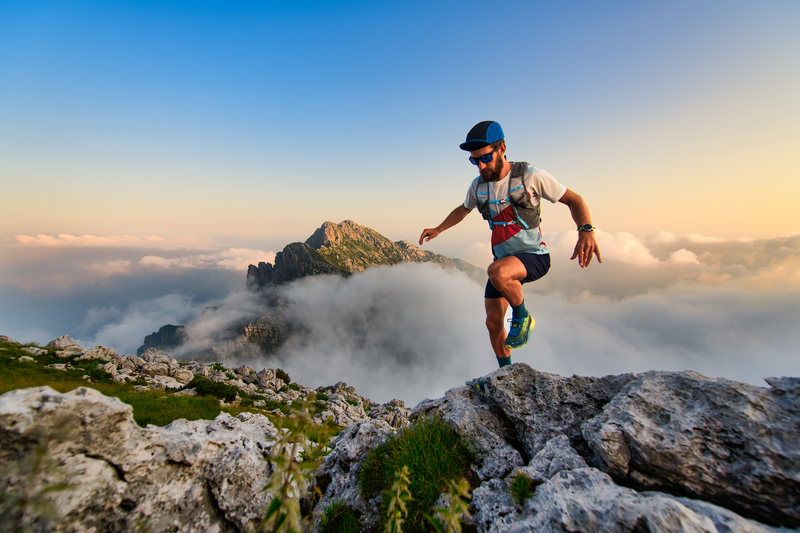
95% of researchers rate our articles as excellent or good
Learn more about the work of our research integrity team to safeguard the quality of each article we publish.
Find out more
ORIGINAL RESEARCH article
Front. Genet. , 24 March 2022
Sec. Computational Genomics
Volume 13 - 2022 | https://doi.org/10.3389/fgene.2022.876514
This article is part of the Research Topic Methods and Applications: Computational Genomics View all 43 articles
As a multifaceted syndrome, sepsis leads to high risk of death worldwide. It is difficult to be intervened due to insufficient biomarkers and potential targets. The reason is that regulatory mechanisms during sepsis are poorly understood. In this study, expression profiles of sepsis from GSE134347 were integrated to construct gene interaction network through weighted gene co-expression network analysis (WGCNA). R package DiffCorr was utilized to evaluate differential correlations and identify significant differences between sepsis and healthy tissues. As a result, twenty-six modules were detected in the network, among which blue and darkred modules exhibited the most significant associations with sepsis. Finally, we identified some novel genes with opposite correlations including ZNF366, ZMYND11, SVIP and UBE2H. Further biological analysis revealed their promising roles in sepsis management. Hence, differential correlations-based algorithm was firstly established for the discovery of appealing regulators in sepsis.
Sepsis and septic shock with subsequent multi-organ failure contribute to the leading causes of death among patients in adult intensive care unit (ICU), which are due to massive inflammatory responses to infection (Abe et al., 2020; Markwart et al., 2020). The incidence of sepsis is approximately 20 million cases each year with 30–50% high mortality in the United States (Fleischmann et al., 2016). Advances in understanding pathophysiology of sepsis reveal that it occurs not only with inflammation-related responses but also modifications in non-immunological pathways (Rello et al., 2017; Yu et al., 2021). Despite great improvement in surgery, pharmacological approaches and serum biomarkers including procalcitonin (PCT), C-reactive protein (CRP), lactate and cell-free DNA has been made in initial detection and therapy of sepsis, the incidence and mortality rates are still rising rapidly due to complexity of sepsis and lack of targeted drugs (Povoa et al., 2005; Saukkonen et al., 2008; Riedel et al., 2011; Rhee et al., 2015). Thus, novel risk genes and related regulatory networks need to be identified to illustrate sepsis etiology and direct researchers to develop effective therapeutic strategies.
Accumulating studies have used transcriptome data comprised of cellular components contents between disease and healthy tissues to decipher potential molecular mechanisms of sepsis (Zhang et al., 2020a; Fang et al., 2021; Yu et al., 2021). Meanwhile, most of these studies incorporated differentially expressed genes and gene correlation data to explore gene interaction networks, followed by enrichment analysis to clarify function of unknown genes (Balamuth et al., 2020; Zhai et al., 2020; Zhang et al., 2020b). Nevertheless, the biggest limitation is that the observed gene correlations may be redundant because they appeared in both two states, adding difficulties in the discovery of true causative genes. The solution to this problem comes to the identification of differential correlations referring to alterations of correlated patterns under different conditions (Ideker and Krogan, 2012; Li et al., 2015; Zhou et al., 2021). Currently, differential correlations in sepsis have been poorly understood, hence it is in urgent need to orchestrate network dynamics for identifying novel candidate genes.
