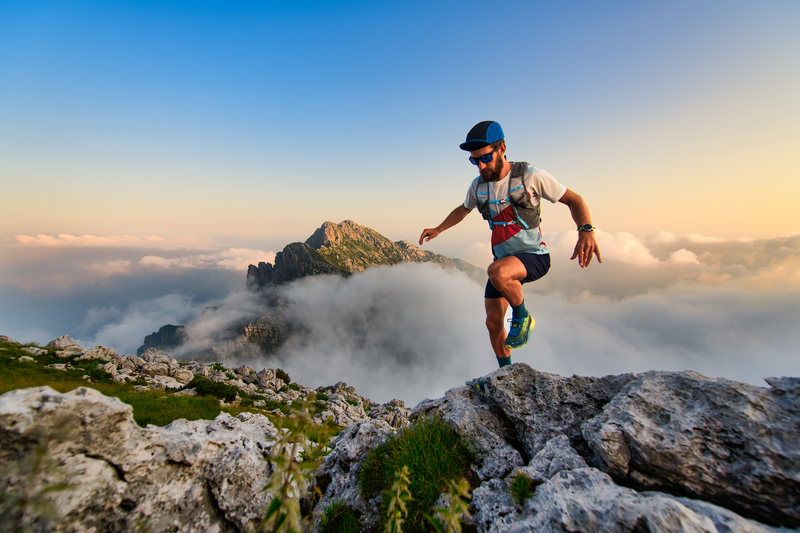
95% of researchers rate our articles as excellent or good
Learn more about the work of our research integrity team to safeguard the quality of each article we publish.
Find out more
ORIGINAL RESEARCH article
Front. Genet. , 08 June 2022
Sec. Cancer Genetics and Oncogenomics
Volume 13 - 2022 | https://doi.org/10.3389/fgene.2022.907392
This article is part of the Research Topic Epigenetic Regulation and Non-histone Post-translational Modification in Cancer View all 15 articles
Purpose: The prognosis of head and neck squamous cell carcinoma (HNSCC) is poor. Necroptosis is a novel programmed form of necrotic cell death. The prognostic value of necroptosis-associated lncRNAs expression in HNSCC has not been explored.
Methods: We downloaded mRNA expression data of HNSCC patients from TCGA databases. Prognostic lncRNAs were identified by univariate Cox regression. LASSO was used to establish a model with necroptosis-related lncRNAs. Kaplan-Meier analysis and ROC were applied to verify the model. Finally, functional studies including gene set enrichment analyses, immune microenvironment analysis, and anti-tumor compound IC50 prediction were performed.
Results: We identified 1,117 necroptosis-related lncRNAs. The Cox regression showed 55 lncRNAs were associated with patient survival (p < 0.05). The risk model of 24- lncRNAs signature categorized patients into high and low risk groups. The patients in the low-risk group survived longer than the high-risk group (p < 0.001). Validation assays including ROC curve, nomogram and correction curves confirmed the prediction capability of the 24-lncRNA risk mode. Functional studies showed the two patient groups had distinct immunity conditions and IC50.
Conclusion: The 24-lncRNA model has potential to guide treatment of HNSCC. Future clinical studies are needed to verify the model.
Head and neck squamous cell carcinomas (HNSCCs) arise from squamous cells in the oral cavity, pharynx and larynx. The most common risk factors for HNSCC include alcohol drinking, smoking and HPV infection (Fakhry et al., 2008). Athough HNSCC can be treated with surgery, radiotherapy and chemotherapy, patients with HNSCCs still suffer from poor survival. To improve patient survival, novel therapeutic targets and effective prognostic tools are needed.
Necroptosis is another mode of regulated cell death mimicking apoptosis and necrosis. Necroptosis is associated with a range of pathological conditions and diseases, including cancer. It is mediated by Fas, TNF, LPS, and death receptors (Vanden Berghe et al., 2014). Binding of ligands and receptors activates RIP3, which phosphorylates MLKL (Sun et al., 1999). Phosphorylated MLKL then translocates to and ruptures cellular membranes, leading to cell swelling and release of intracellular components (Dondelinger et al., 2014; Hildebrand et al., 2014; Wang et al., 2014).
A plethora of evidence shows necroptosis of tumor cells is often associated with tumor aggressiveness and metastasis. RIP3, a molecular marker of necroptosis, is an independent factor associated with survival in breast cancer (Koo et al., 2015). RIP3 expression was also decreased in colorectal cancer and was an independent prognostic factor of survival (Feng et al., 2015). In acute myeloid leukemia, RIP3 expression was reduced in most samples and overexpression of RIP3 in DA1-3b leukemia cells induced necroptosis (Nugues et al., 2014). Li et al. reported that necroptosis was associated with survival of HNSCC patients (Li et al., 2020).
Long non-coding RNAs (lncRNAs) regulate gene expression and are involved in tumorogenesis (Kumar and Goyal, 2017; Peng et al., 2017). Specially, Jiang et al. reported dysregulation of lncRNAs was involved in HNSCC(Jiang et al., 2019). Although necroptosis plays an important role in patient survival of a variety of tumors, the role of necroptosis-related lncRNAs in HNSCC has not been reported.
