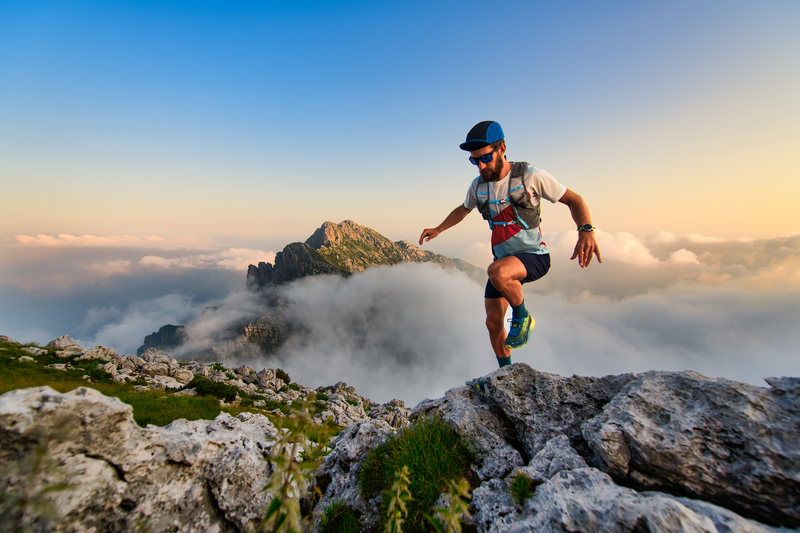
94% of researchers rate our articles as excellent or good
Learn more about the work of our research integrity team to safeguard the quality of each article we publish.
Find out more
ORIGINAL RESEARCH article
Front. Genet. , 13 May 2022
Sec. Statistical Genetics and Methodology
Volume 13 - 2022 | https://doi.org/10.3389/fgene.2022.878607
This article is part of the Research Topic Network Based Causal Inference Methods Using Multi-Omics Data View all 7 articles
Objective: We identify and explore the candidate susceptibility genes for cirrhosis and their underlying biological mechanism.
Methods: We downloaded the genome-wide association studies summary data of 901 cirrhosis cases and 451,363 controls and integrated them with reference models of five potential tissues from the Genotype-Tissue Expression (GTEx) Project, including whole blood, liver, pancreas, spleen, and thyroid, to identify genes whose expression is predicted to be associated with cirrhosis. Then, we downloaded gene expression data of individuals with hepatocellular carcinoma from TCGA database to conduct differential expression analysis to validate these identified genes and explored their possible role in driving cirrhosis via functional enrichment and gene set enrichment analysis (GSEA).
Results: We identified 10 significant genes (SKIV2L, JPH4, UQCC2, RP11-91I8.3, MAU2, ERAP1, PUS3, ZNF677, ARHGAP40, and SHANK3) associated with cirrhosis at a Bonferroni-corrected threshold of p < 0.01, among which two (SKIV2L and JPH4) were identified in the liver and five (SKIV2L, JPH4, MAU2, SHANK3, and UQCC2) were validated by differential expression analysis at an FDR-corrected threshold of p < 0.01. The enrichment analysis showed that the degradation process of RNA, which is enriched by 58 genes, is significantly under-enriched in liver cancer tissues (p = 0.0268).
Conclusion: We have identified several candidate genes for cirrhosis in multiple tissues and performed differential genetic analysis using the liver cancer database to verify the significant genes. We found that the genes SKIV2L and JPH4 identified in the liver are of particular concern. Finally, through enrichment analysis, we speculate that the process of mRNA transcription and RNA degradation may play a role in cirrhosis.
Cirrhosis is a diffuse liver disease characterized by fibrosis and structural abnormalities due to different mechanisms of liver injury (Tsochatzis et al., 2014; Fukui et al., 2016; Garcia-Tsao et al., 2017). The progression and outcome of cirrhosis are very serious. Cirrhosis will increase the risk of hepatocellular carcinoma (HCC) and will ultimately lead to death inevitably (Tsochatzis et al., 2014; Villanueva, 2019). Studies have shown that cirrhosis is the 14th most common cause of death in adults worldwide (Lozano et al., 2012). In addition, it can also cause serious complications that worsen the prognosis of cirrhosis (Fukui et al., 2016). Cirrhosis may also involve multiple organs throughout the body (Arroyo et al., 2021). Cirrhosis is known to cause changes in the blood (Bajaj et al., 2019); patients with cirrhosis have symptoms of splenomegaly in the advanced stage (Fofiu et al., 2021), and studies have shown that patients with liver dysfunction also suffer from pancreatic dysfunction (Epstein et al., 1982). Furthermore, according to our previous studies, there is a correlation between cirrhosis and thyroid dysfunction (Huang et al., 2021).
Many studies have explored the risk factors of cirrhosis, mainly including heavy alcohol intake (Roerecke et al., 2019), chronic viral hepatitis B and C infections (Mokdad et al., 2014), autoimmune hepatitis (Basyte-Bacevice et al., 2019), and a growing obesity epidemic (Mokdad et al., 2014). Research studies have also shown that the occurrence of cirrhosis is related to the genetic background of the host (Fabris et al., 2009; Kupcinskas et al., 2017; Basyte-Bacevice et al., 2019). In recent years, genome-wide association studies (GWAS) have identified a number of susceptibility loci (Innes et al., 2020; Emdin et al., 2021). However, most of the identified variants are located in non-coding regions, which make their functional characterization of disease occurrence inaccurate and complicated (Maurano et al., 2012). In addition, complicated linkage disequilibrium (LD) may obscure the causal variation leading to the association. These challenges have prompted the development of GWAS to map susceptibility genes and new analytical methods (Tam et al., 2019).
