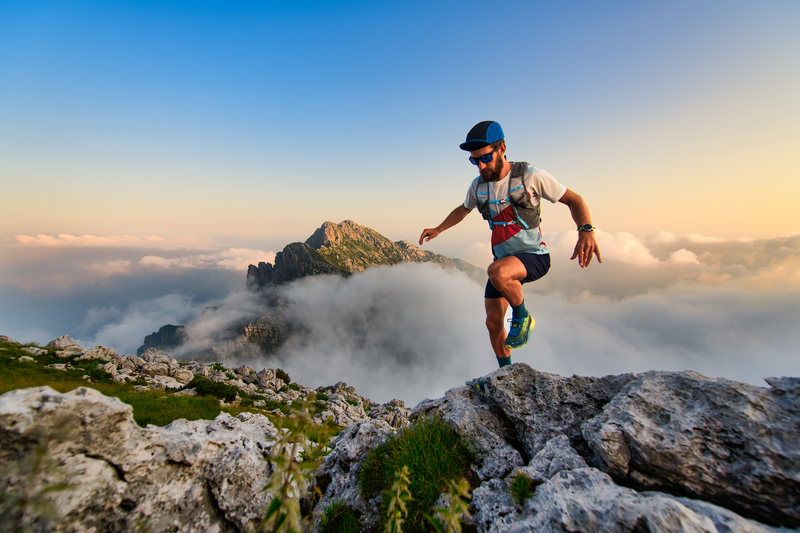
95% of researchers rate our articles as excellent or good
Learn more about the work of our research integrity team to safeguard the quality of each article we publish.
Find out more
ORIGINAL RESEARCH article
Front. Genet. , 24 August 2022
Sec. Genomics of Plants and the Phytoecosystem
Volume 13 - 2022 | https://doi.org/10.3389/fgene.2022.897696
This article is part of the Research Topic Legume Breeding in Transition: Innovation and Outlook View all 28 articles
Common bean is considered a recalcitrant crop for in vitro regeneration and needs a repeatable and efficient in vitro regeneration protocol for its improvement through biotechnological approaches. In this study, the establishment of efficient and reproducible in vitro regeneration followed by predicting and optimizing through machine learning (ML) models, such as artificial neural network algorithms, was performed. Mature embryos of common bean were pretreated with 5, 10, and 20 mg/L benzylaminopurine (BAP) for 20 days followed by isolation of plumular apice for in vitro regeneration and cultured on a post-treatment medium containing 0.25, 0.50, 1.0, and 1.50 mg/L BAP for 8 weeks. Plumular apice explants pretreated with 20 mg/L BAP exerted a negative impact and resulted in minimum shoot regeneration frequency and shoot count, but produced longer shoots. All output variables (shoot regeneration frequency, shoot counts, and shoot length) increased significantly with the enhancement of BAP concentration in the post-treatment medium. Interaction of the pretreatment × post-treatment medium revealed the need for a specific combination for inducing a high shoot regeneration frequency. Higher shoot count and shoot length were achieved from the interaction of 5 mg/L BAP × 1.00 mg/L BAP followed by 10 mg/L BAP × 1.50 mg/L BAP and 20 mg/L BAP × 1.50 mg/L BAP. The evaluation of data through ML models revealed that R2 values ranged from 0.32 to 0.58 (regeneration), 0.01 to 0.22 (shoot counts), and 0.18 to 0.48 (shoot length). On the other hand, the mean squared error values ranged from 0.0596 to 0.0965 for shoot regeneration, 0.0327 to 0.0412 for shoot count, and 0.0258 to 0.0404 for shoot length from all ML models. Among the utilized models, the multilayer perceptron model provided a better prediction and optimization for all output variables, compared to other models. The achieved results can be employed for the prediction and optimization of plant tissue culture protocols used for biotechnological approaches in a breeding program of common beans.
Grain legumes are an important pillar of the agricultural system, are considered a vital source of high-quality protein for food and fodder, and play a significant role in sustainable cropping systems (Vanlauwe et al., 2019). Common bean (Phaseolus vulgaris L.) is an important grain legume crop and is mostly used worldwide for its pods and palatable seeds (Nadeem et al., 2020a). Common bean contains good concentrations of high-quality protein, minerals particularly zinc and iron, vitamins, and antioxidants and is considered a “grain of hope” for the impoverished community. Common bean was originated in Mesoamerica (Bitocchi et al., 2012) and its domestication in Andean and Mesoamerican regions resulted in the formation of two unique gene pools: Andean gene pool and Mesoamerican gene pool (Kami et al., 1995; Mamidi et al., 2013; Asfaw et al., 2017; Blair et al., 2018; Campa et al., 2018). Common bean is considered one of the most varied legume crops by reflecting variations in its growth habit, plant height, pods, maturity, seed weight and size (Yeken et al., 2019; Nadeem et al., 2020b).
Climate change is becoming a serious threat to agriculture, and various biotic (pathogens and insects) or abiotic (drought and edaphic) factors are contributing significantly to the global common bean production loss (Castillo et al., 2015). Keeping these in view, scientists are trying to develop climate-resilient common bean cultivars having improved agronomic and nutritional traits. The mentioned target can be achieved by the application of modern biotechnological techniques and for that reason, optimization of the in vitro plant tissue culture technique for whole plant regeneration is highly demanding. To date, a reasonable number of in vitro regeneration protocols have been established and documented. In vitro regeneration of common bean is an arduous task due to its recalcitrant nature, genotype dependence, lack of reproducibility, low shoot counts with stunted growth, rooting, and acclimatization. Hence, there is always a need to develop a new, efficient, and repeatable protocol for the application of biotechnological techniques to produce elite cultivars, especially for recalcitrant crops (Aasim et al., 2013). To achieve the objective, selection of potent explants with a high regeneration protocol is highly significant. Considering this, a novel explant “plumular apices” and an in vitro regeneration protocol of pretreatment of explants with high benzylaminopurine (BAP) concentration was employed for common bean. Pretreatment is the process of treating seeds or explants with variable stimulants like cytokinins at low to high doses for a certain period, followed by culturing the explants on a post-treatment medium, supplemented with low plant growth regulators (PGRs) or without any PGRs (Özkan and Aasim, 2019).
Conventional plant breeding methodologies include the assessment and classification of genetic diversity, yield component analysis, yield stability analysis, enhanced tolerance to stresses, and hybrid breeding programs. On the other hand, in vitro micropropagation, doubled haploid production, artificial polyploidy induction and Agrobacterium-mediated gene transformation techniques are considered in vitro-based biotechnological breeding methodologies (Niazian and Niedbala, 2020). In plant tissue culture studies, the impacts of input (uni or multi) factors on the regeneration potential (outputs) of desired plants are studied. In general, classical statistical techniques have been employed for analyzing and interpreting the output variables. These techniques are generally based on variance analysis and linear regression models for estimating the correlation between input (independent) and output (dependent) variables. Although these approaches are highly effective, lack of efficacy of complex and nonlinear inputs (Hesami and Jones, 2021; Earl et al., 2021) and high probability (Abbasi et al., 2016; Jamshidi et al., 2016; Farhadi et al., 2020) are the major concerns in plant tissue culture studies due to the sensitivity. These types of issues can be overcome by modern high throughput technologies like machine learning (ML) and artificial neural network (ANN) models for testing and optimizing the output variables concerning the input parameters. Although the application of ML and ANN models in plant sciences specifically in the area of plant tissue culture is in its early stages, it is successfully documented for different aspects of plant tissue culture ranging from in vitro sterilization to in vitro regeneration and from in vitro callogenesis to secondary metabolite production (Hesami et al., 2020a; García-Pérez et al., 2020; Hameg et al., 2020; Hesami & Jones, 2020; Niazian and Niedbala, 2020; Pepe et al., 2021; Salehi et al., 2021; Aasim et al., 2022). In these studies, researchers employed different ML algorithms, and the selection of specific ML models is generally based on the expertise and target set in the study. These data-driven models are highly efficient to parse and interpret different types of datasets (non-normal, nonlinear, and nondeterministic unpredictable data) by using all spectral data along with avoiding irrelevant spectral bands and multicollinearity (Salehi et al., 2020). In this study, an in vitro regeneration protocol of common bean was established using novel plumular apice explants. The results regarding output variables were analyzed and interpreted, and input variables were predicted by response surface methodology (RSM). In addition, the results for the output variables were validated using different ML algorithms (support vector regression—SVR, Gaussian process regression—GPR, XGBoost regression—XGBoost, and random forest regression—RF) and an ANN-based multilayer perceptron (MLP) regression model. The performance was evaluated by tabulating the R2 and the mean squared error (MSE) metric values for each model (Hesami et al., 2019; Kirtis et al., 2022). The results achieved in this study will open a new window to evaluate the efficiency of the plant tissue culture protocols that are predominantly developed for breeding purposes.