We thus explored the potential roles of different necroptosis-related lncRNAs on the survival of HNSCC patients. We developed a novel risk-score model with necroptosis-related lncRNAs according to their expression levels. The results might further our understanding of necroptosis in HNSCC.
The Cancer Genome Atlas (TCGA) (https://portal.gdc.cancer.gov/repository) has transcriptomic data of more than 20,000 cancer and normal samples. In the present study RNA sequencing (RNA-seq) data of tumor tissues of 487 HNSCC patients and 42 matched normal tissues was downloaded from TCGA database. Our study was conducted by reviewing public database and ethical approval was not required.
The expression data of 67 necroptosis-associated genes was used for analysis (Supplementary Table S1). Correlation analysis was performed among 67 necroptosis-related genes and differentially expressed lncRNAs in the combined matrices. 1,117 lncRNAs with Pearson correlation coeffi-cients >0.4 and p < 0.001 were identified to be necroptosis-related lncRNAs.
The clinical data of HNSCC patients was downloaded from TCGA data portal. The univariate Cox proportional hazard regression analysis was used to screen prognostic genes. Least absolute shrinkage and selection operator (Lasso) regression was conducted with 10-fold cross-validation and a p value of 0.05. After identification of the prognostic lncRNAs, the risk scores were determined as follow (X: coefficients, Y: expression level of lncRNAs):
HNSCC patients were allocated into either low- or high-risk groups according to the median risk score (Meng et al., 2019; Hong et al., 2020). The Chi-square test was used to determine the prognostic significance value of the risk model, and overall survival (OS) time was compared between the two groups via Kaplan-Meier analysis. The “survival”, “survminer” and “timeROC” R packages were used to plot the 1-, 3-, and 5-years receiver operating characteristics (ROC) curves. The risk scores were also evaluated as an independent risk factor with other clinical parameters by Cox regression with rms R package. Then a nomogram for prediction of the 1-, 3-, and 5-years OS was set up using risk score and clinical parameters. The ROC, calibration curves and Hosmer-Lemeshow test of the nomogram were assessed in the validation set.
To explore the biological pathways that might be responsible for poor patient survival, we employed R (Bioconductor package gsea) to perform gene set enrichment analyses (GSEA). Potential biological mechanisms of the prognostic model were also explored. KEGG gene sets in the GSEA database were downloaded. We chose gene sets with a FDR value < 0.05 and a FDR <0.25.
CIBERSORT, EPIC, MCPcounter, QUANTISEQ, TIMER, and XCELL were used to evaluate cells in the tumor microenvironment (TME) (http://timer.cistrome.org/). ggplot2, ggtext, limma, and scales R packages and Wilcoxon signed-rank test were performed to analyze cell types in TME (Hong et al., 2020). TME scores and immune checkpoint were compared between the two groups with ggpubr R package.
To predict therapeutic response, the R package pRRophetic was utilized to measure the half-maximal inhibitory concentrations (IC50) of each HNSCC sample on Genomics of Drug Sensitivity in Cancer (GDSC) (https://www.cancerrxgene.org/) (Geeleher et al., 2014).
Figure 1 shows the flow diagram depicting the present study. We compared the expression levels of 67 necroptosis-associated genes (Supplementary Table S1) between 42 healthy samples and 487 HNSCC cancer samples from the TCGA data and identified 14,086 lncRNAs. Among these lncRNAs, 1,117 lncRNAs met the criteria (Pearson correlation coefficients >0.4 and p values <0.001) (Figure 2A). We identified 717 differentially expressed necroptosis-related lncRNAs (|Log2FC| > 1 and p < 0.05) (Figure 2B); 697 were upregulated and 20 were downregulated. Univariate Cox regression showed 55 lncRNAs were significantly correlated with OS (p < 0.05 for all) (Figures 2C,D).
FIGURE 2. Necroptosis-related lncRNA prognostic signature identified in HNSCC. (A) Correlation network of necroptosis-related genes and necroptosis-related lncRNAs. (B) The volcano plot of 717 lncRNAs. Red dots represented upregulated lncRNAs and black dots represented down-regulated lncRNAs. (C,D) The expression and univariate Cox regression of 55 prognostic lncRNAs. *p < 0.05; **p < 0.01; ***p < 0.001.
To avoid overfitting and to quantify the impact of necroptosis-related lncRNAs on the prognosis of each HNSCC patient, we constructed a 24 lncRNAs prognostic signature by LASSO regression analysis (Figures 3A,B). All 24 lncRNAs positively regulated necroptosis genes in the Sankey diagram (Figure 3C).
FIGURE 3. Construction of prognostic signature in HNSCC. (A) LASSO with 10-fold cross-validation. (B) Coefficient profile plots. (C) The Sankey diagram shows the connection degree between the 24 prognostic lncRNAs and the necroptosis-related genes.
We established the following formula to calculate the risk score of every HNSCC patient.