Here, an in silico framework was proposed to identify hub genes with differential correlations in sepsis (Figure 1A). First, the networks of gene expression were constructed using weighted gene co-expression network analysis (WGCNA), which found correlated genes based on gene connectivity and formed gene modules (Ghazalpour et al., 2006; Yang et al., 2018). Twenty-six modules were detected in the network, among which blue and darkred modules exhibited the most significant associations with sepsis. Next, differential correlations of genes in these two modules were calculated and significant differences between sepsis and healthy tissues utilizing R package DiffCorr were identified. Finally, we identified some novel genes including ZNF366, ZMYND11, SVIP and UBE2H. Further biological analysis revealed their promising roles in sepsis management.
FIGURE 1. Clustering dendrogram of sepsis and healthy tissues. (A) The workflow of this study. (B) Clustering dendrogram of 156 patients with sepsis and 82 healthy subjects and trait heatmap. (C) The relationship between soft threshold (power) and network properties. Left panel: The relationship between soft-threshold (power) and scale-free topology. Right panel: The relationship between soft threshold (power) and mean connectivity.
The HTA2.0 microarray data of 156 patients with sepsis and 82 healthy subjects was downloaded from GEO with primary data accession number GSE134347 (https://www.ncbi.nlm.nih.gov/geo/query/acc.cgi?acc=GSE134347). Patient characteristics were described in (Scicluna et al., 2020).
Screening of correlated gene pairs was performed by an R package WGCNA (Langfelder and Horvath, 2008). And step-by-step calculation was as follows:
Step 1 The Pearson correlation coefficient between gene Xi and Xj was calculated, and matrix X was converted into the correlation matrix S between genes:
Step 2 Weighted adjacency matrix aij was constructed and suitable soft-threshold power β was selected:
Step 3 The degree of separation of nodes was calculated. The adjacency matrix is converted into unsigned topological overlap matrix (TOM) to calculate degree of intergenic dissimilarity (Zhang and Horvath, 2005), based on which genes were distributed in different modules:
In the above formula, lij represented the sum of product of adjacency coefficients of all common adjacent genes of gene i and j. aij represented the adjacency coefficient between gene i and j. ki represented synthesis of adjacency coefficients of gene i with all neighboring nodes. If TOMij was 0, it meant that gene i and j were isolated and not connected to all other genes. If TOMij was 1, it meant that these two genes were adjacent to all surrounding genes and were also connected to each other. In other words, TOM represented the similarity of genes, so the dissimilarity between genes could be calculated:
Step 4 DistTOMij was used for hierarchical clustering, and genes were divided into different co-expression modules (Li and Horvath, 2007). WGCNA adopted Dynamic Tree Cut Method to construct the cluster tree, which was a top-down merging algorithm. Through iteration and decomposition of gene clusters, stable gene clusters were eventually achieved (Langfelder et al., 2008). Here, the minimum module size was set as 30 to identify modules and draw dendrogram.
Step 5 Identify trait-related modules. Herein, trait was defined as disease. We defined gene significance (GS) as relationship between gene expression levels and disease. Moreover, module membership (MM) represented the degree of relationship between module feature genes and disease. The higher MM represented the higher correlation between modules and disease. At last, we identified two modules most relevant to disease. One module exhibited positive correlation and another negative correlation.
R package DiffCorr was utilized for the visualization and identification of differential correlations in biological networks. This package was based on Fisher’s z-test and details were explained in (Fukushima, 2013; Zhou et al., 2021).
R package clusterProfiler was implemented to conduct enrichment analysis of clustered genes in blue and darkred modules. We used a hypergeometric distribution test for the classification of enrichment terms. And p values were adjusted by false discovery rate (FDR) method, the cutoff of which was set to be 0.05 (Yu et al., 2012).
Cytoscape (3.9.0) was used to realize visualization of networks (Doncheva et al., 2019).
We applied Student’s t-test to identify genes differentially expressed between sepsis and healthy samples. p values were adjusted by the Benjamini–Hochberg method (Hochberg and Benjamini, 1990). Differentially expressed genes were defined as adjusted p value less than 0.05. We employed Fisher’s z-test to evaluate differential correlations of gene pairs between sepsis and healthy patients. And lfdr less than 0.05 was regarded as significant differential correlations.
Pearson’s correlation coefficient was applied to cluster samples from GSE134347. After removing outliers, a sample clustering tree was drawn (Figure 1B). Co-expression network was constructed from 25,245 coding and non-coding genes through WGCNA approach. We set soft-thresholding power five for satisfying scale-free topology of network, in which the corresponding R2 was 0.81 (Figure 1C). And we detected twenty-six modules in the network, as shown in a cluster dendrogram (Supplementary Material S1). The members in each module were listed in Supplementary Material S2. Apart from the grey module consisted of many un-classified members, orange module contained the minimum 33 genes, while the maximum 10,821 genes were included in turquoise module.