The transcriptome-wide association study (TWAS) allows us to explore the genetic basis underlying complicated diseases such as cirrhosis from the perspective of gene expression, by integrating GWAS data and external expression quantitative trait loci (eQTL) data (Bossé et al., 2020). TWAS uses one or more constructed eQTL reference panels to predict gene–trait associations (Wainberg et al., 2019). To date, many TWAS analyses have been applied to the identification of candidate genes for complicated diseases, such as mental disorders (Derks and Gamazon, 2020), pancreatic cancer (Liu et al., 2020), lung cancer (Zhu et al., 2021), and pulmonary fibrosis (Gong et al., 2020) and provided many valuable clues for a better understanding of the occurrence and progression of diseases. However, for many complicated traits, the biologically relevant tissues are unknown. Most existing studies identify gene–trait associations based on a single tissue, and the significant gene effects identified thereby are exaggerated. Also, studies have shown that eQTL with larger effect tends to regulate gene expression in multiple tissues. TWAS analysis in multiple tissues can improve the accuracy of the results.
In this study, we employed TWAS to explore the cirrhosis-related genes. Considering cirrhosis is associated with multiple tissues, we used reference panels of eQTL for five tissues, including whole blood, liver, pancreas, spleen, and thyroid, together with GWAS summary data on cirrhosis to identify tissue-specific susceptibility genes. Meanwhile, we also used differential gene expression analysis and functional enrichment analyses to validate the identified susceptibility genes. Our study might pave the way to further reveal the mechanism underlying cirrhosis.
We obtained the GWAS summary data of cirrhosis (n = 452,264, prevalence = 1.99%) from the Gene ATLAS website (http://geneatlas.roslin.ed.ac.uk/), a large database of associations between hundreds of traits and millions of variants using White British individuals for the UK Biobank (Canela-Xandri et al., 2018). The association statistics was calculated under a linear mix model, with sex, array batch, UK Biobank Assessment Center, age, age2 (Canela-Xandri et al., 2018), and the top 20 genotype PCs included as fixed effects. Following Marees et al., (2018), we filtered out variants with MAF <0.01 or HWE p-value <1e−10. Finally, we used LDSC (version 1.0.1) to transform the filtered summary data into the LD-score format as well as computed the heritability.
FUSION was applied to the formatted GWAS summary data for tissue-related TWAS analysis, including whole blood, liver, pancreas, spleen, and thyroid (Gusev et al., 2016). Briefly, FUSION uses precomputed gene expression weights, along with disease GWAS summary statistics, to calculate the association of each gene with disease. The association statistics was defined as TWAS Z-score and estimated as following:
We downloaded gene expression data (HTSeq-Counts) and corresponding clinical information of 377 individuals with hepatocellular carcinoma from TCGA database (Adrian Ally et al., 2017), retained those with paired adjacent tissue samples, and obtained data with 100 samples (50 tumor and 50 adjacent tissue samples). In quality control steps, we first mapped the ENSEMBL ID to gene symbol ID and averaged those expressions that mapped to the same symbol. Then, we filtered out genes expressed in fewer than three samples. Finally, we retained the expression matrix with 100 samples and 13,124 genes and applied TMM normalization to the filtered matrix. We used the limma package (version 3.50.0) to conduct differential expression analysis (Ritchie et al., 2015). Gene with | log2 (fold change) |≥1 and false discovery rates (FDR)-adjusted p-value<0.01 was defined as differentially expressed genes (DEGs).
We used the clusterProfiler package (version 4.0.5) to perform Gene Ontology (GO) and Kyoto Encyclopedia of Genes and Genomes (KEGG) enrichment analyses on cirrhosis-related genes identified by TWAS. Considering the small number of genes to be analyzed, we set a relatively relaxed significance threshold of FDR<0.1. In addition, we used the clusterProfiler package to perform gene set enrichment analysis (GSEA) on differentially expressed genes in HCC samples versus adjacent samples. The pathway reached a threshold of FDR<0.05 was considered significant.
The flowchart of the study is shown in Supplementary Material (Figure 1). We evaluated the association between predicted gene expression and cirrhosis using FUSION. Totally, we found that ten genes across four tissues were significantly associated with cirrhosis after the Bonferroni correction (Figure 2; Table 1, Supplementary Table S1). Specifically, in liver tissue, we defined SKIV2L at 6p21.33 (Padjusted = 0.011) and JPH4 at 14q11.2 (Padjusted = 0.019) as cirrhosis-related genes, while in pancreas tissue, we found UQCC2 at 6p21.31 (Padjusted = 0.027), RP11-91I8.3 (Padjusted = 0.041), and MAU2 at 19p13.11 (Padjusted = 0.047) significantly associated with cirrhosis. Other significant genes included ERAP1 at 5q15 (Padjusted = 0.019) and PUS3 at 11q24.2 (Padjusted = 0.018) in spleen tissue and ZNF677 at 19q13.42 (Padjusted = 0.029), ARHGAP40 at 20q11.23 (Padjusted = 0.017), and SHANK3 at 22q13.33 (Padjusted = 0.030) in thyroid tissue. We did not define genes expressed in whole blood as significantly associated with cirrhosis.
