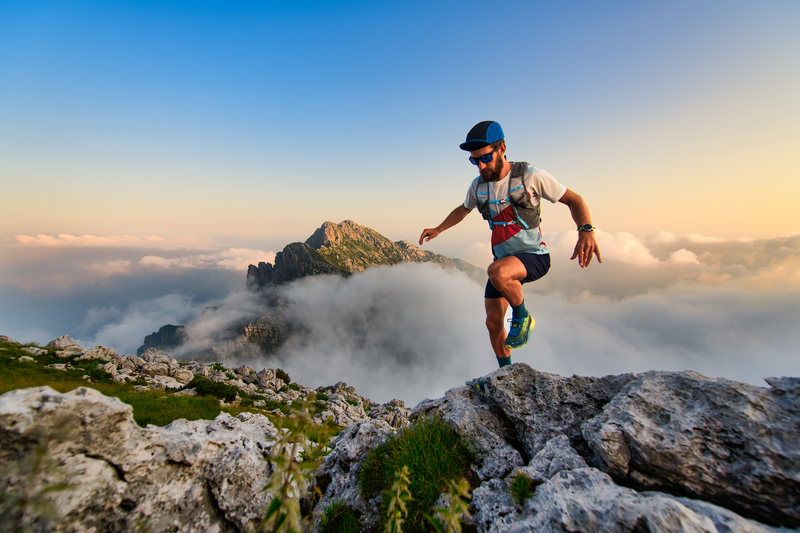
95% of researchers rate our articles as excellent or good
Learn more about the work of our research integrity team to safeguard the quality of each article we publish.
Find out more
REVIEW article
Front. Genet. , 05 August 2022
Sec. Evolutionary and Population Genetics
Volume 13 - 2022 | https://doi.org/10.3389/fgene.2022.910386
This article is part of the Research Topic Genetics of Domestication and Diversification Towards Evolution of Crop Plants View all 7 articles
Leveraging innovative tools to speed up prebreeding and discovery of genotypic sources of adaptation from landraces, crop wild relatives, and orphan crops is a key prerequisite to accelerate genetic gain of abiotic stress tolerance in annual crops such as legumes and cereals, many of which are still orphan species despite advances in major row crops. Here, we review a novel, interdisciplinary approach to combine ecological climate data with evolutionary genomics under the paradigm of a new field of study: genome–environment associations (GEAs). We first exemplify how GEA utilizes in situ georeferencing from genotypically characterized, gene bank accessions to pinpoint genomic signatures of natural selection. We later discuss the necessity to update the current GEA models to predict both regional- and local- or micro-habitat–based adaptation with mechanistic ecophysiological climate indices and cutting-edge GWAS-type genetic association models. Furthermore, to account for polygenic evolutionary adaptation, we encourage the community to start gathering genomic estimated adaptive values (GEAVs) for genomic prediction (GP) and multi-dimensional machine learning (ML) models. The latter two should ideally be weighted by de novo GWAS-based GEA estimates and optimized for a scalable marker subset. We end the review by envisioning avenues to make adaptation inferences more robust through the merging of high-resolution data sources, such as environmental remote sensing and summary statistics of the genomic site frequency spectrum, with the epigenetic molecular functionality responsible for plastic inheritance in the wild. Ultimately, we believe that coupling evolutionary adaptive predictions with innovations in ecological genomics such as GEA will help capture hidden genetic adaptations to abiotic stresses based on crop germplasm resources to assist responses to climate change.
“I shall endeavor to find out how nature’s forces act upon one another, and in what manner the geographic environment exerts its influence on animals and plants. In short, I must find out about the harmony in nature” Alexander von Humboldt—Letter to Karl Freiesleben, June 1799.
Crop wild relatives (CWR) and landraces are well known for providing new alleles for plant breeding (Tanksley and McCouch, 1997). They also can improve dietary proteins and essential micronutrients for undernourished communities (Blair, 2013). However, their diversity is often unexplored and underutilized (Bronnvik and von Wettberg, 2019; Ramirez-Villegas et al., 2022). Major cultivars typically lack adaptation to abiotic stresses (i.e., heat and drought), jeopardizing worldwide yield stability, given increasing effects of climate change (Cortés et al., 2020a). Luckily, landraces and CWR, as well as orphan crop species, offer novel adaptive alleles (Herron et al., 2020).
For instance, among legumes, bambara groundnut (Vigna subterranea), chickpea (Cicer arietinum), cowpea (V. unguiculata), grass pea (Lathyrus sativus), groundnut (Arachis hypogaea), marama bean (Tylosema esculentum) (Cullis et al., 2018), tarwi (Lupinus mutabilis) (Atchison et al., 2016; Gulisano et al., 2019), and tepary bean—(Phaseolus acutifolius) (Buitrago-Bitar et al., 2021; Burbano-Erazo et al., 2021) constitute genepools of unexplored adaptive diversity for abiotic stresses. Among cereals, orphan crops include teff (Eragrostis tef), sorghum (Sorghum bicolor), and finger millet (Eleusine coracana) or pearl and proso millets (Panicum spp, Pennisetum glaucum). Many of these crops have interesting drought-tolerance traits and some capacity to grow in compacted soils. Cowpeas, groundnuts, and lesser known cereals are already traditional food sources and biocultural components for vulnerable areas, especially in Sub-Saharan Africa and parts of Asia and Latin America (Xiong et al., 2016).
Yet, despite their tolerance to drought and heat plus their high nutritional quality, the utilization of these orphan crops is limited partly because of the poor characterization of their genetic background (Wu et al., 2022). Therefore, a key research area in orphan crop improvement is to expand the use of modern molecular prebreeding tools for them (Ahmad et al., 2020), that is, genome resequencing (Fuentes-Pardo and Ruzzante, 2017; Wu et al., 2020) and genomic prediction (Desta and Ortiz, 2014), to select genotypes for production in dry climates.
Still, a major question to harness crop prebreeding for climate change pressures is whether there is enough heritable variation in traits associated with tolerance to abiotic stress. In this context, genomic characterizations of reference collections comprising CWR, landraces, and orphan crops that span contrasting habitats offer a straightforward scenario to identify natural standing adaptation to abiotic pressures (Ramirez-Villegas et al., 2022).
The spirit behind this novel approach is to detect genomic regions that correlate with habitat heterogeneity as an indication of the natural selection imprint to environmental gradients (Forester et al., 2016). Since these signatures rely on a natural equilibrium between genotypes and their environment (Hancock et al., 2011), the ideal base population must prioritize natural genotypes and landraces and avoid improved cultivars, for which it is not realistic to assume that enough generations have passed as to display divergent selection to environmental heterogeneity. Hence, the goal of the present work is to review key developments to explore and utilize natural adaptation in wild genepools for climate change adaptation.
Coupling ecological genomics innovations (Cortinovis et al., 2020b) with evolutionary adaptive trajectories (Ramírez et al., 2010; López-Hernández and Cortés, 2019; Cortinovis et al., 2020a; Ramirez-Villegas et al., 2020) helps capturing adaptations in CWR and landraces, as shown for teosinte (Pyhäjärvi et al., 2013), rice (Meyer et al., 2016), Glycine (Anderson et al., 2016), and barley (Russell et al., 2016).
The current assortment of genomic pipelines that analyze environmental variation in order to infer the genetic basis of adaptation to natural selection include genome-wide selection scans—GWSS (Zahn and Purnell, 2016), and genome-wide environmental scans—GWES (Rellstab et al., 2015) also known as genome–environment associations—GEAs, a term we prefer based on its simplicity. While GWSS relies on the outlier Bayesian tests contrasted against a genomic background distribution (Antao et al., 2008), GEA uses mixed linear models (MLMs) that incorporate random effects, such as kinship and population stratification (Kruglyak, 2008). GEA behaves like traditional genome-wide association study (GWAS), but instead of modeling a set of phenotypic traits, it considers an environmentally derived variable into its additive genetic factors. These inferences could be misleading (Maher, 2008; Pennisi, 2014) if they overlook the confounding factors (Lambert and Black, 2012; Wolf and Ellegren, 2017) such as demographic (Barton et al., 2019) and genomic (Wray et al., 2013; Huber et al., 2016; Ellegren and Wolf, 2017) constraints also prevalent in GWAS studies. Hence, MLM-based models, which are capable of handling these spurious sources of error, are currently the optimum approach for the use of GEA and environmental variables to unveil the extent and genetic bases of local adaptation in diverse natural populations (Abebe et al., 2015).
In the last decade, the GEA pipeline has been utilized to characterize signatures of environmental adaptation in a rich spectrum of plant species (Table 1). For instance, Eckert et al. (2010) studied environmental associations with aridity across the range of the pine tree Pinus taeda, showing utility of the GEA approach for long-lifecycle, forestry species. This approach proved useful for additional tree species in studies by Holliday et al. (2016) and Pluess et al. (2016), respectively, who demonstrated local adaptation to climate gradients in Populus trichocarpa and Fagus sylvatica. More recently Ingvarsson and Bernhardsson (2020) address climate adaptation in P. tremula under present and future scenarios. With the increasing need for biomass as a fuel source, GEA is likely to continue its important role in the genetic analysis of other trees and woody species.