Next, we quantified module-trait associations (Figure 2), in which the blue and darkred modules exhibited the most significant associations with sepsis. The corresponding correlation coefficients of blue and darkred modules were 0.88 (p = 2 × 10–78) and −0.77 (p = 2 × 10–48), respectively. In addition, GS and MM analysis demonstrated that genes highly significantly associated with sepsis were also the most crucial factors of modules associated with sepsis (Supplementary Material S3).
FIGURE 2. Identification of modules associated with the clinical traits of sepsis. Heatmap of the correlation between the module eigengenes and clinical traits of sepsis. All genes were clustered into twenty-six modules, of which each was labeled with one color.
Then, Gene Ontology (GO) and Kyoto Encyclopedia of Genes and Genomes (KEGG) enrichment analysis of these two modules were performed. As shown in Figure 3A, genes in the blue module were significantly enriched in ncRNA metabolic process, Herpes simplex virus 1 infection, and Th17 cell differentiation. Notably, as a less well studied subset of CD4+ Th cells, reduced Th17 cell responses have been observed in sepsis on account of low expression levels of involved transcription factors, bringing about increased susceptibility of patients to secondary fungal infections (Monneret et al., 2011; Hotchkiss et al., 2013). Our study provided additional evidence on the pivotal roles of Th17 cell function in sepsis. As shown in Figure 3B, genes in the darkred module were enriched in neutrophil activation involved in immune response and neutrophil degranulation. Consistent with previous studies, abnormal behaviors of neutrophil including delayed apoptosis have been witnessed during the early stage of sepsis (Drifte et al., 2013). Meanwhile, the most severely reduced neutrophil function has been investigated in patients with the highest risk of acquiring nosocomial infections (Nedeva, 2021), bestowing the importance of neutrophil responses on sepsis occurrence and progression.
FIGURE 3. Functional enrichment analysis of genes in the blue and darkred modules. (A) Left panel: GO analysis showed top ten enriched biological processes in blue module. Right panel: KEGG analysis showed top ten enriched pathways in blue module. (B) Left panel: GO analysis showed top ten enriched biological processes in darkred module. Right panel: KEGG analysis showed top ten enriched pathways in darkred module.
We further chose genes in the blue and darkred modules to estimate differential correlations, which were grouped based on expression patterns in each subtype (sepsis or healthy) under the cluster. molecule function of DiffCorr package. We utilized (1-correlation coefficient) as a distance measure based on cutree function. Two functions named get. eigen.molecule and get. eigen.molecule.graph were applied for module networks visualization (Figure 4). The comp.2. cc.fdr function offered the resultant pair-wise differential correlations among blue and darkred modules.
FIGURE 4. Module networks. The blue (A) and darkred (B) module networks from GSE134347 were shown. Each node represented one module. Each edge represented module correlation.
R package DiffCorr also identified oppositely correlated pairs. For example, two genes positively correlated in sepsis tissues and negatively correlated in healthy tissues, or vice versa. These switched gene pairs were worthy noticed for their crucial roles in understanding molecular mechanisms in the progression of sepsis. Totally one hundred and seventy-four oppositely correlated gene pairs from the blue module and forty-nine from the darkred module were obtained (Supplementary Material S4), whose interaction networks were presented in Figure 5. The top ten significant differentially correlated gene pairs between sepsis and healthy tissues from blue and darkred modules were shown in Table 1.
FIGURE 5. Differential co-expressed gene networks in the blue (A) and darkred (B) modules from GSE134347. Each node represented one gene. Each edge represented correlation between two genes, in which red meant positive correlation and green negative correlation. The thicker edge represented the stronger correlation coefficient.
TABLE 1. Top ten significant differentially correlated gene pairs from blue and darkred modules between healthy and sepsis tissues.
Lastly, we focused on four hub genes acting as master regulators due to possessing most links with other genes. Zinc finger protein 366 (ZNF366), also known as DC-SCRIPT, belongs to the zinc ring finger protein family and has recently been reported to regulate dendritic cell development (Søndergaard et al., 2015; Wang et al., 2018). ZNF366 has been shown to suppress toll like receptor-mediated expression of IL-10 through modulating NF-κBp65 activation (Søndergaard et al., 2015). Decreased numbers and disabilities of dendritic cells have been widely observed in sepsis followed by immune responses alterations (Roquilly and Villadangos, 2015; Venet and Monneret, 2017; Wang et al., 2020). Additionally, sepsis-induced dendritic cell blockade has been reported to prevent mice from sepsis-induced death (Meng et al., 2017). We also observed its elevated levels in sepsis compared to healthy samples (Figure 6A). Given the great importance of ZNF366 in coordinating dendritic cell function, our study expanded its roles in sepsis progression which needed further validations.
