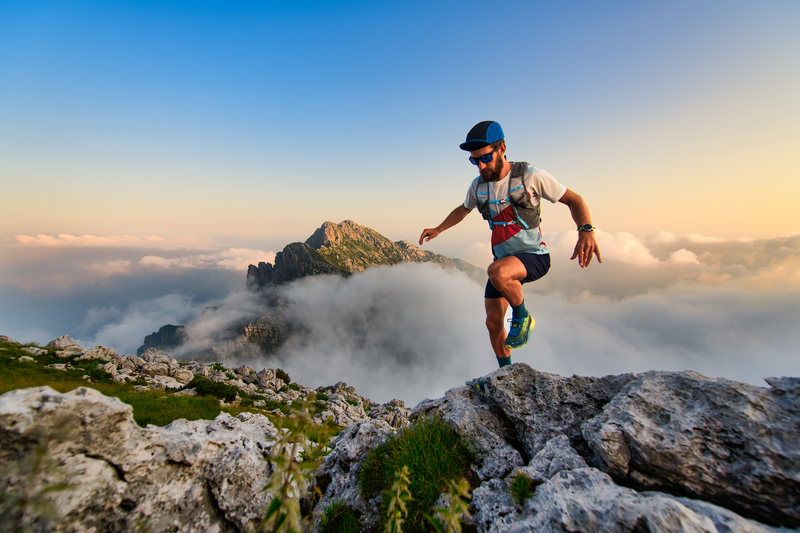
95% of researchers rate our articles as excellent or good
Learn more about the work of our research integrity team to safeguard the quality of each article we publish.
Find out more
ORIGINAL RESEARCH article
Front. Genet. , 22 July 2022
Sec. Cancer Genetics and Oncogenomics
Volume 13 - 2022 | https://doi.org/10.3389/fgene.2022.881948
This article is part of the Research Topic Genetic and proteomic biomarkers in solid tumor detection and treatment View all 64 articles
Background: Owing to complex molecular mechanisms in gastric cancer (GC) oncogenesis and progression, existing biomarkers and therapeutic targets could not significantly improve diagnosis and prognosis. This study aims to identify the key genes and signaling pathways related to GC oncogenesis and progression using bioinformatics and meta-analysis methods.
Methods: Eligible microarray datasets were downloaded and integrated using the meta-analysis method. According to the tumor stage, GC gene chips were classified into three groups. Thereafter, the three groups’ differentially expressed genes (DEGs) were identified by comparing the gene data of the tumor groups with those of matched normal specimens. Enrichment analyses were conducted based on common DEGs among the three groups. Then protein–protein interaction (PPI) networks were constructed to identify relevant hub genes and subnetworks. The effects of significant DEGs and hub genes were verified and explored in other datasets. In addition, the analysis of mutated genes was also conducted using gene data from The Cancer Genome Atlas database.
Results: After integration of six microarray datasets, 1,229 common DEGs consisting of 1,065 upregulated and 164 downregulated genes were identified. Alpha-2 collagen type I (COL1A2), tissue inhibitor matrix metalloproteinase 1 (TIMP1), thymus cell antigen 1 (THY1), and biglycan (BGN) were selected as significant DEGs throughout GC development. The low expression of ghrelin (GHRL) is associated with a high lymph node ratio (LNR) and poor survival outcomes. Thereafter, we constructed a PPI network of all identified DEGs and gained 39 subnetworks and the top 20 hub genes. Enrichment analyses were performed for common DEGs, the most related subnetwork, and the top 20 hub genes. We also selected 61 metabolic DEGs to construct PPI networks and acquired the relevant hub genes. Centrosomal protein 55 (CEP55) and POLR1A were identified as hub genes associated with survival outcomes.
Conclusion: The DEGs, hub genes, and enrichment analysis for GC with different stages were comprehensively investigated, which contribute to exploring the new biomarkers and therapeutic targets.
Gastric cancer (GC) is the third leading cause of cancer deaths and the fifth most frequently diagnosed cancer worldwide, with over 1 million new cases and 700,000 deaths each year (Bray et al., 2018). Most GC patients are already in the advanced stage at the initial visit, and these patients have a poor prognosis and even have to undergo radical surgery (Irino et al., 2021; Manzanedo et al., 2021; Stocker et al., 2021). In the past decades, gene sequencing and molecular targeted therapy have been increasingly widely used in clinical practice. Therefore, it is of great clinical value to explore the core genes and molecular mechanisms in pathogenesis for the diagnosis and treatment of GC.
In an analysis of key circulating exosomal miRNAs, four key miRNAs (hsa-miR-130b-3p, hsa-miR-151a-3p, hsa-miR-15b-3p, and hsa-miR-1246) and the interaction network or enrichments based on their targets (TAOK1, CMTM6, SCN3A, WASF3, IGF1, CNOT7, GABRG1, and PRKD1) may help understand the molecular mechanisms in the GC development (Qian et al., 2021). The study by Mou et al. (2015)demonstrated that four SNP loci (rs2279115, rs804270, rs909253, and rs3765524) showed a potential association with GC risk. In addition, many targets and markers have been applied to the diagnosis and treatment of GC in basic experiments and achieved preliminary results. HER2 monoclonal antibody conjugated RNase-A-associated CdTe quantum dots (Ruan et al., 2012) and BRCAA1 monoclonal antibody conjugated fluorescent magnetic nanoparticles (Wang et al., 2011) both exhibited great potential in applications such as in situ GC targeted imaging and selective therapy of GC. The study by Zhang et al. (2015)showed that miR-19b-3p and miR-16-5p were biomarkers that own great potential in applications such as early screening and progression evaluation of GC. Furthermore, the microarray-based prewarning system, which could be applied in the early detection of GC, was developed by Cui et al. (2005).
At present, many bioinformatic studies about GC have been published, but the differentially expressed genes (DEGs) and signaling pathways revealed by different studies were not consistent. The study by Yang and Gong (2021) showed that OLFM4, IGF2BP3, CLDN1, and MMP1 were the most significantly upregulated DEGs, which significantly enriched in negative regulation of growth, fatty acid binding, and cellular response to zinc ions. In a bioinformatics analysis conducted by Xu et al. (2021), the expressions of ITGB1 and alpha-2 collagen type I (COL1A2) were significantly increased in GC tissues, and 63 characteristic DEGs were mainly involved in regulating extracellular matrix (ECM)–receptor interactions and the PI3K-Akt signaling pathway. Xu et al. (2020) also found that SLC1A3 promotes GC progression via the PI3K/AKT signaling pathway.
