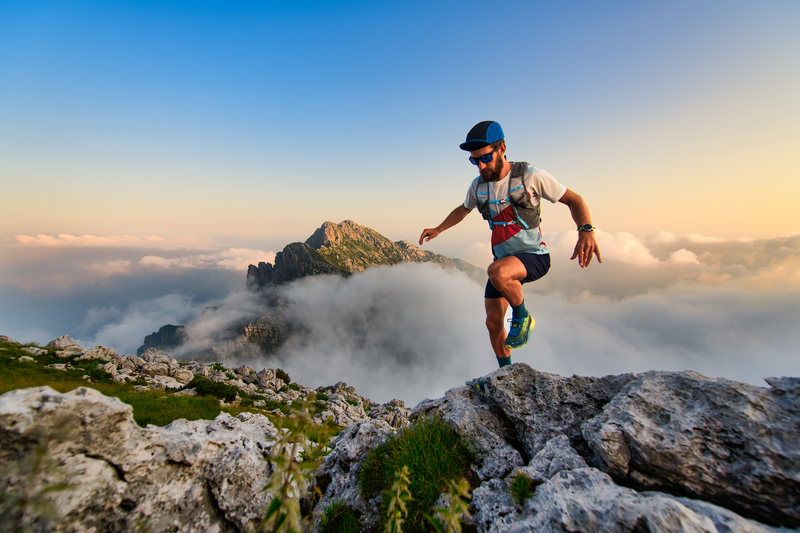
94% of researchers rate our articles as excellent or good
Learn more about the work of our research integrity team to safeguard the quality of each article we publish.
Find out more
ORIGINAL RESEARCH article
Front. Genet. , 02 June 2022
Sec. Genomic Assay Technology
Volume 13 - 2022 | https://doi.org/10.3389/fgene.2022.914384
Introduction: Ulcerative colitis (UC) is a chronic inflammatory disease of the intestine whose cause and underlying mechanisms are not fully understood. The aim of this study was to use bioinformatics analysis to identify differentially expressed genes (DEGs) with diagnostic and therapeutic potential in UC.
Materials and methods: Three UC datasets (GSE179285, GSE75214, GSE48958) were downloaded from the Gene Expression Omnibus (GEO) database. DEGs between normal and UC tissues were identified using the GEO2R online tool. The Gene Ontology (GO) and Kyoto Encyclopedia of Genes and Genomes (KEGG) pathway enrichment analyses of the DEGs were performed using Metascape. Protein-protein interaction network (PPI) analysis and visualization using STRING and Cytoscape. Finally, the miRNA gene regulatory network was constructed by Cytoscape to predict potential microRNAs (miRNAs) associated with DEGs.
Results: A total of 446 DEGs were identified, consisting of 309 upregulated genes and 137 downregulated genes. The enriched functions and pathways of the DEGs include extracellular matrix, regulation of cell adhesion, inflammatory response, response to cytokine, monocarboxylic acid metabolic process, response to toxic substance. The analysis of KEGG pathway indicates that the DEGs were significantly enriched in Complement and coagulation cascades, Amoebiasis, TNF signaling pathway, bile secretion, and Mineral absorption. Combining the results of the PPI network and CytoHubba, 9 hub genes including CXCL8, ICAM1, CXCR4, CD44, IL1B, MMP9, SPP1, TIMP1, and HIF1A were selected. Based on the DEG-miRNAs network construction, 7 miRNAs including miR-335-5p, mir-204-5p, miR-93-5p, miR106a-5p, miR-21-5p, miR-146a-5p, and miR-155-5p were identified as potential critical miRNAs.
Conclusion: In summary, we identified DEGs that may be involved in the progression or occurrence of UC. A total of 446 DEGs,9 hub genes and 7 miRNAs were identified, which may be considered as biomarkers of UC. Further studies, however, are needed to elucidate the biological functions of these genes in UC.
UC is a group of chronic inflammatory diseases of the intestine whose etiology is not yet fully understood. UC begins in the rectum and usually extends proximally in a continuous manner through part or the entire colon (Danese and Fiocchi, 2011; Ordás et al., 2012). The typical clinical manifestations of UC are bloody diarrhea, abdominal pain, fecal urgency, and tenesmus. In some patients, extraintestinal manifestations may precede the onset of gastrointestinal symptoms (Feuerstein et al., 2019). Globally, UC is more common than Crohn’s disease (Ng et al., 2017), and a variety of drugs are available for its treatment (Ungaro et al., 2017). However, up to 15% of cases do not respond to medication or have chronic colitis secondary to dysplasia and require surgical treatment (Dignass et al., 2012). For this reason, understanding the precise molecular mechanisms underlying UC is vital for developing therapeutic approaches.
Bioinformatics is an emerging discipline that is considered as one of the promising and critical approaches for early diagnosis, effective treatment and prediction of clinical challenges in the prognosis of cancer patients (Wang and Liotta, 2011). This new strategy has been widely used in the study of various cancers (Li et al., 2017; Yan et al., 2018; Tsai and Gamblin, 2019), and has been instrumental in the identification of novel biomarkers in several non-tumor diseases (Chen et al., 2018; Cakmak and Demir, 2020; Xie et al., 2020).
Bioinformatics and microrray technology are widely used in screening for genetic alterations at the genomic level, and to search DEGs and functional pathways participating in the development and progression of inflammatory bowel disease (IBD). However, independent microarray analysis makes it difficult to get reliable results because of its high false-positivity rate. Thus, in the present study, to identify DEGs between normal and UC colonic mucosa tissues, 3 mRNA microarray datasets were downloaded from Gene Expression Omnibus (GEO). Gene Ontology (GO), Kyoto Encyclopedia of Genes and Genomes (KEGG) pathway enrichment analysis and protein-protein interaction (PPI) network analyses were then conducted to identify molecular mechanisms underlying the development and progression of UC. Finally, the miRNA gene regulatory network was constructed using Cytoscape to predict potential microRNAs (miRNAs) associated with DEGs. In conclusion, a total of 446 DEGs, 9 hub genes and 7 potential miRNAs were identified as candidate biomarkers for UC.