TABLE 1. Examples of GEA studies carried out in plant species. This compilation of previous studies explicitly refers to genome–environment association studies (GEA) using the Scopus database https://www.scopus.com/ with the following search parameters: TITLE-ABS-KEY (“Genome – Environment Associations”) AND (LIMIT-TO (DOCTYPE, “ar”)). The table is sorted chronologically. Method abbreviations are shown at the bottom of the table.
Meanwhile, for model plants, GEA has also gained in popularity and has a somewhat longer history: Hancock et al. (2011) and Lasky et al. (2012) have explored natural adaptive genetic variation in Arabidopsis at a continental scale and for water use efficiency, Yoder et al. (2014) disclosed the genetic basis of adaptation to drought and heat stress in Medicago truncatula.
For orphan crops, rather than nondomesticated natural plant species, fewer GEA studies have been undertaken. Although still limited to the easier-to-grow annual grain species compared to perennial and root/tuber crops, GEA is starting to make important contributions to genetic analysis of landraces and WCR germplasm, often richly represented in the world’s major gene banks for crop species. The analytical pipeline for GEA studies is shown in Figure 1, with the input data, analytical models, and outputs found when inferring genome-wide signatures of environmental adaptation in crop wild relatives (CWR) and landraces that span heterogeneous environments. The reader is referred to pertinent examples of GEA in crop species or their CWR which include Lasky et al. (2015), who prospected natural tolerance to aluminum toxicity and drought in cultivated sorghum; Berthouly-Salazar et al. (2016), who captured genomic regions involved in adaptation on two climate gradients in pearl millet; Cortés and Blair (2018a), who evaluated drought-tolerance sources of common bean (Phaseolus vulgaris) WCR; or López-Hernández and Cortés (2019), who identified pervasive divergent adaptation to continental-level heat gradients in wild accessions of this same species.
FIGURE 1. Analytical pipeline to infer genome-wide signatures of environmental adaptation in crop wild relatives (CWR) and landraces that span heterogeneous environments. The green shaded box refers to gene bank collections, while white, red and blue shaded boxes represent input data, analytical models and output inferences, respectively (Cortés et al., 2020a; Cortés et al., 2020b; Cortés and López-Hernández, 2021). Genomic prediction (GP) and genomic-estimated adaptation values (GEAVs) promise speeding up plant breeding goals.
Historically, based on the technological improvements in sequencing and SNP detection and as a means to improve GEA, the field has moved from the candidate gene approach (Cortés et al., 2012a; Cortés et al., 2012b; Blair et al., 2016; Buitrago-Bitar et al., 2021) into full genomic scans (Cortés and Blair, 2018a; López-Hernández and Cortés, 2022), which better account for linkage disequilibrium (LD) heterogeneity. In this movement, the targeting of discrete responses to abiotic pressures has aided GEA studies, by explicitly relying on the mechanistic ecophysiological models and traits (Cortés et al., 2013) for overall conditions such as drought (Cortés and Blair, 2018a) and heat stress (López-Hernández and Cortés, 2022).
As discussed previously, GEA is becoming a key tool to prospect for new genes among crop wild accessions and landraces as an alternative to traditional phenotyping and GWAS analyses (Cortés et al., 2022). However, there is still room for innovation. For example, we envision dual GEA models that combine inferences at various spatial and temporal scales, following Cortés et al. (2020a), by 1) predicting regional- and microhabitat-wise evolutionary adaptation with in situ ecological georeferencing of accessions, and by 2) revealing the genomic architecture of adaptation via cutting-edge predictive models (Rellstab et al., 2015; Forester et al., 2016).
Concerning the first point, standardized climate data for GEA studies are as important as high-quality genotyping to guarantee analytical power (Waldvogel et al., 2020). Climate-based inferences may target extreme regions, like those where drought is coupled with extreme temperatures (Lei et al., 2019). The high-resolution climate data can be gathered from worldwide repositories (such as WorldClim, https://www.worldclim.org/) using georeferencing and statistical downscaling (Zellweger et al., 2019) in order to build explicit heat–stress physiological indices (Cortés et al., 2013; López-Hernández and Cortés, 2019) at regional and local scales, respectively. The use of explicit indices, instead of raw environmental variables, helps describing physiological processes more accurately, especially those that confer tolerance to abiotic stress. For instance, the same environmental dataset can be inputted into an evapotranspiration model to infer drought stress (Cortés et al., 2013) and its genetic bases (Cortés et al., 2012a; Cortés et al., 2012b; Blair et al., 2016; Cortés and Blair, 2018a), or to assess heat tolerance (López-Hernández and Cortés, 2019). It is equally paramount to collect the spatial high-resolution climate data to improve predictions not only at regional scales (Pluess et al., 2016), but also at microhabitat levels (Cortés et al., 2015; Frachon et al., 2018), where adaptive variation to cope with abiotic pressures is overlooked but is sufficient (Cortés and Wheeler, 2018). Remote sensing (Zellweger et al., 2019) also promises better capture of environmental heterogeneity (Ratcliffe et al., 2019).
Concerning the second opportunity for improvement, characterizing genome-wide signatures of environmental adaptation to habitat-inferred stress in CWR and landraces that span heterogeneous climates will benefit from inputting habitat-based abiotic stress indices into the last-generation mixed linear models (MLMs) and machine learning (ML) (Schrider and Kern, 2018; Cortés et al., 2020b), capable of handling spurious effects (Barton et al., 2019) in multidimensional data, while detecting predictive genomic regions that correlate with habitat/environmental heterogeneity, as an indication of the genomic imprint by climate gradients (Hancock et al., 2011).
Overall, CWR and landraces undeniably harbor unique adaptations to abiotic stresses, rarely present in the cultivated and improved genepools (Tester and Langridge, 2010). However, unlocking and utilizing this potential (Tanksley and McCouch, 1997; Langridge and Robbie, 2019) has remained challenging partly due to phenotyping bottlenecks in the wild, and the complex inheritance (Morran et al., 2011) of trait variation for abiotic stress tolerance, typically involving many loci with low effects (López-Hernández and Cortés, 2019). To bridge this gap, we propose extending genomic prediction (GP) models and genomic estimated breeding values (GEBVs) to account for the habitat-based dimensions by coining the analogous parameter genomic estimated adaptation values—GEAVs (Capblancq et al., 2020; Arenas et al., 2021), equivalent to the polygenic risk score (PRS) within a preimplantation genetic diagnosis framework. To compute GEAVs, the GP models used must be calibrated (trained and tested) to predict the environmental indices and polygenic adaptability (liability) thresholds.
GP works either on the basis of shared relatedness (typically measured as relationships due to recent coancestry) or on the basis of linkage disequilibrium (LD) between the SNP marker loci and the genetic variants that underlie phenotypic variation (Thistlethwaite et al., 2020). The relationships between the training and testing datasets are critically important and therefore must be optimized as part of any GP effort. As a general suggestion, the more diverse the training dataset is, the more robust the prediction will be. Hence, coupling cross-validation calibration curves under various training/testing ratios with simple measures of diversity, such as heterozygosity and runs of homozygosity (ROH), is appropriate. However, in the absence of demonstrated relationships or LD, there is no apparent basis for GP’s predictive accuracy, raising the possibility of statistical artifacts. To overcome this issue, pairwise LD among SNP markers helps to interpret GWAS-type GEA associations (Morton, 2005), and this a prerequisite for any GP initiative.
The predictive ability of GP models may be further biased depending on whether the entire SNP set is used, or the most predictive SNP data are chosen after the GWAS-type analysis (Wray et al., 2013), the latter being a common practice in modern GP studies. The polygenic infinitesimal model that makes GP so unique may be jeopardized by subsampling the SNP markers because relying only on GEA-derived SNP markers would disregard SNPs with low effects that are usually missed, but that may still account for the overall missing heritability.
Better approaches to be implemented and reported include 1) weighted GP models using de novo GWAS-based GEA estimates gathered from other (or even the same) panels (Spindel et al., 2016) and 2) optimization of the marker set by computing the saturating curves of the predictive ability, given various sets of markers ranked by their beta effects from the exact same GP model and not from any parallel GWAS-derived GEA models (Resende et al., 2012; Tan et al., 2017). GP can also be improved in efficiency by validating the GEAV scores across diverse populations, allowing better G × E predictions within the nascent field of enviromics (Resende et al., 2021; Cooper and Messina, 2021; Costa-Neto et al., 2021). GEAVs can boost incorporation of landraces and CWR as parents in marker-assisted backcrossing (MAB). Marker set optimization for environmental GP (Jarquín et al., 2013; Lopez-Cruz et al., 2015) would benefit crop prebreeding initiatives for abiotic tolerance MAB, mergeable with the speed-breeding strategies (Migicovsky and Myles, 2017; Watson et al., 2018), high-throughput screening (Cuppen, 2007), and ML-updated best linear unbiased prediction (BLUP) models (Wenlong et al., 2018; Crossa et al., 2019; Abdollahi-Arpanahi et al., 2020; Cortés et al., 2020b; Wang et al., 2020; Zingaretti et al., 2020; Montesinos-López et al., 2021).
