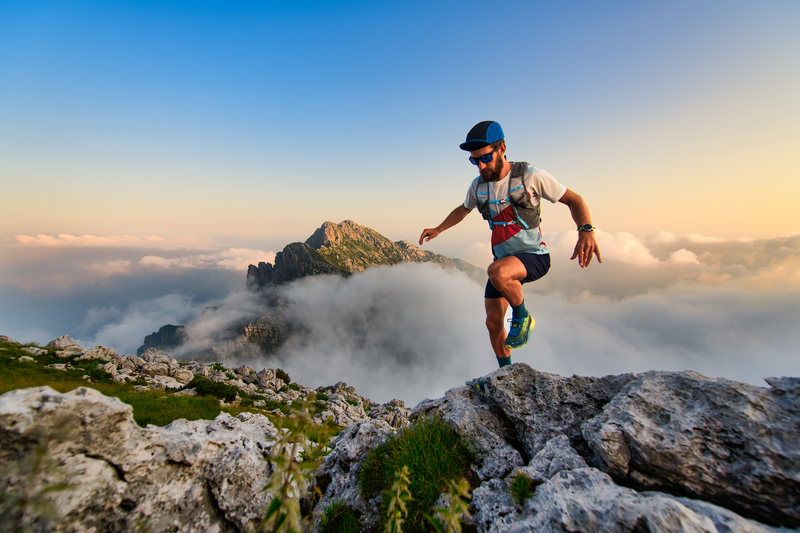
95% of researchers rate our articles as excellent or good
Learn more about the work of our research integrity team to safeguard the quality of each article we publish.
Find out more
ORIGINAL RESEARCH article
Front. Genet. , 15 September 2022
Sec. Computational Genomics
Volume 13 - 2022 | https://doi.org/10.3389/fgene.2022.905650
The transient receptor potential (TRP) channel is a type of channel protein widely distributed in peripheral and central nervous systems. Genes encoding TRP can be regulated by natural aromatic substances and serve as a therapeutic target for many diseases. However, the role of TRP-related genes in lung adenocarcinoma (LUAD) remains unclear. In this study, we used data from TCGA to screen and identify 17 TRP-related genes that are differentially expressed between LUAD and normal lung tissues. Based on these differentially expressed genes (DEGs), we classified all patients with LUAD into two subtypes. Significant differences in prognosis, clinical features, and immune cell infiltration characteristics were observed between the two subtypes. Subsequently, a prognostic signature with 12 genes was established by applying the least absolute shrinkage and selection operator (LASSO) Cox regression method, and all patients with LUAD were classified into low- and high-risk groups. Patients with LUAD in the low-risk group had a significantly longer survival time than those in the high-risk group (p < 0.001), which was confirmed by LUAD data from the GSE72094 and GSE68571 validation datasets. Combined with clinical characteristics, the risk score was found to be an independent predictor of overall survival (OS) in patients with LUAD. Additionally, patients with high TRP scores exhibited poorer clinical characteristics and immune status while showing a sensitive response to chemotherapeutic agents. In conclusion, the TRP score is a promising biomarker for determining the prognosis, molecular subtype, tumor microenvironment, and guiding personalized treatment in patients with LUAD.
Lung cancer is one of the most common causes of cancer-related deaths worldwide. Primary lung cancer is caused by exposure to smoking, ionizing radiation, and environmental pollution. Non-small cell lung cancer (NSCLC) accounts for the vast majority of lung cancer cases, among which lung adenocarcinoma (LUAD) is the most common histological subtype of lung cancer (Denisenko et al., 2018; Bade and Dela Cruz, 2020; Zhang et al., 2020). Despite recent developments in targeted therapy and immunotherapy in the treatment of lung cancer, which have improved patient prognosis, the 5-year survival rate remains below 20% (Hirsch et al., 2017; Greenawalt et al., 2019). Given the limitations of LUAD treatment, new therapeutic targets are needed to improve clinical outcomes; therefore, there is an urgent need to establish reliable and novel prognostic models to make targeted therapy more feasible.
