- 1Institute of Food Crops, Jiangsu Academy of Agricultural Sciences, Nanjing, China
- 2Jiangsu High Quality Rice R&D Center, Nanjing Branch of China National Center for Rice Improvement, Nanjing, China
- 3Excellence and Innovation Center, Jiangsu Academy of Agricultural Sciences, Nanjing, China
- 4Key Laboratory of Jiangsu Province for Agrobiology, Nanjing, China
Panicle architecture is an important agronomic trait in rice that affects rice yields and quality. The GRAIN SIZE 3 (GS3) locus has been identified as a major quantitative trait locus (QTL) affecting grain length and weight. The current understanding of the function of the GS3 gene, especially concerning the regulatory mechanism of panicle development, is still in its infancy. In this study, we generated GS3 near-isogenic lines (NILs) by successive crossing and backcrossing of TD70 (large grain) with Kasalath (small grain), using Kasalath as the recurrent parent. To identify potential transcription dynamic changes in rice panicle formation and grain shape, we deeply analyzed transcriptional profiles for the NILs (NIL-GS3 and NIL-gs3) at three different panicle developmental stages (S, M, and L). A total of 887, 1,768, and 1,478 differentially expressed genes (DEGs) were identified at stages S, M, and L, respectively. We also found 542 differential expressed long non-coding RNAs (lncRNAs). Co-expression analysis further revealed significant clusters associated with different development periods in NIL-gs3 lines. Gene Ontology and KEGG enrichment analysis revealed G-protein signaling and hormones pathway were successively activated at the M and L stages of NIL-gs3, which indicated activation of the G-protein signaling pathway might trigger the down-streaming hormone signaling transduction. we found that other hormones such ABA, Auxin, CK were significantly enriched in the L stage in the NIL-gs3. We highlighted the synergistic interplay of G-protein and multiple hormones signaling pathways and their essential roles in regulating rice panicle formation and the grain shape. Our study provides an invaluable resource for further molecular mechanistic studies that affect rice grain size and provide new insight for directed selection by marker-assisted backcross breeding.
Introduction
Rice is one of the most important food crops globally, feeding more than half of the world’s population. Grain weight is determined by grain size and grain filling degree, whereas the yield is based on grain weight, panicle quantity, and quantity per panicle. It is important to improve grain size in rice breeding, as it is closely related to yield, and quality. Thus, investigating the gene expression regulation associated with grain development can potentially provide an avenue for improving crop yield and quality.
Many genetic studies examining grain-shape traits have been carried out in the past few decades, and many grain shape-related genes and quantitative trait loci (QTL) have been cloned (Huang et al., 2013; Wang Y et al., 2019). Among them, genes associated with grain length have been identified, including GS3(Grain Size 3), qGL3(Grain Length 3), GL7/GW7(Grain Length/Width 7), GL4(Grain Length 4), TGW3/GL3.3(Grain Length 3.3), GS9(Grain Size 9), and GLA(Grain Length and Awn) (Mao et al., 2010; Zhang et al., 2012; Wang S et al., 2015; Wang Y et al., 2015; Wu et al., 2017; Xia et al., 2018; Ying et al., 2018; Zhao et al., 2018; Zhang et al., 2019). The GS3 gene is located in the pericentromeric region of rice chromosome 3 and encodes a predicted membrane protein that regulates grain size by controlling cell proliferation. Furthermore, this QTL has been shown to control grain size and play a role as a negative regulator concerning grain and organ size (Fan et al., 2006; Mao et al., 2010).
During rice grain development, multiple grain shape-related genes, and their interactions affect the developmental outcome. Another gene, GW8, which belongs to the SBP transcription factor family, has been shown to promote cell proliferation and grain filling when overexpressed and contributes to grain widening and increased yields. Furthermore, GW8 can bind to the GL7/GW7/SLG promoter to inhibit its transcription and regulate cell proliferation in spikelet glumes (Wang et al., 2012; Wang S et al., 2015). Additionally, both GLW7 and GW8 positively regulate the cell size of the grain hull to increase the rice grain length and yield. Small and round seed 5 (SRS5) encodes an α-tubulin, with a one amino acid change (p.Arg308Leu) that reduces the rice seed length by hindering cell elongation. Furthermore, although GLW7 can interact with SRS5 to regulate grain size, the underlying mechanism remains unclear. However, GLW7 has been shown to bind the DEP1 promoter, and significantly affect panicle length and primary branch number. Additionally, the GLW7 and GS3 genes have been shown to act independently (Si et al., 2016). Thus, a complete characterization of these genes can aid in improved molecular breeding.
GW2 and GW5 positively regulate the expression of GS3, and GW5 can be down-regulated by repressing GW2 transcription. Moreover, GS3 can alleviate the effect of GW5 on grain length, and GW5 also can alleviate the effect of GS3 on grain width (Yan et al., 2011). Furthermore, GS3 and qGL3 are negative regulators of grain length and weight, with a GS3/qGL3 loss-of-function leading to a long grain length. In near-isogenic lines (NILs), GS3, and qGL3 have exhibited a cumulative effect on rice grain length regulation, possibly because of a common receptor protein kinase signal pathway (Gao et al., 2015).