GEO (http://www.ncbi.nlm.nih.gov/geo) (Edgar et al., 2002) is a public functional genomics data repository of high throughput gene expression data, chips and microarrays. The GSE75214 (Vancamelbeke et al., 2017) and GSE48958 (Van der Goten et al., 2014) datasets generated using the Affymetrix GPL6244 platform (Affymetrix Human Genome 1.0 ST Array), and GSE179285 (Keir et al., 2021) generated on the GPL6480 platform (Agilent-014850 Whole Human Genome Microarray 4 × 44K G4112F) were downloaded from GEO. Annotated information from the platform was used to convert the probes to the corresponding gene symbols. The GSE179285 dataset contained 23 UC inflamed colonic mucosa tissue samples and 23 control samples; the GSE75214 dataset contained 74 biopsies from inflamed colonic mucosa of active UC patients and 11 healthy control samples; and the GSE48958 dataset contained 7 colonic mucosal biopsies from UC patients with active disease and 8 colonic mucosal biopsies from controls.
Identification of DEGs between UC and normal samples was performed using GEO2R (http://www.ncbi.nlm.nih.gov/geo/geo2r). GEO2R is an online interactive tool that allows users to identify DEGs for different experimental conditions by comparing two or more datasets in the GEO series. Adjusted p-values (adj. P) and Benjamini and Hochberg’s false discovery rates were applied to provide a balance between discovering statistically significant genes and limiting false positives. Probe sets without corresponding gene symbols or genes with more than one probe set were deleted or normalized, respectively. Log FC (fold change) >1 and adj. p-value <0.01 were considered statistically significant.
Metascape (https://metascape.org/gp/index.html#/main/step1) (Yingyao Zhou et al., 2019) is an analytical website that integrates functional enrichment, interactomic analysis, genetic annotation, and membership search, utilizing more than 40 individual knowledge bases in a comprehensive portal. KEGG is a database resource for elucidating high-level functions and effects of biological systems (Kanehisa, 2002; Kanehisa et al., 2017). GO is a major bioinformatics initiative for high-quality functional gene annotation based on biological processes (BP), molecular functions (MF) and cellular components (CC) (Pomaznoy et al., 2018). Metascape was used to predict the functions of DEGs, with the screening conditions set as Min overlap = 3 and Min Enrichment = 1.5. p < 0.01 was considered statistically significant.
The PPI network was constructed using the Search Tool for the Retrieval of Interacting Genes (STRING; http://string-db.org) (version 11.5) (Franceschini et al., 2013) online database, and the parameters were set as follows: meaning of network edges: confidence level; minimum required interaction score: medium confidence (0.400); active interaction sources: Select Textmining, Experiments, Databases, Co-expression, Neighborhood, Gene Fusion and Co-occurrence. Identification of functional interactions between proteins may provide insights into the mechanisms underlying the development of diseases. Cytoscape (version 3.8.2) is an open-source bioinformatics software platform for visualizing molecular interaction networks (Smoot et al., 2011). Molecular Complex Detection (MCODE) (version 2.0) is a plug-in in Cytoscape used to identify densely connected regions by clustering a given network based on the topology (Bandettini et al., 2012). Using Cytoscape to map the PPI network, the MCODE was used to identify the most significant modules in the PPI network. The following selection criteria were used: MCODE scores >5, degree cut-off = 2, node score cut-off = 0.2, Max depth = 100 and k-score = 2.
The top 9 genes were obtained using MCC algorithm with Cytoscape’s plug-in cytoHubba. Metascape was used to predict the functions of hub genes, with the screening conditions set as Min overlap = 3 and Min Enrichment = 1.5. p < 0.01 was considered statistically significant.
The top 9 hub genes were mapped to the respective miRNAs with NetworkAnalyst 3.0 (Guangyan Zhou et al., 2019) (https://www.networkanalyst.ca/), an online platform for visualization that helps to identify miRNA-gene interactions in Gene Regulatory Networks. For each hub gene, miRNAs were identified as having a degree cutoff = 1.0. Lastly, a mapping of these hub genes and miRNAs was performed by Cytoscape 3.8.2.
Three datasets (GSE179285, GSE75214 and GSE48958) containing gene expression profiles of healthy and UC inflamed/active colonic mucosa tissue samples were retrieved from the GEO database. Details for the three datasets are presented in Table 1. After standardization of the microarray results, DEGs (894 in GSE179285, 1236 in GSE75214 and 1244 in GSE48958) were identified. The DEGs in the GSE179285, GSE75214 and GSE48958 datasets included 634 upregulated and 260 downregulated genes, 790 upregulated and 446 downregulated genes and 839 upregulated and 405 downregulated genes, respectively. All DEGs were identified by comparing gene expression profiles between healthy controls and UC inflamed/active samples. The gene expression profiles of DEGs in the three datasets containing 2 sets of sample data are shown in Figure 1.
FIGURE 1. Volcano plots indicating differentially expressed genes (DEGs) among the control and UC groups. (A–C) DEGs of the GSE179285, GSE75214 and GSE48958 datasets are shown, separately. red data points represent up-regulated genes and blue ones represent down-regulated genes. Genes without any significant differences are in black.
These genes were screened further and Venn diagrams were mapped to represent these genes. As shown in Figure 2, 446 DEGs were found to be significantly differentially expression in 3 groups, of which 309 genes were upregulated and 137 genes were downregulated (Table 2).
FIGURE 2. Venn diagrams showing the differentially expressed genes (DEGs) that overlapped among the 3 datasets retrieved from Gene Expression Omnibus (GEO). (A,B) indicate the overlap of up-regulated and down-regulated genes in the GSE179285, GSE75214, and GSE48958 datasets, separately.
To predict the biological functions of the DEGs, we performed functional enrichment analysis of up-regulated and downregulated genes. Results of GO analysis showed that the up-regulated genes were mainly enriched in extracellular matrix, regulation of cell adhesion, inflammatory response and response to cytokines (Figure 3A, Supplementary Table S1), while the down-regulated genes were significantly enriched in monocarboxylic acid metabolic process and response to toxic substances (Figure 3B, Supplementary Table S2). KEGG pathway analysis indicated that the up-regulated genes were significantly enriched in Complement and coagulation cascades, Amoebiasis and TNF signaling pathway (Figure 3C, Supplementary Table S3), while the down-regulated genes were mainly enriched in bile secretion and Mineral absorption (Figure 3D, Supplementary Table S4).