The commercial common bean cultivar “Karacaşehir-90” was selected for this study as the plant material. Manually selected uniform seeds were surface sterilized with 3.5% (w/v) NaOCl for 15 min. Thereafter, seeds were continuously rinsed with sterilized dH2O water for 5–7 min and this process was repeated thrice to remove the traces of NaOCl. Seeds (Figure 1A) were awaited for 24 h in dH2O, followed by isolation of mature embryos (Figure 1B) under aseptic conditions. A two-step experiment was designed for this research. At first, mature embryos isolated from sterilized seeds were inoculated on MS (Murashige and Skoog, 1962) media supplemented with 5, 10, and 20 mg/L BAP (pretreatment medium) for 20 days. In the second step, plumular apice explants (Figure 1C) were carefully isolated from pretreated mature explants, followed by inoculation on MS media supplemented with low BAP (0.25, 0.50, 1.00, and 1.50 mg L−1) concentrations (post-treatment medium). The explants were cultured for 8 weeks on the post-treatment medium. Four different concentrations (0.25, 0.50, 1.0, and 1.50 mg L−1) of indole-3-butyric acid (IBA) were used for in vitro rooting. For acclimatization, rooted plantlets were transferred to pots filled with vermiculite, wrapped in a polyethylene bag, and placed in the growth room.
FIGURE 1. In vitro regeneration and rooting of common bean Cv. Karacaşehir 90 (A) sterilized seed with the intact embryo, (B) isolated embryo ready for inoculating on the pretreatment medium, (C) pretreated mature embryo used for isolating the plumular apice explant, (D) multiple shoot induction from the plumular apice explant, and (E) acclimatized plant in a pot containing vermiculite.
The basal media used for pretreatment, post-treatment, and rooting were prepared by adding MS (4.4 g/L), commercial sugar (30 g/L), and polyvinyl proline (25 mg L−1). The pH of all media was adjusted to ∼5.8 with the aid of 1N HCl or 1N NaOH. The medium was gelled with agar (6.5 g/L) and autoclaved at 121°C for 20 min. All experiments were carried out in the growth room at 24 ± 2°C and 16-h light photoperiod, equipped with white light-emitting diodes at approximately 2000 LUX. All chemicals used in this study were procured from Duchefa (MS, BAP, IBA, and agar) and Sigma-Aldrich (polyvinyl proline).
The RSM approach was used to model and optimize the selected responses to changing variables and graphical representation of the results. RSM generates continuous multivariable predictions represented as quadratic surfaces, allowing the prediction of optimal values in three-dimensional space. Pretreatment, post-treatment, and their interactive effect values were used as input variables. On the other hand, regeneration frequency (%), shoot count, and shoot length (cm) were used for the response surface calculations. The degree of predicted mathematical model compliance to obtained values was expressed as R2 fit values. All RSM data analyses, such as analysis of variance, regression, and generation of quadratic polynomial surface equations, graphics and optimal value predictions, were conducted using Minitab v20.4 statistical software.
In this study, interactions of pretreatment (5, 10, and 20 mg/L BAP) × post-treatment BAP doses (0.25, 0.50, 1.0, and 1.50 mg/L) were used as input variables, whereas, in vitro regeneration frequency, shoot count, and shoot length were measured as the output variables. ML algorithms of SVR (Hesami et al., 2020b; Katirci and Takci, 2021), GPR (Hu et al., 2019), XGBoost (Chen and Guestrin, 2016), RF (Aggarwal, 2018), and MLP neural network (Silva et al., 2019) were utilized to train and test the model. The performance of the model was assessed using leave-one-out cross-validation (Sammut and Webb, 2011). The hyperparameters of the ML models were optimized using the grid search technique to find the best model. The open-source Python language (Van Rossum and Drake, 1994) was used to code algorithms using the sklearn library (Pedregosa et al., 2011). MLP, SVR, GP, XGBoost, and RF algorithms were used to predict the outputs. The model performance was evaluated by calculating R2 (coefficient of determination) and MSE values (Hesami et al., 2019), which are presented in Eqs. 1 and 2.
The dataset that is used in plant tissue culture studies may not be linear; hence, nonlinear regression models are essential like the SVR model (Drucker, 1997) as expressed in Eq. 3. In SVR, the output y is a real number and it can be used for nonlinear variables.
In the above equation, b depicts the bias, w represents the weight, and the elevated features space is presented as
The GPR model is another nonparametric supervised learning method that is used mainly to perform Bayesian nonlinear regression and classification tasks. It is a powerful ML algorithm that uses the Gaussian probability density function. The GPR works efficiently with a small dataset, with more accuracy, ease of calculation, and consistency.
The approach is presented in Eq. 4 for each input x and output y produced by this function.
Extreme gradient boosting (XGBoost) is a decision-tree-based ensemble ML algorithm that uses a gradient boosting framework that can be used for both regression and classification problems (Chen and Guestrin, 2016). In ML, ensemble learning algorithms combine multiple ML algorithms to obtain a better model. The XGBoost model generates the regression or classification trees by taking previous trees and factoring in their predictions to create a new tree to decrease prediction error. Eq. 5 indicates the XGBoost objective function and Eq. 6 shows the model of XGBoost at iteration j that needs to be minimized.
where
The RF model is an alternative supervised ensemble learning method based on the decision trees (Breiman, 2001), which can also be implemented for regression and classification problems. It is one of the most widely used ML models due to its simplicity in design, high efficiency, less susceptibility to overfitting, handling the noise, and ability to manage a large number of features. The forest is generated by multiple decision trees and each tree possesses the same distribution. The MSE metric is used to solve the regression models. It determines the distance between the nodes to define which branch is better for the forest. The following Eq. 7 describes this concept (Pavlov et al., 2019).
where y is the value of the data point and n is the number of samples.
The MLP is the most well-known ANN model that consists of more than one perceptron, which includes a nonlinear activation function. MLP is a supervised learning method containing one or more hidden layers. The training continues until the following equation is minimized.
where
Generating an MLP structure is the most important part that significantly influences the performance of the model. It is a prerequisite to defining the number of neurons in each layer and the number of hidden layers during the construction of the model. MLP is often applied to supervised learning problems. The backpropagation method is implemented to tune the weights and biases of the layers (Hesami et al., 2019).