Risk score = AC008764.8×(-0.2393)+AC104083.1×(-0.0743)+AC127521.1×(-0.9739)+LINC00539×(-1.2625)+AC243829.2×(-0.4868)+AL121845.4×(-0.2809)+AC020911.1×(-0.6943)+AC007347.1×(0.4674)+ALMS1-IT1×(0.6387)+PLS3-AS1×(0.3155)+ZNF197-AS1×(0.4949)+LINC00942×(0.0017)+AC068790.2×(0.3482)+AC139256.3×(-0.9441)+LINC00861×(-0.8428)+WDFY3-AS2×(1.1448)+AC116025.2×(1.0996)+AC007128.1×(0.0713)+RAB11B-AS1×(-0.0588)+AC009121.3× (-1.6935)+POLH-AS1×(0.4292)+AC021016.1×(-0.0412)+CDKN2A-DT×(-0.2129)+PCED1B-AS1×(0.1493) (Meng et al., 2019).
In the training set, test set and entire set, the distribution of risk scores and survival times were compared between the high-risk group and the low-risk group (Figures 4A–C). More patients died in the high-risk group (Figures 4D–F). The heat maps of 24 lncRNAs are shown in Figures 4G–I. Survival curves show the high-risk groups of the three sets had poor prognoses (Figures 4J–L). Besides, some typical clinicopathologic parameters were identified to be prognostic factors (Figure 4M).
FIGURE 4. The prognosis analysis of the three sets (training set: A, D, G, J; test set: B, E, H, K; entire set: C, F, I, L). (A–C) Risk scores of the high-risk and low-risk groups in the respective three sets. (D–F) Comparison of survival between the two groups in the respective three sets. (G–I) The heat maps of 24 lncRNAs. (J–L) Kaplan–Meier survival curves of patients in the two groups in the respective three sets. (M) Kaplan–Meier survival curves of OS stratified by clinicopathologic parameters between the two groups.
In both the training set and the test set, risk score, age and tumor stage were identified to be independent prognostic factors. The hazard ratios (HR) of these factors are shown in Figures 5A–D). A nomogram was established to predict the 1-, 3-, and 5-years OS (Figure 5E). The predicted survival showed close agreement with observed actual survival (Figure 5F).
FIGURE 5. Nomogram of the model. (A,B) Tumor stage and risk score were risk factors in the training set. (C,D) Only risk score was risk factor in the test sets. (E) The nomogram that integrated the risk score, age, and tumor stage to predict OS. (F) The calibration curves for OS. (G–I) ROC curves of the three sets (training set: A, C, G; test set: B, D, H; entire set: I).
The ROC curves showed the sensitivity and specificity of the model were high (Figures 5G–I).
GSEA results showed that nine of top ten pathways were involved in carcinogenesis. For example, pentose and glucuronate interconversions, aldarate metabolism, and starch and sucrose metabolism were significantly enriched in the high-risk group. On the other hand, eight pathways enriched in the low-risk group were related to immunity (p < 0.05; FDR <0.25; |NES| > 1.9), such as T cell receptor signaling pathway and natural killer (NK) cell-mediated cytotoxicity (Figure 6A; Supplementary Table S2). Therefore, the low-risk group had a favorable TME. On the contrary, the high-risk group had an unfavorable TME.
FIGURE 6. TME and immunotherapy. (A) Top 10 pathways identified by GSEA (B)The heat maps of immune cells. (C) Risk scores were corrected with immune cells. (D) ssGSEA scores of immune functions. (E) Comparison of immune-related scores between the two groups. (F) The expression of 34 checkpoints. (G)Twelve targeted and immunotherapeutic drugs with different IC50 between the low-risk group (green) and the high-risk group (red).
Single sample GSEA (ssGSEA) was performed to calculate numbers for different types of immune cells. Tumors of the low-risk group were infiltrated by more immune cells as exhibited in the heatmap (p < 0.05 for all) (Figure 6B; Supplementary Table S3). Correlations between risk scores and activities of immune cell types are shown in Figure 6C. All of the 13 immune-related pathways had higher activity in the low-risk group (Figure 6D).
ESTIMATE was used to generate immune scores and stromal scores. Figure 6E shows both immune scores and stromal scores (microenvironment) were higher in the low-risk group. Besides, the immune checkpoint expression was lower in the low-risk group (Figure 6F).
Finally, we found IC50 of the anti-tumor compounds, such as AKT inhibitors, JNK inhibitor and sunitinib, was usually lower in the low-risk group (Figure 6G).
The human genome produces a large amount of RNA transcripts that do not encode for proteins (Djebali et al., 2012). lncRNAs are among those transcripts. They are usually longer than 200 nucleotides and have many functions, including regulating cancer development (Huarte, 2015; Marchese et al., 2017; Mattick, 2018).
Necroptosis is mediated by RIP1 and RIP3 (Chan and Baehrecke, 2012; Pasparakis and Vandenabeele, 2015). RIP1 phosphorylates RIP3, which phosphorylates MLKL. In necrosomes phosphorylation of MLKL leads to MLKL oligomerization. Oligomerized MLKL causes cell death by breaking down cell membranes (Sun et al., 2012; Guicciardi et al., 2013). Although necroptosis may cause cancer cell death, cell death may inhibit immune response (Pasparakis and Vandenabeele, 2015; Wang et al., 2017). Necroptosis may elicit necrosis-associated inflammation. Inflammation could contribute to progression of cancer and promote resistance to anticancer treatments. In addition necroptosis may also fail to elicit strong immunogenic reactions.
