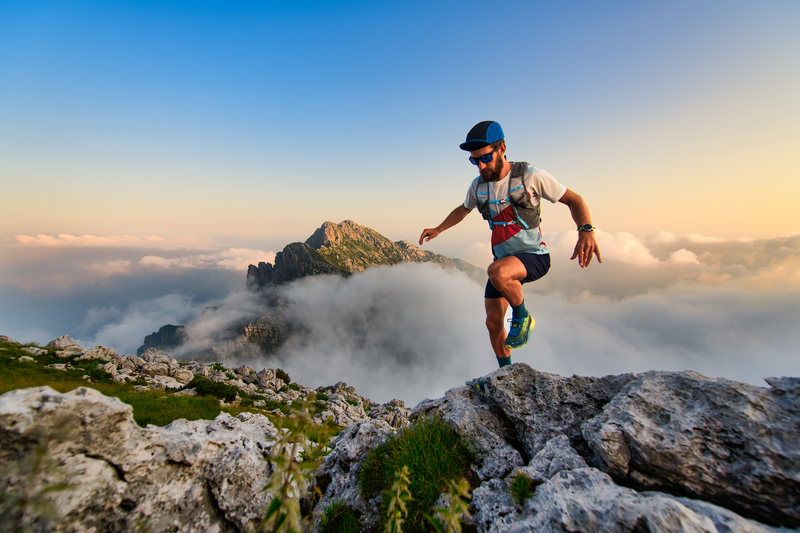
95% of researchers rate our articles as excellent or good
Learn more about the work of our research integrity team to safeguard the quality of each article we publish.
Find out more
ORIGINAL RESEARCH article
Front. Genet. , 25 May 2022
Sec. Computational Genomics
Volume 13 - 2022 | https://doi.org/10.3389/fgene.2022.889348
A high proportion of critically ill patients with coronavirus disease 2019 (COVID-19) experience thrombosis, and there is a strong correlation between anticoagulant therapy and the COVID-19 survival rate, indicating that common COVID-19 and thrombosis targets have potential therapeutic value for severe COVID-19.Gene expression profiling data were downloaded from Gene Expression Omnibus (GEO), and common differentially expressed genes (co-DEGs) were identified. The potential biological functions of these co-DEGs were explored by functional enrichment analysis, and protein–protein interaction (PPI) networks were constructed to elucidate the molecular mechanisms of the co-DEGs. Finally, hub genes in the co-DEG network were identified, and correlation analysis was performed.We identified 8320 upregulated genes and 7651 downregulated genes from blood samples of COVID-19 patients and 368 upregulated genes and 240 downregulated genes from blood samples of thrombosis patients. The enriched cellular component terms were mainly related to cytosolic ribosomes and ribosomal subunits. The enriched molecular function terms were mainly related to structural constituents of ribosomes and electron transfer activity. Construction of the PPI network and identification of hub genes ultimately confirmed that RPS7, IGF1R, DICER1, ERH, MCTS1, and TNPO1 were jointly upregulated hub genes, and FLNA and PXN were jointly downregulated hub genes.The identification of novel potential biomarkers provides new options for treating COVID-19-related thrombosis and reducing the rate of severe COVID-19.
The coronavirus disease 2019 (COVID-19) pandemic has had a significant impact on global public health, social interactions and the economy (Han et al., 2020; Global Burden of Disease 2020 Health Financing Collaborator Network, 2021; Lal et al., 2021; Mcintyre et al., 2022). As of February 2022, nearly 400 million people have been diagnosed with COVID-19 worldwide, and more than 5.7 million people have died. COVID-19 can induce different complications and affect different organs (Drake et al., 2021; Wang et al., 2021), mainly the respiratory tract, heart, gastrointestinal tract (Serban et al., 2021; Bulte et al., 2022), central nervous system (Sriwastava et al., 2021), liver, and kidney (Gross et al., 2020). Elderly individuals and patients with underlying diseases are prone to severe cases, and their mortality rates are significantly higher than those of other populations (Ghasemiyeh et al., 2021). Patients who die from COVID-19 infection in the late stage of disease progression can develop acute respiratory distress syndrome (ARDS) (Zangrillo et al., 2022), cytokine storm syndrome (CSS) (Yongzhi, 2021), acute kidney injury (AKI) (Ronco et al., 2020), septic shock (Wei et al., 2021), multiple organ failure (MOF) (Devaux et al., 2020) and cardiac arrest (Shao et al., 2020), resulting in death. Especially in patients with severe COVID-19, cardiac manifestations such as arrhythmia, myocardial infarction, acute heart failure and cardiogenic shock are common and are associated with increased mortality (Bangalore et al., 2020; Lala et al., 2020). Researchers have proposed various hypotheses about the direct and indirect mechanisms of COVID-19-related heart damage, but the exact mechanism is still unclear (Chung et al., 2021). The earlier theory that COVID-19 heart damage is due to acute viral myocarditis has been disproved by the lack of cases meeting the histological criteria for myocarditis (Halushka and Vander Heide, 2021). Subsequently, further studies have suggested that coagulation abnormalities, including thrombotic microangiopathy and complications of venous and arterial thromboembolism, are common in critically ill patients with COVID-19 (Levi et al., 2020). The pathological results suggest that the most common pathological cause of myocardial cell necrosis is the occurrence of microthrombi (Pellegrini et al., 2021). Moreover, a series of studies have also found that severe acute respiratory syndrome coronavirus 2 (SARS-CoV-2) infection promotes hypercoagulability, and there is a strong correlation between the use of anticoagulants and COVID-19 survival (Nadkarni et al., 2020; Fröhlich et al., 2021; Lawler et al., 2021). A series of studies have confirmed that COVID-19 is associated with venous thromboembolic events, including pulmonary embolism, lower extremity deep venous thrombosis and cerebral venous sinus thrombosis, and there is a correlation between thrombosis and mortality in patients with COVID-19 (Rosovsky et al., 2020). Some studies have even found a 51.5-fold increased risk of in-hospital mortality in patients with D-2mer ≥2.0 μg/ml (Zhang L. et al., 2020), but the actual prevalence and characteristics of venous thromboembolism (VTE) remain unclear (Sastry et al., 2022). The incidence of VTE reported by different studies varies significantly (ranging from 0.7% to 57%) due to differences in disease severity and diagnostic imaging techniques used (Cui et al., 2020; Klok et al., 2020; Lodigiani et al., 2020; Suh et al., 2021). Even with anticoagulant prophylaxis, the incidence of VTE in COVID-19 patients remains high and is much higher than that in non-COVID patients receiving thromboprophylaxis (Lodigiani et al., 2020). Cytokine storms were initially believed to be the main factor for COVID-19 thrombosis (Lowenstein and Solomon, 2020), antiphospholipid antibodies were later detected in COVID-19 (Zhang Y. et al., 2020; Zuo et al., 2020), and the presence of prothrombotic antibodies also constituted one of the risk factors. Studies have also suggested that elevated levels of neutrophil extracellular traps are closely related to thrombotic events (Zuo et al., 2021), but their overall role in COVID-19-related thrombosis remains unclear (Bonaventura et al., 2021). At present, most reports on thrombosis in patients with COVID-19 are case reports (Achim et al., 2022; Bleiziffer et al., 2022; Jeckelmann et al., 2022; Russell et al., 2022; Takasu et al., 2022) and some observational studies (Maier et al., 2020). Even after strict ethical review, only a small number of large studies have confirmed the relationship between the use of anticoagulants and the survival rate of COVID-19 (Lawler et al., 2021). There is a good correlation. Therefore, it is still necessary to obtain a theoretical basis for the mechanism of COVID-19 thrombosis. In summary, exploring targets that can be used to treat both COVID-19 and thrombosis has theoretical guiding significance for the assessment and treatment of COVID-19 patients. However, there are various difficulties in clinical research on COVID-19, including ethical requirements and limited patient contact. The use of public databases solves the above problems. Further analysis of relevant datasets from public databases we can be used to identify and develop reliable biomarkers and treatment strategies for thrombosis in patients with COVID-19 and thus to better understand the mechanism of thrombosis in patients with COVID-19 and identify the corresponding targets. Such studies will promote follow-up drug research and development. We identified 8320 upregulated genes and 7651 downregulated genes from blood samples of COVID-19 patients and 368 upregulated genes and 240 downregulated genes from blood samples of thrombosis patients. We identified hub genes that may contribute to the evaluation and treatment of COVID-19-related thrombosis.