Mature embryo explants exposed to 5, 10, and 20 mg L−1 BAP for 20 days resulted in enhanced embryo size of approximately 60–70% explants, which allowed to isolate plumular apice explants easily under sterile conditions (Figure 1C). Thereafter, explants were inoculated on a post-treatment medium, which resulted in multiple shoot induction within 2–3 weeks along with callus induction from the basal end of some explants. The explants were cultured in the growth room for 8 weeks to induce multiple shoots (Figure 1D). The analysis of variance exhibited the variable response of input variables (pretreatment, post-treatment, and pretreatment × post-treatment) on in vitro regeneration of common bean. Results revealed the significant impact of pretreatment on the regeneration frequency (p < 0.01) and shoot length (p < 0.05). Results on post-treatment (p < 0.01) application of BAP and combination of pretreatment × post-treatment × post-treatment (p < 0.01) revealed a significant impact only on the shoot length. On the other hand, shoot counts have remained insignificant to all input variables (Table 1).
Results revealed that elevated pretreatment concentrations negatively affected the regeneration frequency and shoot counts, which ranged from 47.91 to 96.51% and 2.99 to 3.60, respectively. In contrast, the mean shoot length increased with elevated pretreatment concentration and ranged from 1.07 to 1.57 cm (Supplementary Table S1). Results of post-treatment revealed a better regeneration frequency at high BAP concentrations ranging from 66.67 to 72.22%. In a similar manner, shoot length also exhibited an increase with a respective increase in BAP concentration. On the other hand, the variable impact of the post-treatment medium (BAP) was observed on shoot counts that ranged from 3.06 to 3.92 (Supplementary Table S1). The results on pretreatment × post-treatment exhibited the negative impact of elevated BAP concentration (pretreatment) on shoot regeneration frequency (%) that ranged from 58.33 to 66.67% (10 m g/L BAP) and 41.67 to 58.33% (20 m g/L BAP). However, exposing explants to 5 mg/L BAP resulted in up to 100% regeneration (Figure 2A). The results on shoot counts and shoot length showed the variable impact of pretreatment × post-treatment concentrations (Figures 2B and C). Outcomes revealed that maximum shoot counts were linked with pretreatment × post-treatment concentrations. The maximum shoot counts were obtained for 10 mg/L × 1.50 mg/L BAP (5.0 shoots), 5 mg/L × 0.50 mg/L BAP (4.67 shoots), and 20 mg/L × 1.50 mg/L BAP (3.33 shoots) (Figure 2B). A similar pattern was also observed with the shoot length. The longest shoots were recorded as 1.15 cm (5 mg L−1 × 1.00 mg L−1), 1.40 cm (10 mg L−1 × 1.50 mg L−1), and 1.79 cm (20 mg L−1 × 1.50 mg L−1) (Supplementary Table S2). Results revealed that exposing explants to a high BAP concentration (both pretreatment and post-treatment) medium yielded relatively longer shoots (Figure 2C) compared to other combinations. In this study, contour plots were also constructed for a better presentation and understanding of the data. In the contour plots, the data were distributed into different subgroups, emphasized with different colors. Contour plots help to find out the best combination for the desired output value. Results of contour plots revealed the optimization of <90% regeneration frequency (Figure 3A), 4.0 shoots per explant (Figure 3B), and 2.4 cm longer shoots (Figure 3C) and presented the doses of the pretreatment and post-treatment medium.
FIGURE 2. 3D response surface plots of in vitro regeneration of common bean (A) regeneration, (B) shoot count, and (C) shoot length.
FIGURE 3. Contour plots of in vitro regeneration of common bean (A) regeneration, (B) shoot count, and (C) shoot length.
In vitro regenerated shoots inoculated on the rooting medium yielded a relatively high rooting frequency. Although most of the plants were rooted within the first 3–4 weeks, they were kept in the rooting medium for a total of 6 weeks before shifting to pots for acclimatization. The survival rate of rooted plantlets in pots was relatively less than expected (Figure 1E). The results revealed that the protocol can be used for in vitro regeneration of common bean.
The experiment design of the study was based on pretreatment doses and post-treatment doses, followed by selecting the best mathematical model. The regression equations (Eq. 9–11) for the response variables [R2 (measured), R2 (Adj.), and R2 (pred.)] were used and their respective values are presented in Table 2.
The results of the regression model depicted more R2 (measured) compared to R2 (Adj.) and R2 (pred.) for all tested output variables used in this study. The R2 values for regeneration were recorded as 56.27 (R2 measured), 48.98 (R2 adj.), and 38.68 (R2 pred.). R2 for shoot length was recorded as 47.84 (R2 measured), 39.14 (R2 adj), and 24.34 (R2 pred.). The results illustrated that the regression models efficiently presented the data. In contrast, a low R2 (measured) value of 13.74 with zero values for both R2 (adj) and R2 (pred.) was attributed to the shoot counts. The comparatively low R2 values for shoot counts reflect that the regression model did not find the association between input and output variables. Overall results illustrated a better impact of pretreatment and post-treatment systems on shoot regeneration and shoot length as compared to shoot counts.
The computations of predicted values for all output variables of in vitro regeneration of common beans were also performed by solving the reference equations (Eq. 7–9) to predict the impact of input variables on output variables. Results indicated a variable combination of pretreatment and post-treatment dose (BAP) for inducing maximum output values. Moreover, results also showed that a combination of 5 mg/L BAP × 0.995 mg/L BAP may yield 98.88% regeneration frequency (%) (Figure 4A). The maximum predicted shoot count (4.142 shoots) was attributed to the 11.061 mg/L BAP × 1.50 mg/L BAP combination (Figure 4B). However, the maximum predicted shoot length of 2.447 cm was attributed to the 20 mg/L BAP × 1.50 mg/L BAP combination (Figure 4C). The predicted values by response prediction models were close to the results attained in this study. The achieved results can be confirmed by checking the contour plots for shoot regeneration frequency (Figure 3A), shoot counts (Figure 3B), and shoot length (Figure 3C).
FIGURE 4. Response prediction of individual output variables on in vitro regeneration of common bean (A) regeneration, (B) shoot count, and (C) shoot length.
In addition to response prediction of individual output variables, the prediction response of multiple output variables was also constructed to optimize the dose concentration by considering two (reg × shoots and shoots × length) or considering all output variables (reg × shoot counts × shoot length). Results revealed that the combination of 5 mg/L × 1.5 mg/L BAP can be used for reg × shoot counts × shoot length (Figure 5A) and regeneration × shoot counts (Figure 5B). In contrast, 19.867 mg/L BAP × 1.5 mg/L BAP combination was predicted for shoot counts × shoot length variables (Figure 5C).
FIGURE 5. Multiple response prediction of output variables on in vitro regeneration of common bean (A) regeneration × shoots × length, (B) regeneration × shoots, and (C) shoots × length.
The R2 and MSE performance metrics were used to predict the shoot count, shoot length, and regeneration (Table 3). Results exhibited variable R2 values of all output variables for all models. The MSE values indicate the error between the measured and predicted values and varied with each model. Comparison between the five models revealed the better performance of MLP with high R2 values for all outputs as compared to other models. However, the values differed for each output variable. Results further illustrated the clear relationship between the R2 and the MSE values. In general, high R2 values with low MSE values were recorded for all models. Results on shoot regeneration revealed the order of MLP (0.58 R2; 0.0596 MSE) > GP (0.49 R2; 0.0724 MSE) > SVR (0.44 R2; 0.0802 MSE) > RF (0.44 R2; 0.0803 MSE) > XGBoost (0.32 R2; 0.0966 MSE). Results on shoot counts were computed in order of MLP (0.22 R2; 0.0327 MSE) > SVR (0.11 R2; 0.0371 MSE) > RF (0.09 R2; 0.0380 MSE) > GP (0.06 R2; 0.0392 MSE) > XGBoost (0.01 R2; 0.0412 MSE). The performance of models on shoot count revealed the order of MLP (0.48 R2; 0.0258 MSE) > GP (0.35 R2; 0.0318 MSE) > RF (0.25 R2; 0.0367 MSE) > SVR (0.23 R2; 0.0377 MSE) > XGBoost (0.18 R2; 0.0404 MSE). Figure 6 presents the difference between the predicted and measured values. The horizontal axis refers to the samples while the vertical axis specifies the data collected from the models and the experimental study. The compatibility of the experimental results revealed the better performance of the MLP model for shoot regeneration, shoot counts, and shoot length (Figure 6). On the contrary, the XGBoost model exhibited the least compatibility between actual and predicted values.