Most published experimental and bioinformatic studies included GC specimens with unclear tumor stages, making it impossible to accurately analyze the DEGs and signaling pathways throughout GC development (Yang et al., 2021; Xu et al., 2021). In this study, therefore, we retrieved three microarray datasets containing gene data with definite GC stages and then divided them into the early stage (ES) group and the late stage (LS) group. The two groups’ DEGs were obtained by comparing GC tissues of the ES and LS groups with adjacent noncancerous gastric tissues. Another three microarray datasets containing gene data with indefinite GC stages were also collected, and DEGs in GC were identified relative to normal tissues. Gene Ontology (GO) and Kyoto Encyclopedia of Genes and Genomes (KEGG) pathway enrichment analyses were conducted on DAVID (https://david.ncifcrf.gov/) and Sangerbox 3.0 (http://vip.sangerbox.com/home.html) using common DEGs from the three groups. Thereafter, protein–protein interaction (PPI) network was constructed using the STRING online tool (https://cn.string-db.org/) and Cytoscape software (Shannon et al., 2003). The effects of significant DEGs and hub genes were verified and explored in other datasets. The related gene expression data and clinical information were also obtained from The Cancer Genome Atlas (TCGA) database (https://portal.gdc.cancer.gov/), which were used to carry out overall survival analysis and somatic mutation analysis. Besides this, hub genes associated with metabolic KEGG pathways were also identified. Through this analysis, we identified the key genes and signaling pathways related to GC, aiming to provide the experimental basis and important insight of new biomarkers and prognostic markers.
Eligible microarray datasets were downloaded from the Gene Expression Omnibus (GEO) database (http://www.ncbi.nlm.nih.gov/geo/). Datasets in accordance with the following criteria were included and considered for subsequent analysis: upload data were between 2010 to 2021; contained the gene data on GC tissues and adjacent normal tissues and at least three samples per group; tissue samples used were from humans; and detail information on technology and platform were obtainable.
When the staging of GC specimens was definite, gene chips were divided into the ES group and LS group based on tumor stages. The ES group and LS group respectively incorporated stage I–II and stage III–IV GC’s microarray data, which were staged according to standards recommended by the American Joint Committee on Cancer. GC gene chips with indefinite tumor stages were classified as the mixed stage (MS) group, incorporating stage I–IV GC’s microarray data. DEGs were obtained by comparing the microarray data of GC with those of adjacent noncancerous gastric tissues. Thereafter, meta-analysis was performed to integrate microarray data for the above each group, and the three groups’ DEGs would be obtained. DEGs from the three groups were intersected to identify common DEGs. In addition, DEGs were ranked according to adjusted p-value (adj.P.Val) from small to large, and the most significant DEGs were also gained by intersecting the top 100 significant DEGs from the three groups. The effects of above significant DEGs were verified and explored in other GEO datasets (GSE103236, GSE51725, and GSE17187) and TCGA database.
Common DEGs were divided into the upregulated group and the downregulated group. Next, GO terms and KEGG pathways enrichment analyses of upregulated DEGs and downregulated DEGs were respectively performed using the DAVID online tool. GO terms consisted of the following items: biological process (BP), molecular function (MF), and cellular component (CC). KEGG pathway analysis was designed to identify significantly enriched pathways of molecular interactions and reactions. The flow diagram of this bioinformatics and meta-analysis is shown in Figure 1.
FIGURE 1. Flow diagram of this bioinformatics and meta-analysis. DEGs, differentially expressed genes; KEGG, Kyoto Encyclopedia of Genes; GO, gene ontology; LNR, lymph node ratio; TCGA, The Cancer Genome Atlas.
The PPI network of DEGs was constructed based on the STRING database, with a confidence score set as 0.9 (highest confidence). Then, PPI network files were imported to Cytoscape v3.8 software, in which we constructed the subnetwork using the MCODE plug-in and calculated the top 20 genes based on the Multiscale Curvature Classification (MCC) algorithm. The DAVID, Metascape (https://metascape.org/), and Sangerbox 3.0 online tools were used for enrichment analysis and visualization of hub genes and the first subnetwork. The effects of hub genes were explored and proved in another three GEO microarray datasets (GSE103236, GSE51725, and GSE17187) and TCGA database. The DEGs converged on KEGG pathways associated with metabolism and were also inputted into the STRING database and Cytoscape software to obtain relevant PPI networks and hub genes.
Integrative meta-analysis of GEO data (ImaGEO) (https://imageo.genyo.es/), an online tool, was adopted to integrate microarray data (Toro-Domínguez et al., 2019). What is more, the fixed effect model of the effect size method and Fisher’s p-value method were both applied to perform a meta-analysis for each group. The adj.P.Val < 0.05 and |Log2 fold change (logFC)| > 1 were set as the parameters to identify DEGs. The Wilcoxon test was employed to compare the statistical differences between the two groups, while the Kruskal–Wallis (KS) test was adopted as a statistical method to compare multiple groups. And beyond that, all cut-off p-values were set as p < 0.05 in this analysis.
First, the microarray datasets containing GSE19826, GSE26899, GSE33335, GSE63089, GSE79973, and GSE118916 were acquired for further analyses. Inadaptable gene chips were removed from selected microarray datasets. After selection, the expression data of 42 GC tissues from GSE19826, GSE26899, and GSE33335 were classified as the ES group, while the expression data of another 53 GC tissues from the above same microarray datasets were classified as the LS group. In addition, GSE63089, GSE79973, and GSE118916 respectively included the gene data of 45, 10, and 15 GC tissues and were classified as the MS group. The characteristics of selected GEO microarray datasets are shown in Table 1.
After intersecting the DEGs obtained using two different meta-analysis methods, 4,125, 3,699, and 3,531 DEGs were gained from the ES, LS, and MS groups, respectively. Then we intersected the above DEGs and identified 1,229 common DEGs, which consisted of 1,065 upregulated genes and 164 downregulated genes. In addition, ATP4A, ATP4B, CPA2, ghrelin (GHRL), KCNE2, GIF, ESRRG, COL1A2, tissue inhibitor matrix metalloproteinase 1 (TIMP1), ADH7, AQP4, thymus cell antigen 1 (THY1), and biglycan (BGN) were identified by intersecting the top 100 significant DEGs from the three groups. GSE103236, GSE51725, and GSE17187 were used to verify the effects of the above 12 DEGs (except AQP4) in tumorigenesis and development. By analyzing the gene data of GSE103236, we found that the expression levels of ESRRG, COL1A2, TIMP1, THY1, and BGN in GC tissues with ES and GC tissues with advanced stage were both significantly different than those in paracancer tissues (Figure 2A). GSE51725 and GSE17187 based on the same GEO platform (GPL570) were normalized, and the batch effect was removed. Compared with the expression data before normalizing (Figure 2B), those after removing the batch effect were found to be more consistent (Figure 2C). In the analysis of integrated gene data, a noticeable correlation was found between the expression level of GHRL and the LNR of the tumor (Figure 2D). Moreover, analysis based on TCGA database showed that the expression levels of 12 significant DEGs (except GIF) were significantly different between GC tissues and adjacent normal gastric tissues (Figure 3A), and statistical correlation could also be found between the expression levels of COL1A2, TIMP1, THY1, and BGN and tumor stage (Figure 3B).