Members of the TRP channel family are potential biomarkers and drug targets in tumor therapy (Xing et al., 2021). Recent studies have identified the TRP channel interactome as a new therapeutic target for breast cancer and have shown that it promotes proliferation and poor prognosis in esophageal squamous cell carcinoma (María Paz et al., 2021; Wang et al., 2021). In addition, TRP-related genes are associated with tumor progression in adenocarcinoma of the prostate (Di Donato et al., 2021) and colon (Liu et al., 2022) and urothelial carcinoma of the bladder (Shapovalov et al., 2016). Therefore, uncovering the role of TRP-related genes in the prognosis of LUAD would be of great significance.
In this study, we performed a systematic expression analysis of TRP-related genes between the normal lung and LUAD tissues and explored the prognostic value of these genes. A 12-gene TRP-related prognostic signature was developed by analyzing RNA-seq data from patients with LUAD in TCGA database and corresponding clinical information. Subsequently, further validation was obtained by analyzing data from the GSE72094 and GSE68571 cohorts. Finally, we analyzed the correlation between TRP-related prognostic signatures and the tumor immune microenvironment. Thus, our data may provide additional evidence for prognostic biomarkers and therapeutic targets in LUAD.
The transcriptome data in the FPKM format and corresponding clinical information of 58 para-cancerous samples and 497 LUAD samples were collected from TCGA database (https://portal.gdc.cancer.gov/). The expression data for GSE72094 and GSE68571 cohorts, as well as the clinical data, were downloaded from the GEO (https://www.ncbi.nlm.nih.gov/geo/) database as the validation set for the risk model. TRP-related genes were derived from the REACTOME_TRP_CHANNELS pathway in the MsigDB database and inflammatory mediator regulation of the TRP channel pathway in the KEGG database, and those genes with duplicate values were removed.
Differential expression analysis was performed on LUAD samples and normal samples from TCGA using the limma R package. The Benjamini–Hochberg (FDR) corrected p-value adj. p-value < 0.05 and |log2 FC| > 0.585 were used as thresholds to screen for DEGs. The same method was used to perform differential expression analysis between tumor subtypes and to screen for genes with significant differences in their expression.
Consistent clustering analysis was performed on LUAD samples using the ConsensusClusterPlus R package. The Euclidean method was used to calculate the clustering distance (the clustering method was k-means), and 100 replications were performed to ensure the stability of the classification. KM survival curves were plotted for patients with subtypes using the survival R package, and the significance of prognostic differences between subtypes was determined using the log-rank test.
One-way Cox regression analysis was performed to screen genes associated with prognosis (OS) based on DEGs among tumor subtypes (p < 0.01). Finally, LASSO regression was used to further screen out key prognosis-related genes and construct a multifactorial regression prognostic model. Tumor samples were classified into high- and low-risk groups using the median risk score as the threshold point, and survival curves for prognostic analysis were generated by the Kaplan–Meier method, and the significance of differences was determined using log-rank tests. ROC (receiver operating characteristic) curves were then plotted using the timeROC R package to evaluate the prediction of scoring by the perturbation scoring model. The risk score was calculated using the following formula:
The CIBERSORT algorithm is a method to characterize complex tissues based on their gene expression profiles. A leukocyte signature gene matrix of 547 genes, LM22, was used to distinguish between 22 immune cell types, including myeloid subpopulations, natural killer (NK) cells, plasma cells, naive and memory B cells, and seven T-cell types. CIBERSORT combined with the LM22 signature matrix was used to estimate the proportion of 22 cell phenotypes in samples, with the sum of the proportions of all immune cell types in each sample equal to 1.
Immune checkpoints refer to a series of molecules expressed in immune cells that regulate the level of immune activation and play an important role in the occurrence of autoimmune diseases. This study aimed to explore the correlation between immune checkpoint gene expression and TRPRS.
The sensitivity of 138 drugs in the GDSC database (IC50 values) was predicted using the pRRophetic R package, combined with the expression data of model genes, and the sensitivity of patients with LUAD to drug treatment was assessed by IC50 values. The differences in IC50 values between high- and low-risk groups were also compared by the Wilcoxon test, and drugs with significant differences between the two groups were screened.