Next-generation GEA models and GEAVs will allow the use of “exotic” parents for targeted predictive prebreeding in crop species. They offer feasible methodologies to trace the sources of abiotic stress adaptation and tolerance targeting crop resources in low-income countries, which are also the most vulnerable to climate change. Here, we have discussed strategies to implement the latest-generation predictive (e.g., Cortés et al., 2013) and genomic (Cortés and Blair, 2018a; López-Hernández and Cortés, 2019) approaches to study adaptation in CWR and landraces (Cortés and Blair, 2018b; Cortés et al., 2020a), but similar work could be done for any orphan crop.
Modern GEA approaches will allow further studies of evolutionary conservatism, parallelism, and convergence in the genetic architecture of adaptation to various types of abiotic stresses across a wide range of environments, landraces, and wild accessions (Cortés and López-Hernández, 2021). Approaches such as these are needed to discern among drivers (Ellegren and Galtier, 2016) of the adaptive landscape of genomic divergence (Feder et al., 2012; Gompert et al., 2014; Cortés et al., 2018b), such as ecological diversity, population structure, ancestral polymorphisms, mutation/recombination rates (Feder et al., 2012; Ellegren and Wolf, 2017; Ravinet et al., 2017; Cortés and Blair, 2018b), and nested levels of divergence (Nosil and Feder, 2011; Wolf and Ellegren, 2017; Cortés et al., 2018a).
How genetic diversity and genomic divergence arise and are shaped based on ecological pressures is one of the main questions in molecular evolution (Tiffin and Ross-Ibarra, 2014) and has been implicit even in the pregenetics-era studies of ecological transects by famous botanists such as von Humboldt. GEA studies can contribute valuable insights into the field of molecular evolution due to their ability to detect convergent or nonconvergent adaptations (Schmutz et al., 2014). Ecologically associated SNPs are likely to exhibit hitchhiking effects (Feder and Nosil, 2010) due to the low recombination rate and extensive LD (Kelleher et al., 2012; Blair et al., 2018).
Therefore, GEA efforts can be enhanced by exploring SNP density and statistics of site frequency spectra (e.g., nucleotide diversity and Tajima’s D) in associated vs. nonassociated regions (Cortés and Blair, 2018a). Figure 2 shows an example of these principles used in common bean to identify and harness natural signatures of environmental adaptation across diverse genepools, with the genome-wide patterns of genetic divergence, as measured by the FST statistic (A). Once the potential confounding demographic patterns have been accounted for, it is then feasible to disentangle genuine signatures of environmental adaptation (B) from spurious concurring genetic drift due to genomic constraining features. Finally, these combined summary statistics (i.e., ecophysiological indices, population stratification, and LD) can ultimately redound in prebreeding efforts aiming to introgress exotic adaptive variation into elite lines (C), for instance, via backcrossing (BC) schemes for abiotic and biotic stresses.
FIGURE 2. An integrated case study inspired in common bean (Phaseolus vulgaris L.) accessions exemplifies how to identify and harness natural signatures of environmental adaptation across diverse genepools. First, (A) genome-wide patterns of genetic divergence, as measured by the FST and delta divergence (Roesti et al., 2014) statistics, inform underlying confounding demographic processes across wild accessions (Blair et al., 2012) and landraces (Blair et al., 2009). Even though highly polymorphic markers have traditionally been preferred for demographic inferences (Blair et al., 2009; Kwak and Gepts, 2009), modern SNP genotyping technologies also enable reconstructing the genomic landscape of divergence at a higher resolution (Cortés et al., 2011; Wu et al., 2020). Once potential confounding demographic patterns have been accounted for, (B) it is then feasible to disentangle genuine (in red) signatures of environmental adaptation (Cortés et al., 2018b) from spurious concurring genetic drift due to genomic constraining features such as low recombining regions, reduced effective population size, and translocations (Blair et al., 2018). In order to improve genome–environment associations (GEA), the field has moved from the candidate gene approach (Cortés et al., 2012a; Cortés et al., 2012b; Blair et al., 2016; Buitrago-Bitar et al., 2021) into full genomic scans (Cortés and Blair, 2018a; López-Hernández and Cortés, 2022), which better account for linkage disequilibrium (LD) heterogeneity. It is also advisable to target discrete abiotic pressures by explicitly relying on the mechanistic ecophysiological models (Cortés et al., 2013) such as drought (Cortés and Blair, 2018a) and heat stress (López-Hernández and Cortés, 2022). Finally, (C) these combined summary statistics (i.e., ecophysiological indices, population stratification, and LD) can ultimately redound in prebreeding efforts aiming to introgress exotic adaptive variation into elite lines, for instance, via backcrossing (BC) schemes for abiotic (Muñoz et al., 2003; Blair et al., 2006; Blair and Izquierdo, 2012; Burbano-Erazo et al., 2021) and biotic (Garzon et al., 2008) stresses, all guided with indirect (Miklas et al., 2006) genomic selection tools such as marker-assisted selection (MAS) and genomic selection, GS (Cortés et al., 2020a; Cortés et al., 2020b; Cortés and López-Hernández, 2021) within a moder enviromics approach (Cooper et al., 2021). Different line colors stand for hypothetical distinct chromosomes. Dashed horizontal lines mark significance thresholds.
All drivers must be considered as ad hoc multiple hypotheses (Chamberlin, 1897) since extensive LD and hitchhiking may not only be due to physical linkage and low recombination/effective population size (Slatkin, 2008), but also to population stratification, sample’s co-ancestry (Price et al., 2010; Blair et al., 2012), within-pathway gene-gene and G × E interactions (Ortiz et al., 2022), and context-dependent effects of epistasis, even if assumed to be minimal in diploid model crop species.
In-depth GEA studies on the molecular mechanisms of evolutionary divergence involving the genomics and functional genetic dissection of adaptive loci enable addressing long-term transdisciplinary questions such as 1) how genomic features (i.e., meiotic crossover hot- vs. cold-spots, as pericentromeric regions and inversions) impact the rate of adaptation and modulate adaptive evolution to new environments (Huang et al., 2020; Huang and Rieseberg, 2020; Todesco et al., 2020), 2) how old adaptive haplotypes (standing variation) and more recent recruitment of novel mutations balance during the rapid events of changing climate (Jones et al., 2012a; Jones et al., 2012b), 3) what effects the noncoding cis-regulatory mutations contribute to the genomic basis of adaptation, and 4) to which scale epigenomic marks [i.e., chromatin accessibility, histone profiling, transposable elements, and sRNA (Kaasik and Chi Lee, 2004; Slotkin and Martienssen, 2007)] regulate plastic gene expression within the same genotype (Bossdorf et al., 2008), and may be transferred via transgenerational epigenetic inheritance (Heard and Martienssen, 2014; Boskovic and Rando, 2018; Hu et al., 2018; Lacal and Ventura, 2018), eventually impacting divergent adaptation in natural populations (Chinnusamy and Zhu, 2009).
Ultimately, GEA is empowering the understanding on how plant genomes interact with their environment while shaping adaptive phenotypes. Such mechanistic insights of the genome functionality in the wild promise leveraging the characterization of landraces and CWR to assist prebreeding efforts through multi-dimensional adaptive scores (e.g. GEAVs), as well as the identification of underlying factors that may facilitate or constrain future adaptive responses to changing climate.
Lastly, GEA studies offer new possibilities to efficiently unlock crop diversity for climate adaptation (Tanksley and McCouch, 1997). Unexplored variation already contained in genebanks (Smale and Jamora, 2020) may speed up resilience to extreme temperatures, and more frequent drought and flooding events (Dwivedi et al., 2016). The modern genome–environment framework, coupled with explicit ecophysiological indices and last-generation association models, promises a scalable strategy to assist with the identification and deployment of exotic variation capable of maturing earlier and harvesting acceptably in erratic climatic conditions. Levering reverse genomic and ecological resources for CWR and landraces will improve available pipelines such as focused identification of germplasm strategy, FIGS (Stenberg and Ortiz, 2021). Such efforts to prebreed resilient crop genotypes with greater accuracy may ultimately enable small-scale farmers’ adaptation to changing climate (Razzaq et al., 2021).
The original contributions presented in the study are included in the article. Further inquiries can be directed to the corresponding author.
AJC and MWB conceived this work. FL-H collected literature and prepared tables. AJC prepared diagrams and wrote a first draft, later edited by MWB and FL-H.