We used RNA-seq data from TCGA to explore genes whose expression was significantly associated with HCC. With the threshold of | log2 (fold change) |≥1 and FDR-adjusted p-value<0.01, we totally defined 7,163 differentially expressed genes in HCC samples versus adjacent samples, including 1,631 upregulated genes and 5,532 downregulated genes (Supplementary Table S2). Notably, five genes identified by TWAS overlapped with these DEGs, four of which were significantly downregulated, including UQCC2, MAU2, SHANK3, and SKIV2L, while the remaining one, JPH4, was significantly upregulated (Supplementary Table S3).
To explore the potential biological mechanisms underlying the identified associations, we first applied functional enrichment analyses to cirrhosis-related genes identified by TWAS. We found that these 10 genes were significantly enriched in several GO terms, including learning and nuclear-transcribed mRNA catabolic process, exonucleolytic, 3′-5' (Figure 3), and three KEGG pathways, including RNA degradation, glutamatergic synapse, and herpes simplex virus type 1 infection (Supplementary Figure S1). Then, we performed GSEA based on the differentially expressed gene sets between cancer and adjacent samples and obtained 631 GO terms (Supplementary Table S4) and significantly differentially enriched 88 KEGG pathways (Supplementary Table S5). We found that learning and nuclear-transcribed mRNA catabolic process, exonucleolytic, and 3′-5′ were among the differentially enriched GO terms (Supplementary Figure S2), while RNA degradation and herpes simplex virus type 1 infection were among the differentially enriched KEGG pathways (Supplementary Figure S3).
In this study, we used tissue-specific TWAS to explore the association of genetically predicted gene expression with cirrhosis in European ancestry and identified 10 genes in four tissues as potential cirrhosis-related genes, including two in the liver (SKIV2L and JPH4), three in the pancreas (UQCC2, RP11-91I8.3, and MAU2), two in the spleen (ERAP1 and PUS3), and three in thyroid tissue (ZNF677, ARHGAP40, and SHANK3). The aforementioned ten genes have never been clearly reported to be associated with cirrhosis. In addition, the expression of five of the 10 identified genes, including JPH4, UQCC2, MAU2, SHANK3, and SKIV2L, also showed significant changes in HCC samples compared to the adjacent liver samples.
SKIV2L is located at 6p21.33 and encodes a gene for helicase, which is a component of the SKI complex (Yang et al., 1998). The SKI complex is thought to be involved in exosome-mediated RNA decay and associated with transcriptionally active genes (Synowsky and Heck, 2008). Genetic variations in SKIV2L are known to be associated with the occurrence of trichohepatoenteric syndrome (Vardi et al., 2018), an autosomal recessive genetic disease that may cause immune dysfunction and liver abnormalities (Fabre et al., 1993). A number of GWAS have shown that SKIV2L is associated with the risk of chronic hepatitis B virus infection (Jiang et al., 2015) and chronic hepatitis C virus infection (Sakaue et al., 2021), two major causes of cirrhosis (Bedossa, 2015) as well as liver damage (Nicoletti et al., 2019) and liver tumors (Sakaue et al., 2021). Experimental studies have shown that SKIV2L, a member of the NSDK, could specifically regulate the production of type I interferon (Zhou et al., 2019). It may fine-tune antiviral responses through RIG1/MDA5 to prevent excessive inflammation that may lead to tissue damage (Eckard et al., 2014). Therefore, deletion or partial functional deficiency of SkIV2L may cause uncontrolled or dysregulated production of RLR ligands or sensors resulting in increased autoinflammatory responses in the absence of viral infection or increased susceptibility to systemic autoimmune diseases (Fernando et al., 2007; McKay et al., 2009; Yoneyama et al., 2014). Another cirrhosis-related gene, JPH4, found in liver tissue, is located at 14q11.2. JPH4 is previously reported as brain-specific and appears to have an active role in certain neurons involved in motor coordination and memory (Hogea et al., 2021). Another research showed that JPH4 is associated with the prognosis of renal cell carcinoma (Zhang et al., 2020). At present, there are few research reports on the JPH4 gene and diseases. Efforts may have to be made to validate its role in liver-related diseases.