FIGURE 6. The expression levels of ZNF366 (A), ZMYND11(B), SVIP (C) and UBE2H (D) in sepsis and healthy samples from GSE134347. These four hub genes were all differentially expressed. ***p < 0.001.
Another zinc finger protein that takes the central position in the differentially co-expressed gene network is MYND-type containing 11 (ZMYND11), which is the negative regulator of NF-κB signaling and vastly impacts the replication of various viruses including Hendra virus and Epstein-Barr virus (Ikeda et al., 2010; Stewart et al., 2013). As previously described, numerous patients with sepsis witnessed the activation of NF-κB pathway initiated by pathogen-associated molecular pattern or danger-associated molecular pattern, emphasizing its great contributions during sepsis (Hayden et al., 2006; Chen et al., 2019; Wang et al., 2019). In line with it, significant lower levels of ZMYND11were detected in sepsis than healthy samples (Figure 6B). Also, impaired NF-kB activation has been correlated with sepsis-induced acute lung injury (Chen et al., 2021). Thus, it could be inferred that ZMYND11 participated in sepsis progression through NF-κB signaling and further mechanisms needed to be explored.
Small p97/VCP-interacting protein (SVIP), localized to the ER membrane by myristoylation, is highly expressed in central nervous system and related to autophagy modulation (Wu et al., 2013; Jia et al., 2019). Further mechanisms have demonstrated that overexpression of SVIP protected hepatocytes from the toxicity of CCL4 through enhancing LC3 lipidation and activating autophagy (Jia et al., 2019). Prior studies have also found its essential roles in lysosomal dynamic stability and autophagosomal-lysosomal fusion (Johnson et al., 2021). Autophagy dysregulation has been observed in organ injury induced by sepsis, in which autophagy exerted vital effects on programmed cell death pathway and inflammation (Lo et al., 2013; Qiu et al., 2018; Zhao et al., 2019). Meanwhile, animal experiments have endowed autophagy with protective roles in septic brain injury (Su et al., 2015). Also, higher levels of SVIP were observed in sepsis compared to healthy samples (Figure 6C). Based on the above evidence, our study firstly illustrated the underlying roles of SVIP in sepsis through autophagy-related pathways.
Firstly identified in yeast and human placenta, ubiquitin conjugating enzyme E2 H (UBE2H) belongs to the structurally and functionally conserved family of E2s and is involved in ubiquitination and proteasome-mediated protein degradation and regulated by TNF-α signaling (Kaiser et al., 1994; Li et al., 2003; Clague et al., 2015). Increasing evidence has indicated the potential roles of UBE2H in human brain diseases such as amyotrophic lateral sclerosis, Alzheimer’s disease and autistic disorder (Martin et al., 2009; Sokolowski et al., 2018; Lim and Joo, 2020). Nevertheless, there is scant report on the links between UBE2H and sepsis. Previous data has shown that body protein loss during sepsis was caused by upregulation of ubiquitin genes and ubiquitin-proteasome pathway (Hasselgren and Fischer, 1997). Moreover, as a hypoxia-mediated gene, UBE2H may be speculated to participate in tissue hypoxia in septic shock (Rello et al., 2017). Here, we also found elevated levels of UBE2H in sepsis compared to healthy samples (Figure 6D). Therefore, our study firstly revealed the function of UBE2H in sepsis, offering experimental clues for further investigations.
As the preliminary steps toward genetic regulatory networks, gene correlation approaches offered clues about uncovering function of mysterious genes. In this study, WGCNA and DiffCorr were employed to find out novel hub genes including ZNF366, ZMYND11, SVIP and UBE2H, and we proposed for the first time their causative factors during sepsis progression. Although biological analysis proved their vital roles in understanding pathogenesis of sepsis, these genes were not confirmed experimentally. Next we plan to integrate more datasets and conduct functional experiments including loss-of-function to underline mechanisms explaining their ability to trigger abnormal host response to infection.
The datasets presented in this study can be found in online repositories. The names of the repository/repositories and accession number(s) can be found in the article/Supplementary Material.
YZ and QF conceived the study. LS conducted the database search and analysis. YT conducted the network construction. LS and QF wrote the manuscript. All authors contributed to interpretation of the results, read, and approved the final version of the manuscript.
The present study was supported by the National Natural Science Foundation of China (81873874).
The authors declare that the research was conducted in the absence of any commercial or financial relationships that could be construed as a potential conflict of interest.
All claims expressed in this article are solely those of the authors and do not necessarily represent those of their affiliated organizations, or those of the publisher, the editors, and the reviewers. Any product that may be evaluated in this article, or claim that may be made by its manufacturer, is not guaranteed or endorsed by the publisher.