FIGURE 3. Analysis of functional enrichment in DEGs. The bar graphs show the top 20 results of the up-regulated gene (A,C) and down-regulated gene (B,D) enrichment analysis. p-value is displayed in color.
PPI analysis of the DEGs was based on the STRING database and the results were visualized using Cytoscape (Figure 4A). Using MCODE, a plug-in in Cytoscape, we identified the most densely connected regions (40 nodes, 275 edges) in the PPI network (Figure 4B). The top 9 genes, including CXCL8, ICAM1, CXCR4, CD44, IL1B, MMP9, SPP1, TIMP1, and HIF1A, were obtained using MCC algorithm with Cytoscape’s plug-in cytoHubba (Figure 4C). The results showed that CXCL8 (Chemokine (C-X-C motif) Ligand 8, score 3.24E+11), ICAM1 (Intercellular Adhesion Molecule 1, score 3.24E+11), CXCR4 (Chemokine (C-X-C motif) Receptor 4, score 3.24E+11), CD44 (CD44 Molecule, score 3.24E+11) and IL1B (Interleukin 1 Beta, score3.24E+11) were the most significant genes, followed by MMP9 (Matrix Metallopeptidase 9, score 3.23E+11), SPP1 (Secreted Phosphoprotein 1, score 3.20E+11), TIMP1 (TIMP Metallopeptidase Inhibitor 1, score 3.14E+11) and HIF1A (Hypoxia Inducible Factor 1 Subunit Alpha, score 3.13E+11).
FIGURE 4. PPI networks of 309 up-regulated genes and 137 down-regulated genes by Cytoscape. The network consists of 446 nodes and 2163 edges. Two edges between nodes represent the interactions between genes. Each gene corresponding to the node is sized and colored according to the degree of interaction. The color grade indicates the change in the degree of each gene from high (orange-red) to low (faint yellow). The nearer the red node, the higher the connection between the Two nodes (A). The densest connected region in the PPI network (40 nodes, 275 edges) was identified using MCODE (B). Using the MCC algorithm in cytoHubba, 9 hub genes were identified in the densest connected regions. The scores are shown in red color. A darker color means a higher score (C).
The hub gene symbols, abbreviations and functions are shown in Table 3. Functional enrichment analysis showed that the 9 hub genes were mainly concentrated in biological processes (BP), namely inflammatory response, response to cytokines, negative regulation of apoptotic signaling pathway, leukocyte migration and positive regulation of cell migration, leukocyte cell-cell adhesion, regulation of neurogenesis, negative regulation of intrinsic apoptotic signaling pathway and morphogenesis of epithelium, as well as one Kyoto Encyclopedia of Genes and Genomes (KEGG) that is leukocyte transendothelial migration (Figures 5A–C; Table 4).
FIGURE 5. Analysis of functional enrichment for hub genes. (A) Bar graph of GO analyses for hub genes. P-value is shown in color. Enrichment term network of hub genes; colors indicate the same cluster ID (B), and P-value (C).
MiRNAs play a variety of roles in the regulation of gene expression. Based on the Network Analyst database, Cytoscape was used to construct miRNAs-hub genes regulatory networks to identify miRNAs aimed at hub genes. Nine hub genes and their correspondent regulatory miRNAs molecules are shown in the Figure 6 and Supplementary Table S5. One miRNA (hsa-mir-204-5p) had 5 target genes (CD44, IL1B, MMP9, CXCR4 and CXCL8). Among the 9 hub genes, CXCL8, CXCR4, SPP1, and ICAM1 were common targets of 2 miRNAs (has-miR-335-5p and has-miR-146a-5p), while CXCL8, HIF1A, SPP1 and ICAM1 were common targets of 2 miRNAs (has-miR-155-5p and has-miR-93-5p).
FIGURE 6. Top 9 hub genes in the integrated miRNA-DEGs network. The pink octagonal shape indicates the 9 hub genes. The gray circles indicate miRNAs with low connective properties to the hub genes. Dark green-light green-light blue diamonds indicate miRNAs with high connective properties to the hub genes.
Studies of genome-wide association have transformed the complex field of polygenic diseases and helped find several genes responsible for UC, resulting in new understanding of the pathogenesis of the disease.
The underlying causes of UC are ambiguous and complex, and its pathogenesis is multifactorial, with environmental and genetic factors interacting to produce a range of clinical manifestations. In this study, 446 DEGs were identified, consisting of 309 upregulated genes and 137 downregulated genes. The results of GO functional classification indicated that the DEGs were mainly enriched in extracellular matrix, regulation of cell adhesion, inflammatory response, response to cytokines, monocarboxylic acid metabolic process and response to toxic substances. KEGG pathway analysis indicated that the up-regulated DEGs were significantly enriched in Complement and coagulation cascades, Amoebiasis and TNF signaling pathway. In the PPI network of DEGs, 9 (CXCL8, ICAM1, CXCR4, CD44, IL1B, MMP9, SPP1, TIMP1, and HIF1A) out of 446 genes had high degree of interation. All of the 9 hub genes were up-regulated in patients with UC. GO analysis showed that these 9 genes were highly enriched in inflammatory response, response to cytokines, negative regulation of apoptotic signaling pathway, leukocyte migration and positive regulation of cell migration, leukocyte cell-cell adhesion, regulation of neurogenesis, negative regulation of intrinsic apoptotic signaling pathway and morphogenesis of epithelium, as well as KEGG that is leukocyte transendothelial migration. Triggers of IBD include chronic inflammatory responses triggered by specific pathogens or viral infections or mucosal barrier defects (Hanauer, 2006). Leukocyte migration is involved in the progression of IBD, so blocking leukocyte migration to the intestine is the major strategy used to control the disease and alleviate symptoms (Veny et al., 2020). It has been shown that the TNF signaling pathway is involved in the progression of UC(Danese et al., 2020). The results of these enriched GO terms and the KEGG pathway suggest that the DEGs or hub genes identified in this study may be involved in the progression of UC through the above-mentioned ways.CXCL8 (Chemokine (C-X-C motif) Ligand 8, IL-8), a classic ELR+CXC chemokine (Baird et al., 2011; Russo et al., 2014; Ha et al., 2017), is one of the first and most extensively studied chemokines that can be released by a wide range of cell types, including neutrophils (Bie et al., 2019), monocytes (Schroder et al., 1988), and macrophages (Wanninger et al., 2009). One of the most important pro-inflammatory factors is CXCL8, which acts as a key factor in numerous inflammatory diseases, including UC. Signaling pathways such as PI3K/Akt, MAPKs, and NF-*B are involved in the pathogenesis of UC through the CXCL8-CXCR1/2 axis (Zhu et al., 2021). In our study, CXCL8 was upregulated in patients with UC, suggesting a potential role as a biological target to predict and guide UC treatment in the future.