FIGURE 6. The relationship between the prediction and actual values for (A) regeneration, (B) shoot length, and (C) shoot count.
A data visualization method was used with colors to indicate the relationship between two variables. In the heatmap, it was detected that there is a strong correlation between BAP and shoot length. The overall results displayed a negative correlation for regeneration (−0.67) and shoot counts (−0.25) with BAP (pretreatment) and a positive correlation between BAP (pretreatment) and shoot length (0.34). Results on BAP (post-treatment) revealed a positive correlation with all output variables. On the other hand, a negative correlation between regeneration and shoot length (−0.21) and a positive correlation between regeneration and shoot counts (0.49) were also observed (Figure 7). These results indicated the dependence of input factors on output variables.
The selection of proper explants is a prerequisite for establishing an in vitro regeneration protocol, especially for recalcitrant plants like edible legumes. The selection of nontraditional and novel explants is one of the possible and potential solutions to overcome the recalcitrant issue in plant tissue cultures (Wang et al., 2011). Plumular apice are a potent and highly efficient explant due to the presence of meristem. To date, researchers tested plumule or plumular apice explant for in vitro shoot regeneration of different edible legumes like pea (Molnár et al., 1999), chickpea (Aasim et al., 2013), peanut (Singh and Hazra, 2009; Day and Aasim, 2017), cowpea (Aasim et al., 2009), pigeon pea (Surekha et al., 2005), and lentils (Aasim, 2012). In all these studies, plumular apices were proved to be efficient for inducing high regeneration frequency with high shoot counts per explant. However, the major problem associated with the use of this potent explant is the isolation from the embryo without any damage due to its smaller size. Pretreatment or pulse treatment of mature (Aasim et al., 2009; Day and Aasim, 2017) or immature embryos (Aasim, 2012) with high cytokinin concentration significantly enhances the embryo size which in turn allows isolation of plumular apice explant properly without any damage (Özkan and Aasim, 2019).
Pretreatment of explants with a high dose of cytokinins or auxins for a certain period is more effective to induce more shoots and more rapid regeneration (Brar et al., 1999; Barpete et al., 2014; Kumari et al., 2017; Özkan and Aasim, 2019) due to the more active division of cells (meristematic cells) found in the explants. However, stunted shoots, heavy callus induction, and deformed shoots (vitrified or hyperhidric shoots) are some of the common and negative features associated with the pretreatment (Aasim et al., 2011a). The pretreatment and post-treatment medium, treatment time, plant, and explants (Day and Aasim, 2017) are some of the factors that regulate the whole regeneration process. The manipulation of triggers (inputs), epigenetic and transcriptional cellular responses to the triggers, and molecules stem cell niche (Sugimoto et al., 2019) lead to nondeterministic and nonlinear developmental patterns in the plant’s cells and tissues (Prasad and Gupta, 2008).
In vitro regeneration is the mainstay of in vitro-based breeding methods, and optimization of input variables for the application of modern biotechnological techniques is highly demanding in the modern era of genome editing. The final output is generally analyzed and interpreted by traditional statistical software programs with the aid of tests like least significant difference test, Duncan’s multiple range test, Tukey’s honestly significant difference test, etc. (Ayuso et al., 2019). These models are not sufficient for the exact prediction of input combinations for the desired output variables. In recent years, modern computer-based software and models have been documented for the exact prediction and validation of the results. The prediction methodologies are divided into three major groups: regression equations, mathematical equations, and computer-based software (Askari et al., 2021). Among these, computer-based software models and simulation programs are gaining popularity with high acceptability by researchers to predict data with more accuracy (Kirtis et al., 2022).
RSM is a computer-based model, used for optimizing and predicting output variables using more than two input variables (Abbasi et al., 2016; Managamuri et al., 2019; Askari et al., 2021; Slimani et al., 2021). The advantage of using contour plots is the distribution of attained results into different subunits, which enables to specify the input variables for the desired output variable (Aasim et al., 2022). RSM predicted the optimal pretreatment and post-treatment BAP concentrations for inducing maximum shoot regeneration frequency, shoot counts and shoot length by estimating the R2 (measured), R2 (Adj.), and R2 (pred.) values of output variables. Furthermore, RSM successfully predicted the input variables by considering individual or multiple output variables. The use of surface plots and contour plots by RSM also clearly illustrated the impact of pretreatment and post-treatment doses of BAP on in vitro regeneration output variables of common bean. The use of RSM in plant or agricultural sciences is limited. However, RSM has been employed successfully for predicting the optimal conditions for in vitro regeneration and secondary metabolite production of different plants (Bansal et al., 2017; Premkumar et al., 2020; Slimani et al., 2021).
Results on pretreatment dose revealed a negative impact on shoot regeneration frequency and shoot count. Investigation of previous studies on pretreatment with cytokinin discerned the variable impact on shoot regeneration with both positive and negative impacts depending on the genotype, cytokinin type, and concentration. The study on peanuts revealed 100% shoot regeneration with more shoot counts from plumular apices preconditioned with 20 mg L−1 BAP as compared to 10 mg L−1 BAP (Day and Aasim, 2017). In a similar manner, other studies on cowpea (Brar et al., 1999), Pongamia pinnata (Belide et al., 2010), and Sophora tonkinensis (Jana et al., 2013) also illustrated the positive impact of pretreatment with cytokinin on shoot regeneration. On the other hand, relatively low regeneration from preconditioned explants has also been documented in peanuts (Akasaka et al., 2000; Matand et al., 2013). The results revealed a decreased shoot count pattern with enhanced pretreatment concentration of BAP. On the contrary, a high concentration of pretreatment dose yielded longer shoots. Results illustrated that shoot counts and shoot length are associated with BAP concentration and other factors like genotype. A previous study on lentils using preconditioned plumular apices explants yielded relatively more shoot counts and shoot length compared to nonconditioned plumular apices explants (Aasim, 2012).
A post-treatment medium enriched with low cytokinin concentration is highly significant and regulates the in vitro regeneration from pretreated explants. Results revealed high shoot regeneration frequency, shoot counts, and shoot length from pretreatment and post-treatment of BAP and confirmed the results achieved in chickpea (Aasim et al., 2011b) and lentils (Aasim, 2012). However, the investigation of previous studies clearly illustrated the significance of the correlation between PGRs type and concentration of both pretreatment and post-treatment medium, explant, and genotype (Tang et al., 2012; Kumari et al., 2017) on in vitro shoot regeneration. The results confirmed the significance of BAP concentration in the pretreatment and post-treatment medium on in vitro shoot count and shoot length of common bean. However, both parameters generated maximum output at a different combination of pretreatment × post-treatment BAP concentration. Previous studies on chickpea and lentils also exhibited a different combination of pretreatment × post-treatment BAP concentration. In chickpea, maximum shoot counts with shorter shoots were associated with high BAP in the post-treatment medium (Aasim et al., 2013). Vice versa, minimum shoot counts with longer shoots of lentils were documented from low BAP concentration in the post-treatment medium (Aasim, 2012). Shoot length is another important factor and maximum shoot length was documented at the high pretreatment × post-treatment combination used in this study. The results are contrary to the findings in peanuts, where shoot length gradually decreased with elevated BAP concentration in the post-treatment medium (Day and Aasim, 2017). Overall results revealed that pretreatment and post-treatment doses of BAP exerted a clear impact on in vitro shoot regeneration of common beans as confirmed in other studies (Jahan et al., 2011; Özkan and Aasim, 2019).