The Supplementary Material for this article can be found online at: https://www.frontiersin.org/articles/10.3389/fgene.2022.876514/full#supplementary-material
Abe, T., Yamakawa, K., Ogura, H., Kushimoto, S., Saitoh, D., Fujishima, S., et al. (2020). Epidemiology of Sepsis and Septic Shock in Intensive Care Units between Sepsis-2 and Sepsis-3 Populations: Sepsis Prognostication in Intensive Care Unit and Emergency Room (SPICE-ICU). j intensive care 8 (1). doi:10.1186/S40560-020-00465-0
Balamuth, F., Alpern, E. R., Kan, M., Shumyatcher, M., Hayes, K., Lautenbach, E., et al. (2020). Gene Expression Profiles in Children with Suspected Sepsis. Ann. Emerg. Med. 75 (6), 744–754. doi:10.1016/j.annemergmed.2019.09.020
Chen, X., Sun, Z., Zhang, H., and Wang, L. (2021). Correlation of Impaired NF-kB Activation in Sepsis-Induced Acute Lung Injury (ALI) in Diabetic Rats. J. Healthc. Eng. 2021, 1–6. doi:10.1155/2021/5657284
Chen, Y., Liu, W., Xu, H., Liu, J., Deng, Y., Cheng, H., et al. (2019). Gga-miR-19b-3p Inhibits Newcastle Disease Virus Replication by Suppressing Inflammatory Response via Targeting RNF11 and ZMYND11. Front. Microbiol. 10, 2006. doi:10.3389/fmicb.2019.02006
Clague, M. J., Heride, C., and Urbé, S. (2015). The Demographics of the Ubiquitin System. Trends Cel Biol. 25 (7), 417–426. doi:10.1016/j.tcb.2015.03.002
Doncheva, N. T., Morris, J. H., Gorodkin, J., and Jensen, L. J. (2019). Cytoscape StringApp: Network Analysis and Visualization of Proteomics Data. J. Proteome Res. 18 (2), 623–632. doi:10.1021/acs.jproteome.8b00702
Drifte, G., Dunn-Siegrist, I., Tissières, P., and Pugin, J. (2013). Innate Immune Functions of Immature Neutrophils in Patients with Sepsis and Severe Systemic Inflammatory Response Syndrome*. Crit. Care Med. 41 (3), 820–832. doi:10.1097/CCM.0b013e318274647d
Fang, Q., Wang, Q., Zhou, Z., and Xie, A. (2021). Consensus Analysis via Weighted Gene Co-expression Network Analysis (WGCNA) Reveals Genes Participating in Early Phase of Acute Respiratory Distress Syndrome (ARDS) Induced by Sepsis. Bioengineered 12 (1), 1161–1172. doi:10.1080/21655979.2021.1909961
Fleischmann, C., Scherag, A., Adhikari, N. K. J., Hartog, C. S., Tsaganos, T., Schlattmann, P., et al. (2016). Assessment of Global Incidence and Mortality of Hospital-Treated Sepsis. Current Estimates and Limitations. Am. J. Respir. Crit. Care Med. 193 (3), 259–272. doi:10.1164/rccm.201504-0781OC
Fukushima, A. (2013). DiffCorr: An R Package to Analyze and Visualize Differential Correlations in Biological Networks. Gene 518 (1), 209–214. doi:10.1016/j.gene.2012.11.028
Ghazalpour, A., Doss, S., Zhang, B., Wang, S., Plaisier, C., Castellanos, R., et al. (2006). Integrating Genetic and Network Analysis to Characterize Genes Related to Mouse Weight. Plos Genet. 2 (8), e130–1192. doi:10.1371/journal.pgen.0020130
Hasselgren, P.-O., and Fischer, J. E. (1997). The Ubiquitin-Proteasome Pathway. Ann. Surg. 225 (3), 307–316. doi:10.1097/00000658-199703000-00011
Hayden, M. S., West, A. P., and Ghosh, S. (2006). NF-κB and the Immune Response. Oncogene 25 (51), 6758–6780. doi:10.1038/sj.onc.1209943
Hochberg, Y., and Benjamini, Y. (1990). More Powerful Procedures for Multiple Significance Testing. Statist. Med. 9 (7), 811–818. doi:10.1002/sim.4780090710
Hotchkiss, R. S., Monneret, G., and Payen, D. (2013). Sepsis-induced Immunosuppression: from Cellular Dysfunctions to Immunotherapy. Nat. Rev. Immunol. 13 (12), 862–874. doi:10.1038/nri3552
Ideker, T., and Krogan, N. J. (2012). Differential Network Biology. Mol. Syst. Biol. 8, 565. doi:10.1038/Msb.2011.99