Vetenskapsrådet and Kungliga Vetenskapsakademien are credited for funding AJC during the conception of this work through grants 4.1-2016-00418 and BS20170036. AGROSAVIA’s Department for Research Capacity Building is acknowledged for sponsoring FL-H’s internship in 2018 to carry out GEA studies for abiotic stresses. United States Department of Agriculture Evans Allen fund (TENX-07) is acknowledged for funding MWB. The Graduate Research School in Genomic Ecology (GENECO) from Lund University is appreciated for the mobility funding that assisted the synergistic kickoff meeting between AJC and MWB in the spring of 2015 at Nashville, TN, United States. Fulbright U.S. Specialist Program is thanked for supporting MWB’s visit to AJC and FL-H in Rionegro (Antioquia, Colombia) in the summer of 2019, during which the foundations of this review were discussed. The British Council is deeply appreciated for the Newton Fund, Grant ID 527023146, awarded to AJC as PI in Colombia, which enabled additional discussions among authors around the GEA paradigm.
The authors declare that the research was conducted in the absence of any commercial or financial relationships that could be construed as a potential conflict of interest.
All claims expressed in this article are solely those of the authors and do not necessarily represent those of their affiliated organizations, or those of the publisher, the editors, and the reviewers. Any product that may be evaluated in this article, or claim that may be made by its manufacturer, is not guaranteed or endorsed by the publisher.
Discussions with D. Ambachew, A. Asfaw, E. Burbano, C. Cordero, C.H. Galeano, G. Hyman, R. León, C.I. Medina, M. Mercado, F. Monserrate, D. Peláez, A. Tofiño, and M. Urban inspired some of the perspectives of GEA using climatic variables and crop utilization in hot and dry climatic zones discussed in this review. Recognition is also given to M.J. Torres for assistance during the preparation of this review. Reviewers and topic editors are thanked for envisioning and enabling an insightful Special Issue on “Genetics of Domestication and Diversification Towards Evolution of Crop Plants.”
Abdollahi-Arpanahi, R., Gianola, D., and Peñagaricano, F. (2020). Deep Learning versus Parametric and Ensemble Methods for Genomic Prediction of Complex Phenotypes. Genet. Sel. Evol. 52. doi:10.1186/s12711-020-00531-z
Abebe, T. D., Naz, A. A., and Léon, J. (2015). Landscape Genomics Reveal Signatures of Local Adaptation in Barley (Hordeum Vulgare L.). Front. Plant Sci. 6, 813. doi:10.3389/fpls.2015.00813
Ahmad, R., Akbar Anjum, M., and Mukhtar Balal, R. (2020). From Markers to Genome Based Breeding in Horticultural Crops: An Overview. Phyton 89, 183–204. doi:10.32604/phyton.2020.08537
Anderson, J. E., Kono, T. J. Y., Stupar, R. M., Kantar, M. B., and Morrell, P. L. (2016). Environmental Association Analyses Identify Candidates for Abiotic Stress Tolerance in Glycine Soja, the Wild Progenitor of Cultivated Soybeans. G3 Genes|Genomes|Genetics 6, 835–843. doi:10.1534/g3.116.026914
Antao, T., Lopes, A., Lopes, R. J., Beja-Pereira, A., and Luikart, G. (2008). LOSITAN: A Workbench to Detect Molecular Adaptation Based on a F St -outlier Method. BMC Bioinforma. 9, 323. doi:10.1186/1471-2105-9-323
Arenas, S., Cortés, A. J., Mastretta-Yanes, A., and Jaramillo-Correa, J. P. (2021). Evaluating the Accuracy of Genomic Prediction for the Management and Conservation of Relictual Natural Tree Populations. Tree Genet. Genomes 17. doi:10.1007/s11295-020-01489-1
Atchison, G. W., Nevado, B., Eastwood, R. J., Contreras-Ortiz, N., Reynel, C., Madrinan, S., et al. (2016). Lost Crops of the Incas: Origins of Domestication of the Andean Pulse Crop Tarwi, Lupinus Mutabilis. Am. J. Bot. 103, 1592–1606. doi:10.3732/ajb.1600171
Barton, N., Hermisson, J., and Nordborg, M. (2019). Why Structure Matters. Elife 8. doi:10.7554/eLife.45380
Berthouly-Salazar, C., Thuillet, A.-C., Rhoné, B., Mariac, C., Ousseini, I. S., Couderc, M., et al. (2016). Genome Scan Reveals Selection Acting on Genes Linked to Stress Response in Wild Pearl Millet. Mol. Ecol. 25, 5500–5512. doi:10.1111/mec.13859
Blair, M. W. (2013). Mineral Biofortification Strategies for Staples: The Example of Common Bean. J. Agric. Food Chem. 61. doi:10.1021/jf400774y
Blair, M. W., Cortés, A. J., Farmer, A. D., Huang, W., Ambachew, D., Penmetsa, R. V., et al. (2018). Uneven Recombination Rate and Linkage Disequilibrium across a Reference SNP Map for Common Bean (Phaseolus vulgaris L.). PLoS One 13, e0189597. doi:10.1371/journal.pone.0189597
Blair, M. W., Cortés, A. J., and This, D. (2016). Identification of an Erecta Gene and its Drought Adaptation Associations with Wild and Cultivated Common Bean. Plant Sci. 242, 250–259. doi:10.1016/j.plantsci.2015.08.004
Blair, M. W., Díaz, L. M., Buendía, H. F., and Duque, M. C. (2009). Genetic Diversity, Seed Size Associations and Population Structure of a Core Collection of Common Beans (Phaseolus vulgaris L.). Theor. Appl. Genet. 119, 955–972. doi:10.1007/s00122-009-1064-8
Blair, M. W., Iriarte, G., and Beebe, S. (2006). QTL Analysis of Yield Traits in an Advanced Backcross Population Derived from a Cultivated Andean × Wild Common Bean (Phaseolus vulgaris L.) Cross. Theor. Appl. Genet. 112, 1149–1163. doi:10.1007/s00122-006-0217-2
Blair, M. W., and Izquierdo, P. (2012). Use of the Advanced Backcross-Qtl Method to Transfer Seed Mineral Accumulation Nutrition Traits from Wild to Andean Cultivated Common Beans. Theor. Appl. Genet. 125, 1015–1031. doi:10.1007/s00122-012-1891-x
Blair, M. W., Soler, A., and Cortés, A. J. (2012). Diversification and Population Structure in Common Beans (Phaseolus vulgaris L.). Plos One 7, e49488. doi:10.1371/journal.pone.0049488
Blanco-Pastor, J. L., Barre, P., Keep, T., Ledauphin, T., Escobar-Gutiérrez, A., Roschanski, A. M., et al. (2020). Canonical Correlations Reveal Adaptive Loci and Phenotypic Responses to Climate in Perennial Ryegrass. Mol. Ecol. Resour. 21, 849–870. doi:10.1111/1755-0998.13289
Blanco-Pastor, J. L., Liberal, I. M., Sakiroglu, M., Wei, Y., Brummer, E. C., Andrew, R. L., et al. (2021). Annual and Perennial Medicago Show Signatures of Parallel Adaptation to Climate and Soil in Highly Conserved Genes. Mol. Ecol. 30, 4448–4465. doi:10.1111/mec.16061
Borrell, J. S., Zohren, J., Nichols, R. A., and Buggs, R. J. A. (2019). Genomic Assessment of Local Adaptation in Dwarf Birch to Inform Assisted Gene Flow. Evol. Appl. 13, 161–175. doi:10.1111/eva.12883
Boskovic, A., and Rando, O. J. (2018). Transgenerational Epigenetic Inheritance. Annu. Rev. Genet. 52.
Bossdorf, O., Richards, C. L., and Pigliucci, M. (2008). Epigenetics for Ecologists. Ecol. Lett. 11, 106–115. doi:10.1111/j.1461-0248.2007.01130.x
Bronnvik, H., and Von Wettberg, E. J. (2019). Bird Dispersal as a Pre-adaptation for Domestication in Legumes: Insights for Neo-Domestication. Front. Plant Sci. 10, 1293.
