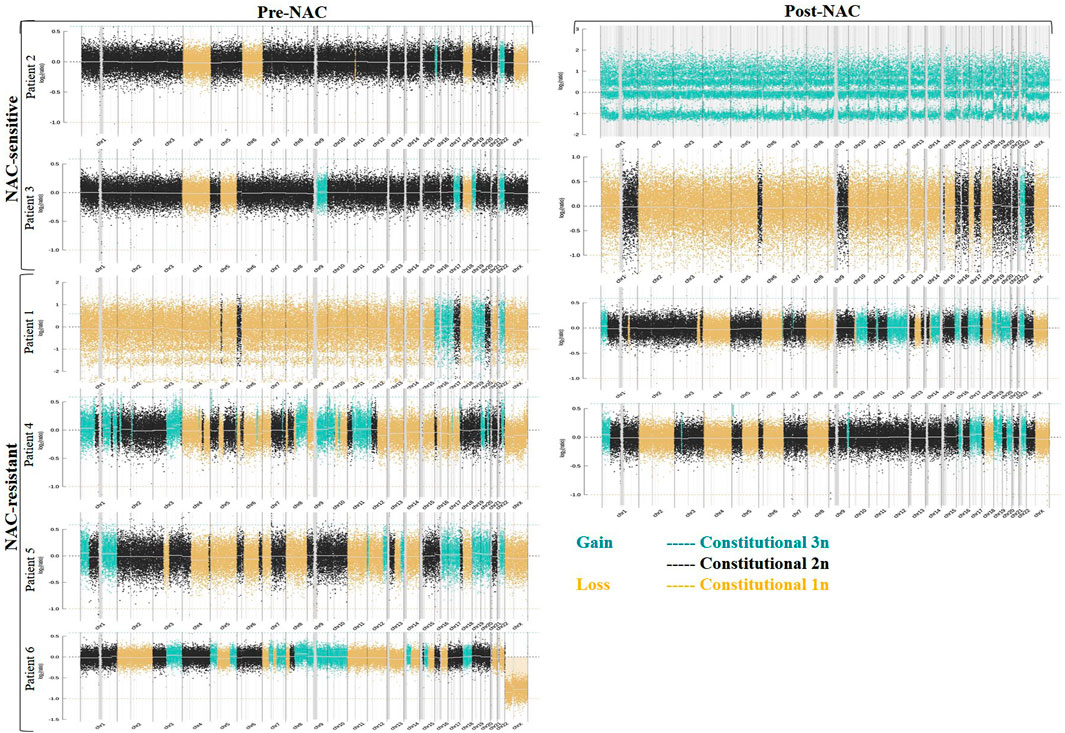
FIGURE 1. Copy number variations (CNVs) in ctDNA from six ovarian cancer (OC) patients that were treated with neoadjuvant chemotherapy (NAC). Data CNVs for all chromosomes based on WISECONDORX analysis were detected from NAC-sensitive, NAC-resistant, and dead patient groups. The copy numbers were segmented in blue (gain) and yellow (loss) lines.
Using the results of NextGENe software, common genes with copy number changes were assessed in pre-NAC and post-NAC treatment by the Vinny plot. We detected 17 common genes with CNV in pre-NAC patients from the NAC-resistant group (Figure 2A; Supplementary Table S4). Among these genes, six common genes, including NOMO2, ABR, GSTT2B, HSF1, TMEM249, and MROH1, exclusively have shown CNV in the NAC-resistant group, while in NAC-sensitive patients, none of these genes were altered before NAC treatment. Furthermore, as shown in Figure 2B; Supplementary Table S5, 38 common genes with CNV were discovered in NAC-resistant patients’ post-NAC data. Furthermore, we discovered 14 genes, including LOC285441, LOC100996414, TTLL10, NXN, SMA4, SMA5, NOMO1, TIMM22, NOMO2, HSF1, ABR, TMEM249, GSTT2B, and MROH1, that exclusively revealed CNVs in NAC-resistant patients following chemotherapy. We indicate the CNVs for the six genes (NOMO2, ABR, GSTT2B, HSF1, TMEM249, and MROH1) that were frequent in the NAC-resistant group pre- and post-treatment as genes associated and found in NAC-resistant patients (See Table 3).
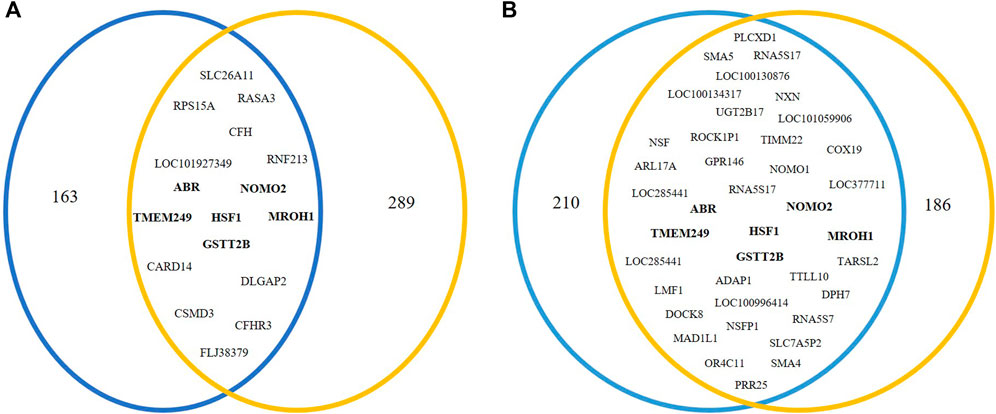
FIGURE 2. Venn diagram analysis to investigate genes related to NAC resistance. In NAC-resistant patients, Venn diagram indicated (A) 17 common genes in pre-NAC-treated patients and (B) 38 common genes in post-NAC-treated patients that were obtained via NextGENe analysis.
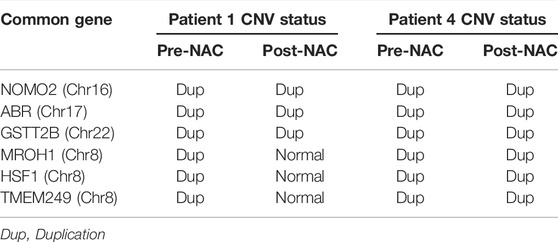
TABLE 3. Common genes in pre- and post-neoadjuvant chemotherapy (NAC) as well as its copy number variation (CNV) status (in pre-and post-NAC) for NAC-resistant patients that were detected by NextGENe software.
Data Mining Approaches for Genes Detected in NAC-Resistant Patients
The pathway enrichment analysis for six common genes detected in NAC-resistant patients indicated that GSTT2B was involved in chemical carcinogenesis and drug metabolism pathways, while ABR and HSF1 were enriched in p75 NTR receptor-mediating signaling and cellular response to heat stress/shock response pathways, respectively (Figure 3A). Gene Ontology analysis illustrated that ABR and HSF1 contributed to apoptosis and programmed cell death (GO: 0012501 and 0006915, respectively) (Supplementary Table S6). Moreover, ABR, GSTT2B, and HSF1 have a role in catalytic activity and metabolic processes (Figure 3B).