In addition, we need to pay attention to the significant genes MAU2 and UQCC2 in pancreatic tissue and the significant gene SHANK3 in thyroid tissue. The results of our analysis show that these three genes are all under-expressed in liver cancer tissue. They are located at 19p13.11, 6p21.31, and 22q13.33, respectively. MAU2 is a regulatory subunit that constitutes cohesin, a highly conserved protein complex that plays an important role in sister chromatid cohesion, chromatin structure, gene expression, and DNA repair (Minina et al., 2017). Recent studies have shown that the gene encoding the cohesin subunit is somatically mutated in a variety of human cancers (Hill et al., 2016). Knockdown of CDCA5, another regulatory subunit of cohesin, can induce liver cancer (Chen et al., 2019). The results of our study may also reveal the role of the cohesin subunit of MAU2 in liver cancer. UQCC2, another prominent gene in pancreatic tissue, is a nuclear-encoded gene for respiratory chain complex III subunits. Studies have shown that mutations in coding genes can lead to respiratory complex III deficiency (Lonlay et al., 2001), which in turn leads to liver failure (Iwama et al., 2011). Therefore, it can be speculated that abnormal expression of the UQCC2 gene can lead to liver damage and cirrhosis by affecting the metabolic process of the respiratory chain. Given that UQCC2 is a downregulated gene in liver cancer tissue, it can be speculated that the damage caused by this metabolism contributes to the occurrence of liver cancer. There is also a significant gene in thyroid tissue, i.e., SHANK3, which is also under-expressed in liver cancer tissue. However, research on SHANK3 has mainly focused on the occurrence of autism (Uchino and Waga, 2015; Li et al., 2018), with little mention of the liver. As far as we know, in the advanced stage of cirrhosis, hepatic encephalopathy may occur, which is manifested as cerebral confusion. Therefore, we do not know the connection between the process of cirrhosis and the brain, which is an interesting association and worth exploring.
In addition to the aforementioned genes validated in liver cancer tissues, we also identified four candidate genes (ZNF677, ARHGAP40, ERAP1, and PUS3) in thyroid and spleen tissues, of which ERAP1 has been reported to be associated with the development of sclerosing cholangitis, a disease that may progress to cirrhosis (Ellinghaus et al., 2016). Other genes have also been demonstrated to be associated with cancers. For example, ZNF677 has been reported to be associated with the occurrence of non-small-cell lung cancer (Heller et al., 2015) and thyroid cancer (Siraj et al., 2021); ARHGAP40 may affect the development of laryngeal squamous cell carcinoma (Wang et al., 2021); the splicing event of the PUS3 gene at 11q24.2 has also been reported to be involved in a variety of cancers (Ajiro et al., 2016). Though few studies on these three genes and liver-related diseases are available now, the potential association may deserve more attention in the future research study.
Functional enrichment analysis found that the 10 genes identified by TWAS were significantly enriched in the catabolism process of nuclear-transcribed mRNA, and the GSEA results of the expression data of liver cancer tissue and adjacent tissue in this study also suggested that the set of genes for the degradation process of transcribed mRNAs is under-expressed in liver cancer tissues. This suggests that the occurrence of cirrhosis may be related to the transcriptional process of susceptibility genes.
There are some limitations to this study. First, our results were only been validated for differential genes in the liver cancer dataset and lacked revalidation results on other liver cirrhosis datasets. Moreover, there is still a certain gap between the disease pathology of liver cirrhosis and liver cancer, which may make our results less specific for liver cirrhosis. Also, the mechanism of susceptibility genes leading to the development of liver pathology is still unclear and needs further exploration. Second, this study is a multi-tissue TWAS analysis; however, there is no overlap of susceptibility genes identified in different tissues. In particular, susceptibility genes in non-liver tissue were not identified in liver tissue as well. Therefore, the results of TWAS must be interpreted with caution for cross-tissue results, especially for genes that are significant in other tissues.
In conclusion, our results present a list of genes that have not been previously focused on cirrhosis, some of which were also validated by differential expression analysis. Functional enrichment analysis, combined with previous knowledge of these identified genes, implies that they may contribute to cirrhosis through the transcriptional process of susceptibility genes and the process of RNA degradation. Our study may further expand our understanding of the pathogenesis of cirrhosis and hepatocellular carcinoma.
Publicly available datasets were analyzed in this study. These data can be found at: GWAS summary data for cirrhosis (http://geneatlas.roslin.ed.ac.uk/), LD reference panel of European from the 1,000 Genome Project (https://data.broadinstitute.org/alkesgroup/FUSION/LDREF.tar.bz2), the GTEx V7-based functional weights for each tissue (https://gtexportal.org/home/), and gene expression data for hepatocellular carcinoma (https://www.cancer.gov/about-nci/organization/ccg/research/structural-genomics/tcga).
PH and R-BY designed the study. X-BZ and X-YY performed the dataset quality control. SY, Y-XZ, and X-YY performed the data analysis. PH, Y-QH, and X-BZ interpreted the analysis results. X-YY and Y-QH wrote the draft of the manuscript. R-BY revised the manuscript. All authors accepted the final manuscript.
This work was supported in part by the Open Research Fund Program of the State Key Laboratory of Virology of China (2021KF008), the Natural Science Foundation of Yunnan Province (2019FA005), and the Priority Academic Program Development of Jiangsu Higher Education Institutions (PAPD).
The authors declare that the research was conducted in the absence of any commercial or financial relationships that could be construed as a potential conflict of interest.
All claims expressed in this article are solely those of the authors and do not necessarily represent those of their affiliated organizations, or those of the publisher, the editors, and the reviewers. Any product that may be evaluated in this article, or claim that may be made by its manufacturer, is not guaranteed or endorsed by the publisher.
We acknowledge the participants and investigators of GWAS-ATLAS for making the summary data, the Genotype-Tissue Expression (GTEx) project for making the expression data of each tissue, and The Cancer Genome Atlas program for making the expression data for hepatocellular carcinoma publicly available for us.