Massively parallel RNA sequencing has identified large amounts of novel lncRNAs. However, functional annotation of lncRNAs is lagging behind. In the present study, we explored the prognostic values of necroptosis-related lncRNAs in HNSCC. We found that several necroptosis-related lncRNAs were closely related to HNSCC prognosis. More specifically, AC007347.1, ALMS1-IT1, PLS3-AS1, ZNF197-AS1, AC068790.2, WDFY3-AS2, AC116025.2, POLH-AS1, and PCED1B-AS1 were risk factors. On the other hand, AC008764.8, AC127521.1, LINC00539, AC243829.2, AL121845.4, AC020911.1, AC139256.3, LINC00861, AC009121.3 and CDKN2A-DT were protective factors for HNSCC patients.
Further analysis showed that AC007347.1, ALMS1-IT1, PLS3-AS1, ZNF197-AS1, AC068790.2, WDFY3-AS2, AC116025.2, POLH-AS1, and PCED1B-AS1 are positive regulators of BRAF, SIRT1, FLT3, FASLG, TRAF2, ATRX, TERT, SPATA2, and TNFRSF1B. BRAF is a proto-oncogene that encodes for the B-Raf protein, a kinase of the RAF protein family (Rebocho and Marais, 2013). The Ras/Raf/MAPK pathway regulates cell growth, differentiation, cell motility and apoptosis (Rebocho and Marais, 2013; Schettini et al., 2018). Abnormal activation of the pathway is responsible for many tumors (Bouchè et al., 2021).
SIRT1 is a member of the HDAC family. Aberrant SIRT1 expression has been found in many tumors (Bradbury et al., 2005; Hida et al., 2007; Stünkel et al., 2007; Chen et al., 2014). ATRX is a member of the SWI-SNF protein family (Stayton et al., 1994; Picketts et al., 1996; Argentaro et al., 2007). SWI-SNF proteins are involved in DNA recombination and repair (Picketts et al., 1996), which are crucial for both development and cancer (Watson et al., 2015). SPATA2 is a TNF receptor modulator. TNF-α pathway modulates immune responses (Swann et al., 2008). TNF-α and IL-1β induced SPATA2 expression in ovarian cancer cells and that increased SPATA2 expression was associated with poor prognosis of ovarian cancer patients (Wieser et al., 2019). Our study suggested SPATA2 expression is also associated with poor prognosis of HNSCC patients. ZBP1 is expressed in many tissues (Fu et al., 1999; Rothenburg et al., 2002) and is a interferon stimulated gene (Fu et al., 1999; Kuriakose and Kanneganti, 2018). ZBP1 expression in tumors is elevated. ZBP1 deletion blocks tumor necroptosis during tumor development and inhibits tumor metastasis (Baik et al., 2021). TNF-α is a pro-inflammatory cytokine mainly secreted by macrophages. There are two receptors for TNF-α, i.e., TNFRSF1A and TNFRSF1B. Although TNF can kill tumor cells, it also contribute to tumorigenesis (Aggarwal, 2003).
On the other hand, AC008764.8, AC127521.1, LINC00539, AC243829.2, AL121845.4, AC020911.1, AC139256.3, LINC00861, AC009121.3 and CDKN2A-DT were protective factors for HNSCC patients. Further analysis showed these lncRNAs were positive regulators of p16INK4a, SPATA2, FLT3, FASLG, TRAF2, ATRX, TERT, BRAF, SIRT1, TNFRSF1B, and BCL2L11. p16INK4a is a tumor suppressor protein encoded by CDKN2A (Witcher and Emerson, 2009). p16INK4a is a negative regulator of cell cycle (Serrano et al., 1993). CDKN2A also encodes for another tumor suppressor protein, which interacts with p53 (Pomerantz et al., 1998). Inactivation of p16INK4a has been observed in various cancers via various mechanisms (Zhao et al., 2016). FLT3 is a receptor tyrosine kinase that is expressed in hematopoietic cells. Activation of FLT3 leads to autophosphorylation and mediates proliferation and differentiation of hematopoietic progenitor cells. However its role in tumorogenesis has not been reported. FASLG is a tumor suppressor and a member of the tumor necrosis factor superfamily (Magerus et al., 2021). FASLG/FAS signaling could induce apoptosis in various cancers (Liu et al., 2009; Kadam and Abhang, 2016; Magerus et al., 2021). TRAFs are intracellular adaptor signaling molecules of immune cells (Rothe et al., 1995; Ye et al., 2002; Park, 2018). TRAF2 promotes p53-dependent apoptosis by activating the JNK signaling cascade in cancer cells (Tsuchida et al., 2020). BCL2L11 is a member of BCL-2 family and regulates function of mitochondria (Concannon et al., 2010; Kilbride et al., 2010). BCL2L11 deletion/downregulation is found in many neoplasms and contribute to acquired drug resistance (Zhang et al., 2016).
By our model, we found pathways such as TNF, RAF and BCL-2 and FASLG/FAS are closely related to HNSCC. Although the protective lncRNAs are positive regulators of several tumor suppressors, they are also associated with several oncogenes. We propose that the prognostic value of a specific lncRNA is determined by the net effect of its multiple target genes.