The GEO database was searched with “COVID-19” and “thrombosis” as keywords, and we searched using keywords and restricted the screening conditions to “Homo sapiens” and “Expression profiling by array” and limited the sample to peripheral blood. After reading the original studies on the data sets that met the screening conditions individually, we focused on the experimental design and methodology. The data set that met the principle of randomized control and did not violate ethical guidelines was taken as the research object. After screening each dataset, GSE19151 and GSE164805 were ultimately selected for further research. The GSE19151 dataset included 70 adults who had used warfarin one or more times for venous thromboembolism and 63 healthy controls. This dataset excluded patients with antiphospholipid syndrome and cancer and was generated using the GPL571 [HG-U133A_2] Affymetrix Human Genome U133A 2.0 Array. The GSE164805 dataset included 10 patients diagnosed with COVID-19 and 5 healthy controls and was generated using the GPL26963 Agilent-085982 Arraystar Human LncRNA V5 microarray. R software (version 4.0.2, http://r-project.org/) was used to download the two datasets and relevant information. The raw data were processed through the “affy” (Gautier et al., 2004) package, and the RMA algorithm was used to perform background correction and data normalization. The normalized results were visualized using boxplots (Supplementary Figures S1, S2).
The “limma” package of R software was used to analyze the disease group and healthy control group of the two datasets, and the differentially expressed genes (DEGs) between the two groups were obtained. To identify the most meaningful DEGs, the following limits were used to determine which DEGs would be retained: (1) | log2 (fold-change) | > 0.5 and (2) adjusted p < 0.05. In addition, adjusted p < 0.05 was considered to indicate a significant difference (Ritchie et al., 2015; Shankar et al., 2020).
Gene Ontology (GO) functional analysis (including cellular component [CC], biological process [BP], and molecular function [MF] analysis) is a powerful bioinformatics tool for classifying gene expression and associated properties (Ashburner et al., 2000). Kyoto Encylopedia of Genes and Genomes (KEGG) pathway analysis was used to determine which cellular pathways may be related to the DEGs (Altermann and Klaenhammer, 2005). The “clusterProfiler" (Yu et al., 2012) package of R software was used for enrichment analysis of the DEGs, the “org.Hs.eg.db” package was used for ID conversion, and the “Goplot” package was used to calculate Z scores (Walter et al., 2015). The results are visually presented as chord graphs and tables.
The co-DEGs of the two datasets were analyzed using a Venn diagram, and the results were visualized using the “ggplot2” (Rosenthal et al., 2019) package of R software. The co-upregulated genes and co-downregulated genes were subsequently uploaded to the Search Tool for the Retrieval of Interacting Genes/Proteins (STRING, http://string-db.org/) database for protein–protein interaction (PPI) network construction, and 0.4 was used as the minimum required interaction score for analysis. The results were input into Cytoscape (Shannon et al., 2003) to construct and visualize the molecular interaction network.
Hub genes are the DEGs that show the highest association with other network components, and they are important nodes in the PPI network. The Cyto-Hubba (Chin et al., 2014) plug-in in Cytoscape software was used to determine the hub genes in the PPI network. To reduce the selection bias caused by different algorithms, the MCC, DMNC, MNC, and EPC algorithms were used to determine the hub genes, and the final results were confirmed and visualized with a Venn diagram.
The selected hub genes were analyzed by the PANTHER(Mi et al., 2019) classification system (http://pantherdb.org/), and the basic classification of each gene was obtained. Then, the Human Protein Atlas (Uhlen et al., 2019) (HPA) (https://www.proteinatlas.org/) was used to explore the expression profile of each hub gene in human immune cells. Subsequently, the Deeply Integrated human Single-Cell Omics (DISCO) database (Li et al., 2022) (https://www.immunesinglecell.org/) was used to clarify the expression level of the hub genes in all blood cells of COVID-19 patients. Finally, the “clusterProfiler” (Yu et al., 2012) package of R software was used for the enrichment analysis of the hub genes, and the “ggplot2” package of R software was used to visually present the results of the enrichment analysis. The results are displayed in a histogram and table.
The “limma” package of R software was used to identify the DEGs of the two datasets. In the GSE19151 dataset, included 70 adults who had used warfarin one or more times for venous thromboembolism and 63 healthy controls, the number of genes after data processing was 21144, including 368 upregulated genes that met the log2 (FC) > 0.5 and adjusted p < 0.05 criteria and 240 downregulated genes that met the log2 (FC) < −0.5 and adjusted p < 0.05 criteria. In the GSE164805 dataset, included 10 patients diagnosed with COVID-19 and 5 healthy controls, the number of genes after data processing was 60494, including 8320 upregulated genes meeting the log2 (FC) > 0.5 and adjusted p < 0.05 criteria and 7651 downregulate genes meeting the log2 (FC) < −0.5 and adjusted p < 0.05 criteria. A volcano plot was generated using the “ggplot2” package to visualize the DEGs (Figure 1).
FIGURE 1. DEGs in the two datasets. (A) Volcano plot of GSE19151; Red represents upregulated genes, blue represents downregulated genes, and gray represents no significantly expressed genes. (B) Volcano plot of GSE164805. The criteria for statistically significant difference of DEGs was adjusted |log2(FC)|>0.5 and p.adj<0.05 in expression.
The main enriched BP terms of the DEGs in the venous thrombosis dataset were SRP-dependent cotranslational protein targeting to membrane (GO:0006614) and cotranslational protein targeting to membrane (GO:0006613). The main enriched CC terms were cytosolic ribosome (GO:0022626) and ribosomal subunit (GO:0044391), and the main enriched MF terms were structural constituent of ribosome (GO:0003735) and electron transfer activity (GO:0009055). The KEGG pathway analysis mainly showed enrichment of the terms ribosome (hsa03010) and cardiac muscle contraction (hsa04260). For the DEGs identified from the COVID-19 dataset, the enriched BP terms mainly included nuclear-transcribed mRNA catabolic process, nonsense-mediated decay (GO:0000184) and translational initiation (GO:0006413), the enriched CC terms mainly included cytosolic ribosome (GO:0022626) and ribosomal subunit (GO:0044391), and the enriched MF term was structural constituent of ribosome (GO:0003735). The KEGG pathway enrichment analysis mainly identified the terms leishmaniasis (hsa05140) and graft-versus-host disease as enriched (hsa05332). For both datasets, the CC and MF terms were similar. All the results are presented in chord diagrams (Figure 2) and tables (Tables 1, 2).