Ikeda, O., Sekine, Y., Mizushima, A., Oritani, K., Yasui, T., Fujimuro, M., et al. (2009). BS69 Negatively Regulates the Canonical NF-Κb Activation Induced by Epstein-Barr Virus-Derived LMP1. Faseb J. 583, 1567–1574. doi:10.1016/j.febslet.2009.04.022
Jia, D., Wang, Y. Y., Wang, P., Huang, Y., Liang, D. Y., Wang, D., et al. (2019). SVIP Alleviates CCl4-Induced Liver Fibrosis via Activating Autophagy and Protecting Hepatocytes. Cell Death Dis 10 (2). doi:10.1038/s41419-019-1311-0
Johnson, A. E., Orr, B. O., Fetter, R. D., Moughamian, A. J., Primeaux, L. A., Geier, E. G., et al. (2021). SVIP Is a Molecular Determinant of Lysosomal Dynamic Stability, Neurodegeneration and Lifespan. Nat. Commun. 12 (1). doi:10.1038/s41467-020-20796-8
Kaiser, P., Seufert, W., Höfferer, L., Kofler, B., Sachsenmaier, C., Herzog, H., et al. (1994). A Human Ubiquitin-Conjugating Enzyme Homologous to Yeast UBC8. J. Biol. Chem. 269 (12), 8797–8802. doi:10.1016/s0021-9258(17)37039-4
Langfelder, P., and Horvath, S. (2008). WGCNA: an R Package for Weighted Correlation Network Analysis. BMC Bioinformatics 9, 559. doi:10.1186/1471-2105-9-559
Langfelder, P., Zhang, B., and Horvath, S. (2008). Defining Clusters from a Hierarchical Cluster Tree: the Dynamic Tree Cut Package for R. Bioinformatics 24 (5), 719–720. doi:10.1093/bioinformatics/btm563
Li, A., and Horvath, S. (2007). Network Neighborhood Analysis with the Multi-Node Topological Overlap Measure. Bioinformatics 23 (2), 222–231. doi:10.1093/bioinformatics/btl581
Li, Y.-P., Lecker, S. H., Chen, Y., Waddell, I. D., Goldberg, A. L., and Reid, M. B. (2003). TNF‐α Increases Ubiquitin‐conjugating Activity in Skeletal Muscle by Up‐regulating UbcH2/E220k. FASEB j. 17 (9), 1048–1057. doi:10.1096/fj.02-0759com
Li, Y., Jin, S., Lei, L., Pan, Z., and Zou, X. (2015). Deciphering Deterioration Mechanisms of Complex Diseases Based on the Construction of Dynamic Networks and Systems Analysis. Sci. Rep. 5. doi:10.1038/Srep09283
Lim, K.-H., and Joo, J.-Y. (2020). Predictive Potential of Circulating Ube2h mRNA as an E2 Ubiquitin-Conjugating Enzyme for Diagnosis or Treatment of Alzheimer's Disease. Ijms 21 (9), 3398. doi:10.3390/ijms21093398
Lo, S., Yuan, S.-S. F., Hsu, C., Cheng, Y.-J., Chang, Y.-F., Hsueh, H.-W., et al. (2013). Lc3 Over-expression Improves Survival and Attenuates Lung Injury through Increasing Autophagosomal Clearance in Septic Mice. Ann. Surg. 257 (2), 352–363. doi:10.1097/SLA.0b013e318269d0e2
Markwart, R., Saito, H., Harder, T., Tomczyk, S., Cassini, A., Fleischmann-Struzek, C., et al. (2020). Epidemiology and burden of Sepsis Acquired in Hospitals and Intensive Care Units: a Systematic Review and Meta-Analysis. Intensive Care Med. 46 (8), 1536–1551. doi:10.1007/s00134-020-06106-2
Martin, I., Vourc'h, P., Mahé, M., Thépault, R.-A., Antar, C., Védrine, S., et al. (2009). Association Study of the Ubiquitin Conjugating Enzyme Gene UBE2H in Sporadic ALS. Amyotroph. Lateral Scler. 10 (5-6), 432–435. doi:10.3109/17482960802444972
Meng, Y., Zhao, Z., Zhu, W., Yang, T., Deng, X., and Bao, R. (2017). CD155 Blockade Improves Survival in Experimental Sepsis by Reversing Dendritic Cell Dysfunction. Biochem. Biophysical Res. Commun. 490 (2), 283–289. doi:10.1016/j.bbrc.2017.06.037
Monneret, G., Venet, F., Kullberg, B.-J., and Netea, M. G. (2011). ICU-acquired Immunosuppression and the Risk for Secondary Fungal Infections. Med. Mycol. 49 (S1), S17–S23. doi:10.3109/13693786.2010.509744
Nedeva, C. (2021). Inflammation and Cell Death of the Innate and Adaptive Immune System during Sepsis. Biomolecules 11 (7), 1011. doi:10.3390/Biom11071011