Tumors have been described as “hot” or “cold” according to infiltration degree by T cells rushing to fight the cancerous cells. Hot tumors typically respond well to immunotherapy treatment using checkpoint inhibitors. Checkpoint inhibitors block signalling through checkpoint receptors to prevent the loss of T cell response to tumors. In contrast, nonimmunogenic “cold” tumors have not yet been infiltrated with T cells. The lack of T cells makes it difficult to provoke an immune response with immunotherapy drugs. IN addition, the microenvironment surrounding cold tumors contains myeloid-derived suppressor cells and T regulatory cells, which are known to dampen the immune response. In our model the patients in the high-risk group were more likely to have cold tumors, which may partially explain why the patients in the high-risk group had poor prognosis.
There were some limitations of our model. As a retrospective study, inherent biases might affect the model. We had performed internal validation by the test set, but we did not perform external validation.
In conclusion, we established a novel necroptosis-associated lncRNA signature for the prognosis of HNSCC. The established signatures suggest that lncRNAs might be associated with responses to targeted therapy and immunotherapy of HNSCC. The potential of this signature in predicting patient survival and treatment responses need to be validated in future tests.
The original contributions presented in the study are included in the article/Supplementary Material, further inquiries can be directed to the corresponding authors.
JH, RL, YW, and LL contributed to conception and design of the study. JH organized the database. YW performed the statistical analysis. JH wrote the first draft of the manuscript. RL, DZ, YW, and LL wrote sections of the manuscript. All authors contributed to manuscript revision, read, and approved the submitted version.
This study was funded by the Fujian Provincial Health Technology Project (Grant number:2021CXA029), the Norman Bethune Medical Science Research Fund (Grant number: B19006CS), the Natural Science Foundation of Fujian Province (Grant number: 2019J01462) and Startup Fund for Scientific Research, Fujian Medical University (Grant number:2018QH1226).
The authors declare that the research was conducted in the absence of any commercial or financial relationships that could be construed as a potential conflict of interest.
All claims expressed in this article are solely those of the authors and do not necessarily represent those of their affiliated organizations, or those of the publisher, the editors and the reviewers. Any product that may be evaluated in this article, or claim that may be made by its manufacturer, is not guaranteed or endorsed by the publisher.
The results shown here are in whole based upon data generated by the TCGA Research Network: https://www.cancer.gov/tcga.
The Supplementary Material for this article can be found online at: https://www.frontiersin.org/articles/10.3389/fgene.2022.907392/full#supplementary-material
Aggarwal, B. B. (2003). Signalling Pathways of the TNF Superfamily: a Double-Edged Sword. Nat. Rev. Immunol. 3 (9), 745–756. doi:10.1038/nri1184
Argentaro, A., Yang, J.-C., Chapman, L., Kowalczyk, M. S., Gibbons, R. J., Higgs, D. R., et al. (2007). Structural Consequences of Disease-Causing Mutations in the ATRX-DNMT3-DNMT3L (ADD) Domain of the Chromatin-Associated Protein ATRX. Proc. Natl. Acad. Sci. U.S.A. 104 (29), 11939–11944. doi:10.1073/pnas.0704057104
Baik, J. Y., Liu, Z., Jiao, D., Kwon, H.-J., Yan, J., Kadigamuwa, C., et al. (2021). ZBP1 Not RIPK1 Mediates Tumor Necroptosis in Breast Cancer. Nat. Commun. 12 (1), 2666. doi:10.1038/s41467-021-23004-3
Berghe, T. V., Linkermann, A., Jouan-Lanhouet, S., Walczak, H., and Vandenabeele, P. (2014). Regulated Necrosis: the Expanding Network of Non-apoptotic Cell Death Pathways. Nat. Rev. Mol. Cell Biol. 15 (2), 135–147. doi:10.1038/nrm3737
Bouchè, V., Aldegheri, G., Donofrio, C. A., Fioravanti, A., Roberts-Thomson, S., Fox, S. B., et al. (2021). BRAF Signaling Inhibition in Glioblastoma: Which Clinical Perspectives? Front. Oncol. 11, 772052. doi:10.3389/fonc.2021.772052