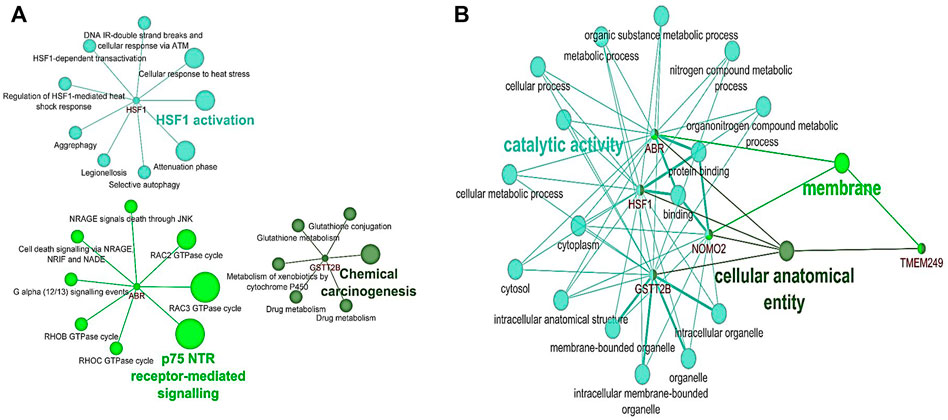
FIGURE 3. Pathway and Gene Ontology analysis for six genes detected in NAC-resistant patients using the ClueGO plugin in Cytoscape. (A) Pathway analysis based on KEGG, Reactome, and WikiPathways. (B) Common results of GO analysis for HSF1, ABR, and GSTT2B according to biological processes and molecular function.
Comparing our CNV results of six common genes detected in NAC-resistant patients with TCGA data through the cBioPortal illustrated that the total alteration frequency is amplification, similar to TCGA data from 585 OC patients (Figure 4). Moreover, the most alteration frequency of CNVs (≥35%) was observed in HSF1, TMEM249, and MROH1 genes from OC patients (Figure 4).
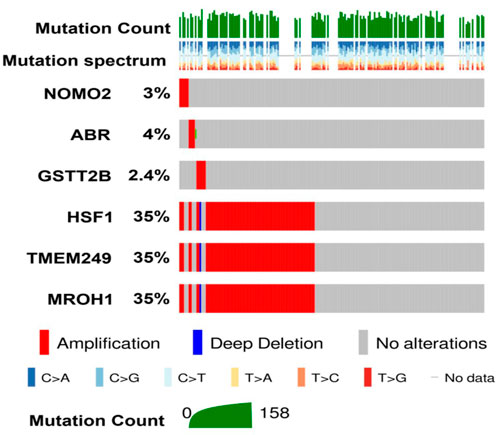
FIGURE 4. Investigation of DNA alterations for six genes detected in NAC resistance in TCGA-ovarian cancer patients on cBioPortal. Oncoplot illustrates the amplification status of six different genes identified in resistant patients across 585 ovarian carcinoma patients from TCGA. Among them, high amplification of HSF1, TMEM249, and MROH1 was observed compared to other identified genes.
Loci of HSF1, TMEM249, and MROH1 genes are arranged on Chr8. CNV alterations in Chr8 in WISECONDORX data were solely found in NAC-resistant patients when compared to NAC-sensitive patients before treatment (Table.2). Investigation of these three genes at the mRNA level on GEPIA2 showed significantly high expression of HSF1, TMEM249, and MROH1 in ovarian serous carcinoma (OSC) compared with normal tissues (all, p < 0.05) (Figure 5A–C). Moreover, the Spearman correlation coefficient shows the positive association between CNVs and mRNA expression of HSF1 (Cor = 0.83, FDR = 6e-74), MROH1 (Cor = 0.72, FDR = 4.8e-49), and TMEM249 (Cor = 0.48, FDR = 3.11e-18) across 585 OC patients from TCGA (Figure 6). As shown in Figure 7, the volcano plot indicates the enrichment of TMEM249, MROH1, and HSF1 protein expression in resistant patients to chemotherapy from TCGA. In other words, the expression of these three proteins significantly increased in resistant patients (n = 90) versus sensitive patients to chemotherapy (n = 194) (all, p < 0.05).
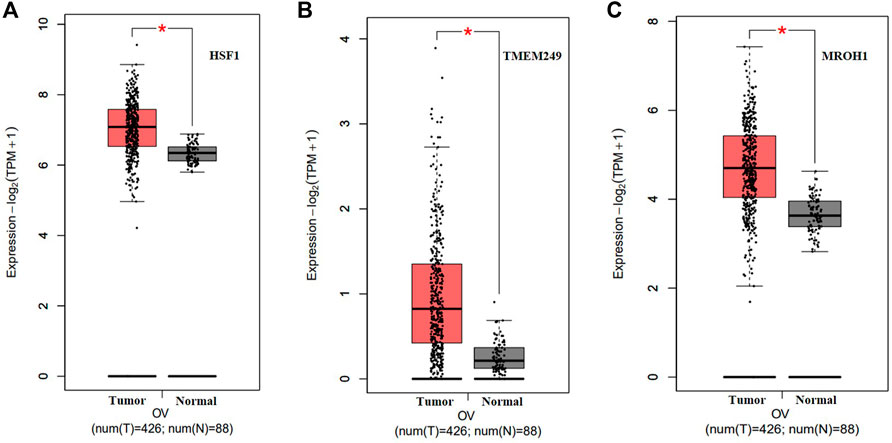
FIGURE 5. mRNA levels of three genes related to NAC resistance in serous ovarian carcinoma (SOC) on Gene Expression Profiling Interactive Analysis2 (GEPIA2). Upregulated expression of (A) HSF1, (B) TMEM249, and (C) MROH1 in mRNA levels significantly for SOC compared with that for normal tissue by GEPIA2 was observed (*: p < 0.05).
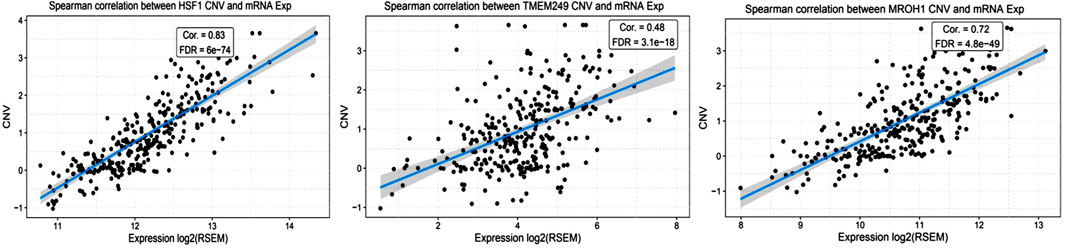
FIGURE 6. Correlation study between mRNA levels and copy number variation of three genes related to NAC resistance through cBioPortal in TCGA-ovarian cancer patients. The correlation coefficient analysis shows the positive association between mRNA expression and copy number variation of HSF1, TMEM249, and MROH1 across 585 ovarian cancer patients from TCGA.