Buitrago-Bitar, M. A., Cortés, A. J., López-Hernández, F., Londoño-Caicedo, J. M., Muñoz-Florez, J. E., Muñoz, L. C., et al. (2021). Allelic Diversity at Abiotic Stress Responsive Genes in Relationship to Ecological Drought Indices for Cultivated Tepary Bean, Phaseolus acutifolius A. Gray, and its Wild Relatives. Genes 12, 556. doi:10.3390/genes12040556
Burbano-Erazo, E., León-Pacheco, R., Cordero-Cordero, C., López-Hernández, F., Cortés, A., and Tofiño-Rivera, A. (2021). Multi-Environment Yield Components in Advanced Common Bean (Phaseolus vulgaris L.) × Tepary Bean (P. acutifolius A. Gray) Interspecific Lines for Heat and Drought Tolerance. Agronomy 11, 1978. doi:10.3390/agronomy11101978
Capblancq, T., Fitzpatrick, M. C., Bay, R. A., Exposito-Alonso, M., and Keller, S. R. (2020). Genomic Prediction of (Mal)Adaptation across Current and Future Climatic Landscapes. Annu. Rev. Ecol. Evol. Syst. 51, 245–269. doi:10.1146/annurev-ecolsys-020720-042553
Chinnusamy, V., and Zhu, J.-K. (2009). Epigenetic Regulation of Stress Responses in Plants. Curr. Opin. Plant Biol. 12, 133–139. doi:10.1016/j.pbi.2008.12.006
Cooper, M., and Messina, C. D. (2021). Can We Harness “Enviromics” to Accelerate Crop Improvement by Integrating Breeding and Agronomy? Front. Plant Sci. 12, 735143. doi:10.3389/fpls.2021.735143
Cortés, A. J., and Blair, M. W. (2018a). Genotyping by Sequencing and Genome-Environment Associations in Wild Common Bean Predict Widespread Divergent Adaptation to Drought. Front. Plant Sci. 9, 128. doi:10.3389/fpls.2018.00128
Cortés, A. J., and Blair, M. W. (2018b). “Lessons from Common Bean on How Wild Relatives and Landraces Can Make Tropical Crops More Resistant to Climate Change,” in Rediscovery of Landraces as a Resource for the Future. Editor O. Grillo (Rijeka, Croatia: InTech). doi:10.5772/intechopen.71669
Cortés, A. J., Chavarro, M. C., and Blair, M. W. (2011). SNP Marker Diversity in Common Bean (Phaseolus vulgaris L.). Theor. Appl. Genet. 123, 827–845. doi:10.1007/s00122-011-1630-8
Cortés, A. J., Chavarro, M. C., Madriñán, S., This, D., and Blair, M. W. (2012a). Molecular Ecology and Selection in the Drought-Related Asr Gene Polymorphisms in Wild and Cultivated Common Bean (Phaseolus vulgaris L.). BMC Genet. 13. doi:10.1186/1471-2156-13-58
Cortés, A. J., Cornille, A., and Yockteng, R. (2022). Evolutionary Genetics of Crop-Wild Complexes. Genes 13. doi:10.3390/genes13030420
Cortés, A. J., Hurtado, P., Blair, M. W., and Chacón-Sánchez, M. I. (2018a). “Common Bean Genome Diversity Reveals the Genomic Consequences of Speciation, Adaptation and Domestication,” in Genetic Diversity in Plants. Editor M. Caliskan (Rijeka, Croatia: InTech).
Cortés, A. J., and López-Hernández, F. (2021). Harnessing Crop Wild Diversity for Climate Change Adaptation. Genes 12, 783. doi:10.3390/genes12050783
Cortés, A. J., López-Hernández, F., and Osorio-Rodriguez, D. (2020a). Predicting Thermal Adaptation by Looking into Populations' Genomic Past. Front. Genet. 11, 564515. doi:10.3389/fgene.2020.564515
Cortés, A. J., Monserrate, F. A., Ramírez-Villegas, J., Madriñán, S., and Blair, M. W. (2013). Drought Tolerance in Wild Plant Populations: The Case of Common Beans (Phaseolus vulgaris L.). Plos One 8, e62898. doi:10.1371/journal.pone.0062898
Cortés, A. J., Restrepo-Montoya, M., and Bedoya-Canas, L. E. (2020b). Modern Strategies to Assess and Breed Forest Tree Adaptation to Changing Climate. Front. Plant Sci. 11, 583323. doi:10.3389/fpls.2020.583323
Cortés, A. J., Skeen, P., Blair, M. W., and Chacón-Sánchez, M. I. (2018b). Does the Genomic Landscape of Species Divergence in Phaseolus Beans Coerce Parallel Signatures of Adaptation and Domestication? Front. Plant Sci. 9, 1816. doi:10.3389/fpls.2018.01816
Cortés, A. J., This, D., Chavarro, C., Madriñán, S., and Blair, M. W. (2012b). Nucleotide Diversity Patterns at the Drought-Related Dreb2 Encoding Genes in Wild and Cultivated Common Bean (Phaseolus vulgaris L.). Theor. Appl. Genet. 125, 1069–1085. doi:10.1007/s00122-012-1896-5
Cortés, A. J., Wheeler, J. A., Sedlacek, J., Lexer, C., and Karrenberg, S. (2015). “Genome-Wide Patterns of Microhabitat-Driven Divergence in the Alpine Dwarf Shrub Salix herbacea L,” in On The Big Challenges Of a Small Shrub: Ecological Genetics Of Salix herbacea L (Uppsala: Acta Universitatis Upsaliensis).
Cortés, A. J., and Wheeler, J. A. (2018). “The Environmental Heterogeneity of Mountains at a Fine Scale in a Changing World,” in Mountains, Climate, and Biodiversity. Editors C. Hoorn, A. Perrigo, and A. Antonelli (NY: Wiley).
Cortinovis, G., Di Vittori, V., Bellucci, E., Bitocchi, E., and Papa, R. (2020a). Adaptation to Novel Environments during Crop Diversification. Curr. Opin. Plant Biol. 56, 203–217. doi:10.1016/j.pbi.2019.12.011
Cortinovis, G., Frascarelli, G., Di Vittori, V., and Papa, R. (2020b). Current State and Perspectives in Population Genomics of the Common Bean. Plants (Basel) 9, 330. doi:10.3390/plants9030330
Costa-Neto, G., Galli, G., Carvalho, H. F., Crossa, J., Fritsche-Neto, R., and De Koning, D. J. (2021). Envrtype: A Software to Interplay Enviromics and Quantitative Genomics in Agriculture. G3 Genes|Genomes|Genetics 11. doi:10.1093/g3journal/jkab040
Cooper, M., and Messina, C. D. (2021). Can We Harness “Enviromics” to Accelerate Crop Improvement by Integrating Breeding and Agronomy?. Front. Plant Sci. 12, 735143. doi:10.3389/fpls.2021.735143
Crossa, J., Martini, J. W. R., Gianola, D., Pérez-Rodríguez, P., Jarquin, D., Juliana, P., et al. (2019). Deep Kernel and Deep Learning for Genome-Based Prediction of Single Traits in Multienvironment Breeding Trials. Front. Genet. 10, 1168. doi:10.3389/fgene.2019.01168
Cullis, C., Chimwamurombe, P., Barker, N., Kunert, K., and Vorster, J. (2018). Orphan Legumes Growing in Dry Environments: Marama Bean as a Case Study. Front. Plant Sci. 9, 1199. doi:10.3389/fpls.2018.01199
Cuppen, E. (2007). Genotyping by Allele-specific Amplification (Kaspar). CSH Protoc. 2007. doi:10.1101/pdb.prot4841
Desta, Z. A., and Ortiz, R. (2014). Genomic Selection: Genome-wide Prediction in Plant Improvement. Trends Plant Sci. 19, 592–601. doi:10.1016/j.tplants.2014.05.006
Du, F. K., Wang, T., Wang, Y., Ueno, S., and Lafontaine, G. (2020). Contrasted Patterns of Local Adaptation to Climate Change across the Range of an Evergreen Oak, Quercus Aquifolioides. Evol. Appl. 13, 2377–2391. doi:10.1111/eva.13030
Dwivedi, S. L., Ceccarelli, S., Blair, M. W., Upadhyaya, H. D., Are, A. K., and Ortiz, R. (2016). Landrace Germplasm for Improving Yield and Abiotic Stress Adaptation. Trends Plant Sci. 21, 31–42. doi:10.1016/j.tplants.2015.10.012