FIGURE 2. GO terms and KEGG pathway enrichment. (A) Details of GO terms and KEGG pathway enrichment in GSE19151; The left side of the figure indicated that the genes were sorted according to logFC. Red represented up-regulation, blue represented down-regulation, and color depth represented change multiples. The right side of the figure indicates the order of enrichment analysis term according to the change of strength. There is a link between genes and term, indicating that they have a subordinate relationship. (B) Details of GO terms and KEGG pathway enrichment in GSE164805.
The “VennDiagram” package of R software was used to extract the co-DEGs of the two datasets (Figure 3C). A total of 103 co-upregulated genes and 106 co-downregulated genes were identified. The co-upregulated genes and co-downregulated genes were uploaded to the STRING database to construct a PPI network (Figures 3A,B), and the results were imported into Cytoscape software for further analysis. The PPI network of the co-upregulated genes included 59 nodes and 57 edges, and the PPI network of the co-downregulated genes included 82 nodes and 126 edges (Figure 4). Next, the Cyto-Hubba plugin was used to identify the hub genes. To reduce the selection bias caused by different algorithms, the MCC, DMNC, MNC, and EPC algorithms were used to extract the hub genes, and the “VennDiagram” package was used to merge the hub genes extracted by the four algorithms (Figure 5). Ultimately, RPS7, IGF1R, DICER1, ERH, MCTS1, and TNPO1 were identified as jointly upregulated hub genes, and FLNA and PXN were identified as jointly downregulated hub genes.
FIGURE 3. PPI network and Venn diagram. (A) A PPI network were constructed by co-upregulated genes. (B) A PPI network were constructed by co-downregulated genes. (C) The Venn diagram showed the co-DEGs of the two datasets.
FIGURE 4. PPI network of DEGs. (A) The PPI network of the co-upregulated genes included 59 nodes and 57 edges. (B) The PPI network of the co-downregulated genes included 82 nodes and 126 edges.
FIGURE 5. The hub genes were merged by the MCC, DMNC, MNC, and EPC algorithms. (A) RPS7, IGF1R, DICER1, ERH, MCTS1, and TNPO1 were jointly upregulated hub genes. (B) FLNA and PXN were identified as jointly downregulated hub genes.
The PANTHER (Mi et al., 2019) classification system was used to analyze the selected hub genes, and the basic classification of each gene was obtained. The results are listed in a table (Table 3). Complex interactions between innate immunity, platelet activation and coagulation lead to immune thrombosis. Immune thrombosis is an important host defense mechanism that limits the systematic transmission of pathogens through blood flow. However, abnormal activation of immune thrombosis can also lead to myocardial infarction, stroke and venous thromboembolism (Stark and Massberg, 2021). To explore the expression of the hub genes in human immune cells, the HPA database was used to analyze each hub gene (Figure 6). HPA RNA-seq data are displayed in different colors based on cell type, including B cells, T cells, natural killer (NK) cells, monocytes, granulocytes, dendritic cells and total peripheral blood mononuclear cells (PBMCs). The DISCO (Li et al., 2022) database was used annotate the single-cell sequencing data of blood cells from patients with COVID-19. The expression level of each hub gene in each cell is shown. The deeper the color is, the higher the expression of the gene in the cell (Figure 7).
FIGURE 6. Blood cell type expression overview shows RNA-seq data from internally generated Human Protein Atlas (HPA) data. Color-coding is based on blood cell type lineages. The resulting transcript expression values calculated as nTPM, resulting from the internal normalization pipeline for 18 immune cell types and total PBMC. (A) DICER1 (B) ERH (C) RPS7 (D) IGF1R (E) MCTS1 (F) TNP01 (G) PXN (H) FLNA.
FIGURE 7. The DISCO database was used annotate the single-cell sequencing data of blood cells from patients with COVID-19. The deeper the color is, the higher the expression of the gene in the cell. (A) DICER1 (B) ERH (C) RPS7 (D) IGF1R (E) MCTS1 (F) TNP01 (G) PXN (H) FLNA.
Functional enrichment analysis of the six coupregulated hub genes (RPS7, IGF1R, DICER1, ERH, MCTS1, and TNPO1) was performed. The results showed that the main enriched BP terms were the ribonucleoprotein complex assembly (GO: 0022618), viral gene expression (GO: 0019080) and translational initiation (GO: 0006413). The main enriched CC terms were the cytosolic part (GO: 0044445) and ribosomal subunit (GO: 0044391), and the main enriched MF term was peptide binding (GO: 0042277). The KEGG pathway analysis mainly showed enrichment of the terms adherens junction (hsa04520), melanoma (hsa05218) and longevity regulating pathway - multiple species (hsa04213). All results are displayed in a bubble diagram (Figure 8) and table (Table 4).
FIGURE 8. Functional enrichment analysis of the six co-upregulated hub genes. (A) Shows the results of biological process terms enriched by BP analysis; (B) Shows the results of biological process terms enriched by CC analysis; (C) Shows the results of biological process terms enriched by MF analysis; (D) Shows the enriched pathway by KEGG analysis. The coloured dots represent the p-value for that term, with red representing greater significance. The size of the dots represents the number of involved genes.
To explore whether the hub genes of COVID-19 and thrombosis are common targets caused by viral induction, we included a third-party data set for corresponding verification. Previous studies have confirmed that viral diseases induce platelet and neutrophil recruitment to the microvascular system to protect host cells from viral infection (Jenne et al., 2013). However, recent research has also shown that compared with that in patients with COVID-19 pneumonia, immunothrombotic vessel occlusion in the lungs is less prominent in patients with influenza pneumonia (Ackermann et al., 2020; Nicolai et al., 2021). We searched the GEO database with “SARS” as the key word, searched using keywords, restricted the screening conditions to “Homo sapiens” and “Expression profiling by array” and limited the sample to peripheral blood. After reading the original studies on the data sets that met the screening conditions individually, we focused on the experimental design and methodology. The data set that met the principle of randomized control and did not violate ethical guidelines was taken as the research object. Finally, GSE5972 was selected for further research. The GSE5972 data set included 50 SARS patients, regardless of age, sex or past medical history. Positive PCR or serum results confirmed that each patient was infected with SARS-CoV. Ten healthy volunteers, five men and five women, were recruited. The chip platform was GPL4387 UHNMAC Homo sapiens 19K Hu19Kv8. Using the aforementioned methodology, we performed background correction and data normalization on the GSE5972 data set and then used the “limma” package of R software to analyze the disease group and the healthy control group to identify DEGs. The inclusion criteria of DEGs were the same as those for the above two data sets. The DEGs of the GSE5972 and GSE19151 data sets were combined and analyzed, the co-DEGs were screened out, and a PPI network was constructed. Then, the hub genes were extracted by Cytoscape software. The final results showed that the upregulated hub genes were IFI44L, TNFSF10, RSAD2, ETFA and MDH1 and that the downregulated hub genes were PXN, MYH9, SPTAN1, TLN1, CXCR4, SUPT6H and ENO1. It can be seen from the results that the hub genes of COVID-19 and thrombosis are not the same as the hub genes of SARS-CoV infection and thrombosis, which also explains why there are many studies on the mechanism of virus-induced thrombosis (Boilard et al., 2014; Tripathi et al., 2014; Koupenova et al., 2019) but the specific mechanism of COVID-19 thrombosis is still unclear (Farkouh et al., 2022; Thomas and Scully, 2022; Vincent et al., 2022). In addition, there have been an increasing number of recent reports of thrombosis after COVID-19 vaccination (De Michele et al., 2022), all of which indicate the existence of characteristic factors of thrombosis in COVID-19, which deserves further exploration.