ICAM1 (Intercellular Adhesion Molecule 1), a member of the immunoglobulin superfamily, is a highly glycosylated transmembrane protein involved in the migration and transport of leukocytes to sites of inflammation (Dustin et al., 1986). Leukocyte trafficking has been identified as a specific target for the treatment of IBD and is a multi-step procedure involving integrins on leukocytes and their immunoglobulin superfamily counterparts as ligands, such as ICAM-1 (Rivera-Nieves et al., 2008; Mosli et al., 2014). Alicaforsen, which treats pouchitis and left-sided UC by inhibiting ICAM-1 production, has been granted orphan drug designation and is available as an unlicensed drug under international regulations (Greuter and Rogler, 2017).
CXCR4 (Chemokine (C-X-C motif) Receptor 4) is a type of amino acid rhodopsin-like G protein-coupled receptor (GPCR) (Wu et al., 2010). The number of immature plasma cells specifically overexpressing CXCR4 is increased in the peripheral blood of UC patients (Hosomi et al., 2011). Mina T Kitazume et al. demonstrated that unique IgG plasma cells exacerbate mucosal inflammation through infiltration of CXCR4 into the inflamed mucosa and activation of “pathogenic” intestinal CD14 macrophages via IgG-ICFcγR signaling, thereby having a critical impact on UC pathogenesis (Uo et al., 2013). Lin et al. suggested that CXCR4 may affect the function of micro vascular endothelial cells (MVECs) during UC and further inhibits the protein C system (PCS) through the JNK MAPK-c-Jun pathway and serves as a potential therapeutic target for UC (Lin et al., 2017). In our study and previous studies, CXCR4 was upregulated in UC, indicating that it was a key target for the treatment of UC.
CD44 (CD44 molecule) is a non-kinase transmembrane glycoprotein which is overexpressed in multiple cell types, as well as in cancer stem cells, and with a known role in the development and progression of cancer (Naor et al., 2002). When CD44 combines with and triggers its primary ligand, hyaluronic acid (HA), it results in the activation of cell signaling pathways, induces cell proliferation, enhances cell survival, mediates cytoskeletal changes, and strengthens cell movement (Ponta et al., 2003). A recent study found that multi-bioresponsive silk fibroin-based nanoparticles targeted against CD44 with on-demand cytoplasmic drug release capacity could alleviate UC (Gou et al., 2019).
IL1B (Interleukin 1 beta, IL-1β) is a critical mediator in the inflammatory response and is not merely crucial for host response and defense against pathogens, but also aggravates damage during chronic disease and acute tissue damage (Lopez-Castejon and Brough, 2011). Earlier studies have shown higher concentrations of IL-1β in the intestinal mucosa of patients with UC (Nakase et al., 2022). In our study, the expression of IL1B was low in normal human intestinal tissues, suggesting that IL1B may be a biological target in UC.
MMP9 (Matrix Metallopeptidase 9) is a member of metalloproteinases (MMPs) that plays an important role not only in tumor invasion and migration (Herszenyi et al., 2007; Herszenyi et al., 2008), but also in inflammation and remodeling in UC (Stallmach et al., 2000; von Lampe et al., 2000). In our study, MMP9 was up-regulated UC tissues compared with normal human colon and rectum tissues, suggesting that MMP9 may be a potential marker of disease activity in patients with UC.
SPP1 (Secreted Phosphoprotein 1/Osteopontin/OPN) is a chemokine-like matrix phosphoglycoprotein that not only affects cell proliferation and invasion, but is also involved in the invasive and metastatic processes of many cancers, and is considered as a prognostic and diagnostic marker for several cancers (Shevde and Samant, 2014). Giannos P et al. concluded from the bioinformatic analysis that SPP1 may be a potential biomarker of genes and a predictor of IFX treatment resistance in the development of UC and CRC (Giannos et al., 2022). Chen F et al. suggested that SPP1/OPN plays an important role in the immune response and is also involved in the mucosal protection mechanism of IBD (Chen et al., 2013). The relationship between SPP1 and UC remains poorly understood and needs to be further explored.
TIMP1 (namely Tissue inhibitor of metalloproteinase 1), is one of the four members of the glycoprotein group (TIMP1-4), and translocation of extracellular matrix is its primary function. TIMP1 is involved in a variety of pathological processes, including wound healing and cancer metastasis (Gardner and Ghorpade, 2003). Huang et al. suggested that TIMP1 is consistently upregulated during the pathology of UC-associated CRC (ucaCRC) and could be a potential biomarker of prognostic deterioration in CRC (Huang et al., 2019). Moreover, plasma TIMP-1 levels reflect to some extent the colonic mucosal expression of TIMP-1 in UC patients and have the prospect of becoming a biomarker for clinical diagnosis of UC as well as for predicting its severity and guiding further treatment (Wang et al., 2009).
Hypoxia-inducible factor (HIF) mediates the major transcriptional response to hypoxia and is a key regulator under hypoxic stress (Semenza, 2003). Three distinct HIF isoforms exist in mammals, of which HIF1A (Hypoxia Inducible Factor 1 Subunit Alpha) is commonly expressed (Wang and Semenza, 1993). Vitamin D/VDR signaling suppresses UC by inhibiting the activation of HIF-1α in colonic epithelial cells (Xue et al., 2021). Qi Lv et al. have shown that the suppression of HIF-1α-mediated glycolysis by costunolide acts specifically in the differentiation of Th17 cells and consequent UC relief, and it also provides a novel prospective for the immunometabolic treatment of colitis (Lv et al., 2021).