FIGURE 2. The roles that significant DEGs play in the oncogenesis, progression, and lymph node metastasis. (A) comparison of the expression levels of ATP4A, ATP4B, CPA2, GHRL, KCNE2, GIF, ESRRG, COL1A2, TIMP1, ADH7, THY1, and BGN between paracancer tissues, GC tissues with early stage and GC tissues with late stage. (B) gene data before normalization. (C) gene data after normalization. (D) comparison of the expression levels of ATP4A, ATP4B, GHRL, KCNE2, GIF, ESRRG, COL1A2, ADH7, THY1, BGN, AQP4, and CPA2 between GC tissues with high LNR and GC tissues with low LNR. The statistical methods adopted for A and D are the Kruskal–Wallis test and Wilcoxon test, respectively. -, p > 0.05; *, p < 0.05; **, p < 0.01. DEGs, differentially expressed genes; GC, gastric cancer; LNR, lymph node ratio.
FIGURE 3. The verification and exploration of significant DEGs in TCGA database. (A) comparison of the expression levels of ATP4A, ATP4B, CPA2, GHRL, KCNE2, ESRRG, COL1A2, TIMP1, ADH7, AQP4, THY1, and BGN between GC and paracancer tissues from TCGA database. (B) the association between the expression levels of COL1A2, TIMP1, THY1, and BGN with tumor stage. DEGs, differentially expressed genes; TCGA, The Cancer Genome Atlas; GC, gastric cancer.
Enrichment analyses of KEGG pathways and GO terms were both performed for upregulated and downregulated DEGs using the DAVID online tool. Regarding upregulated DEGs, KEGG pathway analysis showed that DEGs were mainly enriched in DNA replication, ECM–receptor interaction, pyrimidine metabolism, cell cycle, and purine metabolism (Figure 4A), while DEGs were mainly enriched in cell division, DNA replication, mitotic nuclear division, sister chromatid cohesion, and G1/S transition of the mitotic cell cycle in GO terms analysis (Figure 4B). As for downregulated DEGs, gastric acid secretion, chemical carcinogenesis, retinol metabolism, drug metabolism–cytochrome P450 and metabolism of xenobiotics by cytochrome P450 were KEGG pathways that play important roles (Figure 4C), while GO terms analysis showed that DEGs were mainly enriched in digestion, the xenobiotic metabolic process, potassium ion import, and gastric acid secretion (Figure 4D). It was also found that most KEGG pathways of downregulated DEGs were related to metabolism, including retinol metabolism, drug metabolism–cytochrome P450, metabolism of xenobiotics by cytochrome P450, metabolic pathways, glycolysis/gluconeogenesis, histidine, alanine, aspartate, glutamate, and tyrosine metabolism. In the analysis of upregulated DEGs, pyrimidine metabolism and purine metabolism were KEGG pathways associated with metabolism. In the end, 31 upregulated DEGs and 30 downregulated DEGs pooled in metabolism-related KEGG pathways were selected for further analysis.
FIGURE 4. Visualization of the enrichment results. Distribution of the upregulated DEGs in GC for different (A) KEGG pathways and (B) GO-enriched functions, and distribution of the down -regulated DEGs in GC for different (C) KEGG pathways and (D) GO-enriched functions. DEGs, differentially expressed genes; GC, gastric cancer; KEGG, Kyoto Encyclopedia of Genes and Genomes; GO, Gene ontology; BP, biological process; CC, cellular component; MF, molecular function.
The PPI network of 1,229 common DEGs, which involved 656 nodes (DEGs) and 2,701 edges, was visualized using Cytoscape v3.8 software. As analysis results of the MCODE plug-in showed, 39 subnetworks were identified, and the first cluster (Figure 5A) was related to mitotic cell cycle, PID Aurora B pathway, regulation of the cell cycle process and chromosome segregation, PID FOXM1 pathway, and PID PLK1 pathway (Figure 5B). Hub genes such as topoisomerase II alpha (TOP2A), cyclin-B2 (CCNB2), KIF11, cyclin-A2 (CCNA2), cell division cycle 20 (CDC20), cell division cycle–associated protein 8 (CDCA8), KIF20A, benzimidazoles 1 homolog beta (BUB1B), targeting protein for Xenopus kinesin-like protein 2 (TPX2), and KIF2C were identified using an MCC algorithm (Figure 5C). Enrichment analysis indicated that the hub genes were significantly enriched in cell division, nuclear division, mitotic cell cycle, microtubule cytoskeleton, PID Aurora B pathway, PID PLK1 pathway, and PID FOXM1 pathway (Figure 5D).
FIGURE 5. PPI subnetwork construction and hub genes identification. (A)the first subnetwork of PPI using MCODE plug-in of Cytoscape. (B) enrichment analysis and visualization of first cluster using Metascape online tool. (C) top 20 hub genes of all common DEGs identified by an MCC algorithm. (D) enrichment analysis and visualization of top 20 hub genes using the Metascape online tool. PPI networks and relevant hub genes of (E–F) 31 upregulated metabolic DEGs and (G–H) 30 downregulated metabolic DEGs. (I) survival curves of GHRL, CEP55, and POLR1A expression. PPI, protein–protein interaction; DEGs, differentially expressed genes; MCC, Multiscale Curvature Classification.
In addition, PPI networks and relevant hub genes of 31 upregulated metabolic DEGs (Figures 5E,F) and 30 downregulated metabolic DEGs (Figures 5G,H) were both constructed and identified. Further, the KM plotters of significant DEGs and hub genes indicated that the expression levels of GHRL, centrosomal protein 55 (CEP55), and POLR1A were associated with survival outcomes in patients with GC (Figure 5I).