Thrombosis and the inflammatory response were once thought to be two independent physiological processes, but in recent years, studies have gradually found that there is a clear correlation between them. Activation of the coagulation system is part of the host response to pathogens and primarily serves to limit their spread in the bloodstream, which is achieved through interactions between innate immune cells and platelets, termed immunothrombosis (Engelmann and Massberg, 2013). Due to the COVID-19 pandemic, this normal physiological response has received more attention (Stark and Massberg, 2021). Histological analysis of pulmonary vessels in COVID-19 patients showed extensive thrombosis with microangiopathy, and the incidence of pulmonary capillary microthrombosis in COVID-19 patients was 9 times higher than that in influenza patients; the difference was statistically significant (Ackermann et al., 2020). Furthermore, data show that immune thrombosis is also a key indicator of the severity of COVID-19 (Nicolai et al., 2020). Preliminary studies in Wuhan, China, showed that most patients with COVID-19 who died of disease had coagulation disorders, characterized by prolonged prothrombin time and partial prothrombin kinase time, similar to the characteristics observed in patients with disseminated intravascular coagulation (Zhou et al., 2020). When pathogens invade the bloodstream and pose a lethal threat to the host, the body tries to control the infection by all available means, and viral diseases induce the recruitment of platelets and neutrophils to the microvasculature to protect host cells from viral infection (Jenne et al., 2013). The main cellular drivers of this process are platelets and innate immune cells, including neutrophils, monocytes and macrophages. In general, the response to viral disease should be similar; however, immune thrombotic vaso-occlusion was less prominent in patients with influenza-related pneumonia than in patients with COVID-19 (Nicolai et al., 2021). This finding illustrates that the immunothrombosis in COVID-19 is not just a general feature of viral infections but may also be a characteristic factor, suggesting that it is worthy of further exploration to reduce the disease severity and mortality of patients with COVID-19. Bioinformatics analysis enables us to understand the molecular mechanisms of disease initiation and progression, providing a new and effective method for identifying potential biomarkers and therapeutic targets for assessing and treating COVID-19 thrombosis. In this study, six co-upregulated genes, RPS7, IGF1R, DICER1, ERH, MCTS1, and TNPO1, and two co-downregulated genes, FLNA and PXN, were identified from COVID-19 and thrombosis datasets. Ribosomal protein S7 (rps7) is an essential component of the 40s subunit of the ribosome and is essential in the translation process and assembly of the ribosome (Fatica and Tollervey, 2002). Studies have shown that RPS7 regulates cell proliferation, the cell cycle and apoptosis through the PI3K/AKT and MAPK signaling pathways (Wang et al., 2013). However, whether RPS7 is specifically overexpressed in patients with COVID-19 with a high risk of thrombosis remains to be determined. Insulin-like growth factor 1 receptor (IGF1R) is a widely expressed membrane-bound tyrosine kinase receptor that can recognize two main ligands, IGF1 and IGF2. IGF1R expression on lymphocytes and macrophages can cause the proliferation of target cells and the production and activation of cytokines. Phosphorylation of IGF1R exacerbates inflammation, and its overexpression increases cytokine levels during influenza infection (Li et al., 2019). IGF1R has also recently been defined by some studies as a novel biomarker for predicting mortality in severe COVID-19 patients (Fraser et al., 2020). Dicer 1, ribonuclease III (DICER1) is a highly conserved RNase III ribonuclease that plays a key role in biological processes related to microRNAs (miRNAs). Recent studies have shown that DICER1 may maintain homeostasis during inflammation (Jiang et al., 2019). If the inflammatory response is out of balance, the crosstalk between inflammation and thrombosis can have serious consequences because not only does inflammation lead to thrombosis, but thrombosis in turn directly triggers inflammation. Thrombin cleaves fibrinogen and activates the cytokine IL-1α, providing a direct link between coagulation and inflammation. ERH mRNA splicing and mitosis factor (ERH) is a small protein that is highly conserved in eukaryotes. Though it was discovered nearly 30 years ago, its biological function is still largely unknown. It is considered to play a role in transcriptional regulation and the cell cycle. Although a series of studies have found that ERH has some characteristics related to RNA metabolism, the molecular functions of the ERH protein family still need to be further explored (Graille and Rougemaille, 2020). Malignant T cell-amplified sequence 1 (MCTS1) is an that was oncogene originally found in human T-cell lymphoma. MCTS1 can affect the cell cycle by shortening the G1/S conversion time, which is achieved by an imbalance between G1 cyclin activators and inhibitors. It has been confirmed that the expression of MCTS1 increases after DNA damage (Huang et al., 2021). In addition, MCTS1 also acts as a translation enhancer. Translation control has been proven to be an important step in regulating gene expression and subsequently cell growth, differentiation and necrosis (Bohlen et al., 2020). These functions enable MCTS1 to play multiple roles in the occurrence and development of malignant tumors, but its mechanisms in the process of inflammation and thrombosis have not been reported and are worth further exploration. Transportin 1 (TNPO1) is a protein involved in protein transduction into the nucleus, and its main target is RNA binding proteins. Studies have shown that TNPO1 is related to the influenza A virus core shell (Larson et al., 2019; Miyake et al., 2019). Moreover, its expression level can significantly predict ARDS mortality (Liao et al., 2021). This finding indicates that TNPO1 plays a key role in the inflammatory response and the occurrence of viral diseases, which show similar pathway activation to COVID-19 and thrombosis. During the COVID-19 pandemic, the most worrisome complications were ARDS and cardiovascular events, such as venous thromboembolism, myocardial infarction and stroke (Bangalore et al., 2020; Zhang Y. et al., 2020; Poissy et al., 2020; Stefanini et al., 2020). This theoretical evidence provides the basis for studying the mechanism of TNPO1 in COVID-19. In addition, two co-downregulated genes, FLNA and PXN, were obtained through bioinformatics analysis. Filamin A (FLNA) is a large actin-binding cytoskeletal protein. It is important for cell movement because it stabilizes the actin network and integrates it with the cell membrane. There is increasing evidence that FLNA is involved in the pathogenesis of cardiovascular and respiratory diseases. The interaction of FLNA with transcription factors and cell signaling molecules determines the function of vascular cells (Bandaru et al., 2021). Paxillin (PXN) is a focal adhesion protein consisting of an LD motif and a conserved cysteine-rich domain. PXN is thought to play a role in active muscle protein uptake and cell adhesion sites. Although PXN itself lacks enzymatic activity, it contains a variety of domains that can bind signals and structural proteins (Tsai et al., 2017). Therefore, PXN is considered to be a cytoskeletal protein that can effectively transmit signals (Stiegler et al., 2012). Inducers of these two co-downregulated genes have theoretical therapeutic value for COVID-19 patients with thrombosis. Based on the correlation between thrombosis and the severity of COVID-19 (Nicolai et al., 2020), further research may even identify a specific mechanism to reduce the rate of severe COVID-19, thereby reducing the threat of COVID-19 to the elderly and people with underlying diseases.
A limitation of our study is that these identified biomarkers were only verified at a theoretical level. Further experimental studies and clinical trials should be performed for accurate validation.