Waterfall plots were plotted using the maftools R package to demonstrate mutations in LUAD samples with mutation frequencies in the top 20 genes and differences in mutations in high- and low-risk groups. The analysis of copy number variations in LUAD samples from TCGA was performed using GISTIC2, and these variations were mapped to highlight CNV differences between the high- and low-risk groups.
The Wilcoxon test was used to compare differences between two sample groups, and the Kruskal–Wallis test was used to compare differences between multiple sample groups, where ns indicates p > 0.05, * indicates p ≤0.05, ** indicates p ≤0.01, *** indicates p ≤0.001, and **** indicates p ≤0.0001.
First, we obtained 4,363 DEGs, including 73 TRP-related genes, using differential expression analysis of tumor samples (N = 497) and normal samples (N = 58) (Figure 1A, p < 0.05). Then, we selected 17 of the most significantly DEGs to plot the gene volcanoes (Figure 1B, p < 0.001). To better understand TRP-related gene interactions, we used the STRING database to obtain TRP-related protein–protein interaction (PPI) networks, with red representing upregulated genes and green representing downregulated genes (Figure 1C). Subsequently, we analyzed the differences in the expression of TRP-related genes in different clinical subgroups according to the grouping of the clinical information of the samples (Figure 2).
FIGURE 1. Differential heatmap of 73 TRP‐related genes (A); volcano map shows the 17 of the most significantly DEGs (B); Protein‐protein Interaction (PPI) of TRP‐related Genes (C).
Based on all TRP-related genes, 497 tumor samples were classified into two different subtypes using consistent clustering: cluster1 (N = 269) and cluster2 (N = 228) (Figure 3A). The results from the Kaplan–Meier plot showed the significant differences in the survival probability and recurrence rate among these two subtypes (Figure 3B, p = 0.029). We further analyzed the CDF delta area curve and found that the area under the CDF curve tended to be stable after two clusters (Figure 3C). Heat maps of TRP subtypes and clinical features were generated from the clustering results, and statistical tests were performed to calculate the significance of the correlation between the results of subtype grouping and those of clinical feature grouping. We found that sex, ethnicity, T stage, and tumor stage were significantly associated with TRP subtypes (Figure 3D). Finally, we clustered the TCGA-LUAD dataset, calculated the proportion of 22 types of immune cells between subtypes, and found significant differences in immune cell infiltration between the two subtypes (Figure 3E).
FIGURE 3. Consistent clustering result of tumor samples (A); Survival curves and CDF curves of the two subtypes were obtained according to the clustering results (B,C); Heat map of TRP subtypes and clinical features (D); 22 immune cell infiltration differences between subtypes (E). *p < 0.05, **p < 0.01, ***p < 0.001, ****p < 0.0001.
We performed univariate Cox screening of prognosis-related genes using DEGs among TRP subtypes, resulting in 158 prognostic factors. The univariate Cox results were then further downscaled using LASSO linear regression to screen 12 prognostic-related signatures (Figures 4A–C). The samples from the TCGA LUAD training set were divided into two groups of high and low risk using the median risk score as the threshold. As shown in Figure 4D, there was a significant survival difference between high- and low-risk groups. The ROC curves for the prognostic features had AUC values of 0.720/0.695/0.671 at 1/3/5 years. Subsequently, we validated and confirmed the validity of the signature in the GSE72094 and GSE68571 validation datasets (Figures 4E, F).
FIGURE 4. LASSO linear regression and univariate Cox analysis screened 12 feature genes associated with prognosis (A-C); Survival curve and ROC curve between the high-risk and low-risk groups, verified by E and F validation sets (D-F).