A summary of gene mutation information of GC patients from TCGA database is shown in Figure 6A. Somatic mutation profiles for 437 GC patients were retrieved. And we used the waterfall plot to present the mutation data for every gene in every sample (Figure 6B). The significant signatures of mutated genes were also explored (Figure 6C). Further, we assessed the effects of the top 10 mutated genes (TTN, TP53, MUC16, LRP1B, SYNE1, ARID1A, CSMD3, FAT4, FLG, and PCLO) in tumor progression. As the statistical analysis showed, TP53 and SYNE1 were associated with tumor stage (Figure 6D), while TTN, LRP1B, and FAT4 were associated with survival events (Figure 6E).
FIGURE 6. Gene mutation information of GC samples from TCGA database. (A) mutation profile landscape in GC samples. (B) waterfall plot showing the mutation details of every gene in every sample. (C) heatmap showing the significant signatures of mutated genes. (D) correlation analysis between tumor stage with mutated genes (TP53 and SYNE1). (E) correlation analysis between survival event (0, alive; 1, death) with mutated genes (TTN, LRP1B, and FAT4). GC, gastric cancer; TCGA, The Cancer Genome Atlas.
As one of the most common malignant tumors with high mortality, GC has always been a serious disease threatening human health. Risk prediction, early diagnosis, and precise therapies are considered the essential measures to improve the prognosis of GC patients. Owing to complex BP in GC occurrence and development, however, existing markers and therapeutic targets could not significantly improve diagnosis or 5-year survival rate (Ajani et al., 2016; Wang et al., 2019). Therefore, a study on the pivotal mechanism of GC occurrence and development is urgently needed, which helps select sensitive and specific biomarkers or therapeutic targets.
In this study, the gene expression data of GSE19826, GSE26899, GSE33335, GSE63089, GSE79973, and GSE118916 were integrated through a meta-analysis method. Then enrichment analysis, PPI network construction, and hub gene identification were performed based on integrated data. In total, 1,065 upregulated and 164 downregulated DEGs throughout GC development were screened out. Enrichment analyses of GO terms and KEGG pathways indicated that DEGs could lead to the incidence and exacerbation of GC by affecting DNA replication, cell and chromosome division, and related metabolic pathways. Furthermore, ECM–receptor interaction and PI3K-Akt were also KEGG pathways that DEGs significantly enriched. The tumor microenvironment (TME), a complex ecosystem composed of ECM, peripheral blood vessels, other non-malignant cells, and signaling molecules, has been proved to be important for tumor invasion, progression, and chemoresistance (Ran et al., 2021). The biological function of PI3K-Akt signaling in GC progression has been well established, which could regulate tumor cell growth, proliferation, apoptosis, and energy metabolism. An activated PI3K-Akt signaling pathway could promote GC progression by enhancing glycolysis, stabilizing mitochondrial membrane potentials, and inhibiting tumor cell apoptosis (Xu et al., 2020). In addition, abundant metabolic pathways were found to be associated with GC, revealing the close connection between the metabolic system and the identified DEGs (Tian et al., 2020). To date, accumulated evidence has suggested that cancer is a metabolic disease, in which cells have lost their normal checks on cell proliferation, resulting in excessive bioenergetic and biosynthetic needs. Therefore, cancer cells must alter their metabolism to sustain such a high demand (Kroemer and Pouyssegur, 2008; Li et al., 2013; Duda et al., 2020). Large amounts of purines and pyrimidines are required for cell proliferation, DNA replication, and energy supply of GC tissues; thus, pyrimidine metabolism and purine metabolism are metabolic pathways upregulated DEGs significantly enriched. Moreover, the majority of downregulated DEGs converged on metabolic pathways such as metabolism of retinol, drug–cytochrome P450, glycolysis/gluconeogenesis, histidine, alanine, aspartate, glutamate, and tyrosine. It has long been recognized that tumor metabolism preferentially relies on glycolysis instead of oxidative phosphorylation of glucose despite the status of oxygen supply, and this type of metabolism is known as the Warburg effect (Kroemer and Pouyssegur, 2008). As a result, key enzymes in the oxidative phosphorylation of glucose are downregulated for cancer cell proliferation and tumorigenicity. Fructose-1,6-bisphosphatase-2 (FBP2), one kind of enzyme participating in glycogen synthesis from carbohydrate precursors, can catalyze the hydrolysis of fructose-1,6-bisphosphate to fructose-6-phosphate and inorganic phosphate in glucose metabolism. FBP2 underexpression may contribute to GC tumor development by stimulating glucose metabolism and inhibiting cell proliferation (Li et al., 2013; Duda et al., 2020). In recent years, glucolipid metabolism therapy of tumors has become a research hotspot, which aims to inhibit the proliferation and metastasis of tumor cells by controlling glycolysis and increasing fat for energy (Hur et al., 2013; Sun et al., 2018). Gastric acid secretion, another important pathway that downregulated DEGs enriched, has been reported by few studies. GC was considered to be associated with gastritis, and intestinal metaplasia developing in atrophic gastritis was believed to be a step in the gastric carcinogenic process. Therefore, patients with GC had reduced gastric acid secretion because of oxyntic atrophic gastritis (Waldum and Rehfeld, 2019; Liu et al., 2020a). Furthermore, decreased GHRL in blood also contributed to this phenomenon.
Up to now, tumor markers such as CEA, CA125, CA199, and CA72-4 have been used as indicators for GC diagnosis. Her2, epidermal growth factor receptor (EGFR), TP53, and PI3K are key oncogenes reported to work (Li et al., 2022; Zhao et al., 2022). Besides these, Xie et al. (2015) established a multi-index prediction model based on the six kinds of biomarkers (CEA, CA199, H.P., P53, PG Ⅰ, and PG Ⅱ), which was designed to achieve early screening and therapeutic evaluation of GC patients. However, the above biomarkers and therapeutic targets had insufficient specificity in the early diagnosis of GC and could not accurately evaluate tumor progression and survival time (Gao and Yang, 2022). In this study, COL1A2, TIMP1, THY1, and BGN were identified as significant DEGs for both the GC with ES and the GC with LS. Further analysis showed that the expression levels of the above four DEGs were associated with tumor stage, and the expression level of GHRL was associated with LNR and survival outcome. Recurrence and metastasis, the primary factors affecting the survival outcome of GC patients, are also the hotspots of current research. The above DEGs may influence tumor stage and LNR by affecting GC recurrence and metastasis. COL1A2 gene can affect cell proliferation, differentiation, adhesion, and metastasis by encoding type I collagen—the most widely expressed collagen among the fibrous collagen family (Pan et al., 2021; Xu et al., 2021). TIMP1 is a natural collagenase inhibitor that can inhibit apoptosis, induce angiogenesis, and stimulate cell proliferation, which may be directly involved in the progression and metastasis of cancers such as GC, breast cancer, and colon cancer (Zhang et al., 2020; Liu et al., 2021). THY1, also known as the CD90 gene, plays an important regulatory role in the cellular interactions between a cell and its matrix. Moreover, previous studies indicated that the THY1 gene can inhibit GC cells’ apoptosis by regulating secreted protein acidic and rich in cysteine protein’s expression levels (Wu et al., 2019; Wang et al., 2021). As an important component of ECM proteins, BGN seems to play an important role in the oncogenesis and progression of various cancers. According to the research by Hu et al. (2016), BGN may promote cancer progression through the chronic activation of tumor angiogenesis, so high expression of BGN was observed in advanced GC. In addition, tight connections between the above four hub genes could be found; thus, definitive linkages and interaction mechanisms needed to be further explored. Therefore, COL1A2, TIMP1, THY1, BGN, and GHRL have great potential in applications such as early screening of GC patients, prediction of therapeutic outcomes, and real-time dynamic monitoring the progress of GC in near future.