In plants, heterotrimeric G-proteins are conserved signaling molecules that play essential roles in multiple signaling pathways. The 3 G protein subunits (α, β, and γ) have different functions in rice. An α subunit (RGA1/D1) loss-of-function leads to a dwarf phenotype and reduced grain size and lowers BR sensitivity, while a decreased β subunit (RGB1) expression also results in a short-grain size and dwarf phenotype. The γ subunit comprises five homologous genes (RGG1, RGG2, qPE9-1/DEP1, GGC2, and GS3) with varying functions. RGG2 is a negative regulator of plant growth and organ size and interacts with RGB1 in the gibberellin (GA) pathway. Furthermore, DEP1 and GGC2 interact with the β subunit to positively regulate grain length. Although GS3 itself does not affect grain length, it can inhibit the effects of DEP1 and GGC2 by competitively binding the β subunit. Therefore, a GS3 deletion results in a long-grain phenotype. Additionally, RGG1 enhances salt tolerance in rice by improving reactive oxygen species (ROS) detoxification (Sun et al., 2018; Miao et al., 2019; Wang Y et al., 2019; Xu et al., 2019). OsMADS1 acts as a key downstream effector of the βγ G protein dimer, with the γ subunits (GS3, DEP1) directly interacting with MADS1 to increase its transcriptional activity thereby affecting downstream target genes and affecting grain morphology. Moreover, the pyramiding of these alleles, OsMADS1lgy3, dep1-1, and gs3, can improve rice yield and quality (Liu et al., 2018). In the Nipponbare genome, GL3.3 (GSK5) encodes a GSK3/SHAGGY-like kinase and negatively regulates grain length. Furthermore, GL3.3 is constitutively expressed in different tissues and organs and exhibits epistatic interactions with GS3 (Xia et al., 2018).
RNA sequencing (RNA-Seq) enables genome-wide transcriptomic analysis and has been applied to examine rice panicle development, fertility, heterosis, and salinity stress (Zhang et al., 2014; Ke et al., 2016; Guo et al., 2017; Zhang et al., 2018; Wang J et al., 2019). In eukaryotes, long non-coding RNAs (lncRNAs) are produced by RNA polymerases, are greater than 200 bp in length, and have no protein potential (Han et al., 2019). LncRNAs have been implicated in plant growth, reproduction, disease resistance, and stress (Lee et al., 2013; Zhang and Chen, 2013; Lv et al., 2019).
In this study, we used NILs to examine dynamic transcriptional changes at different stages with/without GS3 in rice panicle development using time-course RNA-Seq. We also examined the potential transcriptional regulatory effects of directed selection of the GS3 gene by marker-assisted backcross breeding (MABB).
Results
Variations in gs3 and Grain Shape Phenotype in NILs
Previous studies have confirmed that GS3 is the major QTL that controls grain length and weight in rice, with an SNP (C→A) mutation in the second exon leading to early termination of translation and a subsequent long-grain phenotype (Fan et al., 2006; Fan et al., 2009; Mao et al., 2010). In NIL-GS3, the ORF length of GS3 was 696 bp and encoded 231 amino acids. The domain prediction identified four functional domains: the N-terminal organ size regulator (OSR) domain, a transmembrane domain (TM), a C-terminal TNFR/NGFR family cysteine-rich structure, and a VWFC structure (Figure 1A). In NIL-gs3, however, the GS3 locus mutated from C to A at 165 bp, resulting in a premature termination codon (TGC→TGA) and a sequence with only 54 amino acids (Figure 1A). The GS3 loci in NIL-GS3 and NIL-gs3 were the same as those reported in Zhenshan 97 and Minghui 63, respectively (Mao et al., 2010).