To explore the association of risk scores with different clinical characteristics, we performed a subgroup analysis of different clinical characteristics and found significant differences in risk scores with respect to age (p = 0.0024), sex (p = 0.006), TNM stage (p = 1.2e-07), N stage (p = 5.6e-07), T stage (p = 2.7e-06), and smoking history (p = 8.6e-06) (Figures 5A–F). We used univariate and multivariate Cox regression analyses to assess whether the risk score could be used as an independent prognostic factor. The results of TCGA training set validation are shown in Figure 6A. Risk scores and tumor stage were independent factors for poor survival in patients with LUAD. This result was further verified by univariate and multivariate analyses of the GSE72094 validation set (Figure 6B). We also performed chi-squared tests for clinical characteristics such as age, gender, stage, and smoking in control and tumor groups, which showed no significant differences (Supplementary Table S1). In addition, the risk score was a good predictor of patient prognosis in groups with different clinical characteristics (Figures 7A–H).
FIGURE 6. Univariate and multivariate Cox regression analyses were performed to assess the risk score and verified by validation set B (A,B).
FIGURE 7. Survival outcomes of patients with different clinical characteristics in the high and low groups (A–H).
Mutations in genes can promote, cause, or orchestrate the malignant progression of tumors, and the study of mutations at the genomic level is important for the development of targeted cancer drugs and novel cancer therapies. To demonstrate the distribution of somatic variants between high- and low-risk groups and to demonstrate the distribution of mutations between samples with different clinical characteristics, the top 20 genes with the highest mutation frequencies were selected for the waterfall plot, as shown in Figure 8A Supplementary Table S2. Because the prognosis of patients in the high-risk group was worse than that of patients in the low-risk group, we speculated whether the risk degree is related to the mutations of some common cancer-promoting genes. Therefore, we further analyzed the gene mutations between high- and low-risk groups. We found a higher frequency of mutations in cancer-promoting genes, such as TP53, MUC16, and TTN, in the high-risk group. Therefore, we demonstrated a correlation between the expression of the degree of risk and the mutation of cancer-promoting genes. Additionally, we compared whether high- and low-risk groups had different levels of amplification and deletion. In high- and low-risk groups, we counted the number of amplifications and deletions of 22 groups of chromosomes. As shown in Figure 8B and Supplementary Table S3, there was a large degree of amplification and deletion in the high-risk group. To explore the correlation between high- and low-risk groups and the tumor microenvironment, we calculated the difference in immune infiltration between high- and low-risk groups based on the TCGA-LUAD dataset. As shown in Figure 8C, we found significant differences between the low- and high-risk groups in nine immune cell types (memory B cells, resting dendritic cells, M0 macrophages, activated mast cells, resting mast cells, monocytes, resting NK cells, memory-activated CD4 T cells, and memory-resting CD4 CT cells). The low-risk group had more immune cell infiltration than the high-risk group.
FIGURE 8. I plotted the waterfall and selected the top 20 genes with the highest frequency of mutations (A); Amplification and deletion of chromosomes in the high-low risk groups (B); Differential results of immune infiltration in high-low risk groups (C). *p < 0.05, **p < 0.01, ***p < 0.001, ****p < 0.0001.
To screen for antineoplastic drugs, we predicted the sensitivity (IC50 values) of 138 drugs in the GDSC database based on the expression profiles of all the characterized genes in our model and screened 53 LUAD-associated drugs (Table 1). Then, we found that response to 41 drugs differed significantly between high- and low-risk groups, and the top 8 drugs (pazopanib, salubrinal, GW843682X, docetaxel, sorafenib, paclitaxel, cytarabine, and temsirolimus) with the most significant differences in their responses are shown in Figure 9.
FIGURE 9. High-risk and low-risk groups respond differently to the eight drugs (A–H). *p < 0.05, **p < 0.01, ***p < 0.001, ****p < 0.0001.