Eckert, A. J., Van Heerwaarden, J., Wegrzyn, J. L., Nelson, C. D., Ross-Ibarra, J., Gonzalez-Martinez, S. C., et al. (2010). Patterns of Population Structure and Environmental Associations to Aridity across the Range of Loblolly Pine (Pinus Taeda L., Pinaceae). Genetics 185, 969–982. doi:10.1534/genetics.110.115543
Elias, J. C. F., Gonçalves-Vidigal, M. C., Ariani, A., Valentini, G., Martiniano-Souza, M. d. C., Vaz Bisneta, M., et al. (2021). Genome-Environment Association Analysis for Bio-Climatic Variables in Common Bean (Phaseolus vulgaris L.) from Brazil. Plants 10, 1572. doi:10.3390/plants10081572
Ellegren, H., and Galtier, N. (2016). Determinants of Genetic Diversity. Nat. Rev. Genet. 17, 422–433. doi:10.1038/nrg.2016.58
Ellegren, H. (2004). Microsatellites: Simple Sequences with Complex Evolution. Nat. Rev. Genet. 5, 435–445. doi:10.1038/nrg1348
Ellegren, H., and Wolf, J. B. W. (2017). Parallelism in Genomic Landscapes of Differentiation, Conserved Genomic Features and the Role of Linked Selection. J. Evol. Biol. 30, 1516–1518. doi:10.1111/jeb.13113
Feder, J. L., Gejji, R., Yeaman, S., and Nosil, P. (2012). Establishment of New Mutations under Divergence and Genome Hitchhiking. Phil. Trans. R. Soc. B 367, 461–474. doi:10.1098/rstb.2011.0256
Feder, J. L., and Nosil, P. (2010). The Efficacy of Divergence Hitchhiking in Generating Genomic Islands during Ecological Speciation. Evolution 64, 1729–1747. doi:10.1111/j.1558-5646.2009.00943.x
Forester, B. R., Jones, M. R., Joost, S., Landguth, E. L., and Lasky, J. R. (2016). Detecting Spatial Genetic Signatures of Local Adaptation in Heterogeneous Landscapes. Mol. Ecol. 25, 104–120. doi:10.1111/mec.13476
Frachon, L., Bartoli, C., Carrère, S., Bouchez, O., Chaubet, A., Gautier, M., et al. (2018). A Genomic Map of Climate Adaptation in Arabidopsis thaliana at a Micro-geographic Scale. Front. Plant Sci. 9, 967. doi:10.3389/fpls.2018.00967
Fuentes-Pardo, A. P., and Ruzzante, D. E. (2017). Whole-Genome Sequencing Approaches for Conservation Biology: Advantages, Limitations and Practical Recommendations. Mol. Ecol. 26, 5369–5406. doi:10.1111/mec.14264
Garzón, L. N., Ligarreto, G. A., and Blair, M. W. (2008). Molecular Marker-Assisted Backcrossing of Anthracnose Resistance into Andean Climbing Beans (Phaseolus vulgaris L.). Crop Sci. 48, 562–570. doi:10.2135/cropsci2007.08.0462
Girma, G., Nida, H., Tirfessa, A., Lule, D., Bejiga, T., Seyoum, A., et al. (2020). A Comprehensive Phenotypic and Genomic Characterization of Ethiopian Sorghum Germplasm Defines Core Collection and Reveals Rich Genetic Potential in Adaptive Traits. Plant Genome 13, e2005. doi:10.1002/tpg2.20055
Gompert, Z., Comeault, A. A., Farkas, T. E., Feder, J. L., Parchman, T. L., Buerkle, C. A., et al. (2014). Experimental Evidence for Ecological Selection on Genome Variation in the Wild. Ecol. Lett. 17, 369–379. doi:10.1111/ele.12238
Guerrero, J., Andrello, M., Burgarella, C., and Manel, S. (2018). Soil Environment Is a Key Driver of Adaptation in Medicago truncatula: New Insights from Landscape Genomics. New Phytol. 219, 378–390. doi:10.1111/nph.15171
Gulisano, A., Alves, S., Martins, J. N., and Trindade, L. M. (2019). Genetics and Breeding of Lupinus Mutabilis: An Emerging Protein Crop. Front. Plant Sci. 10, 1385. doi:10.3389/fpls.2019.01385
Hancock, A. M., Brachi, B., Faure, N., Horton, M. W., Jarymowycz, L. B., Sperone, F. G., et al. (2011). Adaptation to Climate across the Arabidopsis thaliana Genome. Science 334, 83–86. doi:10.1126/science.1209244
Heard, E., and Martienssen, R. A. (2014). Transgenerational Epigenetic Inheritance: Myths and Mechanisms. Cell 157, 95–109. doi:10.1016/j.cell.2014.02.045
Herron, S. A., Rubin, M. J., Ciotir, C., Crews, T. E., Van Tassel, D. L., and Miller, A. J. (2020). Comparative Analysis of Early Life Stage Traits in Annual and Perennial Phaseolus Crops and Their Wild Relatives. Front. Plant Sci. 11, 34. doi:10.3389/fpls.2020.00034
Holliday, J. A., Zhou, L., Bawa, R., Zhang, M., and Oubida, R. W. (2016). Evidence for Extensive Parallelism but Divergent Genomic Architecture of Adaptation along Altitudinal and Latitudinal Gradients in P Opulus Trichocarpa. New Phytol. 209, 1240–1251. doi:10.1111/nph.13643
Hu, L., Xiao, P., Jiang, Y., Dong, M., Chen, Z., Li, H., et al. (2018). Transgenerational Epigenetic Inheritance under Environmental Stress by Genome-wide DNA Methylation Profiling in Cyanobacterium. Front. Microbiol. 9, 1479. doi:10.3389/fmicb.2018.01479
Hu, Y., Peng, X., Wang, F., Chen, P., Zhao, M., and Shen, S. (2021). Natural Population Re-sequencing Detects the Genetic Basis of Local Adaptation to Low Temperature in a Woody Plant. Plant Mol. Biol. 105, 585–599. doi:10.1007/s11103-020-01111-x
Huang, K., Andrew, R. L., Owens, G. L., Ostevik, K. L., and Rieseberg, L. H. (2020). Multiple Chromosomal Inversions Contribute to Adaptive Divergence of a Dune Sunflower Ecotype. Mol. Ecol. 29, 2535–2549. doi:10.1111/mec.15428
Huang, K., and Rieseberg, L. H. (2020). Frequency, Origins, and Evolutionary Role of Chromosomal Inversions in Plants. Front. Plant Sci. 11, 296. doi:10.3389/fpls.2020.00296
Huber, C. D., Degiorgio, M., Hellmann, I., and Nielsen, R. (2016). Detecting Recent Selective Sweeps while Controlling for Mutation Rate and Background Selection. Mol. Ecol. 25, 142–156. doi:10.1111/mec.13351
Ingvarsson, P. K., and Bernhardsson, C. (2020). Genome‐wide Signatures of Environmental Adaptation in European Aspen ( Populus Tremula ) under Current and Future Climate Conditions. Evol. Appl. 13, 132–142. doi:10.1111/eva.12792
Jarquín, D., Crossa, J., Lacaze, X., Du Cheyron, P., Daucourt, J., Lorgeou, J., et al. (2013). A Reaction Norm Model for Genomic Selection Using High-Dimensional Genomic and Environmental Data. Theor. Appl. Genet. 127, 595–607. doi:10.1007/s00122-013-2243-1
Jones, F. C., Chan, Y. F., Schmutz, J., Grimwood, J., Brady, S. D., Southwick, A. M., et al. (2012a). A Genome-wide SNP Genotyping Array Reveals Patterns of Global and Repeated Species-Pair Divergence in Sticklebacks. Curr. Biol. 22, 83–90. doi:10.1016/j.cub.2011.11.045
Jones, F. C., Grabherr, M. G., Grabherr, M. G., Chan, Y. F., Russell, P., Mauceli, E., et al. (2012b). The Genomic Basis of Adaptive Evolution in Threespine Sticklebacks. Nature 484, 55–61. doi:10.1038/nature10944
Kaasik, K., and Chi Lee, C. (2004). Reciprocal Regulation of Haem Biosynthesis and the Circadian Clock in Mammals. Nature 430, 467–471. doi:10.1038/nature02724