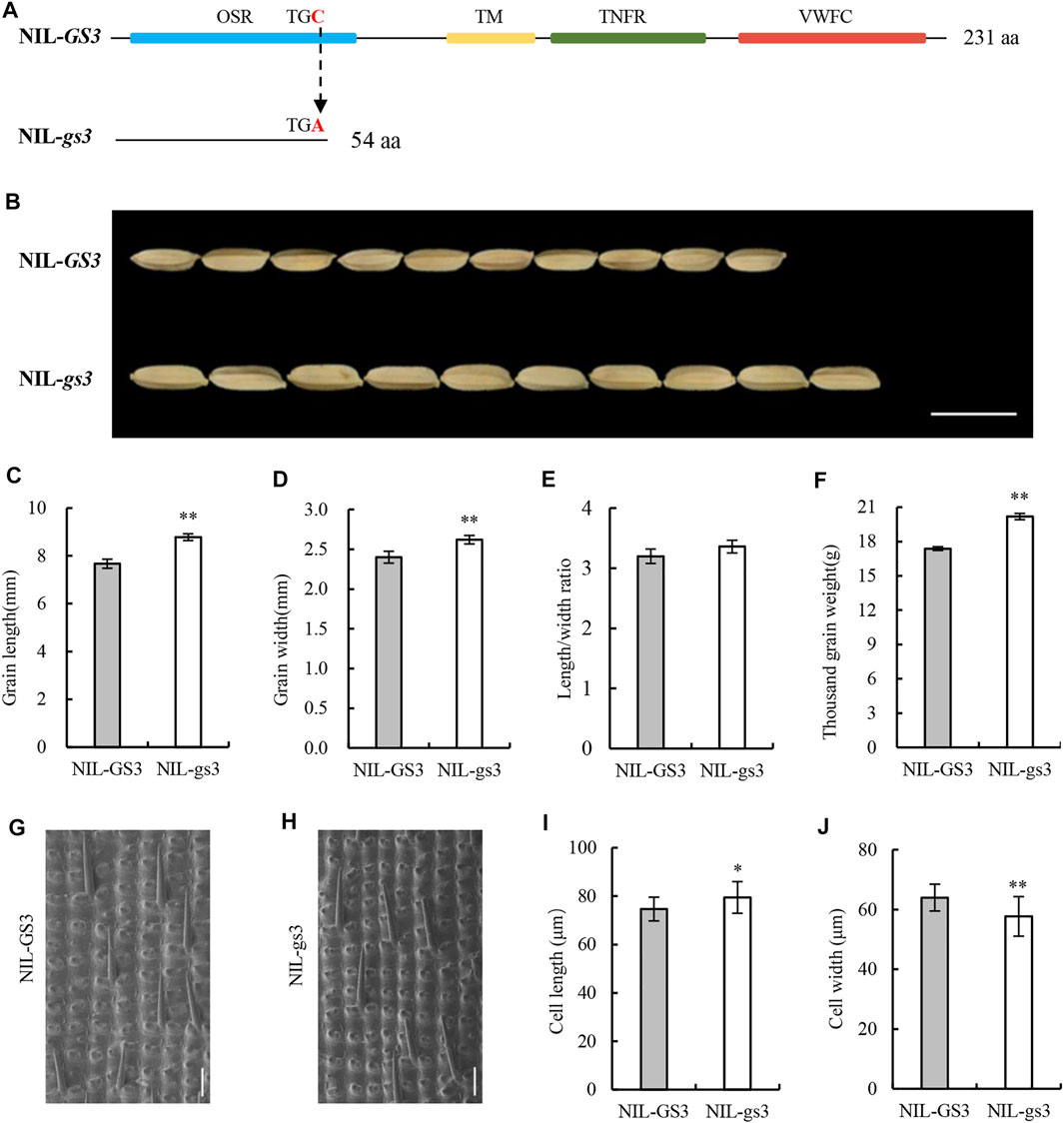
FIGURE 1. Differences in the GS3 gene structure and the grain phenotype in NIL-GS3 and NIL-gs3. (A) Structural differences in GS3 between the NILs. (B) Appearance of mature grains. Bar = 10 mm. Grain (C) length, (D) width, (E) length/width ratio, and (F) thousand-grain weight. (G, H) Imaging of epidermal cells in the middle glume of mature grains using a scanning electron microscope. Bar = 100 μm. (I, J) Cell length and width statistics. Data are displayed as a mean ± SD (n = 20). Asterisks indicate significance (Student’s t-test, *p < 0.05, **p < 0.01).
When comparing the grain length, length-to-width ratio, and thousand-grain weight, NIL-gs3 increased by 14.32, 4.69, and 16.17%, respectively, relative to NIL-GS3 (p < 0.05). Additionally, the grain width of NIL-gs3 increased by 9.17%, but we did not note any significant difference in grain thickness. Therefore, the gs3 locus contained in NIL-gs3 had a positive effect on rice grain size (Figures 1B–F; Supplementary Table S1).
To examine the cytological basis of grain size differences in the GS3 isogenic lines, we examined the morphology of the cells in the middle portion of the glumes of mature NIL-GS3 and NIL-gs3 seeds and compared them using SEM. The results showed that the glume cell length was significantly longer in NIL-gs3 than NIL-GS3 (6.50%, in the grain-length orientation), whereas the cell width decreased significantly (9.77%). Subsequently, the glume epidermal cells in NIL-gs3 appeared to be more slender, and the length-to-width ratio increased. These findings indicated that the GS3 gene regulates rice grain shape by regulating glume cell morphology (Figure 1G–J; Supplementary Table S1).
Transcriptome Assembly and Potential Coding Prediction
To comprehensively analyze the transcriptome changes in rice grain development following a GS3 mutation, we examined three representative stages (S:small panicle size, M:medium panicle size, and L:large panicle size) from the beginning of panicle to the beginning of the heading in the NILs. We obtained a total of 114.78 G base pairs, with an average of 6.38 G per sample. We then successfully aligned the reads to the reference genome IRGSP-1.0 using Hisat2. The results showed that the percentage of unique alignments to the genome was between 89.49 and 91.96%, with an average of 90.84% (Supplementary Table S2).
After RABT assembly, we obtained 85,411 transcripts from 44,668 genes, with 5,936 new transcripts identified in the intergenic regions (Supplementary Table S3; Figure 2). We then predicted coding potentials using CPC2 and PLEK and identified 18,343 transcripts (15,136 genes) as lncRNA candidates. Among them, 4,143 were located in the intergenic regions and 155 were located in introns. These two types of lncRNAs can be collectively called lincRNAs. Additionally, 1,255 lncRNA transcripts overlapped with gene exons, presumably because of alternative splicing (Supplementary Table S3). Quantitative analysis of the expression profiles showed that lncRNA expression, especially lincRNA expression, was lower than that of coding genes, consistent with previous studies (Lv et al., 2019).