A major role of microRNAs (miRNAs) is to regulate the expression of most human genes; they play a crucial role in the pathogenesis of autoimmune diseases, such as UC (Zhou et al., 2021). MiR-93 may be involved in the pathogenic process of IBD through its involvement in the regulation of autophagic activity (Chen et al., 2014). Omidbakhsh A et al. suggested that the difference in miR106a expression in the peripheral blood of UC and Crohn’s disease (CD) patients in active versus inactive phases suggests that miR106a may act as a potential biomarker for diagnosing and monitoring of disease activity (Omidbakhsh et al., 2018). LuX et al. reported that inhibition of miR-21-5p during UC rats reduced inflammation and apoptosis levels in RAW264.7 cells, suggesting a potential therapeutic role for miR-21-5p in human UC (Lu et al., 2020). Jabandziev et al. analyzed miRNA expression in the colonic tissue of 60 pediatric UC patients and identified miR-146a-5p as a diagnostic factor for primary sclerosing cholangitis in UC patients (Jabandziev et al., 2021). MiRNAs are involved in tumor formation in UC, and patients with UC have an increased risk of developing colitis-associated cancer (CAC) over 8 years (Chen et al., 2016). It has been reported that miR-155-5p promotes the metastasis of colon cancer cells by targeting the AU-rich element (ARE) in the 3′-UTR region of HuR mRNA to upregulate human antigen R (HuR); colon cancer cells could be inhibited from migrating when miR-155-5p is blocked from binding to the ARE in HuR (Al-Haidari et al., 2018). Our current findings suggest that several miRNAs, including miR-335-5p, mir-204-5p, miR-93-5p, miR106a-5p, miR-21-5p, miR-146a-5p, and miR-155-5p, may play key roles in UC. In addition, studies about genes and miRNAs in UC remains to be limited.
There is no doubt that the gene-miRNA regulatory network plays an important role in UC pathophysiology. This increases the understanding of UC and provides targeted therapeutic strategies and predictions for UC. One limitation of the study was that the microarray expression profiles were analyzed using bioinformatics analysis without being validated with basic experiments. In addition, we did not explore the detailed mechanisms of how the hub genes and miRNA regulate UC. Therefore, there is need to validate our findings using more clinical samples and further studies.
In summary, we identified DEGs that can be potentially participating in the progression or occurrence of UC. A total of 446 DEGs,9 hub genes and 7 miRNAs were identified, which may be considered as biomarkers of UC. However, still further studies are necessary to clarify the biological functions of such genes in UC.
Publicly available datasets were analyzed in this study. The names of the repository/repositories and accession number(s) can be found in the article/Supplementary Material.
WH participated in the design of the study, carried out the study, performed the statistical analysis, and drafted the manuscript. TF helped to carried out the study. XC reviewed and edited the manuscript. All authors read and approved the final manuscript.
The authors declare that the research was conducted in the absence of any commercial or financial relationships that could be construed as a potential conflict of interest.
All claims expressed in this article are solely those of the authors and do not necessarily represent those of their affiliated organizations, or those of the publisher, the editors and the reviewers. Any product that may be evaluated in this article, or claim that may be made by its manufacturer, is not guaranteed or endorsed by the publisher.
Thanks to all the people in our laboratory for their help.
The Supplementary Material for this article can be found online at: https://www.frontiersin.org/articles/10.3389/fgene.2022.914384/full#supplementary-material
Al-Haidari, A., Algaber, A., Madhi, R., Syk, I., and Thorlacius, H. (2018). MiR-155-5p Controls Colon Cancer Cell Migration via Post-Transcriptional Regulation of Human Antigen R (HuR). Cancer Lett. 421, 145–151. doi:10.1016/j.canlet.2018.02.026
Baird, A.-M., Gray, S. G., and O'Byrne, K. J. (2011). Epigenetics Underpinning the Regulation of the CXC (ELR+) Chemokines in Non-small Cell Lung Cancer. PLoS One 6 (1), e14593. doi:10.1371/journal.pone.0014593
Bandettini, W. P., Kellman, P., Mancini, C., Booker, O. J., Vasu, S., Leung, S. W., et al. (2012). MultiContrast Delayed Enhancement (MCODE) Improves Detection of Subendocardial Myocardial Infarction by Late Gadolinium Enhancement Cardiovascular Magnetic Resonance: a Clinical Validation Study. J. Cardiovasc Magn. Reson. 14, 83. doi:10.1186/1532-429X-14-83
Bie, Y., Ge, W., Yang, Z., Cheng, X., Zhao, Z., Li, S., et al. (2019). The Crucial Role of CXCL8 and its Receptors in Colorectal Liver Metastasis. Dis. Markers 2019, 1–12. doi:10.1155/2019/8023460
Çakmak, H. A., and Demir, M. (2020). MicroRNA and Cardiovascular Diseases. Balk. Med. J. 37 (2), 60–71. doi:10.4274/balkanmedj.galenos.2020.2020.1.94
Chen, F., Liu, H., Shen, Q., Yuan, S., Xu, L., Cai, X., et al. (2013). Osteopontin: Participation in Inflammation or Mucosal Protection in Inflammatory Bowel Diseases? Dig. Dis. Sci. 58 (6), 1569–1580. doi:10.1007/s10620-012-2556-y
Chen, L., Su, W., Chen, H., Chen, D.-Q., Wang, M., Guo, Y., et al. (2018). Proteomics for Biomarker Identification and Clinical Application in Kidney Disease. Adv. Clin. Chem. 85, 91–113. doi:10.1016/bs.acc.2018.02.005
Chen, R., Lai, L. A., Brentnall, T. A., and Pan, S. (2016). Biomarkers for Colitis-Associated Colorectal Cancer. World J. Gastroenterol. 22 (35), 7882–7891. doi:10.3748/wjg.v22.i35.7882
Chen, W.-X., Ren, L.-H., and Shi, R.-H. (2014). Implication of miRNAs for Inflammatory Bowel Disease Treatment: Systematic Review. World J. Gastrointest. Pathophysiol. 5 (2), 63–70. doi:10.4291/wjgp.v5.i2.63
Danese, S., and Fiocchi, C. (2011). Ulcerative Colitis. N. Engl. J. Med. 365 (18), 1713–1725. doi:10.1056/NEJMra1102942
Danese, S., Fiorino, G., and Peyrin-Biroulet, L. (2020). Positioning Therapies in Ulcerative Colitis. Clin. Gastroenterol. Hepatol. 18 (6), 1280–1290.e1. doi:10.1016/j.cgh.2020.01.017
Dignass, A., Lindsay, J. O., Sturm, A., Windsor, A., Colombel, J.-F., Allez, M., et al. (2012). Second European Evidence-Based Consensus on the Diagnosis and Management of Ulcerative Colitis Part 2: Current Management. J. Crohn's Colitis 6 (10), 991–1030. doi:10.1016/j.crohns.2012.09.002
Dustin, M. L., Rothlein, R., Bhan, A. K., Dinarello, C. A., and Springer, T. A. (1986). Induction by IL 1 and Interferon-Gamma: Tissue Distribution, Biochemistry, and Function of a Natural Adherence Molecule (ICAM-1). J. Immunol. 137 (1), 245–254.