Bradbury, C. A., Khanim, F. L., Hayden, R., Bunce, C. M., White, D. A., Drayson, M. T., et al. (2005). Histone Deacetylases in Acute Myeloid Leukaemia Show a Distinctive Pattern of Expression that Changes Selectively in Response to Deacetylase Inhibitors. Leukemia 19 (10), 1751–1759. doi:10.1038/sj.leu.2403910
Chan, F. K.-M., and Baehrecke, E. H. (2012). RIP3 Finds Partners in Crime. Cell 148 (1-2), 17–18. doi:10.1016/j.cell.2011.12.020
Chen, X., Hokka, D., Maniwa, Y., Ohbayashi, C., Itoh, T., and Hayashi, Y. (2014). Sirt1 Is a Tumor Promoter in Lung Adenocarcinoma. Oncol. Lett. 8 (1), 387–393. doi:10.3892/ol.2014.2057
Concannon, C. G., Tuffy, L. P., Weisová, P., Bonner, H. P., Dávila, D., Bonner, C., et al. (2010). AMP Kinase-Mediated Activation of the BH3-Only Protein Bim Couples Energy Depletion to Stress-Induced Apoptosis. J. Cell Biol. 189 (1), 83–94. doi:10.1083/jcb.200909166
Djebali, S., Davis, C. A., Merkel, A., Dobin, A., Lassmann, T., Mortazavi, A., et al. (2012). Landscape of Transcription in Human Cells. Nature 489 (7414), 101–108. doi:10.1038/nature11233
Dondelinger, Y., Declercq, W., Montessuit, S., Roelandt, R., Goncalves, A., Bruggeman, I., et al. (2014). MLKL Compromises Plasma Membrane Integrity by Binding to Phosphatidylinositol Phosphates. Cell Rep. 7 (4), 971–981. doi:10.1016/j.celrep.2014.04.026
Fakhry, C., Westra, W. H., Li, S., Cmelak, A., Ridge, J. A., Pinto, H., et al. (2008). Improved Survival of Patients with Human Papillomavirus-Positive Head and Neck Squamous Cell Carcinoma in a Prospective Clinical Trial. JNCI J. Natl. Cancer Inst. 100 (4), 261–269. doi:10.1093/jnci/djn011
Feng, X., Song, Q., Yu, A., Tang, H., Peng, Z., and Wang, X. (2015). Receptor-interacting Protein Kinase 3 Is a Predictor of Survival and Plays a Tumor Suppressive Role in Colorectal Cancer. neo 62 (4), 592–601. doi:10.4149/neo_2015_071
Fu, Y., Comella, N., Tognazzi, K., Brown, L. F., Dvorak, H. F., and Kocher, O. (1999). Cloning of DLM-1, a Novel Gene that Is Up-Regulated in Activated Macrophages, Using RNA Differential Display. Gene 240 (1), 157–163. doi:10.1016/s0378-1119(99)00419-9
Geeleher, P., Cox, N. J., and Huang, R. (2014). Clinical Drug Response Can Be Predicted Using Baseline Gene Expression Levels and In Vitro Drug Sensitivity in Cell Lines. Genome Biol. 15 (3), R47. doi:10.1186/gb-2014-15-3-r47
Guicciardi, M. E., Malhi, H., Mott, J. L., and Gores, G. J. (2013). Apoptosis and Necrosis in the Liver. Compr. Physiol. 3 (2), 977–1010. doi:10.1002/cphy.c120020
Hida, Y., Kubo, Y., Murao, K., and Arase, S. (2007). Strong Expression of a Longevity-Related Protein, SIRT1, in Bowen's Disease. Arch. Dermatol Res. 299 (2), 103–106. doi:10.1007/s00403-006-0725-6
Hildebrand, J. M., Tanzer, M. C., Lucet, I. S., Young, S. N., Spall, S. K., Sharma, P., et al. (2014). Activation of the Pseudokinase MLKL Unleashes the Four-Helix Bundle Domain to Induce Membrane Localization and Necroptotic Cell Death. Proc. Natl. Acad. Sci. U.S.A. 111 (42), 15072–15077. doi:10.1073/pnas.1408987111
Hong, W., Liang, L., Gu, Y., Qi, Z., Qiu, H., Yang, X., et al. (2020). Immune-Related lncRNA to Construct Novel Signature and Predict the Immune Landscape of Human Hepatocellular Carcinoma. Mol. Ther. - Nucleic Acids 22, 937–947. doi:10.1016/j.omtn.2020.10.002
Huarte, M. (2015). The Emerging Role of lncRNAs in Cancer. Nat. Med. 21 (11), 1253–1261. doi:10.1038/nm.3981
Jiang, Y., Cao, W., Wu, K., Qin, X., Wang, X., Li, Y., et al. (2019). LncRNA LINC00460 Promotes EMT in Head and Neck Squamous Cell Carcinoma by Facilitating Peroxiredoxin-1 into the Nucleus. J. Exp. Clin. Cancer Res. 38 (1), 365. doi:10.1186/s13046-019-1364-z
Kadam, C. Y., and Abhang, S. A. (2016). Apoptosis Markers in Breast Cancer Therapy. Adv. Clin. Chem. 74, 143–193. doi:10.1016/bs.acc.2015.12.003
Kilbride, S. M., Farrelly, A. M., Bonner, C., Ward, M. W., Nyhan, K. C., Concannon, C. G., et al. (2010). AMP-activated Protein Kinase Mediates Apoptosis in Response to Bioenergetic Stress through Activation of the Pro-apoptotic Bcl-2 Homology Domain-3-Only Protein BMF. J. Biol. Chem. 285 (46), 36199–36206. doi:10.1074/jbc.M110.138107