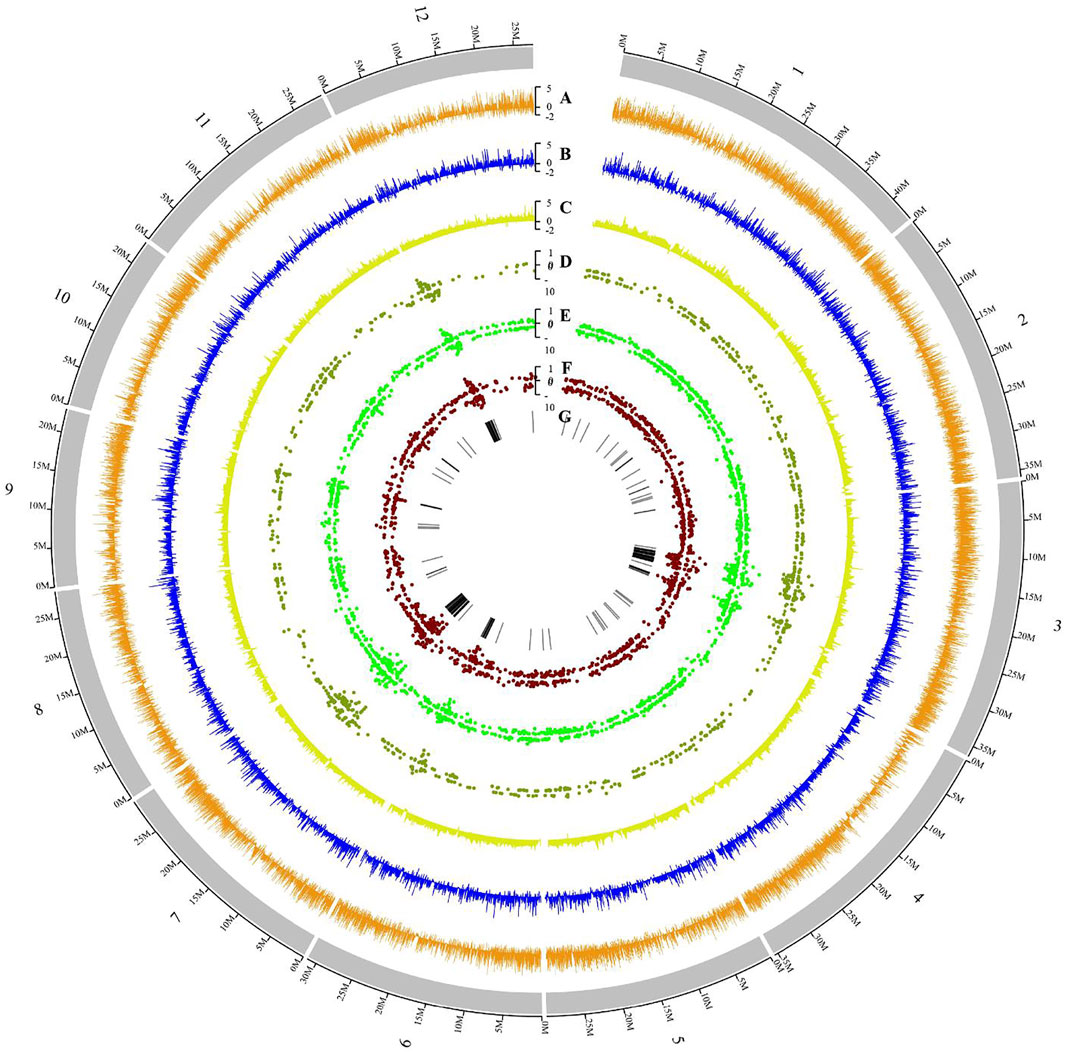
FIGURE 2. Gene expression distributions in NIL-GS3 and NIL-gs3 during panicle development. (A) Distribution of all gene expression profile; (B) LncRNA expression profiles; (C) LncRNA expression profile. The Y-axis shows log10-scaled average FPKM values. Distributions of deferentially expressed genes in NIL-GS3 and NIL-gs3 at the (D) S stage (E) M stage, and (F) L stage. The Y-axis shows the Log2 (Fold change). (G) Distribution of common DEGs within the three stages.
Identification of DEGs Between NILs
During rice growth and development, dynamic changes in gene expression occur in different tissues and organs during different developmental stages. At the booting stage, many genes associated with cell proliferation, cell expansion, transcription factors, and hormone regulation modulate inflorescence and panicle morphogenesis (Ke et al., 2016; Wang Y et al., 2019; Zha et al., 2019). When identifying DEGs at the different developmental stages in NIL-GS3, we identified 5,974 DEGs at the S stage relative to the M stage, 2,395 at the L stage relative to M, and 9,400 at the L stage relative to the S. Among these three groups of DEGs, a total of 742 genes were commonly significantly differently expressed. We also observed this phenomenon in the three stages of spikelet development in NIL-gs3 (Supplementary Table S4). These findings clearly showed the presence of gene expression stratification at different developmental stages.
To further examine expressional changes during different developmental stages, we compared differential expression between the NIL groups at each stage (Figure 3; Supplementary Table S4). During the S stage, we identified 887 DEGs (167 lncRNAs), 434 upregulated (85 lncRNAs), and 453 downregulated (82 lncRNAs) in NIL-gs3 relative to the NIL-GS3. For the M stage, we identified 1,768 DEGs (318 lncRNAs), 852 upregulated (129 lncRNAs) and 916 downregulated (189 lncRNAs); For the L stage, we identified 1,478 DEGs (280 lncRNAs), 670 upregulated (151 lncRNAs), and 808 down-regulated (129 lncRNAs). Furthermore, the number of DEGs increased with the growth of the panicle, thus suggesting that GS3 affected the expression of more genes in the mid-late stages of panicle development (Figure 3A). When comparing the young NIL panicles at the same stage, 215 genes (66 lncRNAs) were commonly differentially expressed across the three stages. Furthermore, hierarchical clustering analysis also showed a similar expression pattern between the groups (Figures 2, 3C). The number of unique differential genes in the S, M, and L stages were 442 (90 LncRNAs), 1,091 (126 LncRNAs), and 925 (120 LncRNAs), respectively (Figure 3B). To confirm the RNA-Seq findings, we randomly selected ten representatives of differentially expressed transcripts, including two lncRNAs, and eight coding genes. The qRT-PCR results showed that three of the transcripts were significantly upregulated, and five were significantly down-regulated among S, M, and L periods. (Supplementary Figure S1). All changes were consistent with expected results from the RNA-Seq data, thus indicating a high degree of reliability.