Transient receptor potential (TRP) channels are a versatile family of ion channels. Within the TRP ion channel family, TRPV1 primarily mediates pain and burning induced by spicy compounds in the somatosensory system (Steinritz et al., 2018). TRP channels play an important role not only in mediating pain but also in the cell cycle, often by regulating gene transcription and affecting other cellular processes such as proliferation, apoptosis, or cell motility (Shapovalov et al., 2016; Yu et al., 2016). Previous studies have shown that the TRP ion channel family is associated with the progression of several cancers. For example, the increased expression of TRPM7 is associated with poor prognosis and metastasis in nasopharyngeal carcinoma. TRPC1, TRPC5/6, TRPM4, TRPM7/8, TRPV1/2, TRPV4, and TRPV6 are strongly associated with progression and could be new therapeutic targets for breast invasive carcinoma (Chen et al., 2015; Saldías et al., 2021). In addition, several studies have identified the TRP ion channel family genes as promising predictors of prognosis and immunotherapeutic efficacy in patients with cancer through pan-cancer analysis (Pan et al., 2022; Wu et al., 2022). However, the role of the TRP ion channel family in LUAD remains elusive. Therefore, we searched for TRP-related genes through the TRP pathway and performed a clustering analysis of patients with LAUD. Then, to investigate the link between patient prognosis and the TRP pathway, we built a survival prediction model based on TRP-related subtypes.
In this study, we downloaded expression profile data and mutation data from TCGA database and analyzed them for differential expression. After screening for DEGs, we performed clustering analysis and determined the differential relationships between subtypes and prognosis, clinical features, and immune infiltration. The focus of this study was to screen out prognostic genes and construct a TRP prognostic stratification scoring system. We downloaded and screened TRP-related crossover genes from MsigDB and KEGG databases, screened 12 prognosis-related signatures, and used the median gene expression as the cutoff value for high- and low-risk groups. The expression data and clinical data were downloaded from the GEO database as the validation set for the risk model, and the stability of the model validity was tested by evaluating and validating training and validation sets. Some of these 12 signatures have been confirmed to be closely related to the occurrence and development of lung cancer. For example, induction of AREG expression sensitizes lung cancer cells to EGFR TKI and increases the tumorigenic dependence of non-small cell lung cancer on the AREG-induced EGFR signaling pathway, thus enhancing the progression of NSCLC (Tu et al., 2018). High CCR2 expression is associated with a poor prognosis of various cancers, while inhibition of CCR2 expression may enhance the inhibitory effect of PD-1 on tumors. Simultaneously, Yi et al. discovered that CCR2 expression was connected with prognosis, favorably correlated with survival rate and prognosis of the M type, negatively correlated with the prognosis of T and N types, and correlated with immune cell infiltration of different malignancies (An et al., 2017). Functional CDKN3, but not dominant-negative CDKN3 mutants, is overexpressed in LUAD, and overexpression of major CDKN3 transcripts is associated with poor survival in patients with LUAD (Fan et al., 2015). RHOV is expressed in lung cancer cell lines and is upregulated in most of the lung tumor cases studied (Shepelev and Korobko, 2013). The overexpression of RHOV in LUAD promotes the progression of LUAD and EGFR-TKI resistance, which may be related to the activation of the AKT/ERK pathway (Chen et al., 2021). RHOV plays a key role in LUAD metastasis and may provide a biomarker for the prognosis and treatment of LUAD (Zhang et al., 2021). Correlations between different clinical characteristics and model scores were explored based on the grouping of clinical characteristics and risk values for each sample in the model. Based on univariate and multivariate Cox regression analyses, we found that risk groups can be used as independent risk factors. In difference analysis, chi-squared tests were performed on the clinical traits such as age, gender, stage, and smoking in the control group and tumor group, and no significant differences were found. The risk score also constructs the survival curve of clinical characteristics, which is clearly important in guiding clinical outcomes. We found that the high- and low-risk groups not only possessed higher mutations and CNV alterations but also predicted worse prognosis, clinical characteristics, and the tumor microenvironment in patients with LUAD. In addition to that, there were significant differences in the frequencies of mutations between high- and low-risk groups for COL11A1, CSMD3, FAT3, LRP1B, MUC16, PCDH15, PCLO, RYR2, SPTA1, TP53, TTN, XIRP2, ZFHX4, and other genes. Additionally, we found a large degree of amplification and deletion in the high-risk group. Finally, the IC50 value for multiple drugs was predicted to be significantly different between high- and low-risk groups based on the expression of all characteristic genes in the constructed model. In conclusion, our study provides new insights into the individualized treatment of LUAD.