Póvoa, P., Coelho, L., Almeida, E., Fernandes, A., Mealha, R., Moreira, P., et al. (2005). C-reactive Protein as a Marker of Infection in Critically Ill Patients. Clin. Microbiol. Infect. 11 (2), 101–108. doi:10.1111/j.1469-0691.2004.01044.x
Qiu, P., Liu, Y., and Zhang, J. (2018). Review: the Role and Mechanisms of Macrophage Autophagy in Sepsis. Inflammation 42 (1), 6–19. doi:10.1007/s10753-018-0890-8
Rello, J., Valenzuela-Sánchez, F., Ruiz-Rodriguez, M., and Moyano, S. (2017). Sepsis: A Review of Advances in Management. Adv. Ther. 34 (11), 2393–2411. doi:10.1007/s12325-017-0622-8
Rhee, C., Murphy, M. V., Li, L., Platt, R., Klompas, M., and Prevention, C. D. C. (2015). Lactate Testing in Suspected Sepsis. Crit. Care Med. 43 (8), 1669–1676. doi:10.1097/Ccm.0000000000001087
Riedel, S., Melendez, J. H., An, A. T., Rosenbaum, J. E., and Zenilman, J. M. (2011). Procalcitonin as a Marker for the Detection of Bacteremia and Sepsis in the Emergency Department. Am. J. Clin. Pathol. 135 (2), 182–189. doi:10.1309/Ajcp1mfyinqlecv2
Roquilly, A., and Villadangos, J. A. (2015). The Role of Dendritic Cell Alterations in Susceptibility to Hospital-Acquired Infections during Critical-Illness Related Immunosuppression. Mol. Immunol. 68 (2), 120–123. doi:10.1016/j.molimm.2015.06.030
Saukkonen, K., Lakkisto, P., Pettila, V., Varpula, M., Karlsson, S., Ruokonen, E., et al. (2008). Cell-free Plasma DNA as a Predictor of Outcome in Severe Sepsis and Septic Shock. Clin. Chem. 54 (6), 1000–1007. doi:10.1373/clinchem.2007.101030
Scicluna, B. P., Uhel, F., Van Vught, L. A., Wiewel, M. A., Hoogendijk, A. J., Baessman, I., et al. (2020). The Leukocyte Non-coding RNA Landscape in Critically Ill Patients with Sepsis. eLife 9. doi:10.7554/eLife.58597
Sokolowski, M., Wasserman, J., and Wasserman, D. (2018). Gene-level Associations in Suicide Attempter Families Show Overrepresentation of Synaptic Genes and Genes Differentially Expressed in Brain Development. Am. J. Med. Genet. 177 (8), 774–784. doi:10.1002/ajmg.b.32694
Søndergaard, J. N., Poghosyan, S., Hontelez, S., Louche, P., Looman, M. W. G., Ansems, M., et al. (2015). DC-SCRIPT Regulates IL-10 Production in Human Dendritic Cells by Modulating NF-κBp65 Activation. J.I. 195 (4), 1498–1505. doi:10.4049/jimmunol.1402924
Stewart, C. R., Marsh, G. A., Jenkins, K. A., Gantier, M. P., Tizard, M. L., Middleton, D., et al. (2013). Promotion of Hendra Virus Replication by MicroRNA 146a. J. Virol. 87 (7), 3782–3791. doi:10.1128/Jvi.01342-12
Su, Y., Qu, Y., Zhao, F., Li, H., Mu, D., and Li, X. (2015). Regulation of Autophagy by the Nuclear Factor κB Signaling Pathway in the hippocampus of Rats with Sepsis. J. Neuroinflammation 12. doi:10.1186/S12974-015-0336-2
Venet, F., and Monneret, G. (2017). Advances in the Understanding and Treatment of Sepsis-Induced Immunosuppression. Nat. Rev. Nephrol. 14 (2), 121–137. doi:10.1038/nrneph.2017.165
Wang, G., Li, X., Zhang, L., Elgaili Abdalla, A., Teng, T., and Li, Y. (2020). Crosstalk between Dendritic Cells and Immune Modulatory Agents against Sepsis. Genes 11 (3), 323. doi:10.3390/genes11030323
Wang, J., Wang, T., Benedicenti, O., Collins, C., Wang, K., Secombes, C. J., et al. (2018). Characterisation of ZBTB46 and DC-SCRIPT/ZNF366 in Rainbow trout, Transcription Factors Potentially Involved in Dendritic Cell Maturation and Activation in Fish. Developmental Comp. Immunol. 80, 2–14. doi:10.1016/j.dci.2016.11.007
Wang, Y.-M., Ji, R., Chen, W.-W., Huang, S.-W., Zheng, Y.-J., Yang, Z.-T., et al. (2019). Paclitaxel Alleviated Sepsis-Induced Acute Lung Injury by Activating MUC1 and Suppressing TLR-4/nf-Κb Pathway. Dddt 13, 3391–3404. doi:10.2147/dddt.s222296<