Koo, G.-B., Morgan, M. J., Lee, D.-G., Kim, W.-J., Yoon, J.-H., Koo, J. S., et al. (2015). Methylation-dependent Loss of RIP3 Expression in Cancer Represses Programmed Necrosis in Response to Chemotherapeutics. Cell Res. 25 (6), 707–725. doi:10.1038/cr.2015.56
Kumar, M. M., and Goyal, R. (2017). LncRNA as a Therapeutic Target for Angiogenesis. Ctmc 17 (15), 1750–1757. doi:10.2174/1568026617666161116144744
Kuriakose, T., and Kanneganti, T.-D. (2018). ZBP1: Innate Sensor Regulating Cell Death and Inflammation. Trends Immunol. 39 (2), 123–134. doi:10.1016/j.it.2017.11.002
Li, J., Huang, S., Zeng, L., Li, K., Yang, L., Gao, S., et al. (2020). Necroptosis in Head and Neck Squamous Cell Carcinoma: Characterization of Clinicopathological Relevance and In Vitro Cell Model. Cell Death Dis. 11 (5), 391. doi:10.1038/s41419-020-2538-5
Liu, Y., Wen, Q.-J., Yin, Y., Lu, X.-T., Pu, S.-H., Tian, H.-P., et al. (2009). FASLG Polymorphism Is Associated with Cancer Risk. Eur. J. Cancer 45 (14), 2574–2578. doi:10.1016/j.ejca.2009.04.001
Magerus, A., Bercher-Brayer, C., and Rieux-Laucat, F. (2021). The Genetic Landscape of the FAS Pathway Deficiencies. Biomed. J. 44 (4), 388–399. doi:10.1016/j.bj.2021.06.005
Marchese, F. P., Raimondi, I., and Huarte, M. (2017). The Multidimensional Mechanisms of Long Noncoding RNA Function. Genome Biol. 18 (1), 206. doi:10.1186/s13059-017-1348-2
Mattick, J. S. (2018). The State of Long Non-coding RNA Biology. ncRNA 4 (3), 17. doi:10.3390/ncrna4030017
Meng, T., Huang, R., Zeng, Z., Huang, Z., Yin, H., Jiao, C., et al. (2019). Identification of Prognostic and Metastatic Alternative Splicing Signatures in Kidney Renal Clear Cell Carcinoma. Front. Bioeng. Biotechnol. 7, 270. doi:10.3389/fbioe.2019.00270
Nugues, A.-L., El Bouazzati, H., Hétuin, D., Berthon, C., Loyens, A., Bertrand, E., et al. (2014). RIP3 Is Downregulated in Human Myeloid Leukemia Cells and Modulates Apoptosis and Caspase-Mediated p65/RelA Cleavage. Cell Death Dis. 5 (8), e1384. doi:10.1038/cddis.2014.347
Park, H. H. (2018). Structure of TRAF Family: Current Understanding of Receptor Recognition. Front. Immunol. 9, 1999. doi:10.3389/fimmu.2018.01999
Pasparakis, M., and Vandenabeele, P. (2015). Necroptosis and its Role in Inflammation. Nature 517 (7534), 311–320. doi:10.1038/nature14191
Peng, W.-X., Koirala, P., and Mo, Y.-Y. (2017). LncRNA-mediated Regulation of Cell Signaling in Cancer. Oncogene 36 (41), 5661–5667. doi:10.1038/onc.2017.184
Picketts, D., Higgs, D. R., Bachoo, S., Blake, D. J., Quarrell, O. W., and Gibbons, R. J. (1996). ATRX Encodes a Novel Member of the SNF2 Family of Proteins: Mutations Point to a Common Mechanism Underlying the ATR-X Syndrome. Hum. Mol. Genet. 5 (12), 1899–1907. doi:10.1093/hmg/5.12.1899
Pomerantz, J., Schreiber-Agus, N., Liégeois, N. J., Silverman, A., Alland, L., Chin, L., et al. (1998). The Ink4a Tumor Suppressor Gene Product, p19Arf, Interacts with MDM2 and Neutralizes MDM2's Inhibition of P53. Cell 92 (6), 713–723. doi:10.1016/s0092-8674(00)81400-2
Rebocho, A. P., and Marais, R. (2013). ARAF Acts as a Scaffold to Stabilize BRAF:CRAF Heterodimers. Oncogene 32 (26), 3207–3212. doi:10.1038/onc.2012.330
Rothe, M., Sarma, V., Dixit, V. M., and Goeddel, D. V. (1995). TRAF2-Mediated Activation of NF-Κb by TNF Receptor 2 and CD40. Science 269 (5229), 1424–1427. doi:10.1126/science.7544915
Rothenburg, S., Schwartz, T., Koch-Nolte, F., and Haag, F. (2002). Complex Regulation of the Human Gene for the Z-DNA Binding Protein DLM-1. Nucleic Acids Res. 30 (4), 993–1000. doi:10.1093/nar/30.4.993
Schettini, F., De Santo, I., Rea, C. G., De Placido, P., Formisano, L., Giuliano, M., et al. (2018). CDK 4/6 Inhibitors as Single Agent in Advanced Solid Tumors. Front. Oncol. 8, 608. doi:10.3389/fonc.2018.00608
Serrano, M., Hannon, G. J., and Beach, D. (1993). A New Regulatory Motif in Cell-Cycle Control Causing Specific Inhibition of Cyclin D/CDK4. Nature 366 (6456), 704–707. doi:10.1038/366704a0
Stayton, C. L., Dabovic, B., Gulisano, M., Gecz, J., Broccoll, V., Glovanazzl, S., et al. (1994). Cloning and Characterization of a New Human Xq13 Gene, Encoding a Putative Helicase. Hum. Mol. Genet. 3 (11), 1957–1964. doi:10.1093/hmg/3.11.1957