TOP2A, CCNB2, KIF11, CCNA2, CDC20, CDCA8, KIF20A, BUB1B, TPX2, and KIF2C were identified as the top 10 hub genes among all DEGs. TOP2A is one kind of type II DNA topoisomerases that can relax negative and positive supercoiling during replication and transcription. The expression level of the TOP2A gene can reflect tumor proliferation and was reported to be associated with peritoneal and hematogenous recurrence. Thus, TOP2A may be utilized as a specific drug target for malignant tumors such as GC (Hou et al., 2020). As previous studies found, CCNB2 was a cell cycle–related gene that can promote the proliferation and tumor growth of GC cells (Liu et al., 2020b). Similar to CCNB2, CCNA2 is a highly conserved cyclin that plays a critical role in the control of the cell cycle at G1/S and in the G2/M transition (Lee et al., 2020). Kinesin superfamily (KIF), a group of proteins that possess ATPase activity and motion characteristics, participate in numerous cellular biological activities such as mitosis and meiosis. Kruppel (KIF) 11, KIF20A, and KIF2C genes have been illustrated as genes that might function as oncogenes in GC (Imai et al., 2017; Sheng et al., 2018). Several lines of evidence have shown that CDC20 plays a vital role in the correct functioning of the spindle assembly checkpoint (SAC), and overexpression of the CDC20 gene is related to intestinal histology and favorable clinicopathologic parameters in GC (Kim et al., 2019). In addition, multidomain protein kinase budding uninhibited by BUB1B may contribute to the process of SAC, which can delay the separation of sister chromatids to prevent defects in segregation (Hudler et al., 2016). CDCA8, also called Borealin/Dasra B, is a crucial cell cycle–regulated chromosomal passenger protein, and its nuclear accumulation is correlated with a poor prognosis for GC (Chang et al., 2006). TPX2 is a microtubule-associated protein that relates to chromosomal instability and helps format normal bipolar spindles and chromosome segregation (Tomii et al., 2017).
Further, the expression levels of GHRL, CEP55, and POLR1A were proved to be associated with survival outcomes in patients with GC. GHRL, a small peptide characterized as the ligand of the growth hormone secretagogue receptor (GHSR), plays role in stimulating pituitary growth hormone release, the regulation of energy balance, gastric acid release, appetite, insulin secretion, gastric motility, and the turnover of the gastric and intestinal mucosa. Previous studies have shown that GHRL works in several key processes of cancer progression, such as cell proliferation, migration, and invasion. The action mechanism of GHRL in promoting or inhibiting cancer progression, however, is still unclear. The regulation of the GHRL–GHSR axis may play a potential critical role (Lin and Hsiao, 2017). As a member of the centrosomal relative protein family, CEP55 has been reported to participate in cell cycle regulation. Tao’s study showed that ectopic overexpression of CEP55 could enhance the cell proliferation, colony formation, and tumorigenicity of GC cells, and CEP55 knockdown induces cell cycle arrest at the G2/M phase in GC cells. Besides, the expression of CEP55 can affect the PI3K/AKT/p21 signaling pathway and cyclin pathway-related proteins (Tao et al., 2014). Folate receptor gene family has a high affinity for folic acid and several reduced folic acid derivatives, and it mediates delivery of 5-methyltetrahydrofolate to the interior of cells. Moreover, mature FOLR1 is an N-glycosylated protein that is predominantly expressed on epithelial cells and is dramatically upregulated on many carcinomas (Kim et al., 2018).
In this study, six eligible microarray datasets were integrated to present the gene expression signatures of GC relative to normal gastric tissues using ImaGEO meta-analysis. COL1A2, TIMP1, THY1, and BGN were identified and verified as significant DEGs throughout GC progression, and the above DEGs are expected to be used as the target molecules in GC diagnosis and therapy. In the future, basal experimentation and clinical tests are needed to verify their roles in early screening and tumor preventing and controlling of GC patients. As enrichment analysis showed, the upregulated DEGs mainly enriched in DNA replication, cell cycle, and ECM–receptor interaction, while most of the downregulated DEGs were related to metabolism. TOP2A, CCNB2, KIF11, CCNA2, CDC20, CDCA8, KIF20A, BUB1B, TPX2, and KIF2C were identified as the top 10 hub genes among all DEGs. Further, the expression levels of GHRL, CEP55, and POLR1A were proved to be associated with survival outcomes in patients with GC. The interaction between hub genes, the intervention mechanism of hub genes on tumors, and the association between hub genes with survival outcomes are the main direction for the next research step.
The identified DEGs, hub genes, and signaling pathways may help us understand the molecular mechanisms of gastric tumor and discover new biomarkers and therapeutic targets for gastric tumor.
The original contributions presented in the study are included in the article/Supplementary Material, and further inquiries can be directed to the corresponding author.
The data used in this study are from publicly available datasets. Ethical approval and informed consent for participation were not required for this study in accordance with the national legislation and the institutional requirements.
YY, LC, and LY designed the study and wrote and amended the original draft. TY and QZ participated in searching microarray datasets and analyzing the gene data. DY, ZH, and MC produced the tables and figures. All authors read and approved the final manuscript.
The current study was supported by a research grant from Capital’s funds for health improvement and research (2020–1–1021).
The authors declare that the research was conducted in the absence of any commercial or financial relationships that could be construed as a potential conflict of interest.