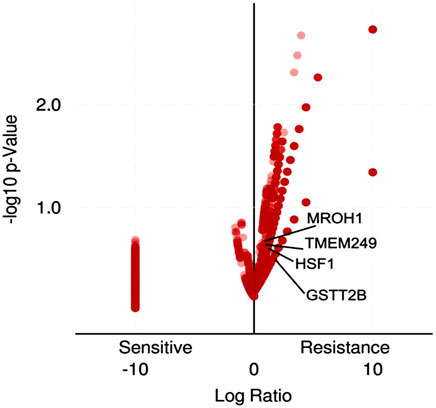
FIGURE 7. Volcano plot enrichment analysis for gene-related protein expression in ovarian cancer patients who were treated with chemotherapy from TCGA using TCGAbiolinks. The expression of HSF1, TMEM249, and MROH1 proteins significantly increased in resistant patients (n = 90) versus sensitive patients to chemotherapy (n = 194).
Survival analyses showed a significant reduction in OS across patients resistant to chemotherapy and a high expressed level of HSF1, TMEM249, and MROH1 compared to sensitive samples (all, p < 0.0001). Moreover, these data illustrate that high expression of MROH1 and TMEM249 significantly in resistant and sensitive samples can reduce OS among these patients (all, p < 0.0001) (Figure 8).
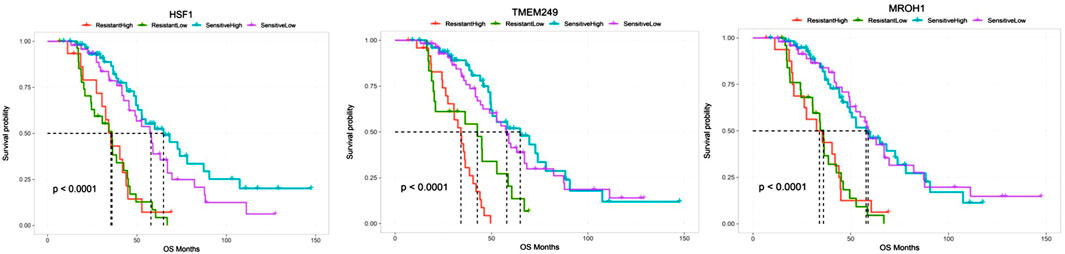
FIGURE 8. Comparing the overall survival between chemo-resistant and -sensitive expression of HSF1, TMEM249, and MROH1 using the CVCDAP portal for ovarian cancer patients. The plot shows a significant reduction in the overall survival across patients resistant to chemotherapy and expressed a high level of HSF1, TMEM249, and MROH1 genes in comparison with sensitive samples. Moreover, the plot indicates that high expression of MROH1 and TMEM249 in chemo-sensitive samples can reduce OS among these patients.
Discussion
ctDNA from liquid biopsy has recently been shown to be a useful diagnostic tool in a variety of cancer patients (Saha et al., 2022). Indeed, it has been shown that ctDNA is correlated to tumor burden and the risk of recurrence (Fleischhacker and Schmidt, 2007). ctDNA as part of total body DNA from cancer cells is superior to other plasma biomarkers such as RNA and protein. It is more stable than RNA (Panawala, 2017) with more sensitivity and clinical correlations. Despite the fact that plasma protein biomarkers are commonly used in clinical management for different cancers, such as AFP, CEA, PSA, and CA15-3 (Mazzucchelli et al., 2000; He et al., 2013), some cancer patients are not positive for these biomarkers. Furthermore, they are found with lower concentrations in the serum of individuals free of cancer (Cheng et al., 2016), while the studies show that ctDNA can more accurately reflect the real-time tumor burden in patients receiving therapy (Bettegowda et al., 2014).
The current study is the first report to indicate the utility of analyzing CNVs in ctDNA using massively parallel sequencing (such as NIPT), before and after NAC treatment in OC patients. The previous study by Fleischhacker and Schmidt, (2007) has shown that ctDNA is low in the early stages of the tumor and difficult to detect. The method in this study can detect copy number alterations of ctDNA in various stages of HGSOC patients, including stages II–IV, through low coverage plasma DNA sequencing and analysis for chromosomal CNVs ≥0.5 Mb. It has previously been demonstrated that tumor DNA from cancer cells has been detected in plasma using NIPT (Amant et al., 2015; Bianchi et al., 2015; Cohen et al., 2016). We also investigated the response to chemotherapy and depicted the CNV changes in NAC-sensitive and NAC-resistant patients. CNV burden in all chromosomes of NAC-sensitive patients was fewer than that of NAC-resistant patients before NAC treatment. Since the CNVs are considered a key factor in the genetic variation of tumors (Hu et al., 2021), it seems that less CNV burden indicated better response in treating patients (Walker et al., 2017). CNVs were also discovered in a variety of genes following chemotherapy, notably in genes that control drug absorption into cells and drug metabolism (Willyard, 2015). As a result, we compared produced CNVs in NAC-sensitive and NAC-resistant patient groups with OC following NAC therapy. After the first course of chemotherapy, the CNV load increased in both NAC-sensitive and NAC-resistant individuals, according to our findings. This phenomenon could be in terms of the death of tumor cells (DNA damage) (Woods and Turchi, 2013) or resistance to therapy (Alfarouk et al., 2015).