Edgar, R., Domrachev, M., and Lash, A. E. (2002). Gene Expression Omnibus: NCBI Gene Expression and Hybridization Array Data Repository. Nucleic Acids Res. 30 (1), 207–210. doi:10.1093/nar/30.1.207
Feuerstein, J. D., Moss, A. C., and Farraye, F. A. (2019). Ulcerative Colitis. Mayo Clin. Proc. 94 (7), 1357–1373. doi:10.1016/j.mayocp.2019.01.018
Franceschini, A., Szklarczyk, D., Frankild, S., Kuhn, M., Simonovic, M., Roth, A., et al. (2013). STRING v9.1: Protein-Protein Interaction Networks, with Increased Coverage and Integration. Nucleic Acids Res. 41 (Database issue), D808–D815. doi:10.1093/nar/gks1094
Gardner, J., and Ghorpade, A. (2003). Tissue Inhibitor of Metalloproteinase (TIMP)-1: the TIMPed Balance of Matrix Metalloproteinases in the Central Nervous System. J. Neurosci. Res. 74 (6), 801–806. doi:10.1002/jnr.10835
Giannos, P., Triantafyllidis, K. K., Giannos, G., and Kechagias, K. S. (2022). SPP1 in Infliximab Resistant Ulcerative Colitis and Associated Colorectal Cancer: An Analysis of Differentially Expressed Genes. Eur. J. Gastroenterol. Hepatol. 34 (6), 598–606. doi:10.1097/MEG.0000000000002349
Gou, S., Huang, Y., Wan, Y., Ma, Y., Zhou, X., Tong, X., et al. (2019). Multi-bioresponsive Silk Fibroin-Based Nanoparticles with On-Demand Cytoplasmic Drug Release Capacity for CD44-Targeted Alleviation of Ulcerative Colitis. Biomaterials 212, 39–54. doi:10.1016/j.biomaterials.2019.05.012
Greuter, T., and Rogler, G. (2017). Alicaforsen in the Treatment of Pouchitis. Immunotherapy 9 (14), 1143–1152. doi:10.2217/imt-2017-0085
Ha, H., Debnath, B., and Neamati, N. (2017). Role of the CXCL8-Cxcr1/2 Axis in Cancer and Inflammatory Diseases. Theranostics 7 (6), 1543–1588. doi:10.7150/thno.15625
Hanauer, S. B. (2006). Inflammatory Bowel Disease: Epidemiology, Pathogenesis, and Therapeutic Opportunities. Inflamm. Bowel Dis. 12 (Suppl. 1), S3–S9. doi:10.1097/01.mib.0000195385.19268.68
Herszenyi, L., Hritz, I., Pregun, I., Sipos, F., Juhasz, M., Molnar, B., et al. (2007). Alterations of Glutathione S-Transferase and Matrix Metalloproteinase-9 Expressions Are Early Events in Esophageal Carcinogenesis. World J. Gastroenterol. 13 (5), 676–682. doi:10.3748/wjg.v13.i5.676
Herszényi, L., Sipos, F., Galamb, O., Solymosi, N., Hritz, I., Miheller, P., et al. (2008). Matrix Metalloproteinase-9 Expression in the Normal Mucosa-Adenoma-Dysplasia-Adenocarcinoma Sequence of the Colon. Pathol. Oncol. Res. 14 (1), 31–37. doi:10.1007/s12253-008-9004-5
Hosomi, S., Oshitani, N., Kamata, N., Sogawa, M., Okazaki, H., Tanigawa, T., et al. (2011). Increased Numbers of Immature Plasma Cells in Peripheral Blood Specifically Overexpress Chemokine Receptor CXCR3 and CXCR4 in Patients with Ulcerative Colitis. Clin. Exp. Immunol. 163 (2), 215–224. doi:10.1111/j.1365-2249.2010.04290.x
Huang, R., Wang, K., Gao, L., and Gao, W. (2019). TIMP1 Is A Potential Key Gene Associated with the Pathogenesis and Prognosis of Ulcerative Colitis-Associated Colorectal Cancer. Onco. Targets Ther. 12, 8895–8904. doi:10.2147/OTT.S222608
Jabandziev, P., Kakisaka, T., Bohosova, J., Pinkasova, T., Kunovsky, L., Slaby, O., et al. (2021). MicroRNAs in Colon Tissue of Pediatric Ulcerative Pancolitis Patients Allow Detection and Prognostic Stratification. J. Clin. Med. 10 (6), 1325. doi:10.3390/jcm10061325
Kanehisa, M., Goto, S., Kawashima, S., and Nakaya, A. (2002). The KEGG Databases at GenomeNet. Nucleic Acids Res. 30, 42–46. discussion 101-103, 119-128, 244-152. doi:10.1093/nar/30.1.42
Kanehisa, M., Furumichi, M., Tanabe, M., Sato, Y., and Morishima, K. (2017). KEGG: New Perspectives on Genomes, Pathways, Diseases and Drugs. Nucleic Acids Res. 45 (D1), D353–D361. doi:10.1093/nar/gkw1092
Keir, M. E., Fuh, F., Ichikawa, R., Acres, M., Hackney, J. A., Hulme, G., et al. (2021). Regulation and Role of αE Integrin and Gut Homing Integrins in Migration and Retention of Intestinal Lymphocytes during Inflammatory Bowel Disease. J. Immunol. 207 (9), 2245–2254. doi:10.4049/jimmunol.2100220
Li, L., Lei, Q., Zhang, S., Kong, L., and Qin, B. (2017). Screening and Identification of Key Biomarkers in Hepatocellular Carcinoma: Evidence from Bioinformatic Analysis. Oncol. Rep. 38 (5), 2607–2618. doi:10.3892/or.2017.5946
Lin, X., Wang, H., Li, Y., Yang, J., Yang, R., Wei, D., et al. (2017). Functional Characterization of CXCR4 in Mediating the Expression of Protein C System in Experimental Ulcerative Colitis. Am. J. Transl. Res. 9 (11), 4821–4835.