All claims expressed in this article are solely those of the authors and do not necessarily represent those of their affiliated organizations, or those of the publisher, the editors, and the reviewers. Any product that may be evaluated in this article, or claim that may be made by its manufacturer, is not guaranteed or endorsed by the publisher.
We thank Gene Expression Omnibus and The Cancer Genome Atlas for providing detailed gene data and clinical information.
Ajani, J. A., D'Amico, T. A., Almhanna, K., Bentrem, D. J., Chao, J., Das, P., Denlinger, C. S., Fanta, P., Farjah, F., Fuchs, C. S., Gerdes, H., Gibson, M., Glasgow, R. E., Hayman, J. A., Hochwald, S., Hofstetter, W. L., Ilson, D. H., Jaroszewski, D., Johung, K. L., Keswani, R. N., Kleinberg, L. R., Korn, W. M., Leong, S., Linn, C., Lockhart, A. C., Ly, Q. P., Mulcahy, M. F., Orringer, M. B., Perry, K. A., Poultsides, G. A., Scott, W. J., Strong, V. E., Washington, M. K., Weksler, B., Willett, C. G., Wright, C. D., Zelman, D., McMillian, N., and Sundar, H. (2016). Gastric Cancer, Version 3.2016, NCCN Clinical Practice Guidelines in Oncology. J. Natl. Compr. Canc Netw. 14 (10), 1286–1312. doi:10.6004/jnccn.2016.0137
Bray, F., Ferlay, J., Soerjomataram, I., Siegel, R. L., Torre, L. A., and Jemal, A. (2018). Global Cancer Statistics 2018: GLOBOCAN Estimates of Incidence and Mortality Worldwide for 36 Cancers in 185 Countries. CA a cancer J. Clin. 68 (6), 394–424. doi:10.3322/caac.21492
Chang, J.-L., Chen, T.-H., Wang, C.-F., Chiang, Y.-H., Huang, Y.-L., Wong, F.-H., Chou, C.-K., and Chen, C.-M. (2006). Borealin/Dasra B Is a Cell Cycle-Regulated Chromosomal Passenger Protein and its Nuclear Accumulation Is Linked to Poor Prognosis for Human Gastric Cancer. Exp. Cell. Res. 312 (7), 962–973. doi:10.1016/j.yexcr.2005.12.015
Cui, D.-X., Zhang, L., Yan, X. J., Zhang, L. X., Xu, J. R., Guo, Y. H., et al. (2005). A Microarray-Based Gastric Carcinoma Prewarning System. Wjg 11 (9), 1273–1282. doi:10.3748/wjg.v11.i9.1273
Duda, P., Janczara, J., McCubrey, J. A., Gizak, A., and Rakus, D. (2020). The Reverse Warburg Effect Is Associated with Fbp2-dependent Hif1α Regulation in Cancer Cells Stimulated by Fibroblasts. Cells 9 (1), 205. doi:10.3390/cells9010205
Gao, W., and Yang, M. (2022). Identification by Bioinformatics Analysis of Potential Key Genes Related to the Progression and Prognosis of Gastric Cancer. Front. Oncol. 12, 881015. doi:10.3389/fonc.2022.881015
Hou, G., Deng, J., You, X., Chen, J., Jiang, Y., Qian, T., Bi, Y., Song, B., Xu, Y., and Yang, X. (2020). Mining Topoisomerase Isoforms in Gastric Cancer. Gene 754, 144859. doi:10.1016/j.gene.2020.144859
Hu, L., Zang, M.-D., Wang, H.-X., Li, J.-F., Su, L.-P., Yan, M., Li, C., Yang, Q.-M., Liu, B.-Y., and Zhu, Z.-G. (2016). Biglycan Stimulates VEGF Expression in Endothelial Cells by Activating the TLR Signaling Pathway. Mol. Oncol. 10 (9), 1473–1484. doi:10.1016/j.molonc.2016.08.002
Hudler, P., Britovsek, N. K., Grazio, S. F., and Komel, R. (2016). Association between Polymorphisms in Segregation Genes BUB1B and TTK and Gastric Cancer Risk. Radiology Oncol. 50 (3), 297–307. doi:10.1515/raon-2015-0047
Hur, H., Xuan, Y., Kim, Y. B., Lee, G., Shim, W., Yun, J., Ham, I.-H., and Han, S.-U. (2013). Expression of Pyruvate Dehydrogenase Kinase-1 in Gastric Cancer as a Potential Therapeutic Target. Int. J. Oncol. 42 (1), 44–54. doi:10.3892/ijo.2012.1687
Imai, T., Oue, N., Nishioka, M., Mukai, S., Oshima, T., Sakamoto, N., Sentani, K., Matsusaki, K., Yoshida, K., and Yasui, W. (2017). Overexpression of KIF11 in Gastric Cancer with Intestinal Mucin Phenotype. Pathobiology 84 (1), 16–24. doi:10.1159/000447303
Irino, T., Matsuda, S., Wada, N., Kawakubo, H., and Kitagawa, Y. (2021). Essential Updates 2019/2020: Perioperative and Surgical Management of Gastric Cancer. Ann. Gastroenterol. Surg. 5 (2), 162–172. doi:10.1002/ags3.12438
Kim, M., Pyo, S., Kang, C. H., Lee, C. O., Lee, H. K., Choi, S. U., and Park, C. H. (2018). Folate Receptor 1 (FOLR1) Targeted Chimeric Antigen Receptor (CAR) T Cells for the Treatment of Gastric Cancer. PloS one 13 (6), e0198347. doi:10.1371/journal.pone.0198347
Kim, Y., Choi, J.-W., Lee, J.-H., and Kim, Y.-S. (2019). Spindle Assembly Checkpoint MAD2 and CDC20 Overexpressions and Cell-In-Cell Formation in Gastric Cancer and its Precursor Lesions. Hum. Pathol. 85, 174–183. doi:10.1016/j.humpath.2018.10.029
Kroemer, G., and Pouyssegur, J. (2008). Tumor Cell Metabolism: Cancer's Achilles' Heel. Cancer Cell. 13 (6), 472–482. doi:10.1016/j.ccr.2008.05.005
Lee, Y., Lee, C. E., Oh, S., Kim, H., Lee, J., Kim, S. B., and Kim, H. S. (2020). Pharmacogenomic Analysis Reveals CCNA2 as a Predictive Biomarker of Sensitivity to Polo-Like Kinase I Inhibitor in Gastric Cancer. Cancers 12 (6), 1418. doi:10.3390/cancers12061418