In vitro rooting of in vitro regenerated shoot is an important step to establishing a successful in vitro regeneration protocol for recalcitrant plants. The availability of higher cytokinin concentration in the culture medium is generally supposed to be inhibitive for inducing in vitro rooting. Previous studies on the use of pretreatment or post-treatment medium in other crops revealed no negative impact on in vitro rooting (Aasim et al., 2009; Day and Aasim, 2017; Özkan and Aasim, 2020), and this study also support their findings and achieved 100% rooting. After successful rooting, rooted plants transferred to pots failed to adopt and a very low frequency of plants survived possibly due to awaiting plants in the rooting medium for a long time, which resulted in damaged roots and ultimately affected the survival percentage.
In recent years, ML and ANN models have been successfully employed in plant tissue culture studies for optimizing different input variables like a basal medium (Alanagh et al., 2014; Arab et al., 2016; Arab et al., 2018), PGR types, concentration for in vitro regeneration (Kirtis et al., 2022), somatic embryogenesis (Niazian et al., 2017), callogenesis (Niazian et al., 2018), in vitro sterilization (Hesami et al., 2019; Aasim et al., 2022), and in vitro induced double haploid production (Niazian and Shariatpanahi, 2020). The detailed investigation of these studies revealed the use of different performance metrics like R2, MSE, RMSE, MAE, etc. to validate different ML and ANN models. In this study, five different ML models including the ANN model were used for optimizing and predicting the results. Results divulged the variable response of all tested models to the target output variable. The best model for all parameters was found to be MLP. However, the RF model ranked second for shoot regeneration and shoot count, and the GP model for shoot length. The results confirmed the previous findings by researchers in plant tissue culture studies. An investigation of ML models revealed that the prediction of the model is dependent on inputs, target outputs, and the type of model used (Hesami et al., 2019; Salehi et al., 2020, 2021; Kirtis et al., 2022).
The performance of all the tested models was validated by computing R2 and MSE scores. Relatively high R2 values for shoot regeneration and low R2 values were recorded for the shoot length. A detailed investigation of ML models in plant tissue culture studies revealed the variable R2 values for different output variables like R2 = 0.94 (Hesami et al., 2019), R2 = 0.56–0.85 (Salehi et al., 2020), and R2 = 0.70 (Hesami and Jones, 2021) and 0.98–1.0 (Kirtis et al., 2022). The R2 values obtained in this study are relatively less than those of the previous findings but still validated the results in an efficient way. A low R2 does not reflect the poor performance of the experiment, but rather reflects the low compatibility between input and output variables. High R2 values reflect the high compatibility between the input and output variables, and they are obtained when the difference between the mean of the measured values and the predicted values is bigger than the difference between the actual and predicted values. The single performance metric does not predict or validate the results accurately, and therefore more than two performance metrics are generally considered for ML modeling. MSE is another powerful performance metric that reflects the error between the actual and predicted values. High MSE values depict the high error and vice versa. The results on MSE values for all output variables exhibited very low values for all the tested models, which reflects the low error between the actual and predicted values (Kirtis et al., 2022).
The development of a successful in vitro regeneration protocol for the common bean is extremely crucial for the application of modern biotechnological techniques for its improvement. The developed protocol can be employed for the application of in vitro biotechnological techniques like genetic transformation and in vitro polyploidy induction for its enhancement. Application of ML and ANN models depicted better performance of the MLP model as compared to other models for better prediction and optimization of all output variables. The results achieved in this study proved that ML models are powerful tools to analyze the data and optimize the complex conditions irrespective of the variable inputs, outputs, and responses of models. The accomplished results can be effectively employed for the prediction and optimization of plant tissue culture protocols used for breeding purposes in the future.
The original contributions presented in the study are included in the article/Supplementary Material, further inquiries can be directed to the corresponding author.
MA (Lab Research Coordination, Response Surface Methodology Analysis, and Article Writing), RK (ML and ANN Analysis and Article Writing), ZM (Response Surface Methodology), SA (Statistical Analysis and Graphical Presentation), MN and AB (Conducting Research and Article Writing), FB (Research Coordination, Article Writing, and Control), TK (Research Coordination), YC (Editing, Reviewing the Article, and Providing Funds), VC (Research Coordination), EH (Article Writing and Editing), and RH (Research coordination, Reviewing, and Editing).
Basic Science Research Program supported this research through the National Research Foundation of Korea (NRF), funded by the Ministry of Education (2019R1A6A1A11052070).
The authors declare that the research was conducted in the absence of any commercial or financial relationships that could be construed as a potential conflict of interest.
All claims expressed in this article are solely those of the authors and do not necessarily represent those of their affiliated organizations, or those of the publisher, the editors, and the reviewers. Any product that may be evaluated in this article, or claim that may be made by its manufacturer, is not guaranteed or endorsed by the publisher.
The Supplementary Material for this article can be found online at: https://www.frontiersin.org/articles/10.3389/fgene.2022.897696/full#supplementary-material
Aasim, M., Day, S., Rezaei, F., and Hajyzadeh, M. (2013). Multiple Shoot Regeneration of Plumular Apices of Chickpea. Turk. J. Agric. For. 37, 33–39. doi:10.3906/tar-1204-3810.3906/biy-1207-44
Aasim, M., Day, S., Rezael, F., Hajyzadeh, M., Mahmud, S. T., and Ozcan, S. (2011b). In Vitro shoot Regeneration from Preconditioned Explants of Chickpea (Cicer Arietinum L.) Cv. Gokce. Afr. J. Biotechnol. 10, 2020–2023. doi:10.5897/AJB11.3214
Aasim, M., Katırcı, R., Akgur, O., Yildirim, B., Mustafa, Z., Nadeem, M. A., et al. (2022). Machine Learning (ML) Algorithms and Artificial Neural Network for Optimizing In Vitro Germination and Growth Indices of Industrial Hemp (Cannabis Sativa L.). Industrial Crops Prod. 181, 114801. doi:10.1016/j.indcrop.2022.114801
Aasim, M., Khawar, K. M., and Özcan, S. (2009). In Vitro micropropagation from Plumular Apices of Turkish Cowpea (Vigna Unguiculata L.) Cultivar Akkiz. Sci. Hortic. 122, 468–471. doi:10.1016/j.scienta.2009.05.023
Aasim, M. (2012). Micropropagation of Lentil (Lens Culinaris Medik.) Using Pulse Treatment of Immature Plumular Apices. Pak. J. Agri. Sci. 49, 149–154.