The Supplementary Material for this article can be found online at: https://www.frontiersin.org/articles/10.3389/fgene.2022.878607/full#supplementary-material
Ajiro, M., Jia, R., Yang, Y., Zhu, J., and Zheng, Z.-M. (2016). A Genome Landscape of SRSF3-Regulated Splicing Events and Gene Expression in Human Osteosarcoma U2OS Cells. Nucleic Acids Res. 44, 1854–1870. doi:10.1093/nar/gkv1500
Ally, A., Balasundaram, M., Carlsen, R., Chuah, E., Clarke, A., Dhalla, N., et al. (2017). Comprehensive and Integrative Genomic Characterization of Hepatocellular Carcinoma. Cell 169, 1327–e23. e23. doi:10.1016/j.cell.2017.05.046
Arroyo, V., Angeli, P., Moreau, R., Jalan, R., Clària, J., Trebicka, J., et al. (2021). The Systemic Inflammation Hypothesis: Towards a New Paradigm of Acute Decompensation and Multiorgan Failure in Cirrhosis. J. Hepatol. 74, 670–685. doi:10.1016/j.jhep.2020.11.048
Bajaj, J. S., Fan, S., Thacker, L. R., Fagan, A., Gavis, E., White, M. B., et al. (2019). Serum and Urinary Metabolomics and Outcomes in Cirrhosis. PLoS One 14, e0223061. doi:10.1371/journal.pone.0223061
Basyte-Bacevice, V., Skieceviciene, J., Valantiene, I., Sumskiene, J., Petrenkiene, V., Kondrackiene, J., et al. (2019). TM6SF2 and MBOAT7 Gene Variants in Liver Fibrosis and Cirrhosis. Ijms 20, 1277. doi:10.3390/ijms20061277
Bedossa, P. (2015). Reversibility of Hepatitis B Virus Cirrhosis after Therapy: Who and Why? Liver Int. 35 (Suppl. 1), 78–81. doi:10.1111/liv.12710
Bossé, Y., Li, Z., Xia, J., Manem, V., Carreras‐Torres, R., Gabriel, A., et al. (2020). Transcriptome‐wide Association Study Reveals Candidate Causal Genes for Lung Cancer. Int. J. Cancer 146, 1862–1878. doi:10.1002/ijc.32771
Canela-Xandri, O., Rawlik, K., and Tenesa, A. (2018). An Atlas of Genetic Associations in UK Biobank. Nat. Genet. 50, 1593–1599. doi:10.1038/s41588-018-0248-z
Chen, H., Chen, J., Zhao, L., Song, W., Xuan, Z., Chen, J., et al. (2019). CDCA5, Transcribed by E2F1, Promotes Oncogenesis by Enhancing Cell Proliferation and Inhibiting Apoptosis via the AKT Pathway in Hepatocellular Carcinoma. J. Cancer 10, 1846–1854. doi:10.7150/jca.28809
de Lonlay, P., Valnot, I., Barrientos, A., Gorbatyuk, M., Tzagoloff, A., Taanman, J.-W., et al. (2001). A Mutant Mitochondrial Respiratory Chain Assembly Protein Causes Complex III Deficiency in Patients with Tubulopathy, Encephalopathy and Liver Failure. Nat. Genet. 29, 57–60. doi:10.1038/ng706
Derks, E. M., and Gamazon, E. R. (2020). Transcriptome‐wide Association Analysis Offers Novel Opportunities for Clinical Translation of Genetic Discoveries on Mental Disorders. World Psychiatry 19, 113–114. doi:10.1002/wps.20702
Eckard, S. C., Rice, G. I., Fabre, A., Badens, C., Gray, E. E., Hartley, J. L., et al. (2014). The SKIV2L RNA Exosome Limits Activation of the RIG-I-like Receptors. Nat. Immunol. 15, 839–845. doi:10.1038/ni.2948
Ellinghaus, D., Jostins, L., Spain, S. L., Cortes, A., Bethune, J., Han, B., et al. (2016). Analysis of Five Chronic Inflammatory Diseases Identifies 27 New Associations and Highlights Disease-Specific Patterns at Shared Loci. Nature Genetics 48, 510–518. doi:10.1038/ng.3528
Emdin, C. A., Haas, M., Ajmera, V., Simon, T. G., Homburger, J., Neben, C., et al. (2021). Association of Genetic Variation with Cirrhosis: A Multi-Trait Genome-wide Association and Gene-Environment Interaction Study. Gastroenterology 160, 1620–1633. e13. doi:10.1053/j.gastro.2020.12.011
Epstein, O., Chapman, R. W. G., Lake-Bakaar, G., Foo, A. Y., Rosalki, S. B., and Sherlock, S. (1982). The Pancreas in Primary Biliary Cirrhosis and Primary Sclerosing Cholanitis. Gastroenterology 83, 1177–1182. doi:10.1016/s0016-5085(82)80125-x
Fabre, A., Bourgeois, P., Chaix, C., Bertaux, K., Goulet, O., and Badens, C. (1993). GeneReviews®: Trichohepatoenteric Syndrome. Seattle (WA).