Kelleher, C. T., Wilkin, J., Zhuang, J., Cortés, A. J., Quintero, Á. L. P., Gallagher, T. F., et al. (2012). SNP Discovery, Gene Diversity, and Linkage Disequilibrium in Wild Populations of Populus Tremuloides. Tree Genet. Genomes 8, 821–829. doi:10.1007/s11295-012-0467-x
Kruglyak, L. (2008). The Road to Genome-wide Association Studies. Nat. Rev. Genet. 9, 314–318. doi:10.1038/nrg2316
Kwak, M., and Gepts, P. (2009). Structure of Genetic Diversity in the Two Major Gene Pools of Common Bean (Phaseolus vulgaris L., Fabaceae). Theor. Appl. Genet. 118, 979–992. doi:10.1007/s00122-008-0955-4
Lacal, I., and Ventura, R. (2018). Epigenetic Inheritance: Concepts, Mechanisms and Perspectives. Front. Mol. Neurosci. 11, 292. doi:10.3389/fnmol.2018.00292
Lambert, C. G., and Black, L. J. (2012). Learning from Our Gwas Mistakes: From Experimental Design to Scientific Method. Biostatistics 13, 195–203. doi:10.1093/biostatistics/kxr055
Langridge, P., and Waugh, R. (2019). Harnessing the Potential of Germplasm Collections. Nat. Genet. 51, 200–201. doi:10.1038/s41588-018-0340-4
Lasky, J. R., Upadhyaya, H. D., Ramu, P., Deshpande, S., Hash, C. T., Bonnette, J., et al. (2015). Genome-Environment Associations in Sorghum Landraces Predict Adaptive Traits. Sci. Adv. 1, e1400218. doi:10.1126/sciadv.1400218
Lasky, J. R., Des Marais, D. L., Mckay, J. K., Richards, J. H., Juenger, T. E., and Keitt, T. H. (2012). Characterizing Genomic Variation of Arabidopsis Thaliana: the Roles of Geography and Climate. Mol. Ecol. 21, 5512–5529. doi:10.1111/j.1365-294x.2012.05709.x
Lei, L., Poets, A. M., Liu, C., Wyant, S. R., Hoffman, P. J., Carter, C. K., et al. (2019). Environmental Association Identifies Candidates for Tolerance to Low Temperature and Drought. G3 Genes|Genomes|Genetics 9, 3423–3438. doi:10.1534/g3.119.400401
Li, J., Chen, G. B., Rasheed, A., Li, D., Sonder, K., Zavala Espinosa, C., et al. (2019). Identifying Loci with Breeding Potential across Temperate and Tropical Adaptation via Eigengwas and Envgwas. Mol. Ecol. 28, 3544–3560. doi:10.1111/mec.15169
Lopez-Cruz, M., Crossa, J., Bonnett, D., Dreisigacker, S., Poland, J., Jannink, J.-L., et al. (2015). Increased Prediction Accuracy in Wheat Breeding Trials Using a Marker × Environment Interaction Genomic Selection Model. G3 Genes|Genomes|Genetics 5, 569–582. doi:10.1534/g3.114.016097
López-Hernández, F., and Cortés, A. J. (2019). Last-Generation Genome-Environment Associations Reveal the Genetic Basis of Heat Tolerance in Common Bean (Phaseolus vulgaris L.). Front. Genet. 10, 954. doi:10.3389/fgene.2019.00954
López-Hernández, F., and Cortés, A. J. (2022). Whole Transcriptome Sequencing Unveils the Genomic Determinants of Putative Somaclonal Variation in Mint (Mentha L.). Int. J. Mol. Sci. 23, 5291. doi:10.3390/ijms23105291
Manel, S., Andrello, M., Henry, K., Verdelet, D., Darracq, A., Guerin, P.-E., et al. (2018). Predicting Genotype Environmental Range from Genome-Environment Associations. Mol. Ecol. 27, 2823–2833. doi:10.1111/mec.14723
Menamo, T., Kassahun, B., Borrell, A. K., Jordan, D. R., Tao, Y., Hunt, C., et al. (2021). Genetic Diversity of Ethiopian Sorghum Reveals Signatures of Climatic Adaptation. Theor. Appl. Genet. 134, 731–742. doi:10.1007/s00122-020-03727-5
Meyer, R. S., Choi, J. Y., Sanches, M., Plessis, A., Flowers, J. M., Amas, J., et al. (2016). Domestication History and Geographical Adaptation Inferred from a SNP Map of African Rice. Nat. Genet. 48, 1083–1088. doi:10.1038/ng.3633
Migicovsky, Z., and Myles, S. (2017). Exploiting Wild Relatives for Genomics-Assisted Breeding of Perennial Crops. Front. Plant Sci. 8, 460. doi:10.3389/fpls.2017.00460
Miklas, P. N., Kelly, J. D., Beebe, S. E., and Blair, M. W. (2006). Common Bean Breeding for Resistance against Biotic and Abiotic Stresses: From Classical to Mas Breeding. Euphytica 147, 105–131. doi:10.1007/s10681-006-4600-5
Montesinos-LóPez, O. A., Montesinos-LóPez, A., PéRez-RodríGuez, P., BarróN-LóPez, J. A., Martini, J. W. R., Fajardo-Flores, S. B., et al. (2021). A Review of Deep Learning Applications for Genomic Selection. BMC Genomics 22. doi:10.1186/s12864-020-07319-x
Morran, S., Eini, O., Pyvovarenko, T., Parent, B., Singh, R., Ismagul, A., et al. (2011). Improvement of Stress Tolerance of Wheat and Barley by Modulation of Expression of Dreb/Cbf Factors. Plant Biotechnol. J. 9, 230–249. doi:10.1111/j.1467-7652.2010.00547.x
Morton, N. E. (2005). Linkage Disequilibrium Maps and Association Mapping. J. Clin. Investigation 115, 1425–1430. doi:10.1172/jci25032
Muñoz, L. C., Blair, M. W., Duque, M. C., Tohme, J., and Roca, W. (2003). Introgression in Common Bean X Tepary Bean Interspecific Congruity-Backcross Lines as Measured by Aflp Markers. Crop Sci. 44, 637–645.
Nosil, P., and Feder, J. L. (2011). Genomic Divergence during Speciation: Causes and Consequences. Philos. Trans. R. Soc. Lond B Biol. Sci. 367, 332–342. doi:10.1098/rstb.2011.0263
Ortiz, R., Crossa, J., Reslow, F., Perez-Rodriguez, P., and Cuevas, J. (2022). Genome-Based Genotype × Environment Prediction Enhances Potato (Solanum tuberosum L.) Improvement Using Pseudo-diploid and Polysomic Tetraploid Modeling. Front. Plant Sci. 13, 785196. doi:10.3389/fpls.2022.785196
Pluess, A. R., Frank, A., Heiri, C., Lalagüe, H., Vendramin, G. G., and Oddou‐Muratorio, S. (2016). Genome-Environment Association Study Suggests Local Adaptation to Climate at the Regional Scale in Fagus sylvatica. New Phytol. 210, 589–601. doi:10.1111/nph.13809
Price, A. L., Zaitlen, N. A., Reich, D., and Patterson, N. (2010). New Approaches to Population Stratification in Genome-wide Association Studies. Nat. Rev. Genet. 11, 459–463. doi:10.1038/nrg2813
Pyhäjärvi, T., Hufford, M. B., Mezmouk, S., and Ross-Ibarra, J. (2013). Complex Patterns of Local Adaptation in Teosinte. Genome Biol. Evol. 5, 1594–1609. doi:10.1093/gbe/evt109
Ramírez-Villegas, J., Khoury, C., Jarvis, A., Debouck, D. G., and Guarino, L. (2010). A Gap Analysis Methodology for Collecting Crop Genepools: A Case Study with Phaseolus Beans. PLoS One 5, e13497. doi:10.1371/journal.pone.0013497
Ramirez-Villegas, J., Khoury, C. K., Achicanoy, H. A., Diaz, M. V., Mendez, A. C., Sosa, C. C., et al. (2022). State of Ex Situ Conservation of Landrace Groups of 25 Major Crops. Nat. Plants 8, 491–499. doi:10.1038/s41477-022-01144-8
Ramirez-Villegas, J., Khoury, C. K., Achicanoy, H. A., Mendez, A. C., Diaz, M. V., Sosa, C. C., et al. (2020). A Gap Analysis Modelling Framework to Prioritize Collecting for Ex Situ Conservation of Crop Landraces. Divers Distrib. 26, 730–742.