Based on bioinformatics analysis and previous studies, this study identified hub genes (RPS7, IGF1R, DICER1, ERH, MCTS1, TNPO1, FLNA, and PXN) that may contribute to the evaluation and treatment of COVID-19 and thrombosis. These findings provide new ideas to treat COVID-19 thrombosis and reduce the rate of severe COVID-19, and further clinical studies are needed to explore these possibilities.
Publicly available datasets were analyzed in this study. This data can be found here: https://www.ncbi.nlm.nih.gov/geo/query/acc.cgi?acc=GSE19151, https://www.ncbi.nlm.nih.gov/geo/query/acc.cgi?acc=GSE164805.
PQ and MH performed bioinformatics and were major contributors in writing the manuscript. TL guided the writing of the manuscript, and critically reviewed the manuscript. All the authors read and approved the final manuscript.
This research was supported by the Major Project of Military Logistics Scientific Research (AWS17J004), National Key Research and Development Plan for Science and Technology for the Winter Olympics of the Ministry of Science and Technology of China (2019YFF0302300), National Natural Science Foundation of China (82000631), Beijing Natural Science Foundation (7222169), Young Elite Scientist Sponsorship Program by CAST 2020QNRC001 (to MJ.H.) and Military Medical Youth Special Project of PLA General Hospital (QNF19035).
The authors declare that the research was conducted in the absence of any commercial or financial relationships that could be construed as a potential conflict of interest.
All claims expressed in this article are solely those of the authors and do not necessarily represent those of their affiliated organizations, or those of the publisher, the editors and the reviewers. Any product that may be evaluated in this article, or claim that may be made by its manufacturer, is not guaranteed or endorsed by the publisher.
The authors thank all staff in the Department of Emergency and Nephrology for their support during the study.
The Supplementary Material for this article can be found online at: https://www.frontiersin.org/articles/10.3389/fgene.2022.889348/full#supplementary-material
Supplementary Figure S1 | Box plots of GSE19151. The dataset included 70 adults who had used warfarin one or more times for venous thromboembolism and 63 healthy controls.
Supplementary Figure S2 | Box plots of GSE164805. The dataset included 10 patients diagnosed with COVID-19 and 5 healthy controls.
Achim, A., Kákonyi, K., Jambrik, Z., and Ruzsa, Z. (2022). A Case Report of COVID-19-Associated Acute Hand Ischaemia in a Young Professional Volleyball Player. Eur. Heart J. Case Rep. 6, ytac099. doi:10.1093/ehjcr/ytac099
Ackermann, M., Verleden, S. E., Kuehnel, M., Haverich, A., Welte, T., Laenger, F., et al. (2020). Pulmonary Vascular Endothelialitis, Thrombosis, and Angiogenesis in Covid-19. N. Engl. J. Med. 383, 120–128. doi:10.1056/nejmoa2015432
Altermann, E., and Klaenhammer, T. R. (2005). PathwayVoyager: Pathway Mapping Using the Kyoto Encyclopedia of Genes and Genomes (KEGG) Database. BMC Genomics 6, 60. doi:10.1186/1471-2164-6-60
Ashburner, M., Ball, C. A., Blake, J. A., Botstein, D., Butler, H., Cherry, J. M., et al. (2000). Gene Ontology: Tool for the Unification of Biology. The Gene Ontology Consortium. Nat. Genet. 25, 25–29. doi:10.1038/75556
Bandaru, S., Ala, C., Zhou, A.-X., and Akyürek, L. M. (2021). Filamin A Regulates Cardiovascular Remodeling. Ijms 22 (12), 6555. doi:10.3390/ijms22126555
Bangalore, S., Sharma, A., Slotwiner, A., Yatskar, L., Harari, R., Shah, B., et al. (2020). ST-Segment Elevation in Patients with Covid-19 - A Case Series. N. Engl. J. Med. 382, 2478–2480. doi:10.1056/nejmc2009020
Bleiziffer, S., Zabel, R., Gummert, J. F., and Deutsch, M. A. (2022). Large Thrombus in Transit in a COVID-19 Patient. Interact. Cardiovasc Thorac. Surg. ivac077. [Epub ahead of print]. doi:10.1093/icvts/ivac077
Bohlen, J., Harbrecht, L., Blanco, S., Clemm Von Hohenberg, K., Fenzl, K., Kramer, G., et al. (2020). DENR Promotes Translation Reinitiation via Ribosome Recycling to Drive Expression of Oncogenes Including ATF4. Nat. Commun. 11, 4676. doi:10.1038/s41467-020-18452-2
Boilard, E., Paré, G., Rousseau, M., Cloutier, N., Dubuc, I., Lévesque, T., et al. (2014). Influenza Virus H1N1 Activates Platelets through FcγRIIA Signaling and Thrombin Generation. Blood 123, 2854–2863. doi:10.1182/blood-2013-07-515536
Bonaventura, A., Vecchié, A., Dagna, L., Martinod, K., Dixon, D. L., Van Tassell, B. W., et al. (2021). Endothelial Dysfunction and Immunothrombosis as Key Pathogenic Mechanisms in COVID-19. Nat. Rev. Immunol. 21, 319–329. doi:10.1038/s41577-021-00536-9
Bulte, J. P., Postma, N., Beukema, M., Inberg, B., Stegeman, A. G., and Van Der Hoeven, H. (2022). COVID 19 and the Risk of Gastro-Intestinal Perforation: A Case Series and Literature Review. J. Crit. Care 67, 100–103. doi:10.1016/j.jcrc.2021.10.020
Chin, C. H., Chen, S. H., Wu, H. H., Ho, C. W., Ko, M. T., and Lin, C. Y. (2014). cytoHubba: Identifying Hub Objects and Sub-networks from Complex Interactome. BMC Syst. Biol. 8 (Suppl. 4), S11. doi:10.1186/1752-0509-8-S4-S11
Chung, M. K., Zidar, D. A., Bristow, M. R., Cameron, S. J., Chan, T., Harding, C. V., et al. (2021). COVID-19 and Cardiovascular Disease: From Bench to Bedside. Circ. Res. 128, 1214–1236. doi:10.1161/circresaha.121.317997
Cui, S., Chen, S., Li, X., Liu, S., and Wang, F. (2020). Prevalence of Venous Thromboembolism in Patients with Severe Novel Coronavirus Pneumonia. J. Thromb. Haemost. 18, 1421–1424. doi:10.1111/jth.14830
De michele, M., Kahan, J., Berto, I., Schiavo, O. G., Iacobucci, M., Toni, D., et al. (2022). Cerebrovascular Complications of COVID-19 and COVID-19 Vaccination. Circ. Res. 130, 1187–1203. doi:10.1161/circresaha.122.319954
Devaux, C. A., Rolain, J.-M., and Raoult, D. (2020). ACE2 Receptor Polymorphism: Susceptibility to SARS-CoV-2, Hypertension, Multi-Organ Failure, and COVID-19 Disease Outcome. J. Microbiol. Immunol. Infect. 53, 425–435. doi:10.1016/j.jmii.2020.04.015