The previous studies have suggested the role of CNVs in genes related to drug resistance (Alfarouk et al., 2015; Willyard, 2015; Costa et al., 2017; Qidwai, 2020). Our findings appear to well indicate that some of the CNVs detected by the NIPT are different in NAC-resistant groups compared to NAC-sensitive groups of OC patients. We found some common genes, including NOMO2, ABR, GSTT2B, HSF1, TMEM249, and MROH1, in the pre- and post-treatment for the NAC-resistant group which was no longer detected in NAC-sensitive patients before and after therapy. These findings suggest that CNVs discovered by the NIPT may contribute to treatment resistance. To confirm the presence of CNV in all patients’ plasma, we matched the findings of genes with CNVs discovered in their plasma to the tissue samples in the TCGA data (Bell et al., 2011) owing to the unavailability of tissue specimens from these patients. It is better to investigate the plasma sequencing data with paired tumor DNA of tissue samples, but the lack of tissue or insufficient tumor tissue sampling of patients is a limitation for this type of study (Cohen et al., 2016). The cBioPortal findings indicated the existence of alteration and amplification in all six genes among TCGA patients so that some of these CNVs have a high frequency in OC tissue samples. Moreover, HSF1, TMEM249, and MROH1 are located on Chr8 which is highlighted in our study for OC patients. The CNVs’ investigation via WISECONDOREX shows Chr8 underwent CNV changes in NAC-resistant patients before NAC treatment, while in NAC-sensitive patients no CNV changes were found on Chr8 before chemotherapy. Amplification of Chr8 genes has also been identified as a recurrent genomic event in lung cancer (Baykara et al., 2015) and malignant peripheral nerve sheath tumor (MPNST) (Dehner et al., 2021). This finding indicates the power role and relationship among CNV alterations on Chr8 and NAC-resistant in OC patients. Other studies showed that approximately 80 genes on Chr8 are involved in cancer biology (Tabarés-Seisdedos and Rubenstein, 2009). We investigated the levels of RNA and protein expression from HSF1, TMEM249, and MROH1, which are related to NAC-resistant patients on TCGA data. DNA copy number variation is an important factor in the expression of genes (Gamazon and Stranger, 2015) and also an important influential factor for the expression of both protein-coding and non-coding genes (Liang et al., 2016). As expected, positive correlations between the level of mRNA and CNVs alterations were observed for HSF1, TMEM249, and MROH1. In this regard, survival analysis confirmed the influence expression of these three genes in the survival rate, so that resistant patients with higher mRNA expression of these genes had a reduced OS. Furthermore, in TCGA-chemotherapy-resistant OC patients, the protein expression of these genes was higher than that in sensitive individuals. So far, dysregulation and different roles of these genes in various cancers have been evaluated. Studies indicated that HSF1 has been implicated in tumorigenesis by its participation in cellular stress response pathways and its effect on regulatory pathways such as p53, mTOR, and insulin signaling (Vihervaara and Sistonen, 2014; Vydra et al., 2014; Powell et al., 2016; Barna et al., 2018). In line with our in silico analysis study, high levels of HSF1 have been identified in different types of cancers (Chen et al., 2021). Overexpression of HSF1 in tumor tissues is correlated with a worse prognosis in cancer patients (Kourtis et al., 2015). Regarding the correlation between a high level of HSF1 and the deterioration of disease in the initiation, promotion, and progression of cancer (Wang et al., 2020), HSF1 could act as a potential therapeutic target (Wang et al., 2020). OC studies reported that HSF1 induces epithelial–mesenchymal transition (EMT) in the in vitro models (Powell et al., 2016) and targeting HSF1 leads to an antitumor effect (Chen et al., 2017). Zhang et al. (2017) identified some parts of human Chr8, a location hotspot, mediated by the master regulator HSF1 in different cancers. Furthermore, they interestingly indicated MROH1 and TMEM249 immediately flanking the upstream and downstream regions of HSF1. As predicted, our CNV data for Chr8 genes, such as HSF1, MROH1, and TMEM249, were duplicated in individuals who were resistant to NAC. Considering HSF1’s critical involvement in cancer development, the lack of HSF1 in NAC-sensitive patients compared to NAC-resistant patients suggests a function for the Chr8 and HSF1 genes in NAC resistance. The activity of the GSTT2 enzyme is important for the protection of cells against toxic products of oxygen and lipid peroxidation (Tan and Board, 1996), which represents a major source of endogenous DNA damage in humans that contributes significantly to cancer and other genetic diseases (Marnett, 2002). Duplicated-CNV and also mRNA expression of GSTT2B in NAC-resistant patients by bioinformatics analysis are consistent with the previous cancer studies (Pool-Zobel et al., 2005; Doherty et al., 2014). Research on colon cancer cells indicated upregulated GSTT2 upon incubation with butyrate that is involved in defense against oxidative stress (Pool-Zobel et al., 2005). Furthermore, Doherty et al. (2014) observed that the expression of GSTT2 was increased in drug-resistant cervical cell models with cisplatin treatment.
Deletion of ABR indicated a tumor-suppressive role in several solid tumors, such as medulloblastoma (McDonald et al., 1994), astrocytomas (Willert et al., 1995), and breast cancer (Liscia et al., 1999). In acute myeloid leukemia, the ABR gene was detected as a prognostic factor in which the blockage of ABR prevents myeloid differentiation (Namasu et al., 2017). In our study, the ABR gene was duplicated in NAC-resistant patients before and after treatment. These data suggested more investigation into the role and function of the ABR gene that might influence resistance to chemotherapy for OC patients.
The NOMO2 gene is known as a diagnostic biomarker in radioresistance in human H460 lung cancer stem-like cells (Yun et al., 2016). We found NOMO2 duplication in NAC-resistant patients by NIPT. These data are consistent with the previous result in metastatic breast cancer by NGS that detected mutation or amplification in cfDNA samples (Page et al., 2017). CNVs were not only found in coding genes but also detected in non-coding genes, such as microRNA and long non-coding genes, although the previous study in bladder cancer indicated CNV alteration in long non-coding RNAs, which can be used as prognostic biomarkers for bladder cancer (Zhong et al., 2021). Furthermore, copy number changes in non-coding RNAs have been identified as prospective therapeutic targets and prognostic markers in lung squamous cell carcinoma (LUSC) (Ning et al., 2021). We are aware that our research has limitations to describe the biological behavior of these CNVs and their relationships with NAC resistance in OC cells. Although the number of records was adequate to establish a conclusion for the trend in data, however, in terms of seeking generalizability, larger sample sizes are strongly suggested, which could be covered via larger and multicenter investigations.
Conclusion
This study’s findings highlighted low-coverage whole-genome sequencing analysis to investigate CNV changes in ctDNA. It seems that detected CNVs through the NIPT in ctDNA could be potential markers of clinical response to NAC treatment. Our results gave a clue that some alterations in the copy number of genes at the DNA level may relate to being the response to NAC treatment in OC patients, although further studies are warranted to understand the role of these CNVs in NAC-resistant patients. Using a platform for prenatal testing in diagnosis or monitoring therapeutic response for other cancer types as a novel way may hold promise that it should be examined.
Data Availability Statement
The data presented in the study are deposited in the European Nucleotide Archive (ENA) at EMBL-EBI under accession number PRJEB53061. (https://www.ebi.ac.uk/ena/browser/view/PRJEB53061).
Ethics Statement
The studies involving human participants were reviewed and approved by the Iran University of Medical Sciences Human Research Ethics Committee in Iran (Ref No: IR.IUMS.REC1397.32825). All procedures performed in this study were in accordance with the 1964 Helsinki Declaration and its later amendments. The patients/participants provided their written informed consent to participate in this study.
Author Contributions
ZM, MA-L, and MT designed and supervised the work; MS, a Ph.D. student, performed all examinations, collected the patient’s samples and data, and also wrote the manuscript; FF participated in data mining and literature review using bioinformatics tools, wrote the bioinformatics sections, prepared the related figures, and assisted in the editing of the manuscript; HA participated in bioinformatics data analysis and in editing the manuscript; AA participated in NGS data analysis and wrote the method section; SA contributed to sample collection; all authors read and approved the final manuscript.
Funding
This work was supported by a grant from the Iran University of Medical Sciences (#32825) and Royan Institute (#97000072).
Conflict of Interest
The authors declare that the research was conducted in the absence of any commercial or financial relationships that could be construed as a potential conflict of interest.
Publisher’s Note
All claims expressed in this article are solely those of the authors and do not necessarily represent those of their affiliated organizations, or those of the publisher, the editors, and the reviewers. Any product that may be evaluated in this article, or claim that may be made by its manufacturer, is not guaranteed or endorsed by the publisher.