Ratcliffe, B., Thistlethwaite, F., El-Dien, O. G., Cappa, E. P., Porth, I., Klápště, J., et al. (2019). Inter- and Intra-generation Genomic Predictions for Douglas-Fir Growth in Unobserved Environments. bioRxiv. doi:10.1101/540765
Ravinet, M., Faria, R., Butlin, R. K., Galindo, J., Bierne, N., Rafajlović, M., et al. (2017). Interpreting the Genomic Landscape of Speciation: A Road Map for Finding Barriers to Gene Flow. J. Evol. Biol. 30, 1450–1477. doi:10.1111/jeb.13047
Razzaq, A., Kaur, P., Akhter, N., Wani, S. H., and Saleem, F. (2021). Next-Generation Breeding Strategies for Climate-Ready Crops. Front. Plant Sci. 12. doi:10.3389/fpls.2021.620420
Rellstab, C., Gugerli, F., Eckert, A. J., Hancock, A. M., and Holderegger, R. (2015). A Practical Guide to Environmental Association Analysis in Landscape Genomics. Mol. Ecol. 24, 4348–4370. doi:10.1111/mec.13322
Resende, M. D. V., Resende, M. F. R., Sansaloni, C. P., Petroli, C. D., Missiaggia, A. A., Aguiar, A. M., et al. (2012). Genomic Selection for Growth and Wood Quality inEucalyptus:capturing the Missing Heritability and Accelerating Breeding for Complex Traits in Forest Trees. New Phytol. 194, 116–128. doi:10.1111/j.1469-8137.2011.04038.x
Resende, R. T., Piepho, H. P., Rosa, G. J. M., Silva‐Junior, O. B., Silva, F. F. E., Resende, M. D. V. D., et al. (2021). Enviromics in Breeding: Applications and Perspectives on Envirotypic‐Assisted Selection. Theor. Appl. Genet. 134, 95–112. doi:10.1007/s00122-020-03684-z
Roesti, M., Gavrilets, S., Hendry, A. P., Salzburger, W., and Berner, D. (2014). The Genomic Signature of Parallel Adaptation From Shared Genetic Variation. Mol. Ecol. 23, 3944–3956. doi:10.1111/mec.12720
Russell, J., Mascher, M., Dawson, I. K., Kyriakidis, S., Calixto, C., Freund, F., et al. (2016). Exome Sequencing of Geographically Diverse Barley Landraces and Wild Relatives Gives Insights into Environmental Adaptation. Nat. Genet. 48, 1024–1030. doi:10.1038/ng.3612
Schmutz, J., Mcclean, P. E., Mamidi, S., Wu, G. A., Cannon, S. B., Grimwood, J., et al. (2014). A Reference Genome for Common Bean and Genome-wide Analysis of Dual Domestications. Nat. Genet. 46, 707–713. doi:10.1038/ng.3008
Schrider, D. R., and Kern, A. D. (2018). Supervised Machine Learning for Population Genetics: A New Paradigm. Trends Genet. 34, 301–312. doi:10.1016/j.tig.2017.12.005
Slatkin, M. (2008). Linkage Disequilibrium - Understanding the Evolutionary Past and Mapping the Medical Future. Nat. Rev. Genet. 9, 477–485. doi:10.1038/nrg2361
Slotkin, R. K., and Martienssen, R. (2007). Transposable Elements and the Epigenetic Regulation of the Genome. Nat. Rev. Genet. 8, 272–285. doi:10.1038/nrg2072
Smale, M., and Jamora, N. (2020). Valuing Genebanks. Food Sec. 12, 905–918. doi:10.1007/s12571-020-01034-x
Spindel, J. E., Begum, H., Akdemir, D., Collard, B., Redoña, E., Jannink, J.-L., et al. (2016). Genome-Wide Prediction Models that Incorporate De Novo Gwas Are a Powerful New Tool for Tropical Rice Improvement. Heredity 116, 395–408. doi:10.1038/hdy.2015.113
Stenberg, J. A., and Ortiz, R. (2021). Focused Identification of Germplasm Strategy (Figs): Polishing a Rough Diamond. Curr. Opin. Insect Sci. 45, 1–6. doi:10.1016/j.cois.2020.11.001
Tan, B., Grattapaglia, D., Martins, G. S., Ferreira, K. Z., Sundberg, B., and Ingvarsson, P. K. (2017). Evaluating the Accuracy of Genomic Prediction of Growth and Wood Traits in Two Eucalyptus Species and Their F1 Hybrids. BMC Plant Biol. 17, 110. doi:10.1186/s12870-017-1059-6
Tanksley, S. D., and Mccouch, S. R. (1997). Seed Banks and Molecular Maps: Unlocking Genetic Potential from the Wild. Science 277, 1063–1066. doi:10.1126/science.277.5329.1063
Tester, M., and Langridge, P. (2010). Breeding Technologies to Increase Crop Production in a Changing World. Science 327, 818–822. doi:10.1126/science.1183700
Thistlethwaite, F. R., Gamal El-Dien, O., Ratcliffe, B., Klápště, J., Porth, I., Chen, C., et al. (2020). Linkage Disequilibrium vs. Pedigree: Genomic Selection Prediction Accuracy in Conifer Species. PLoS One 15, e0232201. doi:10.1371/journal.pone.0232201
Tiffin, P., and Ross-Ibarra, J. (2014). Advances and Limits of Using Population Genetics to Understand Local Adaptation. Trends Ecol. Evol. 29, 673–680. doi:10.1016/j.tree.2014.10.004
Todesco, M., Owens, G. L., Bercovich, N., Légaré, J. S., Soudi, S., Burge, D. O., et al. (2020). Massive Haplotypes Underlie Ecotypic Differentiation in Sunflowers. Nature 584, 602–607. doi:10.1038/s41586-020-2467-6
Waldvogel, A.-M., Schreiber, D., Pfenninger, M., and Feldmeyer, B. (2020). Climate Change Genomics Calls for Standardized Data Reporting. Front. Ecol. Evol. 8, 242. doi:10.3389/fevo.2020.00242
Wang, H., Cimen, E., Singh, N., and Buckler, E. (2020). Deep Learning for Plant Genomics and Crop Improvement. Curr. Opin. Plant Biol. 54, 34–41. doi:10.1016/j.pbi.2019.12.010
Watson, A., Ghosh, S., Williams, M. J., Cuddy, W. S., Simmonds, J., Rey, M.-D., et al. (2018). Speed Breeding Is a Powerful Tool to Accelerate Crop Research and Breeding. Nat. Plants 4, 23–29. doi:10.1038/s41477-017-0083-8
Wenlong, M., Qiu, Z., Song, J., Li, J., Cheng, Q., Zhai, J., et al. (2018). A Deep Convolutional Neural Network Approach for Predicting Phenotypes From Genotypes. Planta. 248, 1307–1318. doi:10.3390/ijms23105291
Wolf, J. B. W., and Ellegren, H. (2017). Making Sense of Genomic Islands of Differentiation in Light of Speciation. Nat. Rev. Genet. 18 (2), 87–100. doi:10.1038/nrg.2016.133
Wray, N. R., Yang, J., Hayes, B. J., Price, A. L., Goddard, M. E., and Visscher, P. M. (2013). Pitfalls of Predicting Complex Traits from SNPs. Nat. Rev. Genet. 14, 507–515. doi:10.1038/nrg3457
Wu, J., Wang, L., Fu, J., Chen, J., Wei, S., Zhang, S., et al. (2020). Resequencing of 683 Common Bean Genotypes Identifies Yield Component Trait Associations across a North-South Cline. Nat. Genet. 52, 118–125. doi:10.1038/s41588-019-0546-0
Wu, X., Cortés, A. J., and Blair, M. W. (2022). Genetic Differentiation of Grain, Fodder and Pod Vegetable Type Cowpeas (Vigna Unguiculata L.) Identified through Single Nucleotide Polymorphisms from Genotyping-By-Sequencing. Mol. Hortic. 2. doi:10.1186/s43897-022-00028-x
Xiong, H., Shi, A., Mou, B., Qin, J., Motes, D., Lu, W., et al. (2016). Genetic Diversity and Population Structure of Cowpea (Vigna Unguiculata L. Walp). PLoS One 11, e0160941. doi:10.1371/journal.pone.0160941
Yoder, J. B., Stanton-Geddes, J., Zhou, P., Briskine, R., Young, N. D., and Tiffin, P. (2014). Genomic Signature of Adaptation to Climate in Medicago truncatula. Genetics 196, 1263–1275. doi:10.1534/genetics.113.159319
Zahn, L. M., and Purnell, B. A. (2016). Genes under Pressure. Science 354, 52. doi:10.1126/science.354.6308.52
Zellweger, F., De Frenne, P., Lenoir, J., Rocchini, D., and Coomes, D. (2019). Advances in Microclimate Ecology Arising from Remote Sensing. Trends Ecol. Evol. 34, 327–341. doi:10.1016/j.tree.2018.12.012
Zhang, X., Sun, Y., Landis, J. B., Zhang, J., Yang, L., Lin, N., et al. (2020). Genomic Insights into Adaptation to Heterogeneous Environments for the Ancient Relictual Circaeaster agrestis (Circaeasteraceae, Ranunculales). New Phytol. 228, 285–301. doi:10.1111/nph.16669
Keywords: gene banks, germplasm collections, landraces, genome–environment associations (GEA), genome-wide environmental scans, genome-wide selection scans (GWSS), genomic prediction (GP), abiotic stress
Citation: Cortés AJ, López-Hernández F and Blair MW (2022) Genome–Environment Associations, an Innovative Tool for Studying Heritable Evolutionary Adaptation in Orphan Crops and Wild Relatives. Front. Genet. 13:910386. doi: 10.3389/fgene.2022.910386
Received: 01 April 2022; Accepted: 30 May 2022;
Published: 05 August 2022.
Edited by:
Petr Smýkal, Palacký University, Olomouc, CzechiaReviewed by:
Michael Benjamin Kantar, University of Hawaii, United StatesCopyright © 2022 Cortés, López-Hernández and Blair. This is an open-access article distributed under the terms of the Creative Commons Attribution License (CC BY). The use, distribution or reproduction in other forums is permitted, provided the original author(s) and the copyright owner(s) are credited and that the original publication in this journal is cited, in accordance with accepted academic practice. No use, distribution or reproduction is permitted which does not comply with these terms.
*Correspondence: Andrés J. Cortés, YWNvcnRlc0BhZ3Jvc2F2aWEuY28=; Matthew W. Blair, bWJsYWlyQHRuc3RhdGUuZWR1
†Secondary address:Andrés J. Cortés, Facultad de Ciencias Agrarias – Departamento de Ciencias Forestales, Universidad Nacional de Colombia – Sede Medellín, Medellín, Colombia
Disclaimer: All claims expressed in this article are solely those of the authors and do not necessarily represent those of their affiliated organizations, or those of the publisher, the editors and the reviewers. Any product that may be evaluated in this article or claim that may be made by its manufacturer is not guaranteed or endorsed by the publisher.
Research integrity at Frontiers
Learn more about the work of our research integrity team to safeguard the quality of each article we publish.