Aasim, M., Sahin-Demirbag, N., Khawar, M., Kendir, H., and Özcan, S. (2011a). Direct Axillary Shoot Regeneration from the Mature Seed Explant of the Hairy Vetch (Vicia Villosa Roth). Arch. Biol. Sci. Belgra 63, 757–762. doi:10.2298/abs1103757a
Abbasi, Z., Hooshyar, S., and Bagherieh-Najjar, M. B. (2016). Improvement of Callus Production and Shoot Regeneration Using Various Organs of Soybean (Glycine Max L. Merr) by Response Surface Methodology. Vitro Cell.Dev.Biol.-Plant 52, 537–545. doi:10.1007/s11627-016-9778-1
Aggarwal, C. C. (2018). Neural Networks and Deep Learning, 10. Switzerland AG: Springer, 978–983. doi:10.1007/978-3-319-94463-0
Akasaka, Y., Daimon, H., and Mii, M. (2000). Improved Plant Regeneration from Cultured Leaf Segments in Peanut (Arachis hypogaea L.) by Limited Exposure to Thidiazuron. Plant Sci. 156, 169–175. doi:10.1016/s0168-9452(00)00251-x
Alanagh, E. N., Garoosi, G.-a., Haddad, R., Maleki, S., Landín, M., and Gallego, P. P. (2014). Design of Tissue Culture Media for Efficient Prunus Rootstock Micropropagation Using Artificial Intelligence Models. Plant Cell Tiss. Organ Cult. 117, 349–359. doi:10.1007/s11240-014-0444-1
Arab, M. M., Yadollahi, A., Eftekhari, M., Ahmadi, H., Akbari, M., and Khorami, S. S. (2018). Modeling and Optimizing a New Culture Medium for In Vitro Rooting of G×N15 Prunus Rootstock Using Artificial Neural Network-Genetic Algorithm. Sci. Rep. 8, 9977–10018. doi:10.1038/s41598-018-27858-4
Arab, M. M., Yadollahi, A., Shojaeiyan, A., and Ahmadi, H. (2016). Artificial Neural Network Genetic Algorithm as Powerful Tool to Predict and Optimize In Vitro Proliferation Mineral Medium for G × N15 Rootstock. Front. Plant Sci. 7, 1526. doi:10.3389/fpls.2016.01526
Asfaw, A., Ambachew, D., Shah, T., and Blair, M. W. (2017). Trait Associations in Diversity Panels of the Two Common Bean (Phaseolus vulgaris L.) Gene Pools Grown under Well-Watered and Water-Stress Conditions. Front. Plant Sci. 8, 733. doi:10.3389/fpls.2017.00733
Askari, M., Abbaspour-Gilandeh, Y., Taghinezhad, E., El Shal, A. M., Hegazy, R., and Okasha, M. (2021). Applying the Response Surface Methodology (RSM) Approach to Predict the Tractive Performance of an Agricultural Tractor during Semi-deep Tillage. Agriculture 11, 1043. doi:10.3390/agriculture11111043
Ayuso, M., García-Pérez, P., Ramil-Rego, P., Gallego, P. P., and Barreal, M. E. (2019). In Vitro culture of the Endangered Plant Eryngium Viviparum as Dual Strategy for its Ex Situ Conservation and Source of Bioactive Compounds. Plant Cell Tiss. Organ Cult. 138, 427–435. doi:10.1007/s11240-019-01638-y
Bansal, M., Sudhakara Reddy, M., and Kumar, A. (2017). Optimization of Cell Growth and Bacoside-A Production in Suspension Cultures of Bacopa Monnieri (L.) Wettst. Using Response Surface Methodology. Vitro Cell.Dev.Biol.-Plant 53, 527–537. doi:10.1007/s11627-017-9847-0
Barpete, S., Aasim, M., Khawar, K. M., Özcan, S. F., and Özcan, S. (2014). Preconditioning Effect of Cytokinins on In Vitro Multiplication of Embryonic Node of Grass Pea (Lathyrus Sativus L.) Cultivar Gürbüz. Turk. J. Biol. 38, 485–492. doi:10.3906/biy-1312-94
Belide, S., Rao Sajjalaguddam, R., and Paladugu, A. (2010). Cytokinin Preconditioning Enhances Multiple Shoot Regeneration in Pongamia Pinnata (L.) Pierre - a Potential, Non-edible Tree Seed Oil Source for Biodiesel. Electron Journ Biotechnol 13, 3–4. doi:10.2225/vol13-issue6-fulltext-5
Bitocchi, E., Nanni, L., Bellucci, E., Rossi, M., Giardini, A., Zeuli, P. S., et al. (2012). Mesoamerican Origin of the Common Bean ( Phaseolus vulgaris L.) Is Revealed by Sequence Data. Proc. Natl. Acad. Sci. U.S.A. 109, E788–E796. doi:10.1073/pnas.1108973109
Blair, M. W., Cortés, A. J., Farmer, A. D., Huang, W., Ambachew, D., Penmetsa, R. V., et al. (2018). Uneven Recombination Rate and Linkage Disequilibrium across a Reference SNP Map for Common Bean (Phaseolus vulgaris L.). PloS one 13, e0189597. doi:10.1371/journal.pone.0189597
Brar, M. S., Al-Khayri, J. M., Morelock, T. E., and Anderson, E. J. (1999). Genotypic Response of Cowpea Vigna Unguiculata (L.) to In Vitro Regeneration from Cotyledon Explants. Vitro Cell.Dev.Biol.-Plant 35, 8–12. doi:10.1007/s11627-999-0002-4
Campa, A., Murube, E., and Ferreira, J. J. (2018). Genetic Diversity, Population Structure, and Linkage Disequilibrium in a Spanish Common Bean Diversity Panel Revealed through Genotyping-By-Sequencing. Genes 9, 518. doi:10.3390/genes9110518
Castillo, B. M., Rodriguez de la O, J. L., Mascorro Gallardo, J. O., and Iturriaga, G. (2015). In Vitro plants of Common Bean (Phaseolus vulgaris L.) Obtained by Direct Organogenesis. J. Agric. Sci. 7, 169–179. doi:10.5539/jas.v7n11p169
Chen, T., and Guestrin, C. (2016). “Xgboost: A Scalable Tree Boosting System,” in Proceedings of the 22nd acm sigkdd international conference on knowledge discovery and data mining, San Francisco, CA, August 13–17, 2016, 785–794. doi:10.1145/2939672.2939785
Day, S., and Aasim, M. (2017). In Vitro Screening of Preconditioned Plumular Apices Explants of Peanut (Arachis Hypogeae) to Different Salts Concentration. Fresenius. Environ. Bull. 4671.