Stünkel, W., Peh, B. K., Tan, Y. C., Nayagam, V. M., Wang, X., Salto-Tellez, M., et al. (2007). Function of the SIRT1 Protein Deacetylase in Cancer. Biotechnol. J. 2 (11), 1360–1368. doi:10.1002/biot.200700087
Sun, L., Wang, H., Wang, Z., He, S., Chen, S., Liao, D., et al. (2012). Mixed Lineage Kinase Domain-like Protein Mediates Necrosis Signaling Downstream of RIP3 Kinase. Cell 148 (1-2), 213–227. doi:10.1016/j.cell.2011.11.031
Sun, X., Lee, J., Navas, T., Baldwin, D. T., Stewart, T. A., and Dixit, V. M. (1999). RIP3, a Novel Apoptosis-Inducing Kinase. J. Biol. Chem. 274 (24), 16871–16875. doi:10.1074/jbc.274.24.16871
Swann, J. B., Vesely, M. D., Silva, A., Sharkey, J., Akira, S., Schreiber, R. D., et al. (2008). Demonstration of Inflammation-Induced Cancer and Cancer Immunoediting during Primary Tumorigenesis. Proc. Natl. Acad. Sci. U.S.A. 105 (2), 652–656. doi:10.1073/pnas.0708594105
Tsuchida, M., Yokosawa, T., Noguchi, T., Shimada, T., Yamada, M., Sekiguchi, Y., et al. (2020). Pro-apoptotic Functions of TRAF2 in P53-Mediated Apoptosis Induced by Cisplatin. J. Toxicol. Sci. 45 (4), 219–226. doi:10.2131/jts.45.219
Wang, H., Sun, L., Su, L., Rizo, J., Liu, L., Wang, L.-F., et al. (2014). Mixed Lineage Kinase Domain-like Protein MLKL Causes Necrotic Membrane Disruption upon Phosphorylation by RIP3. Mol. Cell 54 (1), 133–146. doi:10.1016/j.molcel.2014.03.003
Wang, T., Jin, Y., Yang, W., Zhang, L., Jin, X., Liu, X., et al. (2017). Necroptosis in Cancer: An Angel or a Demon? Tumour Biol. 39 (6), 101042831771153. doi:10.1177/1010428317711539
Watson, L. A., Goldberg, H., and Bérubé, N. G. (2015). Emerging Roles of ATRX in Cancer. Epigenomics 7 (8), 1365–1378. doi:10.2217/epi.15.82
Wieser, V., Tsibulak, I., Degasper, C., Welponer, H., Leitner, K., Parson, W., et al. (2019). Tumor Necrosis Factor Receptor Modulator Spermatogenesis-Associated Protein 2 Is a Novel Predictor of Outcome in Ovarian Cancer. Cancer Sci. 110 (3), 1117–1126. doi:10.1111/cas.13955
Witcher, M., and Emerson, B. M. (2009). Epigenetic Silencing of the p16INK4a Tumor Suppressor Is Associated with Loss of CTCF Binding and a Chromatin Boundary. Mol. Cell 34 (3), 271–284. doi:10.1016/j.molcel.2009.04.001
Ye, H., Arron, J. R., Lamothe, B., Cirilli, M., Kobayashi, T., Shevde, N. K., et al. (2002). Distinct Molecular Mechanism for Initiating TRAF6 Signalling. Nature 418 (6896), 443–447. doi:10.1038/nature00888
Zhang, H., Duan, J., Qu, Y., Deng, T., Liu, R., Zhang, L., et al. (2016). Onco-miR-24 Regulates Cell Growth and Apoptosis by Targeting BCL2L11 in Gastric Cancer. Protein Cell 7 (2), 141–151. doi:10.1007/s13238-015-0234-5
Keywords: risk score, prognosis, squamous cell carcinoma, necroptosis, immune, tumor
Citation: Huang J, Lu R, Zhong D, Weng Y and Liao L (2022) A Novel Necroptosis-Associated IncRNAs Signature for Prognosis of Head and Neck Squamous Cell Carcinoma. Front. Genet. 13:907392. doi: 10.3389/fgene.2022.907392
Received: 29 March 2022; Accepted: 04 May 2022;
Published: 08 June 2022.
Edited by:
Yan Gong, Wuhan University, ChinaReviewed by:
Zhe Wang, Affiliated Zhongshan Hospital of Dalian University, ChinaCopyright © 2022 Huang, Lu, Zhong, Weng and Liao. This is an open-access article distributed under the terms of the Creative Commons Attribution License (CC BY). The use, distribution or reproduction in other forums is permitted, provided the original author(s) and the copyright owner(s) are credited and that the original publication in this journal is cited, in accordance with accepted academic practice. No use, distribution or reproduction is permitted which does not comply with these terms.
*Correspondence: Lianming Liao, bGlhbzc3MjAwMkBhbGl5dW4uY29t; Youliang Weng, d3lsNzc4OEBzaW5hLmNvbQ==
†These authors have contributed equally to this work
Disclaimer: All claims expressed in this article are solely those of the authors and do not necessarily represent those of their affiliated organizations, or those of the publisher, the editors and the reviewers. Any product that may be evaluated in this article or claim that may be made by its manufacturer is not guaranteed or endorsed by the publisher.
Research integrity at Frontiers
Learn more about the work of our research integrity team to safeguard the quality of each article we publish.