The median risk score is used as a threshold to distinguish between high- and low-risk groups, and the essence of the grouping is to find factors influencing tumor development. Our study not only illustrates the differences between high- and low-risk scores in clinical traits and survival prognosis but also demonstrates the correlation between high- and low-risk groups in terms of genetic mutations, immune cell infiltration, and tumor drug resistance. The clinical term high risk includes mainly the risk of the disease, the risk of disease progression, the difficulty of curing the disease, and the risk of tumor recurrence. Thus, the high-risk group constructed in our study has an inclusive relationship with clinical high-risk, and our risk model can be used to predict the development of clinical risk and prognosis for survival, to assess the degree of clinical risk and to guide clinical treatment options.
There are still some limitations to our study. The problem of sample imbalance is an important and common problem in data analysis and is largely related to the original data itself and the method of analysis. Although we try to avoid the effects of sample imbalance when analyzing the data, the imbalance cannot be completely avoided, and we can only try to reduce the interference of sample imbalance in the analysis of data, the construction of models, and the evaluation of model validity. Although several independent external validations were performed in this study, there may be an innate case-selection bias in the results when collecting tissue and information retrospectively in publicly available databases. Therefore, reliable in vitro and in vivo experiments as well as large-scale prospective clinical trials are needed to confirm our findings.
In conclusion, we performed a comprehensive and systematic bioinformatics analysis and identified the TRP-related prognostic gene signature for LUAD patients. The TRP score is a promising biomarker for determining the prognosis, molecular subtype, tumor microenvironment, and drug selection in patients with LUAD. Therefore, our study provides new insights into the individualized treatment of LUAD.
The original contributions presented in the study are included in the article/Supplementary Material; further inquiries can be directed to the corresponding author.
Written informed consent was obtained from the individual(s) for the publication of any potentially identifiable images or data included in this article.
MH conceived and designed the study. MH and GW acquired the data and drafted the manuscript. ZW and KR performed the statistical analysis and technical support. QX and ZY provided all the funding for this study. All authors contributed to the manuscript and approved the submitted version.
The authors declare that the research was conducted in the absence of any commercial or financial relationships that could be construed as a potential conflict of interest.
All claims expressed in this article are solely those of the authors and do not necessarily represent those of their affiliated organizations, or those of the publisher, the editors, and the reviewers. Any product that may be evaluated in this article, or claim that may be made by its manufacturer, is not guaranteed or endorsed by the publisher.
The Supplementary Material for this article can be found online at: https://www.frontiersin.org/articles/10.3389/fgene.2022.905650/full#supplementary-material
SUPPLEMENTARY TABLE S1 | Chi-square test results of clinical characteristics in tumor group and control group.
SUPPLEMENTARY TABLE S2 | Chi-square test results of the top 20 genes with the highest mutation frequency in the high-low risk group.
SUPPLEMENTARY TABLE S3 | Chi-square test results of chromosome amplification and deletion in high and low-risk groups.