Acknowledgments
The authors are so grateful to their colleagues at the Iran University of Medical Sciences and Royan Stem Cell Technology Company for their financial and technical support and Tehran University of Medical Sciences (Imam Khomeini hospital) for support in collecting samples.
Supplementary Material
The Supplementary Material for this article can be found online at: https://www.frontiersin.org/articles/10.3389/fgene.2022.938985/full#supplementary-material
References
Alfarouk, K. O., Stock, C.-M., Taylor, S., Walsh, M., Muddathir, A. K., Verduzco, D., et al. (2015). Resistance to Cancer Chemotherapy: Failure in Drug Response from ADME to P-Gp. Cancer Cell Int. 15, 71. doi:10.1186/s12935-015-0221-1
Amant, F., Verheecke, M., Wlodarska, I., Dehaspe, L., Brady, P., Brison, N., et al. (2015). Presymptomatic Identification of Cancers in Pregnant Women During Noninvasive Prenatal Testing. JAMA Oncol. 1 (6), 814–819. doi:10.1001/jamaoncol.2015.1883
Andrews, S. (2010). “FastQC: A Quality Control Tool for High Throughput Sequence Data,” in Babraham Bioinformatics (Cambridge, United Kingdom: Babraham Institute).
Ashburner, M., Ball, C. A., Blake, J. A., Botstein, D., Butler, H., Cherry, J. M., et al. (2000). Gene Ontology: Tool for the Unification of Biology. Nat. Genet. 25 (1), 25–29. doi:10.1038/75556
Barna, J., Csermely, P., and Vellai, T. (2018). Roles of Heat Shock Factor 1 beyond the Heat Shock Response. Cell. Mol. Life Sci. 75 (16), 2897–2916. doi:10.1007/s00018-018-2836-6
Baykara, O., Bakir, B., Buyru, N., Kaynak, K., and Dalay, N. (2015). Amplification of Chromosome 8 Genes in Lung Cancer. J. Cancer 6 (3), 270–275. doi:10.7150/jca.10638
Bell, D., Berchuck, A., Birrer, M., Chien, J., Cramer, D. W., Dao, F., et al. (2011). Integrated Genomic Analyses of Ovarian Carcinoma. Nature 474 (7353), 609–615. doi:10.1038/nature10166
Bettegowda, C., Sausen, M., Leary, R. J., Kinde, I., Wang, Y., Agrawal, N., et al. (2014). Detection of Circulating Tumor DNA in Early- and Late-Stage Human Malignancies. Sci. Transl. Med. 6 (224), 224ra24. doi:10.1126/scitranslmed.3007094
Bhatla, N., and Denny, L. (2018). FIGO Cancer Report 2018. Int. J. Gynecol. Obstet. 143 Suppl 2, 2–3. doi:10.1002/ijgo.12608
Bianchi, D. W., Chudova, D., Sehnert, A. J., Bhatt, S., Murray, K., Prosen, T. L., et al. (2015). Noninvasive Prenatal Testing and Incidental Detection of Occult Maternal Malignancies. JAMA 314 (2), 162–169. doi:10.1001/jama.2015.7120
Bindea, G., Mlecnik, B., Hackl, H., Charoentong, P., Tosolini, M., Kirilovsky, A., et al. (2009). ClueGO: A Cytoscape Plug-In to Decipher Functionally Grouped Gene Ontology and Pathway Annotation Networks. Bioinformatics 25 (8), 1091–1093. doi:10.1093/bioinformatics/btp101
Bray, F., Ferlay, J., Soerjomataram, I., Siegel, R. L., Torre, L. A., and Jemal, A. (2018). Global Cancer Statistics 2018: GLOBOCAN Estimates of Incidence and Mortality Worldwide for 36 Cancers in 185 Countries. CA A Cancer J. Clin. 68 (6), 394–424. doi:10.3322/caac.21492
Bushnell, B. (2014). BBMap: A Fast, Accurate, Splice-Aware Aligner. US: Lawrence Berkeley National Laboratory. Retrieved from: https://escholarship.org/uc/item/1h3515gn LBNL Report #: LBNL-7065E.
Cerami, E., Gao, J., Dogrusoz, U., Gross, B. E., Sumer, S. O., Aksoy, B. A., et al. (2012). The cBio Cancer Genomics Portal: An Open Platform for Exploring Multidimensional Cancer Genomics Data. Cancer Discov. 2 (5), 401–404. doi:10.1158/2159-8290.cd-12-0095
Chen, F., Fan, Y., Cao, P., Liu, B., Hou, J., Zhang, P., et al. (2021). Pan-Cancer Analysis of the Prognostic and Immunological Role of HSF1: A Potential Target for Survival and Immunotherapy. Oxid. Med. Cell Longev. 2021, 5551036. doi:10.1155/2021/5551036
Chen, Y.-F., Wang, S.-Y., Yang, Y.-H., Zheng, J., Liu, T., and Wang, L. (2017). Targeting HSF1 Leads to an Antitumor Effect in Human Epithelial Ovarian Cancer. Int. J. Mol. Med. 39 (6), 1564–1570. doi:10.3892/ijmm.2017.2978
Cheng, F., Su, L., and Qian, C. (2016). Circulating Tumor DNA: A Promising Biomarker in the Liquid Biopsy of Cancer. Oncotarget 7 (30), 48832–48841. doi:10.18632/oncotarget.9453
Cohen, P. A., Flowers, N., Tong, S., Hannan, N., Pertile, M. D., and Hui, L. (2016). Abnormal Plasma DNA Profiles in Early Ovarian Cancer Using a Non-invasive Prenatal Testing Platform: Implications for Cancer Screening. BMC Med. 14 (1), 126. doi:10.1186/s12916-016-0667-6
Colaprico, A., Silva, T. C., Olsen, C., Garofano, L., Cava, C., Garolini, D., et al. (2016). TCGAbiolinks: An R/Bioconductor Package for Integrative Analysis of TCGA Data. Nucleic Acids Res. 44 (8), e71. doi:10.1093/nar/gkv1507
Costa, G. L., Amaral, L. C., Fontes, C. J. F., Carvalho, L. H., de Brito, C. F. A., and de Sousa, T. N. (2017). Assessment of Copy Number Variation in Genes Related to Drug Resistance in Plasmodium Vivax and Plasmodium Falciparum Isolates from the Brazilian Amazon and a Systematic Review of the Literature. Malar. J. 16 (1), 152. doi:10.1186/s12936-017-1806-z
Dehner, C., Moon, C. I., Zhang, X., Zhou, Z., Miller, C., Xu, H., et al. (2021). Chromosome 8 Gain Is Associated with High-Grade Transformation in MPNST. JCI insight 6 (6), e146351. doi:10.1172/jci.insight.146351
Diefenbach, R. J., Lee, J. H., Kefford, R. F., and Rizos, H. (2018). Evaluation of Commercial Kits for Purification of Circulating Free DNA. Cancer Genet. 228-229, 21–27. doi:10.1016/j.cancergen.2018.08.005
Doherty, B., Lawlor, D., Gillet, J. P., Gottesman, M., O'Leary, J. J., and Stordal, B. (2014). Collateral Sensitivity to Cisplatin in KB-8-5-11 Drug-Resistant Cancer Cells. Anticancer Res. 34 (1), 503–507.