Fabris, C., Toniutto, P., Falleti, E., Fontanini, E., Cussigh, A., Bitetto, D., et al. (2009). MTHFR C677T Polymorphism and Risk of HCC in Patients with Liver Cirrhosis: Role of Male Gender and Alcohol Consumption. Alcohol. Clin. Exp. Res. 33, 102–107. doi:10.1111/j.1530-0277.2008.00816.x
Fernando, M. M. A., Stevens, C. R., Sabeti, P. C., Walsh, E. C., McWhinnie, A. J. M., Shah, A., et al. (2007). Identification of Two Independent Risk Factors for Lupus within the MHC in United Kingdom Families. Plos Genet. 3, e192. doi:10.1371/journal.pgen.0030192
Fofiu, R., Bende, F., Popescu, A., Şirli, R., Lupușoru, R., Ghiuchici, A.-M., et al. (2021). Spleen and Liver Stiffness for Predicting High-Risk Varices in Patients with Compensated Liver Cirrhosis. Ultrasound Med. Biol. 47, 76–83. doi:10.1016/j.ultrasmedbio.2020.09.004
Fukui, H., Saito, H., Ueno, Y., Uto, H., Obara, K., Sakaida, I., et al. (2016). Evidence-based Clinical Practice Guidelines for Liver Cirrhosis 2015. J. Gastroenterol. 51, 629–650. doi:10.1007/s00535-016-1216-y
Garcia‐Tsao, G., Abraldes, J. G., Berzigotti, A., and Bosch, J. (2017). Portal Hypertensive Bleeding in Cirrhosis: Risk Stratification, Diagnosis, and Management: 2016 Practice Guidance by the American Association for the Study of Liver Diseases. Hepatology 65, 310–335. doi:10.1002/hep.28906
Gong, W., Guo, P., Liu, L., Guan, Q., and Yuan, Z. (2020). Integrative Analysis of Transcriptome-wide Association Study and mRNA Expression Profiles Identifies Candidate Genes Associated with Idiopathic Pulmonary Fibrosis. Front. Genet. 11, 604324. doi:10.3389/fgene.2020.604324
Gusev, A., Ko, A., Shi, H., Bhatia, G., Chung, W., Penninx, B. W. J. H., et al. (2016). Integrative Approaches for Large-Scale Transcriptome-wide Association Studies. Nat. Genet. 48, 245–252. doi:10.1038/ng.3506
Heller, G., Altenberger, C., Schmid, B., Marhold, M., Tomasich, E., Ziegler, B., et al. (2015). DNA Methylation Transcriptionally Regulates the Putative Tumor Cell Growth Suppressor ZNF677 in Non-small Cell Lung Cancers. Oncotarget 6, 394–408. doi:10.18632/oncotarget.2697
Hill, V. K., Kim, J.-S., and Waldman, T. (2016). Cohesin Mutations in Human Cancer. Biochim. Biophys. Acta (Bba) - Rev. Cancer 1866, 1–11. doi:10.1016/j.bbcan.2016.05.002
Hogea, A., Shah, S., Jones, F., Carver, C. M., Hao, H., Liang, C., et al. (2021). Junctophilin‐4 Facilitates Inflammatory Signalling at Plasma Membrane‐endoplasmic Reticulum Junctions in Sensory Neurons. J. Physiol. 599, 2103–2123. doi:10.1113/JP281331
Huang, P., Hou, Y., Zou, Y., Ye, X., Yu, R., and Yang, S. (2021). The Causal Effects of Primary Biliary Cholangitis on Thyroid Dysfunction: A Two-Sample Mendelian Randomization Study. Front. Genet. 12, 791778. doi:10.3389/fgene.2021.791778
Innes, H., Buch, S., Hutchinson, S., Guha, I. N., Morling, J. R., Barnes, E., et al. (2020). Genome-Wide Association Study for Alcohol-Related Cirrhosis Identifies Risk Loci in MARC1 and HNRNPUL1. Gastroenterology 159, 1276–1289. e7. doi:10.1053/j.gastro.2020.06.014
Iwama, I., Baba, Y., Kagimoto, S., Kishimoto, H., Kasahara, M., Murayama, K., et al. (2011). Case Report of a Successful Liver Transplantation for Acute Liver Failure Due to Mitochondrial Respiratory Chain Complex III Deficiency. Transplant. Proc. 43, 4025–4028. doi:10.1016/j.transproceed.2011.09.042
Jiang, D. K., Ma, X. P., Yu, H., Cao, G., Ding, D. L., Chen, H., et al. (2015). Genetic Variants in Five Novel Loci Including CFB and CD40 Predispose to Chronic Hepatitis B. Hepatology 62, 118–128. doi:10.1002/hep.27794
Kupcinskas, J., Valantiene, I., Varkalaitė, G., Steponaitiene, R., Skieceviciene, J., Sumskiene, J., et al. (2017). PNPLA3 and RNF7 Gene Variants Are Associated with the Risk of Developing Liver Fibrosis and Cirrhosis in an Eastern European Population. Jgld 26, 37–43. doi:10.15403/jgld.2014.1121.261.pnp
Li, Y., Jia, X., Wu, H., Xun, G., Ou, J., Zhang, Q., et al. (2018). Genotype and Phenotype Correlations for SHANK3 De Novo Mutations in Neurodevelopmental Disorders. Am. J. Med. Genet. 176, 2668–2676. doi:10.1002/ajmg.a.40666