An, J., Xue, Y., Long, M., Zhang, G., Zhang, J., and Su, H. (2017). Targeting CCR2 with its antagonist suppresses viability, motility and invasion by downregulating MMP-9 expression in non-small cell lung cancer cells. Oncotarget 8 (24), 39230–39240. doi:10.18632/oncotarget.16837
Bade, B. C., and Dela Cruz, C. S. (2020). Lung cancer 2020: Epidemiology, etiology, and prevention. Clin. Chest Med. 41, 1–24. doi:10.1016/j.ccm.2019.10.001
Chen, H., Xia, R., Jiang, L., Zhou, Y., Xu, H., Peng, W., et al. (2021). Overexpression of RhoV promotes the progression and EGFR-TKI resistance of lung adenocarcinoma. Front. Oncol. 11, 619013. PMID: 33767988. doi:10.3389/fonc.2021.619013
Chen, J. P., Wang, J., Luan, Y., Wang, C. X., Li, W. H., Zhang, J. B., et al. (2015). TRPM7 promotes the metastatic process in human nasopharyngeal carcinoma. Cancer Lett. 356, 483–490. doi:10.1016/j.canlet.2014.09.032
Denisenko, T. V., Budkevich, I. N., and Zhivotovsky, B. (2018). Cell death-based treatment of lung adenocarcinoma. Cell Death Dis. 9, 117. doi:10.1038/s41419-017-0063-y
Di Donato, M., Ostacolo, C., Giovannelli, P., Di Sarno, V., Gomez Monterrey, I. M., Campiglia, P., et al. (2021). Therapeutic potential of TRPM8 antagonists in prostate cancer. Sci. Rep. 11 (1), 23232. doi:10.1038/s41598-021-02675-4
Fan, C., Chen, L., Huang, Q., Shen, T., Welsh, E. A., Teer, J. K., et al. (2015). Overexpression of major CDKN3 transcripts is associated with poor survival in lung adenocarcinoma. Br. J. Cancer 113 (12), 1735–1743. PMID: 26554648. doi:10.1038/bjc.2015.378
Greenawalt, E. J., Edmonds, M. D., Jain, N., Adams, C. M., Mitra, R., and Eischen, C. M. (2019). Targeting of SGK1 by miR-576-3p inhibits lung adenocarcinoma migration and invasion. Mol. Cancer Res. 17, 289–298. doi:10.1158/1541-7786.MCR-18-0364
Hirsch, F. R., Scagliotti, G. V., Mulshine, J. L., Kwon, R., Curran, W. J., Wu, Y. L., et al. (2017). Lung cancer: Current therapies and new targeted treatments. Lancet 389, 299–311. doi:10.1016/S0140-6736(16)30958-8
Liu, J-J., Li, L-Z., and Xu, P. (2022). Upregulation of TRPM8 can promote the colon cancer liver metastasis through mediating akt/GSK-3 signal pathway. Biotechnol. Appl. Biochem. 69 (1), 230–239. doi:10.1002/bab.2102
María Paz, S., Diego, M., Orellana-Serradell, O., Silva, I., Lavanderos, B., and Cruz, P. (2021). TRP channels interactome as a novel therapeutic target in breast cancer. Front. Oncol. 11, 621614.
Pan, T., Gao, Y., Xu, G., Zhou, P., Li, S., Guo, J., et al. (2022). Pan-cancer analyses reveal the genetic and pharmacogenomic landscape of transient receptor potential channels. NPJ Genom. Med. 7, 32. doi:10.1038/s41525-022-00304-1
Saldías, M. P., Maureira, D., Orellana-Serradell, O., Silva, I., Lavanderos, B., Cruz, P., et al. (2021). TRP channels interactome as a novel therapeutic target in breast cancer. Front. Oncol. 11, 621614. doi:10.3389/fonc.2021.621614
Shapovalov, G., Ritaine, A., Skryma, R., and Prevarskaya, N. (2016). Role of TRP ion channels in cancer and tumorigenesis. Semin. Immunopathol. 38 (3), 357–369. Epub 2016 Feb 3.PMID: 26842901. doi:10.1007/s00281-015-0525-1
Shepelev, M. V., and Korobko, I. V. (2013). The RHOV gene is overexpressed in human non-small cell lung cancer. Cancer Genet. 206 (11), 393–397. Epub 2013 Nov 5.PMID: 24388711. doi:10.1016/j.cancergen.2013.10.006
Steinritz, D., Stenger, B., Dietrich, A., Gudermann, T., and Popp, T. (2018). TRPs in tox: Involvement of transient receptor potential-channels in chemical-induced organ toxicity-A structured review. Cells 7 (8), 98. doi:10.3390/cells7080098
Tu, C. Y., Wang, B. W., Cheng, F. J., Chen, C. H., Hsia, T. C., Wei, Y. L., et al. (2018). Incense burning smoke sensitizes lung cancer cells to EGFR TKI by inducing AREG expression. Am. J. Cancer Res. 8 (12), 2575–2589. eCollection 2018.PMID: 30662813.