Drake, T. M., Riad, A. M., Fairfield, C. J., Egan, C., Knight, S. R., Pius, R., et al. (2021). Characterisation of In-Hospital Complications Associated with COVID-19 Using the ISARIC WHO Clinical Characterisation Protocol UK: a Prospective, Multicentre Cohort Study. Lancet 398, 223–237. doi:10.1016/S0140-6736(21)00799-6
Engelmann, B., and Massberg, S. (2013). Thrombosis as an Intravascular Effector of Innate Immunity. Nat. Rev. Immunol. 13, 34–45. doi:10.1038/nri3345
Farkouh, M. E., Stone, G. W., Lala, A., Bagiella, E., Moreno, P. R., Nadkarni, G. N., et al. (2022). Anticoagulation in Patients with COVID-19: JACC Review Topic of the Week. J. Am. Coll. Cardiol. 79, 917–928. doi:10.1016/j.jacc.2021.12.023
Fatica, A., and Tollervey, D. (2002). Making Ribosomes. Curr. Opin. Cell Biol. 14, 313–318. doi:10.1016/s0955-0674(02)00336-8
Fraser, D. D., Cepinskas, G., Patterson, E. K., Slessarev, M., Martin, C., Daley, M., et al. (2020). Novel Outcome Biomarkers Identified with Targeted Proteomic Analyses of Plasma from Critically Ill Coronavirus Disease 2019 Patients. Crit. Care Explor 2, e0189. doi:10.1097/cce.0000000000000189
Fröhlich, G. M., Jeschke, E., Eichler, U., Thiele, H., Alhariri, L., Reinthaler, M., et al. (2021). Impact of Oral Anticoagulation on Clinical Outcomes of COVID-19: a Nationwide Cohort Study of Hospitalized Patients in Germany. Clin. Res. Cardiol. 110, 1041–1050. doi:10.1007/s00392-020-01783-x
Gautier, L., Cope, L., Bolstad, B. M., and Irizarry, R. A. (2004). affy--analysis of Affymetrix GeneChip Data at the Probe Level. Bioinformatics 20, 307–315. doi:10.1093/bioinformatics/btg405
Global Burden of Disease 2020 Health Financing Collaborator Network (2021). Tracking Development Assistance for Health and for COVID-19: a Review of Development Assistance, Government, Out-Of-Pocket, and Other Private Spending on Health for 204 Countries and Territories, 1990-2050. Lancet 398, 1317–1343. doi:10.1016/S0140-6736(21)01258-7
Ghasemiyeh, P., Mohammadi-Samani, S., Firouzabadi, N., Dehshahri, A., and Vazin, A. (2021). A Focused Review on Technologies, Mechanisms, Safety, and Efficacy of Available COVID-19 Vaccines. Int. Immunopharmacol. 100, 108162. doi:10.1016/j.intimp.2021.108162
Graille, M., and Rougemaille, M. (2020). ERH Proteins: Connecting RNA Processing to Tumorigenesis? Curr. Genet. 66, 689–692. doi:10.1007/s00294-020-01065-z
Gross, O., Moerer, O., Weber, M., Huber, T. B., and Scheithauer, S. (2020). COVID-19-associated Nephritis: Early Warning for Disease Severity and Complications? Lancet 395, e87–e88. doi:10.1016/s0140-6736(20)31041-2
Halushka, M. K., and Vander heide, R. S. (2021). Myocarditis Is Rare in COVID-19 Autopsies: Cardiovascular Findings across 277 Postmortem Examinations. Cardiovasc. Pathol. 50, 107300. doi:10.1016/j.carpath.2020.107300
Han, E., Tan, M. M. J., Turk, E., Sridhar, D., Leung, G. M., Shibuya, K., et al. (2020). Lessons Learnt from Easing COVID-19 Restrictions: an Analysis of Countries and Regions in Asia Pacific and Europe. Lancet 396, 1525–1534. doi:10.1016/s0140-6736(20)32007-9
Huang, Z., Su, Q., Li, W., Ren, H., Huang, H., and Wang, A. (2021). MCTS1 Promotes Invasion and Metastasis of Oral Cancer by Modifying the EMT Process. Ann. Transl. Med. 9, 997. doi:10.21037/atm-21-2361
Jeckelmann, C., Djokic, B., Duchatelle, V., and Girod, G. (2022). Case Report: Mechanical Mitral Prosthetic Valve Thrombosis in the Context of COVID-19 Despite Effective Anticoagulation. Eur. Heart J. Case Rep. 6, ytac006. doi:10.1093/ehjcr/ytac006
Jenne, C. N., Wong, C. H. Y., Zemp, F. J., Mcdonald, B., Rahman, M. M., Forsyth, P. A., et al. (2013). Neutrophils Recruited to Sites of Infection Protect from Virus Challenge by Releasing Neutrophil Extracellular Traps. Cell Host Microbe 13, 169–180. doi:10.1016/j.chom.2013.01.005
Jiang, C., Xu, J., Zhu, W., Cai, Y., Wang, S., Guo, Y., et al. (2019). Abnormal Expression of DICER1 Leads to Dysregulation of Inflammatory Effectors in Human Synoviocytes. Mediat. Inflamm. 2019, 6768504. doi:10.1155/2019/6768504
Klok, F. A., Kruip, M. J. H. A., Van Der Meer, N. J. M., Arbous, M. S., Gommers, D. A. M. P. J., Kant, K. M., et al. (2020). Incidence of Thrombotic Complications in Critically Ill ICU Patients with COVID-19. Thrombosis Res. 191, 145–147. doi:10.1016/j.thromres.2020.04.013
Koupenova, M., Corkrey, H. A., Vitseva, O., Manni, G., Pang, C. J., Clancy, L., et al. (2019). The Role of Platelets in Mediating a Response to Human Influenza Infection. Nat. Commun. 10, 1780. doi:10.1038/s41467-019-09607-x
Lal, A., Erondu, N. A., Heymann, D. L., Gitahi, G., and Yates, R. (2021). Fragmented Health Systems in COVID-19: Rectifying the Misalignment between Global Health Security and Universal Health Coverage. Lancet 397, 61–67. doi:10.1016/s0140-6736(20)32228-5
Lala, A., Johnson, K. W., Januzzi, J. L., Russak, A. J., Paranjpe, I., Richter, F., et al. (2020). Prevalence and Impact of Myocardial Injury in Patients Hospitalized with COVID-19 Infection. J. Am. Coll. Cardiol. 76, 533–546. doi:10.1016/j.jacc.2020.06.007
Larson, G. P., Tran, V., Yú, S., Caì, Y., Higgins, C. A., Smith, D. M., et al. (2019). EPS8 Facilitates Uncoating of Influenza A Virus. Cell Rep. 29, 2175–2183. doi:10.1016/j.celrep.2019.10.064
Lawler, P. R., Goligher, E. C., Berger, J. S., Lawler, P. R., Goligher, E. C., Berger, J. S., et al. (2021). Therapeutic Anticoagulation with Heparin in Noncritically Ill Patients with Covid-19. N. Engl. J. Med. 385, 790–802. doi:10.1056/NEJMoa2105911
Levi, M., Thachil, J., Iba, T., and Levy, J. H. (2020). Coagulation Abnormalities and Thrombosis in Patients with COVID-19. Lancet Haematol. 7, e438–e440. doi:10.1016/s2352-3026(20)30145-9
Li, G., Zhou, L., Zhang, C., Shi, Y., Dong, D., Bai, M., et al. (2019). Insulin-Like Growth Factor 1 Regulates Acute Inflammatory Lung Injury Mediated by Influenza Virus Infection. Front. Microbiol. 10, 2541. doi:10.3389/fmicb.2019.02541