Elies, A., Rivière, S., Pouget, N., Becette, V., Dubot, C., Donnadieu, A., et al. (2018). The Role of Neoadjuvant Chemotherapy in Ovarian Cancer. Expert Rev. Anticancer Ther. 18 (6), 555–566. doi:10.1080/14737140.2018.1458614
Faircloth, B. C., and Glenn, T. C. (2012). Not All Sequence Tags Are Created Equal: Designing and Validating Sequence Identification Tags Robust to Indels. PloS one 7, e42543. doi:10.1371/journal.pone.0042543
Filoche, S., Lawton, B., Beard, A., Dowell, A., and Stone, P. (2017). New Screen on the Block: Non-invasive Prenatal Testing for Fetal Chromosomal Abnormalities. J. Prim. Health Care 9 (4), 248–253. doi:10.1071/hc16055
Fleischhacker, M., and Schmidt, B. (2007). Circulating Nucleic Acids (CNAs) and Cancer-A Survey. Biochimica Biophysica Acta (BBA) - Rev. Cancer 1775 (1), 181–232. doi:10.1016/j.bbcan.2006.10.001
Gamazon, E. R., and Stranger, B. E. (2015). The Impact of Human Copy Number Variation on Gene Expression: Figure 1. Briefings Funct. genomics 14 (5), 352–357. doi:10.1093/bfgp/elv017
Guan, X., Cai, M., Du, Y., Yang, E., Ji, J., and Wu, J. (2020). CVCDAP: An Integrated Platform for Molecular and Clinical Analysis of Cancer Virtual Cohorts. Nucleic Acids Res. 48 (W1), W463–w471. doi:10.1093/nar/gkaa423
Hastings, P. J., Lupski, J. R., Rosenberg, S. M., and Ira, G. (2009). Mechanisms of Change in Gene Copy Number. Nat. Rev. Genet. 10 (8), 551–564. doi:10.1038/nrg2593
He, C.-Z., Zhang, K.-H., Li, Q., Liu, X.-H., Hong, Y., and Lv, N.-H. (2013). Combined Use of AFP, CEA, CA125 and CAl9-9 Improves the Sensitivity for the Diagnosis of Gastric Cancer. BMC Gastroenterol. 13 (1), 87. doi:10.1186/1471-230x-13-87
Hu, W., Li, M., Zhang, Q., Liu, C., Wang, X., Li, J., et al. (2021). Establishment of a Novel CNV-Related Prognostic Signature Predicting Prognosis in Patients with Breast Cancer. J. Ovarian Res. 14 (1), 103. doi:10.1186/s13048-021-00823-y
Huang, C.-C., Du, M., and Wang, L. (2019). Bioinformatics Analysis for Circulating Cell-Free DNA in Cancer. Cancers 11 (6), 805. doi:10.3390/cancers11060805
Jin, X., Yan, J., Chen, C., Chen, Y., and Huang, W-K. (2021). Integrated Analysis of Copy Number Variation, Microsatellite Instability, and Tumor Mutation Burden Identifies an 11-Gene Signature Predicting Survival in Breast Cancer. Front. Cell Dev. Biol. 9, 721505. doi:10.3389/fcell.2021.721505
Kanehisa, M., and Goto, S. (2000). KEGG: Kyoto Encyclopedia of Genes and Genomes. Nucleic Acids Res. 28 (1), 27–30. doi:10.1093/nar/28.1.27
Kerkhof, J., Schenkel, L. C., Reilly, J., McRobbie, S., Aref-Eshghi, E., Stuart, A., et al. (2017). Clinical Validation of Copy Number Variant Detection from Targeted Next-Generation Sequencing Panels. J. Mol. Diagnostics 19 (6), 905–920. doi:10.1016/j.jmoldx.2017.07.004
Kourtis, N., Moubarak, R. S., Aranda-Orgilles, B., Lui, K., Aydin, I. T., Trimarchi, T., et al. (2015). FBXW7 Modulates Cellular Stress Response and Metastatic Potential through HSF1 Post-translational Modification. Nat. Cell Biol. 17 (3), 322–332. doi:10.1038/ncb3121
Le Page, C., Huntsman, D. G., Provencher, D. M., and Mes-Masson, A.-M. (2010). Predictive and Prognostic Protein Biomarkers in Epithelial Ovarian Cancer: Recommendation for Future Studies. Cancers 2 (2), 913–954. doi:10.3390/cancers2020913
Lee, Y. J., Chung, Y. S., Lee, J. Y., Nam, E. J., Kim, S. W., Kim, S., et al. (2018). Impact of Increased Utilization of Neoadjuvant Chemotherapy on Survival in Patients with Advanced Ovarian Cancer: Experience from a Comprehensive Cancer Center. J. Gynecol. Oncol. 29 (4), e63. doi:10.3802/jgo.2018.29.e63
Li, H., and Durbin, R. (2009). Fast and Accurate Short Read Alignment with Burrows-Wheeler Transform. Bioinformatics 25 (14), 1754–1760. doi:10.1093/bioinformatics/btp324
Li, H., Handsaker, B., Wysoker, A., Fennell, T., Ruan, J., Homer, N., et al. (2009). The Sequence Alignment/Map Format and SAMtools. Bioinformatics 25 (16), 2078–2079. doi:10.1093/bioinformatics/btp352
Liang, L., Fang, J.-Y., and Xu, J. (2016). Gastric Cancer and Gene Copy Number Variation: Emerging Cancer Drivers for Targeted Therapy. Oncogene 35 (12), 1475–1482. doi:10.1038/onc.2015.209
Liscia, D. S., Morizio, R., Venesio, T., Palenzona, C., Donadio, M., and Callahan, R. (1999). Prognostic Significance of Loss of Heterozygosity at Loci on Chromosome 17p13.3-ter in Sporadic Breast Cancer Is Evidence for a Putative Tumour Suppressor Gene. Br. J. Cancer 80 (5-6), 821–826. doi:10.1038/sj.bjc.6690427
Liu, C.-J., Hu, F.-F., Xia, M.-X., Han, L., Zhang, Q., and Guo, A.-Y. (2018). GSCALite: A Web Server for Gene Set Cancer Analysis. Bioinformatics 34 (21), 3771–3772. doi:10.1093/bioinformatics/bty411
Marnett, L. J. (2002). Oxy Radicals, Lipid Peroxidation and DNA Damage. Toxicology 181-182, 219–222. doi:10.1016/s0300-483x(02)00448-1
Mathai, R., Vidya, R., Reddy, B., Thomas, L., Udupa, K., Kolesar, J., et al. (2019). Potential Utility of Liquid Biopsy as a Diagnostic and Prognostic Tool for the Assessment of Solid Tumors: Implications in the Precision Oncology. J. Clin. Med. 8 (3), 373. doi:10.3390/jcm8030373
Matsui, A., Ihara, T., Suda, H., Mikami, H., and Semba, K. (2013). Gene Amplification: Mechanisms and Involvement in Cancer. Biomol. Concepts 4 (6), 567–582. doi:10.1515/bmc-2013-0026
Mazzucchelli, R., Colanzi, P., Pomante, R., Muzzonigro, G., and Montironi, R. (2000). Prostate Tissue and Serum Markers. Adv. Clin. Path 4 (3), 111–120.