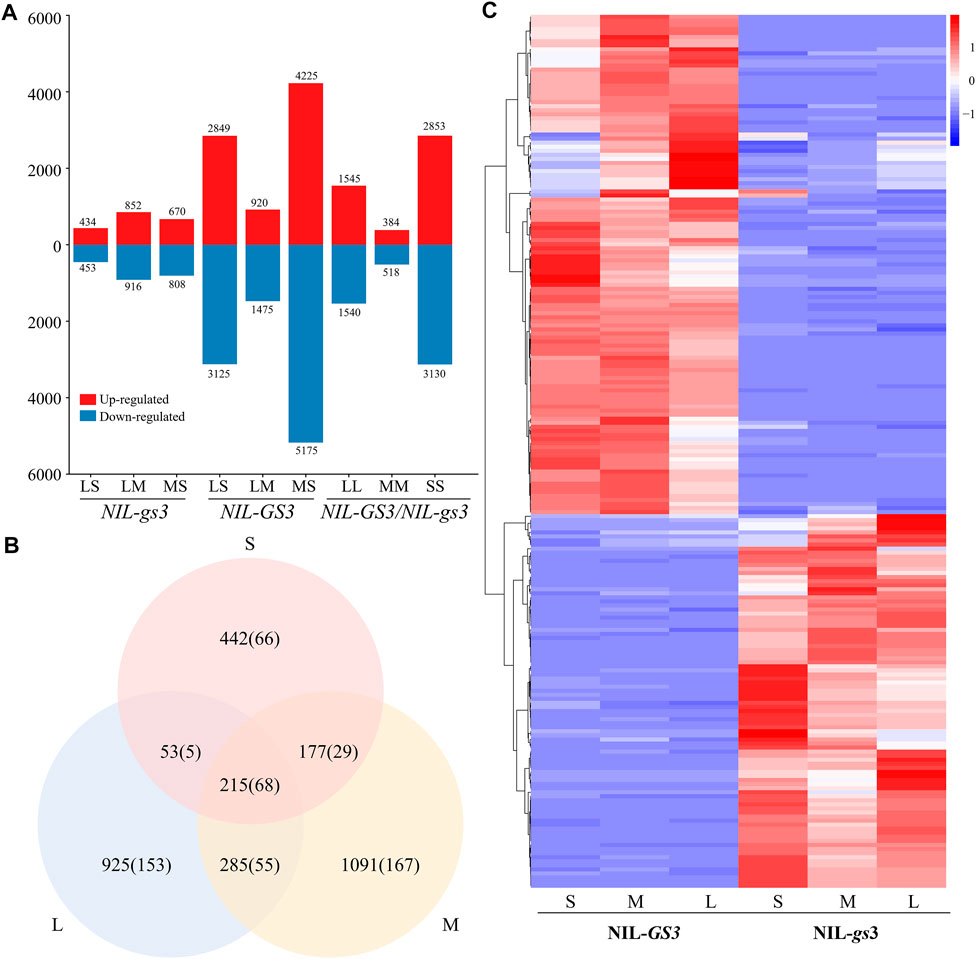
FIGURE 3. NIL-GS3 and NIL-gs3 differential expression at different stages of panicle development (A) Identification of DEGs at different developmental stages. (B) Number of differentially expressed lncRNAs at different stages. (C) Comparative analysis of DEGs at different developmental stages. The number of lncRNAs is in parentheses. (D) Expression profiles of the DEGs for all three stages. FPKM values were normalized to the Z-scores.
A Dynamic Expression Landscape During Kernel Development
The c-means fuzzy clustering algorithm was employed for clustering gene expression profiles in all developmental stages (Kumar and Futschik, 2007). The fuzzy clustering algorithm could assign a membership value for each gene expression profile of each cluster using different fuzziness values. As a result, nine distinct clusters of temporal patterns representing NILs and development stages were generated (Figure 4A; Supplementary Table S5). Among them, cluster 7, cluster 4, and cluster 6 were identified, which represented different development stages (S, M, and L) and significantly upregulated in the corresponding stage of NIL-gs3, whereas cluster 8, cluster 5, and cluster 1 are upregulated and showed stage-specific in three stages of NIL-GS3.