Earl, C., Belitz, M. W., Laffan, S. W., Barve, V., Barve, N., Soltis, D. E., et al. (2021). Spatial Phylogenetics of Butterflies in Relation to Environmental Drivers and Angiosperm Diversity Across North America. Iscience 24 (4), 102239. doi:10.1016/j.isci.2021.102239
Farhadi, S., Salehi, M., Moieni, A., Safaie, N., and Sabet, M. S. (2020). Modeling of Paclitaxel Biosynthesis Elicitation in Corylus Avellana Cell Culture Using Adaptive Neuro-Fuzzy Inference System-Genetic Algorithm (ANFIS-GA) and Multiple Regression Methods. PLoS ONE 15 (8), e0237478. doi:10.1371/journal.pone.0237478
García-Pérez, P., Lozano-Milo, E., Landín, M., and Gallego, P. P. (2020). Machine Learning Technology Reveals the Concealed Interactions of Phytohormones on Medicinal Plant In Vitro Organogenesis. Biomolecules 10 (5), 746. doi:10.3390/biom10050746
Hameg, R., Arteta, T. A., Landin, M., Gallego, P. P., and Barreal, M. E. (2020). Modeling and Optimizing Culture Medium Mineral Composition for In Vitro Propagation of Actinidia Arguta. Front. Plant Sci. 11, 2088. doi:10.3389/fpls.2020.554905
Hesami, M., and Jones, A. M. P. (2020). Application of Artificial Intelligence Models and Optimization Algorithms in Plant Cell and Tissue Culture. Appl. Microbiol. Biotechnol. 104, 9449–9485. doi:10.1007/s00253-020-10888-2
Hesami, M., and Jones, A. M. P. (2021). Modeling and Optimizing Callus Growth and Development in Cannabis Sativa Using Random Forest and Support Vector Machine in Combination with a Genetic Algorithm. Appl. Microbiol. Biotechnol. 105, 5201–5212. doi:10.1007/s00253-021-11375-y
Hesami, M., Naderi, R., and Tohidfar, M. (2020a). Introducing a Hybrid Artificial Intelligence Method for High-Throughput Modeling and Optimizing Plant Tissue Culture Processes: the Establishment of a New Embryogenesis Medium for chrysanthemum, as a Case Study. Appl. Microbiol. Biotechnol. 104 (23), 10249–10263. doi:10.1007/s00253-020-10978-1
Hesami, M., Naderi, R., Tohidfar, M., and Yoosefzadeh-Najafabadi, M. (2019). Application of Adaptive Neuro-Fuzzy Inference System-Non-Dominated Sorting Genetic Algorithm-II (ANFIS-NSGAII) for Modeling and Optimizing Somatic Embryogenesis of Chrysanthemum. Front. Plant Sci. 10, 869. doi:10.3389/fpls.2019.00869
Hesami, M., Naderi, R., Tohidfar, M., and Yoosefzadeh-Najafabadi, M. (2020b). Development of Support Vector Machine-Based Model and Comparative Analysis with Artificial Neural Network for Modeling the Plant Tissue Culture Procedures: Effect of Plant Growth Regulators on Somatic Embryogenesis of chrysanthemum, as a Case Study. Plant Methods 16 (1), 1–15. doi:10.1186/s13007-020-00655-9
Hu, Z., Jin, Y., Hu, Q., Sen, S., Zhou, T., and Osman, M. T. (2019). Prediction of Fuel Consumption for Enroute Ship Based on Machine Learning. IEEE Access 7, 119497–119505. doi:10.1109/ACCESS.2019.2933630
Jahan, A. A., Anis, M., and Aref, I. M. (2011). Preconditioning of Axillary Buds in Thidiazuron-Supplemented Liquid Media Improves In Vitro Shoot Multiplication in Nyctanthes arbor-tristis L. Appl. Biochem. Biotechnol. 163 (7), 851–859. doi:10.1007/s12010-010-9089-7
Jamshidi, S., Yadollahi, A., Ahmadi, H., Arab, M. M., and Eftekhari, M. (2016). Predicting In Vitro Culture Medium Macro-Nutrients Composition for Pear Rootstocks Using Regression Analysis and Neural Network Models. Front. Plant Sci. 7, 274. doi:10.3389/fpls.2016.00274
Jana, S., Sivanesan, I., and Jeong, B. R. (2013). Effect of Cytokinins on In Vitro Multiplication of Sophora Tonkinensis. Asian Pac. J. Trop. Biomed. 3, 549–553. doi:10.1016/S2221-1691(13)60111-2
Kami, J., Velásquez, V. B., Debouck, D. G., and Gepts, P. (1995). Identification of Presumed Ancestral DNA Sequences of Phaseolin in Phaseolus vulgaris. Proc. Natl. Acad. Sci. U.S.A. 92, 1101–1104. doi:10.1073/pnas.92.4.1101
Katirci, R., and Takci, H. (2021). Makine Öğrenmesi Kullanarak Krom Kaplama Örtme Gücünün Tahmin Edilmesi. Firat Univ. J. Eng. 33, 709–719. doi:10.35234/fumbd.950667
Kirtis, A., Aasim, M., and Katırcı, R. (2022). Application of Artificial Neural Network and Machine Learning Algorithms for Modeling the In Vitro Regeneration of Chickpea (Cicer Arietinum L.). PCTOC 2, 1–2. doi:10.1007/s11240-022-02255-y
Kumari, K., Lal, M., and Saxena, S. (2017). Enhanced Micropropagation and Tiller Formation in Sugarcane through Pretreatment of Explants with Thidiazuron (TDZ). 3 Biotech. 7, 1–4. doi:10.1007/s13205-017-0910-7
Mamidi, S., Rossi, M., Moghaddam, S. M., Annam, D., Lee, R., Papa, R., et al. (2013). Demographic Factors Shaped Diversity in the Two Gene Pools of Wild Common Bean Phaseolus vulgaris L. Heredity 110 (3), 267–276. doi:10.1038/hdy.2012.82
Managamuri, U., Vijayalakshmi, M., Krishna Ganduri, V. S. R., Rajulapati, S. B., and Poda, S. (2019). Assessment of Optimized Process Parameters for Superior Bioactive Metabolite Production by Nonomuraea Longicatena VSM-16 Using Response Surface Methodology. Jyp 11, 377–381. doi:10.5530/jyp.2019.11.77
Matand, K., Wu, N., Wu, H., Tucker, E., and Love, K. (2013). More Improved Peanut (Arachis hypogaea L.) Protocol for Direct Shoot Organogenesis in Mature Dry-Cotyledonary and Root Tissues. J. Biotech. Res. 5, 24. doi:10.5897/AJB10.823
Molnár, Z., Jenes, B., and Ördog, V. (1999). Genetic Transformation of Pea (Pisum Sativum L.) via Particle Bombardment. Stara Lesna, Slovak Republic: Recent Advances in Plant Biotechnology. September, 4-11.
Murashige, T., and Skoog, F. (1962). A Revised Medium for Rapid Growth and Bio Assays with Tobacco Tissue Cultures. Physiol. Plant 15, 473–497. doi:10.1111/j.1399-3054.1962.tb08052.x
Nadeem, M. A., Gündoğdu, M., Ercişli, S., Karaköy, T., Saracoğlu, O., HabyarimanaBaloch, E. F. S., et al. (2020b). Uncovering Phenotypic Diversity and DArTseq Marker Loci Associated with Antioxidant Activity in Common Bean. Genes 11, 36. doi:10.3390/genes11010036
Nadeem, M. A., Karaköy, T., Yeken, M. Z., Habyarimana, E., Hatipoğlu, R., Çiftçi, V., et al. (2020a). Phenotypic Characterization of 183 Turkish Common Bean Accessions for Agronomic, Trading, and Consumer-Preferred Plant Characteristics for Breeding Purposes. Agronomy 10, 272. doi:10.3390/agronomy10020272
Niazian, M., and Niedbała, G. (2020). Machine Learning for Plant Breeding and Biotechnology. Agriculture 10, 436. doi:10.3390/agriculture10100436
Niazian, M., Noori, S. A. S., Galuszka, P., Tohidfar, M., and Mortazavian, S. M. M. (2017). Genetic Stability of Regenerated Plants via Indirect Somatic Embryogenesis and Indirect Shoot Regeneration of Carum Copticum L. Industrial Crops Prod. 97, 330–337. doi:10.1016/j.indcrop.2016.12.044
Niazian, M., Sadat-Noori, S. A., Abdipour, M., Tohidfar, M., and Mortazavian, S. M. M. (2018). Image Processing and Artificial Neural Network-Based Models to Measure and Predict Physical Properties of Embryogenic Callus and Number of Somatic Embryos in Ajowan (Trachyspermum Ammi (L.) Sprague). Vitro Cell.Dev.Biol.-Plant 54, 54–68. doi:10.1007/s11627-017-9877-7
Niazian, M., and Shariatpanahi, M. E. (2020). In Vitro-based Doubled Haploid Production: Recent Improvements. Euphytica 216, 1–21. doi:10.1007/s10681-020-02609-7
Özkan, H., and Aasim, M. (2020). Comparison of In Vitro Regeneration Potential of Different Preconditioned and Nonconditioned Explants of Peanut (Arachis hypogaea L). J. Environ. Agric. Sci. 22, 11–19.
Özkan, H., and Aasim, M. (2019). Potential of Pretreated Explants of Peanut (Arachis Hypogeae Linn.) to Micropropagation under In Vitro Conditions. Pak. J. Agric. Sci. 56. doi:10.21162/PAKJAS/19.8262
Pavlov, A. V., Serdyuk, J. A., and Ustinov, A. B. (2019). Machine Learning and the Schrödinger Equation. J. Phys. Conf. Ser. 1236 (No. 1), 012050. doi:10.1088/1742-6596/1236/1/012050
Pedregosa, F., Varoquaux, G., Gramfort, A., Michel, V., Thirion, B., Grisel, O., et al. (2011). Scikit-learn: Machine Learning in Python. J Mach Learn Res12, 2825–2830.