Li, H., Wang, J., Xu, H., Xing, R., Pan, Y., Li, W., Cui, J., Zhang, H., and Lu, Y. (2013). Decreased Fructose-1,6-Bisphosphatase-2 Expression Promotes Glycolysis and Growth in Gastric Cancer Cells. Mol. Cancer 12 (1), 110. doi:10.1186/1476-4598-12-110
Li, X., Li, S., Zhang, Z., and Huang, D. (2022). Association of Multiple Tumor Markers with Newly Diagnosed Gastric Cancer Patients: a Retrospective Study. PeerJ 10, e13488. doi:10.7717/peerj.13488
Lin, T.-C., and Hsiao, M. (2017). Ghrelin and Cancer Progression. Biochimica Biophysica Acta (BBA) - Rev. Cancer 1868 (1), 51–57. doi:10.1016/j.bbcan.2017.02.002
Liu, A. G., Zhong, J. C., Chen, G., He, R. Q., He, Y. Q., Ma, J., Yang, L. H., Wu, X. J., Huang, J. T., Li, J. J., Mo, W. J., and Qin, X. G. (2020). Upregulated Expression of SAC3D1 Is Associated with Progression in Gastric Cancer. Int. J. Oncol. 57 (1), 122–138. doi:10.3892/ijo.2020.5048
Liu, H., Xiang, Y., Zong, Q.-B., Zhang, X.-Y., Wang, Z.-W., Fang, S.-Q., Zhang, T.-C., and Liao, X.-H. (2021). miR-6745-TIMP1 axis Inhibits Cell Growth and Metastasis in Gastric Cancer. Aging 13 (21), 24402–24416. doi:10.18632/aging.203688
Liu, M., Li, S., Du, M., Chu, H., Wang, M., and Zhang, Z. (2020). Novel CpG-SNPs in the Gastric Acid Secretion Pathway GNAI3 and Susceptibility to Gastric Cancer. Gene 736, 144447. doi:10.1016/j.gene.2020.144447
Manzanedo, I., Pereira, F., Serrano, Á., and Pérez-Viejo, E. (2021). Review of Management and Treatment of Peritoneal Metastases from Gastric Cancer Origin. J. Gastrointest. Oncol. 12 (Suppl. 1), S20–S29. doi:10.21037/jgo-20-232
Mou, X., Li, T., Wang, J., Ali, Z., Zhang, Y., Chen, Z., Deng, Y., Li, S., Su, E., Jia, Q., He, N., Ni, J., and Cui, D. (2015). Genetic Variation of BCL2 (Rs2279115), NEIL2 (Rs804270), LTA (Rs909253), PSCA (Rs2294008) and PLCE1 (Rs3765524, Rs10509670) Genes and Their Correlation to Gastric Cancer Risk Based on Universal Tagged Arrays and Fe3O4 Magnetic Nanoparticles. J. Biomed. Nanotechnol. 11 (11), 2057–2066. doi:10.1166/jbn.2015.2113
Pan, H., Ding, Y., Jiang, Y., Wang, X., Rao, J., Zhang, X., Yu, H., Hou, Q., and Li, T. (2021). LncRNA LIFR-AS1 Promotes Proliferation and Invasion of Gastric Cancer Cell via miR-29a-3p/COL1A2 axis. Cancer Cell. Int. 21 (1), 7. doi:10.1186/s12935-020-01644-7
Qian, X., Xie, F., Wei, H., and Cui, D. (2021). Identification of Key Circulating Exosomal microRNAs in Gastric Cancer. Front. Oncol. 11, 693360. doi:10.3389/fonc.2021.693360
Ran, T., Chen, Z., Zhao, L., Ran, W., Fan, J., Hong, S., and Yang, Z. (2021). LAMB1 Is Related to the T Stage and Indicates Poor Prognosis in Gastric Cancer. Technol. Cancer Res. Treat. 20, 153303382110049. doi:10.1177/15330338211004944
Ruan, J., Song, H., Qian, Q., Li, C., Wang, K., Bao, C., and Cui, D. (2012). HER2 Monoclonal Antibody Conjugated RNase-A-Associated CdTe Quantum Dots for Targeted Imaging and Therapy of Gastric Cancer. Biomaterials 33 (29), 7093–7102. doi:10.1016/j.biomaterials.2012.06.053
Shannon, P., Markiel, A., Ozier, O., Baliga, N. S., Wang, J. T., Ramage, D., Amin, N., Schwikowski, B., and Ideker, T. (2003). Cytoscape: a Software Environment for Integrated Models of Biomolecular Interaction Networks. Genome Res. 13 (11), 2498–2504. doi:10.1101/gr.1239303
Sheng, Y., Wang, W., Hong, B., Jiang, X., Sun, R., Yan, Q., Zhang, S., Lu, M., Wang, S., Zhang, Z., Lin, W., and Li, Y. (2018). Upregulation of KIF20A Correlates with Poor Prognosis in Gastric Cancer. Cmar 10, 6205–6216. doi:10.2147/CMAR.S176147
Stocker, G., Thieme, R., and Lordick, F. (2021). Neoadjuvante und perioperative Therapie des Magenkarzinoms, aktuelle Studien und neue Biomarker. Chirurg 92 (6), 499–505. doi:10.1007/s00104-021-01355-3
Sun, K., Hu, P., and Xu, F. (2018). LINC00152/miR-139-5p Regulates Gastric Cancer Cell Aerobic Glycolysis by Targeting PRKAA1. Biomed. Pharmacother. 97, 1296–1302. doi:10.1016/j.biopha.2017.11.015
Tao, J., Zhi, X., Tian, Y., Li, Z., Zhu, Y., Wang, W., Xie, K., Tang, J., Zhang, X., Wang, L., and Xu, Z. (2014). CEP55 Contributes to Human Gastric Carcinoma by Regulating Cell Proliferation. Tumor Biol. 35 (5), 4389–4399. doi:10.1007/s13277-013-1578-1
Tian, J., Xue, W., Yin, H., Zhang, N., Zhou, J., Long, Z., Wu, C., Liang, Z., Xie, K., Li, S., Li, L., Wu, Z., Daria, V., Zhao, Y., Wang, F., and Wang, M. (2020). Differential Metabolic Alterations and Biomarkers between Gastric Cancer and Colorectal Cancer: A Systematic Review and Meta-Analysis. Ott 13, 6093–6108. doi:10.2147/OTT.S247393
Tomii, C., Inokuchi, M., Takagi, Y., Ishikawa, T., Otsuki, S., Uetake, H., Kojima, K., and Kawano, T. (2017). TPX2 Expression Is Associated with Poor Survival in Gastric Cancer. World J. Surg. Onc 15 (1), 14. doi:10.1186/s12957-016-1095-y
Toro-Domínguez, D., Martorell-Marugán, J., López-Domínguez, R., García-Moreno, A., González-Rumayor, V., Alarcón-Riquelme, M. E., and Carmona-Sáez, P. (2019). ImaGEO: Integrative Gene Expression Meta-Analysis from GEO Database. Bioinforma. Oxf. Engl. 35 (5), 880–882. doi:10.1093/bioinformatics/bty721