Li, M., Zhang, X., Ang, K. S., Ling, J., Sethi, R., Lee, N. Y. S., et al. (2022). DISCO: a Database of Deeply Integrated Human Single-Cell Omics Data. Nucleic Acids Res. 50, D596–d602. doi:10.1093/nar/gkab1020
Liao, S. Y., Casanova, N. G., Bime, C., Camp, S. M., Lynn, H., and Garcia, J. G. N. (2021). Identification of Early and Intermediate Biomarkers for ARDS Mortality by Multi-Omic Approaches. Sci. Rep. 11, 18874. doi:10.1038/s41598-021-98053-1
Lodigiani, C., Iapichino, G., Carenzo, L., Cecconi, M., Ferrazzi, P., Sebastian, T., et al. (2020). Venous and Arterial Thromboembolic Complications in COVID-19 Patients Admitted to an Academic Hospital in Milan, Italy. Thrombosis Res. 191, 9–14. doi:10.1016/j.thromres.2020.04.024
Lowenstein, C. J., and Solomon, S. D. (2020). Severe COVID-19 Is a Microvascular Disease. Circulation 142, 1609–1611. doi:10.1161/circulationaha.120.050354
Maier, C. L., Truong, A. D., Auld, S. C., Polly, D. M., Tanksley, C.-L., and Duncan, A. (2020). COVID-19-associated Hyperviscosity: a Link between Inflammation and Thrombophilia? Lancet 395, 1758–1759. doi:10.1016/s0140-6736(20)31209-5
Mcintyre, P. B., Aggarwal, R., Jani, I., Jawad, J., Kochhar, S., Macdonald, N., et al. (2022). COVID-19 Vaccine Strategies Must Focus on Severe Disease and Global Equity. Lancet 399, 406–410. doi:10.1016/s0140-6736(21)02835-x
Mi, H., Muruganujan, A., Huang, X., Ebert, D., Mills, C., Guo, X., et al. (2019). Protocol Update for Large-Scale Genome and Gene Function Analysis with the PANTHER Classification System (v.14.0). Nat. Protoc. 14, 703–721. doi:10.1038/s41596-019-0128-8
Miyake, Y., Keusch, J. J., Decamps, L., Ho-Xuan, H., Iketani, S., Gut, H., et al. (2019). Influenza Virus Uses Transportin 1 for vRNP Debundling during Cell Entry. Nat. Microbiol. 4, 578–586. doi:10.1038/s41564-018-0332-2
Nadkarni, G. N., Lala, A., Bagiella, E., Chang, H. L., Moreno, P. R., Pujadas, E., et al. (2020). Anticoagulation, Bleeding, Mortality, and Pathology in Hospitalized Patients with COVID-19. J. Am. Coll. Cardiol. 76, 1815–1826. doi:10.1016/j.jacc.2020.08.041
Nicolai, L., Leunig, A., Brambs, S., Kaiser, R., Joppich, M., Hoffknecht, M. L., et al. (2021). Vascular Neutrophilic Inflammation and Immunothrombosis Distinguish Severe COVID‐19 from Influenza Pneumonia. J. Thromb. Haemost. 19, 574–581. doi:10.1111/jth.15179
Nicolai, L., Leunig, A., Brambs, S., Kaiser, R., Weinberger, T., Weigand, M., et al. (2020). Immunothrombotic Dysregulation in COVID-19 Pneumonia Is Associated with Respiratory Failure and Coagulopathy. Circulation 142, 1176–1189. doi:10.1161/circulationaha.120.048488
Pellegrini, D., Kawakami, R., Guagliumi, G., Sakamoto, A., Kawai, K., Gianatti, A., et al. (2021). Microthrombi as a Major Cause of Cardiac Injury in COVID-19: A Pathologic Study. Circulation 143, 1031–1042. doi:10.1161/circulationaha.120.051828
Poissy, J., Goutay, J., Caplan, M., Parmentier, E., Duburcq, T., Lassalle, F., et al. (2020). Pulmonary Embolism in Patients with COVID-19: Awareness of an Increased Prevalence. Circulation 142, 184–186. doi:10.1161/circulationaha.120.047430
Ritchie, M. E., Phipson, B., Wu, D., Hu, Y., Law, C. W., Shi, W., et al. (2015). Limma Powers Differential Expression Analyses for RNA-Sequencing and Microarray Studies. Nucleic acids Res. 43, e47. doi:10.1093/nar/gkv007
Ronco, C., Reis, T., and Husain-syed, F. (2020). Management of Acute Kidney Injury in Patients with COVID-19. Lancet Respir. Med. 8, 738–742. doi:10.1016/s2213-2600(20)30229-0
Rosenthal, R., Cadieux, E. L., Cadieux, E. L., Salgado, R., Bakir, M. A., Moore, D. A., et al. (2019). Neoantigen-directed Immune Escape in Lung Cancer Evolution. Nature 567, 479–485. doi:10.1038/s41586-019-1032-7
Rosovsky, R. P., Sanfilippo, K. M., Wang, T. F., Rajan, S. K., Shah, S., Martin, K. A., et al. (2020). Anticoagulation Practice Patterns in COVID‐19: A Global Survey. Res. Pract. Thromb. Haemost. 4, 969–983. doi:10.1002/rth2.12414
Russell, J., Wagoner, M., Dupont, J., Myers, D., Muthu, K., and Thotakura, S. (2022). Left Ventricular Thrombus of Unknown Etiology in a Patient with COVID-19 Disease with No Significant Medical History. Cardiovasc. Revasc. Med. S1553–8389(22)00121-X. doi:10.1016/j.carrev.2022.03.010
Sastry, S., Cuomo, F., and Muthusamy, J. (2022). COVID-19 and Thrombosis: The Role of Hemodynamics. Thrombosis Res. 212, 51–57. doi:10.1016/j.thromres.2022.02.016
Serban, D., Tribus, L. C., Vancea, G., Stoian, A. P., Dascalu, A. M., Suceveanu, A. I., et al. (2021). Acute Mesenteric Ischemia in COVID-19 Patients. Jcm 11 (1), 200. doi:10.3390/jcm11010200
Shankar, R., Leimanis, M. L., Newbury, P. A., Liu, K., Xing, J., Nedveck, D., et al. (2020). Gene Expression Signatures Identify Paediatric Patients with Multiple Organ Dysfunction Who Require Advanced Life Support in the Intensive Care Unit. EBioMedicine 62, 103122. doi:10.1016/j.ebiom.2020.103122
Shannon, P., Markiel, A., Ozier, O., Baliga, N. S., Wang, J. T., Ramage, D., et al. (2003). Cytoscape: a Software Environment for Integrated Models of Biomolecular Interaction Networks. Genome Res. 13, 2498–2504. doi:10.1101/gr.1239303
Shao, F., Xu, S., Ma, X., Xu, Z., Lyu, J., Ng, M., et al. (2020). In-hospital Cardiac Arrest Outcomes Among Patients with COVID-19 Pneumonia in Wuhan, China. Resuscitation 151, 18–23. doi:10.1016/j.resuscitation.2020.04.005
Sriwastava, S., Tandon, M., Podury, S., Prasad, A., Wen, S., Guthrie, G., et al. (2021). COVID-19 and Neuroinflammation: a Literature Review of Relevant Neuroimaging and CSF Markers in Central Nervous System Inflammatory Disorders from SARS-COV2. J. Neurol. 268, 4448–4478. doi:10.1007/s00415-021-10611-9
Stark, K., and Massberg, S. (2021). Interplay between Inflammation and Thrombosis in Cardiovascular Pathology. Nat. Rev. Cardiol. 18, 666–682. doi:10.1038/s41569-021-00552-1