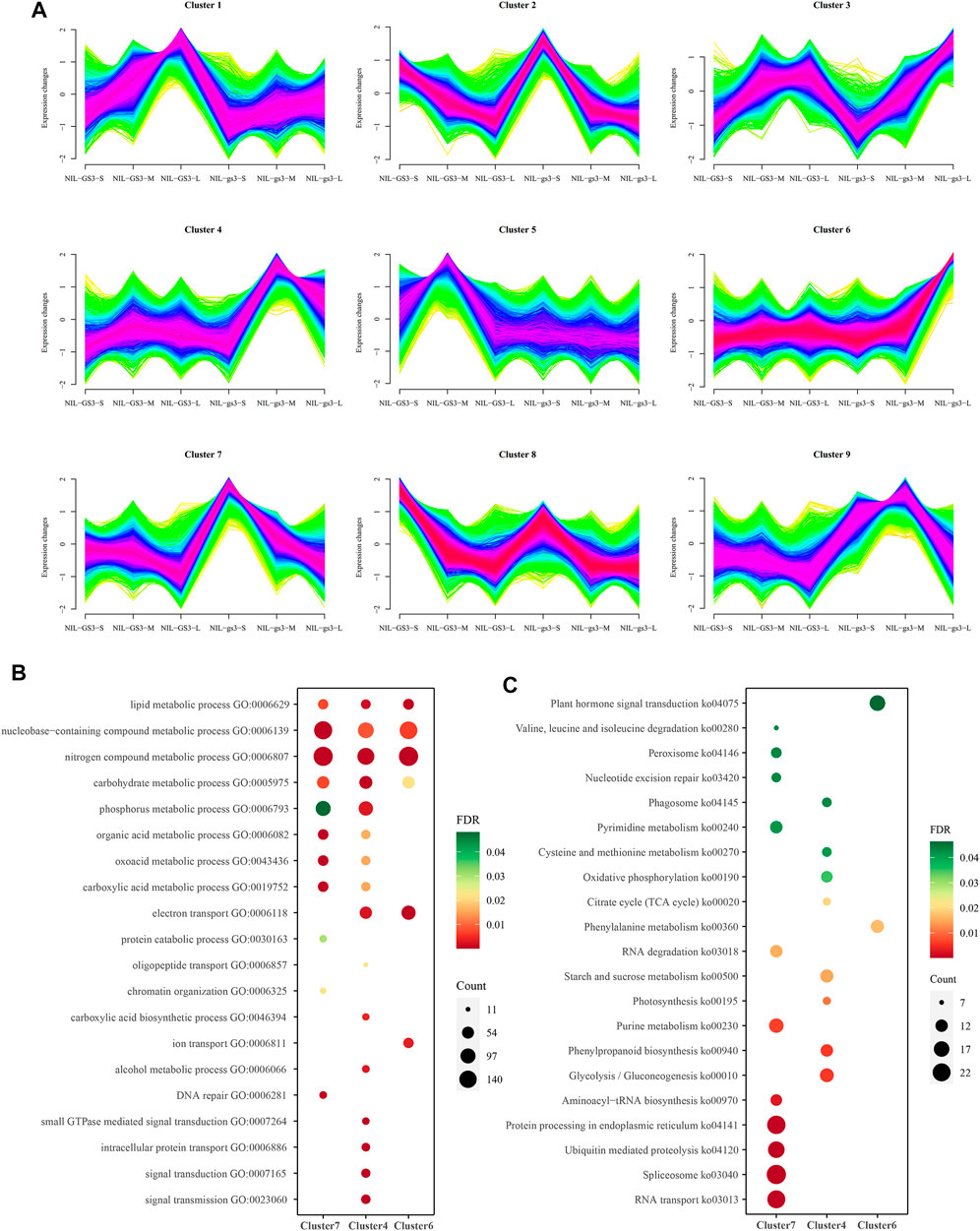
FIGURE 4. Clustering of expression profiles using a fuzzy clustering algorithm. (A) The expression patterns of 9 clusters generated by Mfuzz software. The expression values were represented by normalized Z-score. (B) GO enrichment analysis of cluster 7, 4, and 6, corresponding to different stages of NIL-GS3 and NIL-gs3 panicle development. Developmental stages include S (1–3 cm), M (4–6 cm), and L (8–10 cm); (C) KEGG enrichment analysis of cluster 7, 4, and 6.
GO and KEGG enrichment analysis of these 9 clusters showed many enriched biological processes and pathways corresponding to the relevant stages (Figure 4B, Supplementary Table S6). For example, genes associated with nitrogen compound metabolic process, lipid metabolic process, carbohydrate metabolic process, and nucleobase-containing compound metabolic process are highly enriched in cluster 7, 4, and 6, corresponding to three stages (S, M, and L). Those genes associated with the organic acid metabolic process, phosphorus metabolic process, and oxoacid metabolic process were significantly enriched in cluster 7 (S stage) and cluster 4 (M stage). Three gamma subunits, GS3 (Os03g0407400), GGC2 (Os08g0456600), and DEP1 (Os09g0441900), were predominantly expressed at the early stage of panicle development and then rapidly declined (Supplementary Table S3). Interestingly, small GTPase mediated signal transduction, intracellular protein transport, and signal transmission was significantly enriched in Cluster4 (M stage). Meanwhile, starch and sucrose metabolism, Glycolysis, and phenylpropanoid biosynthesis were also enriched explicitly in the M stage. Most genes such as OsRab6A1, OsRop4, OsWD40, and OsRho associated with the G-protein signaling pathway were significantly upregulated in the M stage (Figure 5A). Besides, genes associated with plant hormone signal transduction such as OsGSK1, OsRLCK98, OsXTH9, OsGH3.3, and OsRR10 were specifically enriched and upregulated in the L stage (Figure 5B). These results suggested that G-protein and hormone signaling pathways may show a synergistic interplay in regulating panicle development in rice.