McDonald, J. D., Daneshvar, L., Willert, J. R., Matsumura, K., Waldman, F., and Cogen, P. H. (1994). Physical Mapping of Chromosome 17p13.3 in the Region of a Putative Tumor Suppressor Gene Important in Medulloblastoma. Genomics 23 (1), 229–232. doi:10.1006/geno.1994.1481
Molparia, B., Nichani, E., and Torkamani, A. (2017). Assessment of Circulating Copy Number Variant Detection for Cancer Screening. PLoS One 12 (7), e0180647. doi:10.1371/journal.pone.0180647
Nakabayashi, M., Kawashima, A., Yasuhara, R., Hayakawa, Y., Miyamoto, S., Iizuka, C., et al. (2018). Massively Parallel Sequencing of Cell-free DNA in Plasma for Detecting Gynaecological Tumour-Associated Copy Number Alteration. Sci. Rep. 8 (1), 11205. doi:10.1038/s41598-018-29381-y
Namasu, C. Y., Katzerke, C., Bräuer-Hartmann, D., Wurm, A. A., Gerloff, D., Hartmann, J.-U., et al. (2017). ABR, a Novel Inducer of Transcription Factor C/EBPα, Contributes to Myeloid Differentiation and Is a Favorable Prognostic Factor in Acute Myeloid Leukemia. Oncotarget 8 (61), 103626–103639. doi:10.18632/oncotarget.22093
Ning, J., Wang, F., Zhu, K., Li, B., Shu, Q., and Liu, W., Characterizing the Copy Number Variation of Non-Coding RNAs Reveals Potential Therapeutic Targets and Prognostic Markers of LUSC. Front. Genet. 2021. 12. 779155. doi:10.3389/fgene.2021.779155
Noguchi, T., Sakai, K., Iwahashi, N., Matsuda, K., Matsukawa, H., Yahata, T., et al. (2020). Changes in the Gene Mutation Profiles of Circulating Tumor DNA Detected Using CAPP-Seq in Neoadjuvant Chemotherapy-Treated Advanced Ovarian Cancer. Oncol. Lett. 19 (4), 2713–2720. doi:10.3892/ol.2020.11356
Page, K., Guttery, D. S., Fernandez-Garcia, D., Hills, A., Hastings, R. K., Luo, J., et al. (2017). Next Generation Sequencing of Circulating Cell-Free DNA for Evaluating Mutations and Gene Amplification in Metastatic Breast Cancer. Clin. Chem. 63 (2), 532–541. doi:10.1373/clinchem.2016.261834
Pan, X., Hu, X., Zhang, Y.-H., Chen, L., Zhu, L., Wan, S., et al. (2019). Identification of the Copy Number Variant Biomarkers for Breast Cancer Subtypes. Mol. Genet. Genomics 294 (1), 95–110. doi:10.1007/s00438-018-1488-4
Pelissier, A., Bonneau, C., Chéreau, E., de La Motte Rouge, T., Fourchotte, V., Daraï, E., et al. (2014). CA125 Kinetic Parameters Predict Optimal Cytoreduction in Patients with Advanced Epithelial Ovarian Cancer Treated with Neoadjuvant Chemotherapy. Gynecol. Oncol. 135 (3), 542–546. doi:10.1016/j.ygyno.2014.09.005
Pool-Zobel, B. L., Selvaraju, V., Sauer, J., Kautenburger, T., Kiefer, J., Richter, K. K., et al. (2005). Butyrate May Enhance Toxicological Defence in Primary, Adenoma and Tumor Human Colon Cells by Favourably Modulating Expression of Glutathione S -transferases Genes, an Approach in Nutrigenomics. Carcinogenesis 26 (6), 1064–1076. doi:10.1093/carcin/bgi059
Powell, C. D., Paullin, T. R., Aoisa, C., Menzie, C. J., Ubaldini, A., and Westerheide, S. D. (2016). The Heat Shock Transcription Factor HSF1 Induces Ovarian Cancer Epithelial-Mesenchymal Transition in a 3D Spheroid Growth Model. PLoS One 11 (12), e0168389. doi:10.1371/journal.pone.0168389
Qidwai, T. (2020). Exploration of Copy Number Variation in Genes Related to Anti-malarial Drug Resistance in Plasmodium Falciparum. Gene 736, 144414. doi:10.1016/j.gene.2020.144414
Rahimi, M., Behjati, F., Khorram Khorshid, H. R., Karimlou, M., and Keyhani, E. (2020). The Relationship between KIT Copy Number Variation, Protein Expression, and Angiogenesis in Sporadic Breast Cancer. Rep. Biochem. Mol. Biol. 9 (1), 40–49. doi:10.29252/rbmb.9.1.40
Raman, L., Dheedene, A., De Smet, M., Van Dorpe, J., and Menten, B. (2018). WisecondorX: Improved Copy Number Detection for Routine Shallow Whole-Genome Sequencing. Nucleic Acids Res. 47 (4), 1605–1614. doi:10.1093/nar/gky1263
Raman, L., Dheedene, A., De Smet, M., Van Dorpe, J., and Menten, B. (2019). WisecondorX: Improved Copy Number Detection for Routine Shallow Whole-Genome Sequencing. Nucleic Acids Res. 47 (4), 1605–1614. doi:10.1093/nar/gky1263
Saha, S., Araf, Y., and Promon, S. K. (2022). Circulating Tumor DNA in Cancer Diagnosis, Monitoring, and Prognosis. J. Egypt Natl. Canc Inst. 34 (1), 8. doi:10.1186/s43046-022-00109-4
Sato, S., and Itamochi, H. (2014). Neoadjuvant Chemotherapy in Advanced Ovarian Cancer: Latest Results and Place in Therapy. Ther. Adv. Med. Oncol. 6 (6), 293–304. doi:10.1177/1758834014544891
Shao, X., Lv, N., Liao, J., Long, J., Xue, R., Ai, N., et al. (2019). Copy Number Variation Is Highly Correlated with Differential Gene Expression: a Pan-Cancer Study. BMC Med. Genet. 20 (1), 175. doi:10.1186/s12881-019-0909-5
Sharbatoghli, M., Vafaei, S., Aboulkheyr Es, H., Asadi-Lari, M., Totonchi, M., and Madjd, Z. (2020). Prediction of the Treatment Response in Ovarian Cancer: a ctDNA Approach. J. Ovarian Res. 13 (1), 124. doi:10.1186/s13048-020-00729-1
Sidiropoulos, K., Viteri, G., Sevilla, C., Jupe, S., Webber, M., Orlic-Milacic, M., et al. (2017). Reactome Enhanced Pathway Visualization. Bioinformatics 33 (21), 3461–3467. doi:10.1093/bioinformatics/btx441
Slenter, D. N., Kutmon, M., Hanspers, K., Riutta, A., Windsor, J., Nunes, N., et al. (2018). WikiPathways: A Multifaceted Pathway Database Bridging Metabolomics to Other Omics Research. Nucleic Acids Res. 46 (D1), D661–d667. doi:10.1093/nar/gkx1064