Waldum, H. L., and Rehfeld, J. F. (2019). Gastric Cancer and Gastrin: on the Interaction of Helicobacter pylori Gastritis and Acid Inhibitory Induced Hypergastrinemia. Scand. J. gastroenterology 54 (9), 1118–1123. doi:10.1080/00365521.2019.1663446
Wang, F.-H., Shen, L., Li, J., Zhou, Z.-W., Liang, H., Zhang, X.-T., Tang, L., Xin, Y., Jin, J., Zhang, Y.-J., Yuan, X.-L., Liu, T.-S., Li, G.-X., Wu, Q., Xu, H.-M., Ji, J.-F., Li, Y.-F., Wang, X., Yu, S., Liu, H., Guan, W.-L., and Xu, R.-H. (2019). The Chinese Society of Clinical Oncology (CSCO): Clinical Guidelines for the Diagnosis and Treatment of Gastric Cancer. Cancer Commun. 39 (1), 10. doi:10.1186/s40880-019-0349-9
Wang, K., Ruan, J., Qian, Q., Song, H., Bao, C., Zhang, X., Kong, Y., Zhang, C., Hu, G., Ni, J., and Cui, D. (2011). BRCAA1 Monoclonal Antibody Conjugated Fluorescent Magnetic Nanoparticles for In Vivo Targeted Magnetofluorescent Imaging of Gastric Cancer. J. Nanobiotechnol 9, 23. doi:10.1186/1477-3155-9-23
Wang, Y., Zheng, K., Chen, X., Chen, R., and Zou, Y. (2021). Bioinformatics Analysis Identifies COL1A1, THBS2 and SPP1 as Potential Predictors of Patient Prognosis and Immunotherapy Response in Gastric Cancer. Biosci. Rep. 41 (1), BSR20202564. doi:10.1042/BSR20202564
Wu, K., Zou, J., Lin, C., and Jie, Z.-G. (2019). MicroRNA-140-5p Inhibits Cell Proliferation, Migration and Promotes Cell Apoptosis in Gastric Cancer through the Negative Regulation of THY1-Mediated Notch Signaling. Biosci. Rep. 39 (7), BSR20181434. doi:10.1042/BSR20181434
Xie, Y., Zhi, X., Su, H., Wang, K., Yan, Z., He, N., Zhang, J., Chen, D., and Cui, D. (2015). A Novel Electrochemical Microfluidic Chip Combined with Multiple Biomarkers for Early Diagnosis of Gastric Cancer. Nanoscale Res. Lett. 10 (1), 477. doi:10.1186/s11671-015-1153-3
Xu, J., Wang, X., Ke, Q., Liao, K., Wan, Y., Zhang, K., Zhang, G., and Wang, X. (2021). Combined Bioinformatics Technology to Explore Pivot Genes and Related Clinical Prognosis in the Development of Gastric Cancer. Sci. Rep. 11 (1), 15412. doi:10.1038/s41598-021-94291-5
Xu, L., Chen, J., Jia, L., Chen, X., Awaleh Moumin, F., and Cai, J. (2020). SLC1A3 Promotes Gastric Cancer Progression via the PI3K/AKT Signalling Pathway. J. Cell. Mol. Med. 24 (24), 14392–14404. doi:10.1111/jcmm.16060
Yang, C., and Gong, A. (2021). Integrated Bioinformatics Analysis for Differentially Expressed Genes and Signaling Pathways Identification in Gastric Cancer. Int. J. Med. Sci. 18 (3), 792–800. doi:10.7150/ijms.47339
Zhang, J., Song, Y., Zhang, C., Zhi, X., Fu, H., Ma, Y., Chen, Y., Pan, F., Wang, K., Ni, J., Jin, W., He, X., Su, H., and Cui, D. (2015). Circulating MiR-16-5p and MiR-19b-3p as Two Novel Potential Biomarkers to Indicate Progression of Gastric Cancer. Theranostics 5 (7), 733–745. doi:10.7150/thno.10305
Zhang, Y., Qin, L., Ma, X., Wang, Y., Wu, Y., and Jiang, J. (2020). Coexpression of Matrix Metalloproteinase-7 and Tissue Inhibitor of Metalloproteinase-1 as a Prognostic Biomarker in Gastric Cancer. Dis. markers 2020 (10), 1. doi:10.1155/2020/8831466
Keywords: gastric cancer, microarray datasets, differentially expressed genes, metabolism, meta-analysis
Citation: Yu Z, Liang C, Tu H, Qiu S, Dong X, Zhang Y, Ma C and Li P (2022) Common Core Genes Play Vital Roles in Gastric Cancer With Different Stages. Front. Genet. 13:881948. doi: 10.3389/fgene.2022.881948
Received: 23 February 2022; Accepted: 31 May 2022;
Published: 22 July 2022.
Edited by:
Apeng Chen, Lanzhou Veterinary Research Institute (CAAS), ChinaReviewed by:
Ehsan Nazemalhosseini-Mojarad, Shahid Beheshti University of Medical Sciences, IranCopyright © 2022 Yu, Liang, Tu, Qiu, Dong, Zhang, Ma and Li. This is an open-access article distributed under the terms of the Creative Commons Attribution License (CC BY). The use, distribution or reproduction in other forums is permitted, provided the original author(s) and the copyright owner(s) are credited and that the original publication in this journal is cited, in accordance with accepted academic practice. No use, distribution or reproduction is permitted which does not comply with these terms.
*Correspondence: Peiyu Li, bGlwZWl5dTYzMDFAMTYzLmNvbQ==
†These authors have contributed equally to this work
Disclaimer: All claims expressed in this article are solely those of the authors and do not necessarily represent those of their affiliated organizations, or those of the publisher, the editors and the reviewers. Any product that may be evaluated in this article or claim that may be made by its manufacturer is not guaranteed or endorsed by the publisher.
Research integrity at Frontiers
Learn more about the work of our research integrity team to safeguard the quality of each article we publish.