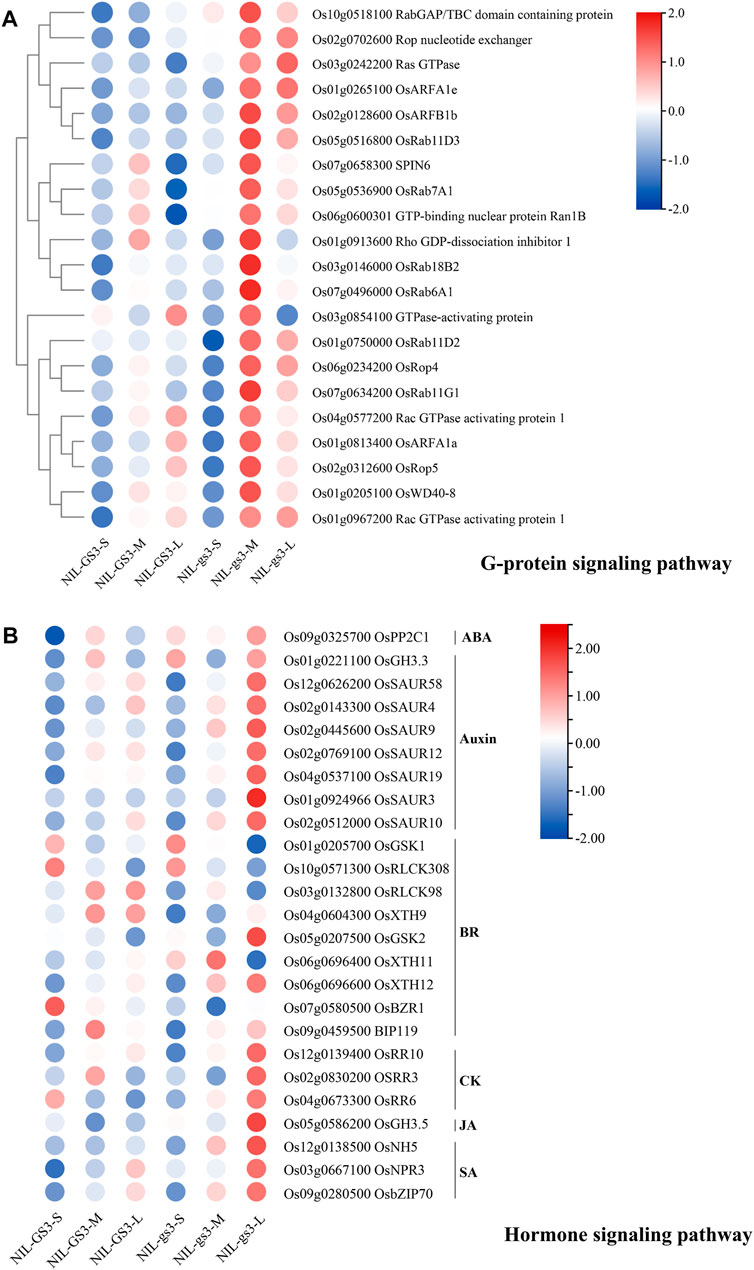
FIGURE 5. The expression profiles of genes associated with G-protein and hormone signaling pathways. (A) The heatmap of genes from the G-protein signaling pathway; (B) The heatmap of genes from the G-protein signaling pathway. FPKM values were normalized to the Z-scores.
Discussion
In rice, GS3 is a major QTL that affects grain length and weight. In large-grain varieties, an SNP (C→A) mutation in the second exon of the GS3 gene leads to premature termination of translation, thus suggesting that GS3 may function as a negative regulator affecting grain length and weight (Fan et al., 2006; Fan et al., 2009). The phenotypic differences between NIL-GS3 and NIL-gs3 are mainly due to functional differences in the GS3 alleles because NIL-GS3 produces a functional protein and NIL-gs3 produces a truncated protein and generates a long grain. The structure of the GS3 locus in the isogenic lines was consistent with the previously reported Zhenshan 97 and Minghui 63 lines (Mao et al., 2010). The NIL-gs3 group showed an increase in grain length and thousand-grain weight and a slight increase in grain width, with no significant change in grain thickness compared with NIL-GS3 (Figures 1A–E; Supplementary Table S1). These findings further confirmed that the GS3 locus is closely related to grain length and weight and that this locus has a negative effect on grain size (Mao et al., 2010). When examining the cytological effect of GS3 in the epidermal cells in glumes, the glume cell length increased, whereas the width decreased in NIL-gs3; thus, resulting in a more elongated morphology. In addition to affecting the morphology, the NIL-gs3 group also showed an increase in proliferation. Thus, the gs3 allele carried by NIL-gs3 increased the rice yield and provided an improved grain shape (Figure 1; Supplementary Table S1).
Previous studies that utilized NILs also identified many DEGs associated with rice growth and development (Moumeni et al., 2015; Tariq et al., 2018) and panicle development, with many of these DEGs associated with morphological development (Ke et al., 2016; Meng et al., 2017; Wang J et al., 2019). In this study, the observed phenotypic difference between the NIL-GS3 and NIL-gs3 groups predominantly resulted from functional differences. Thus, we examined the panicles of these NILs at three different stages (S, M, and L) and constructed transcriptional profiles. Comparative analysis identified DEGs in the NIL-gs3 group at the S (n = 887), M (n = 1768), and L (n = 1,478), thus suggesting that GS3 had a more significant influence on the middle and late stages of panicle development (Figure 3A). Besides, many differential expressed lncRNAs were also identified and showed dynamic changes during panicle development, which suggested that lncRNAs may also play a regulatory role in rice panicle development.
To better characterize the potential effect of NIL-gs3 in regulating panicle development, we further employed the c-means fuzzy clustering method for partitioning gene clusters that have a similar expression profile (Figure 4A). The clustering information could help identify genes underlining major biological processes and understanding how these genes are involved in complex panicle development. Nine clusters were generated, and clusters 7, 4, and 6 were correlated explicitly with different panicle development stages. Through GO and KEGG enrichment, many biological processes/pathways were significantly enriched across different development stages. G-protein and hormone signal pathways were noteworthy successively activated at the M and L stages of NIL-gs3 (Figures 4B,C). As shown in Figure 5, genes G-protein signaling pathway were significantly upregulated in the M stage of NIL-gs3, which indicated that the GS3 gene, as negative regulators, and activated the G-protein signaling pathway in NIL-gs3 lines (Figure 5A). Genes associated with hormone signal pathways, including Auxin, BR, CK were also significantly upregulated in the L stage of NIL-gs3 (Figure 5B). These results suggested that G-protein coupled with hormone signaling pathway regulated the panicle development and then affected kernel size, consistent with previous reports (Mao et al., 2010; Sun et al., 2018). Previous studies have shown that the BR signaling pathway is highly correlated with cell elongation and growth (Uozu et al., 2000; Jan et al., 2004; Hernandez-Nistal et al., 2010). Herein, OsGSK1, OsRLCK308 were mainly upregulated in early development. OsXTH11 was upregulated in the S stage and reached the peak in the M stage, whereas OsXTH12 was upregulated in the M stage and reached the peak in the L stage. Thus, different genes from the BR signaling pathway might play a distinct role in different development stages. Compared with the BR pathway, Auxin, CK, JA, and SA pathways were mainly activated in the L stage in NIL-gs3 lines. These findings suggested that the synergistic interplay of G-protein and hormone signaling pathway might play an essential role in rice panicles development and impact grain size.