Tabarés-Seisdedos, R., and Rubenstein, J. L. R. (2009). Chromosome 8p as a Potential Hub for Developmental Neuropsychiatric Disorders: Implications for Schizophrenia, Autism and Cancer. Mol. Psychiatry 14 (6), 563–589. doi:10.1038/mp.2009.2
Tan, K. L., and Board, P. G. (1996). Purification and Characterization of a Recombinant Human Theta-Class Glutathione Transferase (GSTT2-2). Biochem. J. 315 (Pt 3), 727–732. doi:10.1042/bj3150727
Tang, Z., Kang, B., Li, C., Chen, T., and Zhang, Z. (2019). GEPIA2: An Enhanced Web Server for Large-Scale Expression Profiling and Interactive Analysis. Nucleic Acids Res. 47 (W1), W556–W560. doi:10.1093/nar/gkz430
Vanderstichele, A., Busschaert, P., Smeets, D., Landolfo, C., Van Nieuwenhuysen, E., Leunen, K., et al. (2017). Chromosomal Instability in Cell-Free DNA as a Highly Specific Biomarker for Detection of Ovarian Cancer in Women with Adnexal Masses. Clin. Cancer Res. 23 (9), 2223–2231. doi:10.1158/1078-0432.ccr-16-1078
Vihervaara, A., and Sistonen, L. (2014). HSF1 at a Glance. J. Cell Sci. 127 (Pt 2), 261–266. doi:10.1242/jcs.132605
Vives-Usano, M., García Pelaez, B., Román Lladó, R., Garzón Ibañez, M., Aldeguer, E., Rodriguez, S., et al. (2021). Analysis of Copy Number Variations in Solid Tumors Using a Next Generation Sequencing Custom Panel. J. Mol. Pathol. 2 (2), 123–134. doi:10.3390/jmp2020013
Vydra, N., Toma, A., and Widlak, W. (2014). Pleiotropic Role of HSF1 in Neoplastic Transformation. Curr. Cancer Drug Targets 14 (2), 144–155. doi:10.2174/1568009614666140122155942
Walker, L. C., Pearson, J. F., Wiggins, G. A. R., Giles, G. G., Hopper, J. L., and Southey, M. C. (2017). Increased Genomic Burden of Germline Copy Number Variants Is Associated with Early Onset Breast Cancer: Australian Breast Cancer Family Registry. Breast Cancer Res. 19 (1), 30. doi:10.1186/s13058-017-0825-6
Wang, G., Cao, P., Fan, Y., and Tan, K. (2020). Emerging Roles of HSF1 in Cancer: Cellular and Molecular Episodes. Biochimica Biophysica Acta (BBA) - Rev. Cancer 1874 (1), 188390. doi:10.1016/j.bbcan.2020.188390
Willert, J. R., Daneshvar, L., Sheffield, V. C., and Cogen, P. H. (1995). Deletion of Chromosome Arm 17p DNA Sequences in Pediatric High-Grade and Juvenile Pilocytic Astrocytomas. Genes Chromosom. Cancer 12 (3), 165–172. doi:10.1002/gcc.2870120303
Willyard, C. (2015). Copy Number Variations' Effect on Drug Response Still Overlooked. Nat. Med. 21 (3), 206. doi:10.1038/nm0315-206
Woods, D., and Turchi, J. J. (2013). Chemotherapy Induced DNA Damage Response. Cancer Biol. Ther. 14 (5), 379–389. doi:10.4161/cbt.23761
Yu, Y., Cao, J., Wu, W., Zhu, Q., Tang, Y., Zhu, C., et al. (2019). Genome-wide Copy Number Variation Analysis Identified ANO1 as a Novel Oncogene and Prognostic Biomarker in Esophageal Squamous Cell Cancer. Carcinogenesis 40 (10), 1198–1208. doi:10.1093/carcin/bgz077
Yun, H. S., Baek, J.-H., Yim, J.-H., Um, H.-D., Park, J. K., Song, J.-Y., et al. (2016). Radiotherapy Diagnostic Biomarkers in Radioresistant Human H460 Lung Cancer Stem-like Cells. Cancer Biol. Ther. 17 (2), 208–218. doi:10.1080/15384047.2016.1139232
Zeng, J., Yin, J., Song, X., Jin, Y., Li, Y., and Pan, L. (2016). Reduction of CA125 Levels During Neoadjuvant Chemotherapy Can Predict Cytoreduction to No Visible Residual Disease in Patients with Advanced Epithelial Ovarian Cancer, Primary Carcinoma of Fallopian Tube and Peritoneal Carcinoma. J. Cancer 7 (15), 2327–2332. doi:10.7150/jca.16761
Zhang, C. Q., Williams, H., Prince, T. L., and Ho, E. S. (2017). Overexpressed HSF1 Cancer Signature Genes Cluster in Human Chromosome 8q. Hum. Genomics 11 (1), 35. doi:10.1186/s40246-017-0131-5
Keywords: NIPT, ctDNA, CNVs, ovarian cancer, copy number variations
Citation: Sharbatoghli M, Fattahi F, Aboulkheyr Es H, Akbari A, Akhavan S, Ebrahimi M, Asadi-Lari M, Totonchi M and Madjd Z (2022) Copy Number Variation of Circulating Tumor DNA (ctDNA) Detected Using NIPT in Neoadjuvant Chemotherapy-Treated Ovarian Cancer Patients. Front. Genet. 13:938985. doi: 10.3389/fgene.2022.938985
Received: 08 May 2022; Accepted: 15 June 2022;
Published: 22 July 2022.
Edited by:
Ehsan Nazemalhosseini-Mojarad, Shahid Beheshti University of Medical Sciences, IranReviewed by:
Nayeralsadat Fatemi, Shahid Beheshti University of Medical Sciences, IranLongwei Qiao, Xuzhou Medical University, China
Copyright © 2022 Sharbatoghli, Fattahi, Aboulkheyr Es, Akbari, Akhavan, Ebrahimi, Asadi-Lari, Totonchi and Madjd. This is an open-access article distributed under the terms of the Creative Commons Attribution License (CC BY). The use, distribution or reproduction in other forums is permitted, provided the original author(s) and the copyright owner(s) are credited and that the original publication in this journal is cited, in accordance with accepted academic practice. No use, distribution or reproduction is permitted which does not comply with these terms.
*Correspondence: Zahra Madjd, bWFqZGphYmFyaS56QGl1bXMuYWMuaXI=; Mehdi Totonchi, bS50b3RvbmNoaUByb3lhbmluc3RpdHV0ZS5vcmc=
†These authors have contributed equally to this work and share first authorship