Liu, D., Zhou, D., Sun, Y., Zhu, J., Ghoneim, D., Wu, C., et al. (2020). A Transcriptome-wide Association Study Identifies Candidate Susceptibility Genes for Pancreatic Cancer Risk. Cancer Res. 80, 4346–4354. doi:10.1158/0008-5472.CAN-20-1353
Lozano, R., Naghavi, M., Foreman, K., Lim, S., Naghavi, M., Foreman, K., et al. (2012). Global and Regional Mortality From 235 Causes of Death for 20 Age Groups in 1990 and 2010: A Systematic Analysis for the Global Burden of Disease Study 2010. The Lancet 380, 2095–2128. doi:10.1016/S0140-6736(12)61728-0
Marees, A. T., de Kluiver, H., Stringer, S., Vorspan, F., Curis, E., Marie-Claire, C., et al. (2018). A Tutorial on Conducting Genome-wide Association Studies: Quality Control and Statistical Analysis. Int. J. Methods Psychiatr. Res. 27, e1608. doi:10.1002/mpr.1608
Maurano, M. T., Humbert, R., Rynes, E., Thurman, R. E., Haugen, E., Wang, H., et al. (2012). Systematic Localization of Common Disease-Associated Variation in Regulatory DNA. Science 337, 1190–1195. doi:10.1126/science.1222794
McKay, G. J., Silvestri, G., Patterson, C. C., Hogg, R. E., Chakravarthy, U., and Hughes, A. E. (2009). Further Assessment of the Complement Component 2 and Factor B Region Associated with Age-Related Macular Degeneration. Invest. Ophthalmol. Vis. Sci. 50, 533–539. doi:10.1167/iovs.08-2275
Minina, E. A., Reza, S. H., Gutierrez-Beltran, E., Elander, P. H., Bozhkov, P. V., and Moschou, P. N. (2017). Arabidopsis Homologue of Scc4/MAU2 Is Essential for Plant Embryogenesis. J. Cel Sci 130, 1051–1063. doi:10.1242/jcs.196865
Mokdad, A. A., Lopez, A. D., Shahraz, S., Lozano, R., Mokdad, A. H., Stanaway, J., et al. (2014). Liver Cirrhosis Mortality in 187 Countries between 1980 and 2010: a Systematic Analysis. BMC Med. 12, 145. doi:10.1186/s12916-014-0145-y
Nicoletti, P., Aithal, G. P., Chamberlain, T. C., Coulthard, S., Alshabeeb, M., Grove, J. I., et al. (2019). Drug‐Induced Liver Injury Due to Flucloxacillin: Relevance of Multiple Human Leukocyte Antigen Alleles. Clin. Pharmacol. Ther. 106, 245–253. doi:10.1002/cpt.1375
Ritchie, M. E., Phipson, B., Di, W., Hu, Y., Law, C. W., Shi, W., et al. (2015). Limma Powers Differential Expression Analyses for RNA-Sequencing and Microarray StudiesNucleic Acids Research 43, e47. doi:10.1093/nar/gkv007
Roerecke, M., Vafaei, A., Hasan, O. S. M., Chrystoja, B. R., Cruz, M., Lee, R., et al. (2019). Alcohol Consumption and Risk of Liver Cirrhosis: A Systematic Review and Meta-Analysis. Am. J. Gastroenterol. 114, 1574–1586. doi:10.14309/ajg.0000000000000340
Sakaue, S., Kanai, M., Tanigawa, Y., Karjalainen, J., Kurki, M., Koshiba, S., et al. (2021). A Cross-Population Atlas of Genetic Associations for 220 Human Phenotypes. Nat. Genet. 53, 1415–1424. doi:10.1038/s41588-021-00931-x
Siraj, A. K., Poyil, P. K., Parvathareddy, S. K., Alobaisi, K., Ahmed, S. O., Al-Sobhi, S. S., et al. (2021). Loss of ZNF677 Expression Is an Independent Predictor for Distant Metastasis in Middle Eastern Papillary Thyroid Carcinoma Patients. Ijms 22, 7833. doi:10.3390/ijms22157833
Speed, D., Holmes, J., and Balding, D. J. (2020). Evaluating and Improving Heritability Models Using Summary Statistics. Nat. Genet. 52, 458–462. doi:10.1038/s41588-020-0600-y
Synowsky, S. A., and Heck, A. J. R. (2008). The Yeast Ski Complex Is a Hetero-Tetramer. Protein Sci. 17, 119–125. doi:10.1110/ps.073155908
Tam, V., Patel, N., Turcotte, M., Bossé, Y., Paré, G., and Meyre, D. (2019). Benefits and Limitations of Genome-wide Association Studies. Nat. Rev. Genet. 20, 467–484. doi:10.1038/s41576-019-0127-1
Tibshirani, R. (1996). Regression Shrinkage and Selection via the Lasso. J. R. Stat. Soc. Ser. B (Methodological) 58, 267–288. doi:10.1111/j.2517-6161.1996.tb02080.x
Tsochatzis, E. A., Bosch, J., and Burroughs, A. K. (2014). Liver Cirrhosis. The Lancet 383, 1749–1761. doi:10.1016/S0140-6736(14)60121-5
Uchino, S., and Waga, C. (2015). Novel Therapeutic Approach for Autism Spectrum Disorder: Focus on SHANK3. Cn 13, 786–792. doi:10.2174/1570159x13666151029105547