Lopez-Castejon, G., and Brough, D. (2011). Understanding the Mechanism of IL-1β Secretion. Cytokine & Growth Factor Rev. 22 (4), 189–195. doi:10.1016/j.cytogfr.2011.10.001
Lu, X., Yu, Y., and Tan, S. (2020). The Role of the miR-21-5p-Mediated Inflammatory Pathway in Ulcerative Colitis. Exp. Ther. Med. 19 (2), 981–989. doi:10.3892/etm.2019.8277
Lv, Q., Xing, Y., Dong, D., Hu, Y., Chen, Q., Zhai, L., et al. (2021). Costunolide Ameliorates Colitis via Specific Inhibition of HIF1α/glycolysis-Mediated Th17 Differentiation. Int. Immunopharmacol. 97, 107688. doi:10.1016/j.intimp.2021.107688
Mosli, M. H., Rivera-Nieves, J., and Feagan, B. G. (2014). T-cell Trafficking and Anti-adhesion Strategies in Inflammatory Bowel Disease: Current and Future Prospects. Drugs 74 (3), 297–311. doi:10.1007/s40265-013-0176-2
Nakase, H., Sato, N., Mizuno, N., and Ikawa, Y. (2022). The Influence of Cytokines on the Complex Pathology of Ulcerative Colitis. Autoimmun. Rev. 21 (3), 103017. doi:10.1016/j.autrev.2021.103017
Naor, D., Nedvetzki, S., Golan, I., Melnik, L., and Faitelson, Y. (2002). CD44 in Cancer. Crit. Rev. Clin. Lab. Sci. 39 (6), 527–579. doi:10.1080/10408360290795574
Ng, S. C., Shi, H. Y., Hamidi, N., Underwood, F. E., Tang, W., Benchimol, E. I., et al. (2017). Worldwide Incidence and Prevalence of Inflammatory Bowel Disease in the 21st Century: a Systematic Review of Population-Based Studies. Lancet 390 (10114), 2769–2778. doi:10.1016/S0140-6736(17)32448-0
Omidbakhsh, A., Saeedi, M., Khoshnia, M., Marjani, A., and Hakimi, S. (2018). Micro-RNAs -106a and -362-3p in Peripheral Blood of Inflammatory Bowel Disease Patients. Open Biochem. J. 12, 78–86. doi:10.2174/1874091X01812010078
Ordás, I., Eckmann, L., Talamini, M., Baumgart, D. C., and Sandborn, W. J. (2012). Ulcerative Colitis. Lancet 380 (9853), 1606–1619. doi:10.1016/S0140-6736(12)60150-0
Pomaznoy, M., Ha, B., and Peters, B. (2018). GOnet: a Tool for Interactive Gene Ontology Analysis. BMC Bioinforma. 19 (1), 470. doi:10.1186/s12859-018-2533-3
Ponta, H., Sherman, L., and Herrlich, P. A. (2003). CD44: from Adhesion Molecules to Signalling Regulators. Nat. Rev. Mol. Cell Biol. 4 (1), 33–45. doi:10.1038/nrm1004
Rivera-Nieves, J., Gorfu, G., and Ley, K. (2008). Leukocyte Adhesion Molecules in Animal Models of Inflammatory Bowel Disease. Inflamm. Bowel Dis. 14 (12), 1715–1735. doi:10.1002/ibd.20501
Russo, R. C., Garcia, C. C., Teixeira, M. M., and Amaral, F. A. (2014). The CXCL8/IL-8 Chemokine Family and its Receptors in Inflammatory Diseases. Expert Rev. Clin. Immunol. 10 (5), 593–619. doi:10.1586/1744666X.2014.894886
Schröder, J. M., Mrowietz, U., and Christophers, E. (1988). Purification and Partial Biologic Characterization of a Human Lymphocyte-Derived Peptide with Potent Neutrophil-Stimulating Activity. J. Immunol. 140 (10), 3534–3540.
Semenza, G. L. (2003). Targeting HIF-1 for Cancer Therapy. Nat. Rev. Cancer 3 (10), 721–732. doi:10.1038/nrc1187
Shevde, L. A., and Samant, R. S. (2014). Role of Osteopontin in the Pathophysiology of Cancer. Matrix Biol. 37, 131–141. doi:10.1016/j.matbio.2014.03.001
Smoot, M. E., Ono, K., Ruscheinski, J., Wang, P.-L., and Ideker, T. (2011). Cytoscape 2.8: New Features for Data Integration and Network Visualization. Bioinformatics 27 (3), 431–432. doi:10.1093/bioinformatics/btq675
Stallmach, A., Chan, C. C., Ecker, K. W., Feifel, G., Herbst, H., Schuppan, D., et al. (2000). Comparable Expression of Matrix Metalloproteinases 1 and 2 in Pouchitis and Ulcerative Colitis. Gut 47 (3), 415–422. doi:10.1136/gut.47.3.415
Tsai, S., and Gamblin, T. C. (2019). Molecular Characteristics of Biliary Tract and Primary Liver Tumors. Surg. Oncol. Clin. N. Am. 28 (4), 685–693. doi:10.1016/j.soc.2019.06.004
Ungaro, R., Mehandru, S., Allen, P. B., Peyrin-Biroulet, L., and Colombel, J.-F. (2017). Ulcerative Colitis. Lancet 389 (10080), 1756–1770. doi:10.1016/S0140-6736(16)32126-2
Uo, M., Hisamatsu, T., Miyoshi, J., Kaito, D., Yoneno, K., Kitazume, M. T., et al. (2013). Mucosal CXCR4+IgG Plasma Cells Contribute to the Pathogenesis of Human Ulcerative Colitis through FcγR-Mediated CD14 Macrophage Activation. Gut 62 (12), 1734–1744. doi:10.1136/gutjnl-2012-303063
Van der Goten, J., Vanhove, W., Lemaire, K., Van Lommel, L., Machiels, K., Wollants, W.-J., et al. (2014). Integrated miRNA and mRNA Expression Profiling in Inflamed Colon of Patients with Ulcerative Colitis. PLoS One 9 (12), e116117. doi:10.1371/journal.pone.0116117
Vancamelbeke, M., Vanuytsel, T., Farré, R., Verstockt, S., Ferrante, M., Van Assche, G., et al. (2017). Genetic and Transcriptomic Bases of Intestinal Epithelial Barrier Dysfunction in Inflammatory Bowel Disease. Inflamm. Bowel Dis. 23 (10), 1718–1729. doi:10.1097/MIB.0000000000001246