Wan, Y., Wang, X., Liu, T., Fan, T., Zhang, Z., Wang, B., et al. (2021). Prognostic value of CCR2 as an immune indicator in lung adenocarcinoma: A study based on tumor-infiltrating immune cell analysis. Cancer Med. 10 (12), 4150–4163. Epub 2021 May 4.PMID: 33949150. doi:10.1002/cam4.3931
Wang, K., Feng, X., Zheng, L., Chai, Z., Yu, J., You, X., et al. (2021). TRPV4 is a prognostic biomarker that correlates with the immunosuppressive microenvironment and chemoresistance of anti-cancer drugs. Front. Mol. Biosci. 8, 690500. doi:10.3389/fmolb.2021.690500
Wu, G., He, M., Yin, X., Wang, W., Zhou, J., Ren, K., et al. (2022). The pan-cancer landscape of crosstalk between TRP family and tumour microenvironment relevant to prognosis and immunotherapy response. Front. Immunol. 13, 837665. doi:10.3389/fimmu.2022.837665
Xing, Y., Wei, X., Liu, Y., Wang, M. M., Sui, Z., Wang, X., et al. (2021). Autophagy inhibition mediated by MCOLN1/TRPML1 suppresses cancer metastasis via regulating a ROS-driven TP53/p53 pathway. Autophagy 18, 1932–1954. Epub ahead of print. PMID: 34878954. doi:10.1080/15548627.2021.2008752
Yu, X., Yu, M., Liu, Y., and Yu, S. (2016). TRP channel functions in the gastrointestinal tract. Semin. Immunopathol. 38 (3), 385–396. Epub 2015 Oct 12.PMID: 26459157. doi:10.1007/s00281-015-0528-y
Zhang, D., Jiang, Q., Ge, X., Shi, Y., Ye, T., Mi, Y., et al. (2021). RHOV promotes lung adenocarcinoma cell growth and metastasis through JNK/c-Jun pathway. Int. J. Biol. Sci. 17 (10), 2622–2632. PMID: 34326698. doi:10.7150/ijbs.59939
Zhang, T., Bao, J., Zhang, M., Ge, Y., Wei, J., Li, Y., et al. (2020). Chemo-photodynamic therapy by pulmonary delivery of gefitinib nanoparticles and 5-aminolevulinic acid for treatment of primary lung cancer of rats. Photodiagnosis Photodyn. Ther. 31, 101807. Epub 2020 May 11.PMID: 32404298. doi:10.1016/j.pdpdt.2020.101807
Keywords: lung adenocarcinoma, prognosis, immune, TRP-related gene, gene signature
Citation: He M, Wu G, Wang Z, Ren K, Yang Z and Xue Q (2022) Development and validation of a TRP-related gene signature for overall survival prediction in lung adenocarcinoma. Front. Genet. 13:905650. doi: 10.3389/fgene.2022.905650
Received: 29 April 2022; Accepted: 25 July 2022;
Published: 15 September 2022.
Edited by:
Zhibin Lv, Sichuan University, ChinaReviewed by:
Fenglong Yang, Fujian Medical University, ChinaCopyright © 2022 He, Wu, Wang, Ren, Yang and Xue. This is an open-access article distributed under the terms of the Creative Commons Attribution License (CC BY). The use, distribution or reproduction in other forums is permitted, provided the original author(s) and the copyright owner(s) are credited and that the original publication in this journal is cited, in accordance with accepted academic practice. No use, distribution or reproduction is permitted which does not comply with these terms.
*Correspondence: Qun Xue, bnRmeXh1ZXF1bkAxNjMuY29t; Zheng Yang, bm5uODg0OEBzaW5hLmNvbQ==
†These authors have contributed equally to this work
Disclaimer: All claims expressed in this article are solely those of the authors and do not necessarily represent those of their affiliated organizations, or those of the publisher, the editors and the reviewers. Any product that may be evaluated in this article or claim that may be made by its manufacturer is not guaranteed or endorsed by the publisher.
Research integrity at Frontiers
Learn more about the work of our research integrity team to safeguard the quality of each article we publish.