Vardi, I., Barel, O., Sperber, M., Schvimer, M., Nunberg, M., Field, M., et al. (2018). Genetic and Structural Analysis of a SKIV2L Mutation Causing Tricho-Hepato-Enteric Syndrome. Dig. Dis. Sci. 63, 1192–1199. doi:10.1007/s10620-018-4983-x
Veturi, Y., and Ritchie, M. D. (2018). How Powerful Are Summary-Based Methods for Identifying Expression-Trait Associations under Different Genetic Architectures? Pac. Symp. Biocomput 23, 228. doi:10.1142/9789813235533_0021
Villanueva, A. (2019). Hepatocellular Carcinoma. N. Engl. J. Med. 380, 1450–1462. doi:10.1056/NEJMra1713263
Wainberg, M., Sinnott-Armstrong, N., Mancuso, N., Barbeira, A. N., Knowles, D. A., Golan, D., et al. (2019). Opportunities and Challenges for Transcriptome-wide Association Studies. Nat. Genet. 51, 592–599. doi:10.1038/s41588-019-0385-z
Wang, J., Liu, D., Gu, Y., Zhou, H., Li, H., Shen, X., et al. (2021). Potential Prognostic Markers and Significant lncRNA-mRNA Co-expression Pairs in Laryngeal Squamous Cell Carcinoma. Open Life Sci. 16, 544–557. doi:10.1515/biol-2021-0052
Yang, Z., Shen, L., Dangel, A. W., Wu, L.-C., and Yu, C. Y. (1998). Four Ubiquitously Expressed Genes,RD(D6S45)-SKI2W(SKIV2L)-DOM3Z-RP1(D6S60E), Are Present between Complement Component Genes FactorBandC4in the Class III Region of the HLA. Genomics 53, 338–347. doi:10.1006/geno.1998.5499
Yoneyama, S., Sakurada, Y., Mabuchi, F., Sugiyama, A., Kubota, T., and Iijima, H. (2014). Genetic Variants in the SKIV2L Gene in Exudative Age-Related Macular Degeneration in the Japanese Population. Ophthalmic Genet. 35, 151–155. doi:10.3109/13816810.2014.921313
Zhang, C., Wang, F., Guo, F., Ye, C., Yang, Y., Huang, Y., et al. (2020). A 13-gene Risk Score System and a Nomogram Survival Model for Predicting the Prognosis of clear Cell Renal Cell Carcinoma. Urol. Oncol. Semin. Original Invest. 38, e1. doi:10.1016/j.urolonc.2019.12.022
Zhou, D., Lai, M., Luo, A., and Yu, C.-Y. (2019). An RNA Metabolism and Surveillance Quartet in the Major Histocompatibility Complex. Cells 8, 1008. doi:10.3390/cells8091008
Zhou, X., Carbonetto, P., and Stephens, M. (2013). Polygenic Modeling with Bayesian Sparse Linear Mixed Models. Plos Genet. 9, e1003264. doi:10.1371/journal.pgen.1003264
Zhu, M., Fan, J., Zhang, C., Xu, J., Yin, R., Zhang, E., et al. (2021). A Cross-Tissue Transcriptome-wide Association Study Identifies Novel Susceptibility Genes for Lung Cancer in Chinese Populations. Hum. Mol. Genet. 30, 1666–1676. doi:10.1093/hmg/ddab119
Keywords: transcriptome-wide association study, cirrhosis, multiple tissues, genome-wide association studies, gene expression
Citation: Zhu X-B, Hou Y-Q, Ye X-Y, Zou Y-X, Xia X-S, Yang S, Huang P and Yu R-B (2022) Identifying and Exploring the Candidate Susceptibility Genes of Cirrhosis Using the Multi-Tissue Transcriptome-Wide Association Study. Front. Genet. 13:878607. doi: 10.3389/fgene.2022.878607
Received: 18 February 2022; Accepted: 30 March 2022;
Published: 13 May 2022.
Edited by:
Jiadong Ji, Shandong University, ChinaReviewed by:
Xuesi Dong, Southeast University, ChinaCopyright © 2022 Zhu, Hou, Ye, Zou, Xia, Yang, Huang and Yu. This is an open-access article distributed under the terms of the Creative Commons Attribution License (CC BY). The use, distribution or reproduction in other forums is permitted, provided the original author(s) and the copyright owner(s) are credited and that the original publication in this journal is cited, in accordance with accepted academic practice. No use, distribution or reproduction is permitted which does not comply with these terms.
*Correspondence: Peng Huang, aHVhbmdwZW5nQG5qbXUuZWR1LmNu; Rong-Bin Yu, cm9uZ2Jpbnl1QG5qbXUuZWR1LmNu
†These authors have contributed equally to this work
Disclaimer: All claims expressed in this article are solely those of the authors and do not necessarily represent those of their affiliated organizations, or those of the publisher, the editors and the reviewers. Any product that may be evaluated in this article or claim that may be made by its manufacturer is not guaranteed or endorsed by the publisher.
Research integrity at Frontiers
Learn more about the work of our research integrity team to safeguard the quality of each article we publish.