Wu, J., Peng, D., Voehler, M., Sanders, C. R., and Li, J. (2013). Structure and Expression of a Novel Compact Myelin Protein - Small VCP-Interacting Protein (SVIP). Biochem. Biophysical Res. Commun. 440 (1), 173–178. doi:10.1016/j.bbrc.2013.09.056
Yang, L., Li, Y., Wei, Z., and Chang, X. (2018). Coexpression Network Analysis Identifies Transcriptional Modules Associated with Genomic Alterations in Neuroblastoma. Biochim. Biophys. Acta (Bba) - Mol. Basis Dis. 1864 (6 Pt B), 2341–2348. doi:10.1016/j.bbadis.2017.12.020
Yu, G., Wang, L.-G., Han, Y., and He, Q.-Y. (2012). clusterProfiler: an R Package for Comparing Biological Themes Among Gene Clusters. OMICS: A J. Integr. Biol. 16 (5), 284–287. doi:10.1089/omi.2011.0118
Yu, X., Qu, C., Ke, L., Tong, Z., and Li, W. (2021). Step-by-Step Construction of Gene Co-expression Network Analysis for Identifying Novel Biomarkers of Sepsis Occurrence and Progression. Ijgm 14, 6047–6057. doi:10.2147/IJGM.S328076
Zhai, J., Qi, A., Zhang, Y., Jiao, L., Liu, Y., and Shou, S. (2020). Bioinformatics Analysis for Multiple Gene Expression Profiles in Sepsis. Med. Sci. Monit. 26. doi:10.12659/msm.920818
Zhang, B., and Horvath, S. (2005). A General Framework for Weighted Gene Co-expression Network Analysis. Stat. Appl. Genet. Mol. Biol. 4. 1. Article17. doi:10.2202/1544-6115.1128
Zhang, Z., Chen, L., Xu, P., Xing, L., Hong, Y., and Chen, P. (2020a). Gene Correlation Network Analysis to Identify Regulatory Factors in Sepsis. J. Transl Med. 18 (1), 381. doi:10.1186/s12967-020-02561-z
Zhang, Z., Pan, Q., Ge, H., Xing, L., Hong, Y., and Chen, P. (2020b). Deep Learning-Based Clustering Robustly Identified Two Classes of Sepsis with Both Prognostic and Predictive Values. eBioMedicine 62, 103081. doi:10.1016/j.ebiom.2020.103081
Zhao, H., Chen, H., Xiaoyin, M., Yang, G., Hu, Y., Xie, K., et al. (2019). Autophagy Activation Improves Lung Injury and Inflammation in Sepsis. Inflammation 42 (2), 426–439. doi:10.1007/s10753-018-00952-5
Keywords: WGCNA, differential correlation, regulatory network, biological analysis, sepsis
Citation: Sheng L, Tong Y, Zhang Y and Feng Q (2022) Identification of Hub Genes With Differential Correlations in Sepsis. Front. Genet. 13:876514. doi: 10.3389/fgene.2022.876514
Received: 15 February 2022; Accepted: 09 March 2022;
Published: 24 March 2022.
Edited by:
Tao Huang, Shanghai Institute of Nutrition and Health, (CAS), ChinaReviewed by:
Meijian Guan, Janssen Pharmaceuticals, Inc., United StatesCopyright © 2022 Sheng, Tong, Zhang and Feng. This is an open-access article distributed under the terms of the Creative Commons Attribution License (CC BY). The use, distribution or reproduction in other forums is permitted, provided the original author(s) and the copyright owner(s) are credited and that the original publication in this journal is cited, in accordance with accepted academic practice. No use, distribution or reproduction is permitted which does not comply with these terms.
*Correspondence: Qiming Feng, ZmVuZ3FpbWluZzA0QDEyNi5jb20=; Yi Zhang, WXpoYW5nX21lZEBmdWRhbi5lZHUuY24=
†These authors have contributed equally to this work
Disclaimer: All claims expressed in this article are solely those of the authors and do not necessarily represent those of their affiliated organizations, or those of the publisher, the editors and the reviewers. Any product that may be evaluated in this article or claim that may be made by its manufacturer is not guaranteed or endorsed by the publisher.
Research integrity at Frontiers
Learn more about the work of our research integrity team to safeguard the quality of each article we publish.