Stefanini, G. G., Montorfano, M., Trabattoni, D., Andreini, D., Ferrante, G., Ancona, M., et al. (2020). ST-elevation Myocardial Infarction in Patients with COVID-19: Clinical and Angiographic Outcomes. Circulation 141, 2113–2116. doi:10.1161/circulationaha.120.047525
Stiegler, A. L., Draheim, K. M., Li, X., Chayen, N. E., Calderwood, D. A., and Boggon, T. J. (2012). Structural Basis for Paxillin Binding and Focal Adhesion Targeting of β-Parvin. J. Biol. Chem. 287, 32566–32577. doi:10.1074/jbc.m112.367342
Suh, Y. J., Hong, H., Ohana, M., Bompard, F., Revel, M.-P., Valle, C., et al. (2021). Pulmonary Embolism and Deep Vein Thrombosis in COVID-19: A Systematic Review and Meta-Analysis. Radiology 298, E70–e80. doi:10.1148/radiol.2020203557
Takasu, S., Ariizumi, M., Matsumoto, S., Nakagawa, H., and Iwadate, K. (2022). Cerebral Venous Sinus Thrombosis Associated with COVID-19: an Autopsy Case Report. Forensic Sci. Med. Pathol. 18, 80–85. doi:10.1007/s12024-022-00458-5
Thomas, M., and Scully, M. (2022). Clinical Features of Thrombosis and Bleeding in COVID-19. Blood. doi:10.1182/blood.2021012247
Tripathi, S., Verma, A., Kim, E.-J., White, M. R., and Hartshorn, K. L. (2014). LL-37 Modulates Human Neutrophil Responses to Influenza A Virus. J. Leukoc. Biol. 96, 931–938. doi:10.1189/jlb.4a1113-604rr
Tsai, W.-C., Yu, T.-Y., Lin, L.-P., Lin, M.-S., Tsai, T.-T., and Pang, J.-H. S. (2017). Platelet Rich Plasma Promotes Skeletal Muscle Cell Migration in Association with Up-Regulation of FAK, Paxillin, and F-Actin Formation. J. Orthop. Res. 35, 2506–2512. doi:10.1002/jor.23547
Uhlen, M., Karlsson, M. J., Zhong, W., Tebani, A., Pou, C., Mikes, J., et al. (2019). A Genome-wide Transcriptomic Analysis of Protein-Coding Genes in Human Blood Cells. Science 366 (6472), eaax9198. doi:10.1126/science.aax9198
Vincent, J.-L., Levi, M., and Hunt, B. J. (2022). Prevention and Management of Thrombosis in Hospitalised Patients with COVID-19 Pneumonia. Lancet Respir. Med. 10, 214–220. doi:10.1016/s2213-2600(21)00455-0
Walter, W., Sánchez-Cabo, F., and Ricote, M. (2015). GOplot: an R Package for Visually Combining Expression Data with Functional Analysis: Fig. 1. Bioinformatics 31, 2912–2914. doi:10.1093/bioinformatics/btv300
Wang, J., Zhu, K., Xue, Y., Wen, G., and Tao, L. (2021). Research Progress in the Treatment of Complications and Sequelae of COVID-19. Front. Med. 8, 757605. doi:10.3389/fmed.2021.757605
Wang, Z., Hou, J., Lu, L., Qi, Z., Sun, J., Gao, W., et al. (2013). Small Ribosomal Protein Subunit S7 Suppresses Ovarian Tumorigenesis through Regulation of the PI3K/AKT and MAPK Pathways. PLoS One 8, e79117. doi:10.1371/journal.pone.0079117
Wei, H., Liang, G., and Vera, R. M. (2021). Dantrolene Repurposed to Treat Sepsis or Septic Shock and COVID-19 Patients. Eur. Rev. Med. Pharmacol. Sci. 25, 3136–3144. doi:10.26355/eurrev_202104_25569
Yongzhi, X. (2021). COVID-19-associated Cytokine Storm Syndrome and Diagnostic Principles: an Old and New Issue. Emerg. Microbes Infect. 10, 266–276. doi:10.1080/22221751.2021.1884503
Yu, G., Wang, L.-G., Han, Y., and He, Q.-Y. (2012). clusterProfiler: an R Package for Comparing Biological Themes Among Gene Clusters. OMICS A J. Integr. Biol. 16, 284–287. doi:10.1089/omi.2011.0118
Zangrillo, A., Belletti, A., Palumbo, D., Calvi, M. R., Guzzo, F., Fominskiy, E. V., et al. (2022). One-Year Multidisciplinary Follow-Up of Patients with COVID-19 Requiring Invasive Mechanical Ventilation. J. Cardiothorac. Vasc. Anesth. 36, 1354–1363. doi:10.1053/j.jvca.2021.11.032
Zhang, L., Yan, X., Fan, Q., Liu, H., Liu, X., Liu, Z., et al. (2020). D‐dimer Levels on Admission to Predict In‐hospital Mortality in Patients with Covid‐19. J. Thromb. Haemost. 18, 1324–1329. doi:10.1111/jth.14859
Zhang, Y., Xiao, M., Zhang, S., Xia, P., Cao, W., Jiang, W., et al. (2020). Coagulopathy and Antiphospholipid Antibodies in Patients with Covid-19. N. Engl. J. Med. 382, e38. doi:10.1056/nejmc2007575
Zhou, F., Yu, T., Du, R., Fan, G., Liu, Y., Liu, Z., et al. (2020). Clinical Course and Risk Factors for Mortality of Adult Inpatients with COVID-19 in Wuhan, China: a Retrospective Cohort Study. Lancet 395, 1054–1062. doi:10.1016/s0140-6736(20)30566-3
Zuo, Y., Estes, S. K., Ali, R. A., Gandhi, A. A., Yalavarthi, S., Shi, H., et al. (2020). Prothrombotic Autoantibodies in Serum from Patients Hospitalized with COVID-19. Sci. Transl. Med. 12 (570), eabd3876. doi:10.1126/scitranslmed.abd3876
Keywords: COVID-19, thrombosis, therapy, bioinformatic analysis, differentially expressed genes
Citation: Qi P, Huang M and Li T (2022) Screening the Potential Biomarkers of COVID-19-Related Thrombosis Through Bioinformatics Analysis. Front. Genet. 13:889348. doi: 10.3389/fgene.2022.889348
Received: 07 March 2022; Accepted: 09 May 2022;
Published: 25 May 2022.
Edited by:
Manal S. Fawzy, Faculty of Medicine, Suez Canal University, EgyptReviewed by:
Aaron N. Johnson, Washington University in St. Louis, United StatesCopyright © 2022 Qi, Huang and Li. This is an open-access article distributed under the terms of the Creative Commons Attribution License (CC BY). The use, distribution or reproduction in other forums is permitted, provided the original author(s) and the copyright owner(s) are credited and that the original publication in this journal is cited, in accordance with accepted academic practice. No use, distribution or reproduction is permitted which does not comply with these terms.
*Correspondence: Tanshi Li, bGl0czMwMUBzaW5hLmNvbQ==
†These authors have contributed equally to this work and share first authorship
Disclaimer: All claims expressed in this article are solely those of the authors and do not necessarily represent those of their affiliated organizations, or those of the publisher, the editors and the reviewers. Any product that may be evaluated in this article or claim that may be made by its manufacturer is not guaranteed or endorsed by the publisher.
Research integrity at Frontiers
Learn more about the work of our research integrity team to safeguard the quality of each article we publish.