Veny, M., Fernández-Clotet, A., and Panés, J. (2020). Controlling Leukocyte Trafficking in IBD. Pharmacol. Res. 159, 105050. doi:10.1016/j.phrs.2020.105050
von Lampe, B., Barthel, B., Coupland, S. E., Riecken, E. O., and Rosewicz, S. (2000). Differential Expression of Matrix Metalloproteinases and Their Tissue Inhibitors in Colon Mucosa of Patients with Inflammatory Bowel Disease. Gut 47 (1), 63–73. doi:10.1136/gut.47.1.63
Wang, G. L., and Semenza, G. L. (1993). General Involvement of Hypoxia-Inducible Factor 1 in Transcriptional Response to Hypoxia. Proc. Natl. Acad. Sci. U.S.A. 90 (9), 4304–4308. doi:10.1073/pnas.90.9.4304
Wang, X., and Liotta, L. (2011). Clinical Bioinformatics: a New Emerging Science. J. Clin. Bioinforma. 1 (1), 1. doi:10.1186/2043-9113-1-1
Wang, Y.-D., Tan, X.-Y., and Zhang, K. (2009). Correlation of Plasma MMP-1 and TIMP-1 Levels and the Colonic Mucosa Expressions in Patients with Ulcerative Colitis. Mediat. Inflamm. 2009, 1–5. doi:10.1155/2009/275072
Wanninger, J., Neumeier, M., Weigert, J., Bauer, S., Weiss, T. S., Schäffler, A., et al. (2009). Adiponectin-stimulated CXCL8 Release in Primary Human Hepatocytes Is Regulated by ERK1/ERK2, P38 MAPK, NF-Κb, and STAT3 Signaling Pathways. Am. J. Physiol.-Gastrointest. Liver Physiol. 297 (3), G611–G618. doi:10.1152/ajpgi.90644.2008
Wu, B., Chien, E. Y. T., Mol, C. D., Fenalti, G., Liu, W., Katritch, V., et al. (2010). Structures of the CXCR4 Chemokine GPCR with Small-Molecule and Cyclic Peptide Antagonists. Science 330 (6007), 1066–1071. doi:10.1126/science.1194396
Xie, K., Kong, S., Li, F., Zhang, Y., Wang, J., and Zhao, W. (2020). Bioinformatics-Based Study to Investigate Potential Differentially Expressed Genes and miRNAs in Pediatric Sepsis. Med. Sci. Monit. 26, e923881. doi:10.12659/MSM.923881
Xue, G., Gao, R., Liu, Z., Xu, N., Cao, Y., Zhao, B., et al. (2021). Vitamin D/VDR Signaling Inhibits Colitis by Suppressing HIF-1α Activation in Colonic Epithelial Cells. Am. J. Physiol.-Gastrointest. Liver Physiol. 320 (5), G837–G846. doi:10.1152/ajpgi.00061.2021
Yan, P., He, Y., Xie, K., Kong, S., and Zhao, W. (2018). In Silico analyses for Potential Key Genes Associated with Gastric Cancer. PeerJ 6, e6092. doi:10.7717/peerj.6092
Zhou, J., Liu, J., Gao, Y., Shen, L., Li, S., and Chen, S. (2021). miRNA-Based Potential Biomarkers and New Molecular Insights in Ulcerative Colitis. Front. Pharmacol. 12, 707776. doi:10.3389/fphar.2021.707776
Zhou, G., Soufan, O., Ewald, J., Hancock, R. E. W., Basu, N., and Xia, J. (2019). NetworkAnalyst 3.0: a Visual Analytics Platform for Comprehensive Gene Expression Profiling and Meta-Analysis. Nucleic Acids Res. 47 (W1), W234–W241. doi:10.1093/nar/gkz240
Zhou, Y., Zhou, B., Pache, L., Chang, M., Khodabakhshi, A. H., Tanaseichuk, O., et al. (2019). Metascape Provides a Biologist-Oriented Resource for the Analysis of Systems-Level Datasets. Nat. Commun. 10 (1), 1523. doi:10.1038/s41467-019-09234-6
Keywords: ulcerative colitis, bioinformatics analysis, differentially expressed genes, microarray, protein-protein interaction, MicroRNAs
Citation: Hu W, Fang T and Chen X (2022) Identification of Differentially Expressed Genes and miRNAs for Ulcerative Colitis Using Bioinformatics Analysis. Front. Genet. 13:914384. doi: 10.3389/fgene.2022.914384
Received: 06 April 2022; Accepted: 09 May 2022;
Published: 02 June 2022.
Edited by:
Wei Xu, Texas A&M University Corpus Christi, United StatesReviewed by:
Chunying Li, Georgia State University, United StatesCopyright © 2022 Hu, Fang and Chen. This is an open-access article distributed under the terms of the Creative Commons Attribution License (CC BY). The use, distribution or reproduction in other forums is permitted, provided the original author(s) and the copyright owner(s) are credited and that the original publication in this journal is cited, in accordance with accepted academic practice. No use, distribution or reproduction is permitted which does not comply with these terms.
*Correspondence: Xiaoqing Chen, Y2hlbnhpYW9xaW5nMjAyMjAzQDE2My5jb20=
Disclaimer: All claims expressed in this article are solely those of the authors and do not necessarily represent those of their affiliated organizations, or those of the publisher, the editors and the reviewers. Any product that may be evaluated in this article or claim that may be made by its manufacturer is not guaranteed or endorsed by the publisher.
Research integrity at Frontiers
Learn more about the work of our research integrity team to safeguard the quality of each article we publish.