Pepe, M., Hesami, M., Small, F., and Jones, A. M. P. (2021). Comparative Analysis of Machine Learning and Evolutionary Optimization Algorithms for Precision Micropropagation of Cannabis Sativa: Prediction and Validation of In Vitro Shoot Growth and Development Based on the Optimization of Light and Carbohydrate Sources. Front. Plant Sci. 12, 757869. doi:10.3389/fpls.2021.757869
Prasad, V. S. S., and Gupta, S. D. (2008). “Applications and Potentials of Artificial Neural Networks in Plant Tissue Culture,” in Plant Tissue Cult. Lett. (Dordrecht: Springer), 47–67. doi:10.1007/978-1-4020-3694-1_3
Premkumar, G., Karuppanapandian, T., Sureshpandian, C., Arumugam, N., Selvam, A., and Rajarathinam, K. (2020). Optimization of a Liquid Culture System for Shoot Regeneration and Achieving an Enriched Level of Scopadulcic Acid B in the Leaf Organ Cultures of Scoparia Dulcis L. By Response Surface Methodology. Vitro Cell.Dev.Biol.-Plant 56, 60–71. doi:10.1007/s11627-019-10037-z
Salehi, A., Mobarhan, M. A., Mohammadi, J., Shahsavarani, H., Shokrgozar, M. A., and Alipour, A. (2020). Efficient Mineralization and Osteogenic Gene Overexpression of Mesenchymal Stem Cells on Decellularized Spinach Leaf Scaffold. Gene 757, 144852. doi:10.1016/j.gene.2020.144852
Salehi, M., Farhadi, S., Moieni, A., Safaie, N., and Hesami, M. (2021). A Hybrid Model Based on General Regression Neural Network and Fruit Fly Optimization Algorithm for Forecasting and Optimizing Paclitaxel Biosynthesis in Corylus Avellana Cell Culture. Plant Methods 17, 1–13. doi:10.1186/s13007-021-00714-9
Sammut C., and Webb, G.I. (Editors) (2011). Encyclopedia of Machine Learning (New York, United States: Springer Science and Business Media). doi:10.1007/1978-0387-30164-8
Silva, T. S., de Freitas Souza, M., Maria da Silva Teófilo, T., Silva dos Santos, M., Formiga Porto, M. A., Martins Souza, C. M., Barbosa dos Santos, J., and Silva, D. V. (2019). Use of Neural Networks to Estimate the Sorption and Desorption Coefficients of Herbicides: A Case Study of Diuron, Hexazinone, and Sulfometuron-Methyl in Brazil. Chemosphere 236, 124333. doi:10.1016/j.chemosphere.2019.07.064
Singh, S., and Hazra, S. (2009). Somatic Embryogenesis from the Axillary Meristems of Peanut (Arachis hypogaea L.). Plant Biotechnol. Rep. . 3, 333-3–340. doi:10.1007/s11816-009-0107-6
Slimani, C., El Goumi, Y., Rais, C., El Ghadraoui, L., Benjelloun, M., and Lazraq, A. (2021). Optimization of Callogenesis/Caulogenesis Induction Protocol in Saffron Plant (Crocus Sativus L.) Using Response Surface Methodology. Biointerface Res. Appl. Chem. 12, 4731–4746. doi:10.33263/BRIAC124.47314746
Smola, A. J., and Schölkopf, B. (2004). A Tutorial on Support Vector Regression. Statistics Comput. 14, 199–222. doi:10.1023/B:STCO.0000035301.49549.88
Sugimoto, K., Temman, H., Kadokura, S., and Matsunaga, S. (2019). To Regenerate or Not to Regenerate: Factors that Drive Plant Regeneration. Curr. Opin. Plant Biol. 47, 138–150. doi:10.1016/j.pbi.2018.12.002
Surekha, C., Beena, M. R., Arundhati, A., Singh, P. K., Tuli, R., Dutta-Gupta, A., et al. (2005). Agrobacterium-mediated Genetic Transformation of Pigeon Pea (Cajanus Cajan (L.) Millsp.) Using Embryonal Segments and Development of Transgenic Plants for Resistance against Spodoptera. Plant Sci. 169, 1074–1080. doi:10.1016/j.plantsci.2005.07.011
Tang, Y., Chen, L., Li, X. M., Li, J., Luo, Q., Lai, J., et al. (2012). Effect of Culture Conditions on the Plant Regeneration via Organogenesis from Cotyledonary Node of Cowpea (Vigna Unguiculata L. Walp). Afr. J. Biotechnol. 11, 3270–3275. doi:10.5897/ajb11.3214
Van Rossum, G., and Drake, F. L. (1994). Python Reference Manual. Surrey, England: Network Theory LTD. doi:10.5555/1610526
Vanlauwe, B., Hungria, M., Kanampiu, F., and Giller, K. E. (2019). The Role of Legumes in the Sustainable Intensification of African Smallholder Agriculture: Lessons Learnt and Challenges for the Future. Agric. Ecosyst. Environ. 284, 106583. doi:10.1016/j.agee.2019.106583
Wang, X. J., Liu, S. T., Xia, H., Wan, S. B., Zhao, C. Z., and Li, A. Q. (2011). Peanut ('Arachis hypogaea L.') Omics and Biotechnology in China. Plant Omics 4, 339–349. doi:10.3316/informit.684496127565121
Yeken, M. Z., Nadeem, M. A., Karaköy, T., Baloch, F. S., and Çiftçi, V. (2019). Determination of Turkish Common Bean Germplasm for Morpho-Agronomic and Mineral Variations for Breeding Perspectives in Turkey. Kahramanmaraş Sütçü İmam Üniv. Tarım Doğa Derg. 22, 38–50. doi:10.18016/ksutarimdoga.vi.563740
Keywords: machine learning algorithms, artificial neural network, in vitro regeneration, plumular apices, coefficient of determination, mean squared error
Citation: Aasim M, Katirci R, Baloch FS, Mustafa Z, Bakhsh A, Nadeem MA, Ali SA, Hatipoğlu R, Çiftçi V, Habyarimana E, Karaköy T and Chung YS (2022) Innovation in the Breeding of Common Bean Through a Combined Approach of in vitro Regeneration and Machine Learning Algorithms. Front. Genet. 13:897696. doi: 10.3389/fgene.2022.897696
Received: 16 March 2022; Accepted: 03 June 2022;
Published: 24 August 2022.
Edited by:
Aamir Raina, Aligarh Muslim University, IndiaReviewed by:
Mohsen Hesami, University of Guelph, CanadaCopyright © 2022 Aasim, Katirci, Baloch, Mustafa, Bakhsh, Nadeem, Ali, Hatipoğlu, Çiftçi, Habyarimana, Karaköy and Chung. This is an open-access article distributed under the terms of the Creative Commons Attribution License (CC BY). The use, distribution or reproduction in other forums is permitted, provided the original author(s) and the copyright owner(s) are credited and that the original publication in this journal is cited, in accordance with accepted academic practice. No use, distribution or reproduction is permitted which does not comply with these terms.
*Correspondence: Faheem Shehzad Baloch, YmFsb2NoZmFoZWVtMTNAZ21haWwuY29t; Yong Suk Chung, eXNjaHVuZ0BqZWp1bnUuYWMua3I=
Disclaimer: All claims expressed in this article are solely those of the authors and do not necessarily represent those of their affiliated organizations, or those of the publisher, the editors and the reviewers. Any product that may be evaluated in this article or claim that may be made by its manufacturer is not guaranteed or endorsed by the publisher.
Research integrity at Frontiers
Learn more about the work of our research integrity team to safeguard the quality of each article we publish.