Conclusion
GS3 is the major QTL that controls grain size in rice. In this study, we identified 887, 1,768, and 1,478 DEGs, including many lncRNAs among three periods when comparing the panicles of NIL-GS3 and NIL-gs3 via RNA-Seq. By further c-means fuzzy clustering, 9 clusters representing distinct expression patterns were generated. Many biological processes or pathways were significantly enriched in different development stages of NIL-gs3 lines. G-protein signaling pathway was mainly activated in the M stage, whereas multiple hormones singling pathways including Auxin, BR, CK, JA, and SA were activated in the L stage. These findings suggested that the difference in grain size between the NILs most likely be attributed to the complex synergistic interplay of G-protein and multiple hormone signaling pathways.
Materials and Methods
Plant Material Sampling and Grain Shape Trait Measurements
We collected a large grain Japonica variety (TD70) and a small grain Indica variety (Kasalath) (Zhang et al., 2015). We then constructed NILs (NIL-gs3 and NIL-GS3) using TD70 as a donor and Kasalath as the background by conventional six-generation backcrossing. The NILs were planted in a greenhouse at the Jiangsu Academy of Agricultural Sciences (Nanjing, China). We used the small (S, 1–3 cm), medium (M, 4–6 cm), and large (L, 8–10 cm) panicle size for specimen collection. We also collected three biological repeats for each of these periods for each of the two NILs (n = 18). Spikelets were snap-frozen in liquid nitrogen following separation and stored at −80°C. The growth and development of these spikelets were highly consistent. We obtained grain length, width, and thickness measurements after harvesting and measured the thousand-grain weight after harvesting and drying at 37°C for 1 week. We observed epidermal cell morphology within the mature grain glumes with a scanning electron microscope (SEM; EVO-LS10, Carl Zeiss Microscopy GmbH, Oberkochen, Germany).
RNA Extraction and Construction of a cDNA Library
Total RNA was extracted from the spikelet tissue using a TRIzol® RNA Purification Kit (Invitrogen, Carlsbad, CA, United States). We determined RNA purity, concentration, and integrity using a NanoDrop 2000 Spectrophotometer (ThermoFisher Scientific, Waltham, MA, United States), Qubit® 4.0 Spectrophotometer (Life Technologies, Carlsbad, CA, United States), and Agilent 2,100 Bioanalyzer® (Agilent Technologies, Palo Alto, CA, United States), respectively. The mRNA was then enriched using Oligo (dT) magnetic beads, and double-stranded cDNA (ds-cDNA) synthesis was performed. The ds-cDNAs were then purified using Agencourt AMPure XP beads (Beckmann Coulter, Pasadena, CA, United States), followed by end repair, polyadenylation, and sequencing connector. Finally, we used AMPure XP beads to screen for fragment size and polymerase chain reaction (PCR) amplification before constructing the sequencing library. The library was sequenced on an Illumina NovaSeq 6,000 sequencer in the PE150 mode (paired-end 150 bp).
Long Non-coding RNA Prediction
We cleaned the raw sequences by removing adapter sequences, and low-quality reads with Q20 (a Phred quality score of 20 represents an error rate of 1 in 100) using the Fastp program (Chen et al., 2018), and eliminated any sequences ≤50 bp. The cleaned reads were then mapped to Nipponbare reference genome (https://rapdb.dna.affrc.go.jp, IRGSP-1.0 version) with the Hisat2 program (Kawahara et al., 2013; Pertea et al., 2016). We extracted unique reads (MAQ ≥20) for analysis using SAM tools v1.6 (Li et al., 2009). We updated the genome annotation with a reference annotation-based transcript (RABT) method, based on IRGSP-1.0 GTF annotation file (https://rapdb.dna.affrc.go.jp), using the StringTie program (Pertea et al., 2016). We extracted updated transcripts using the Gffreads program and performed a potential coding prediction using the CPC2 (Kang et al., 2017) and PLEK programs (Li et al., 2014). We considered transcripts with a length greater than 200 bp and consistently identified them as non-coding using both algorithms to be lncRNAs.
Identification of Differentially Expressed Genes
We determined the expression abundance of each transcript based on the fragments per kilobase of transcript per million fragments mapped (FPKM) value and identified unexpressed or false positive transcripts using the largest FPKM value < 1 in multiple samples (Trapnell et al., 2010). We identified differentially expressed genes (DEGs) at different stages of panicle development using DESeq2 software (Love et al., 2014) with a Log2 (fold-change) ≥1 and q-value <0.05 required. We then visualized DEG commonalities between samples using a Venn plot.
Fuzzy C-Means Clustering
Expressed genes from three development stages in NILs were grouped into different clusters using the Mfuzz package in R with fuzzy c-means (Kumar and Futschik, 2007). The different clusters represented the different expression patterns during rice panicle development. The gene expression values were standardized to have a mean value of zero and one standard deviation for each gene profile. The transformed expression values were then clustered using the fuzzy c-means clustering algorithm by the Mfuzz package.
GO and KEGG Enrichment Analysis
The ClusterProfile package (Yu et al., 2012) was used for enrichment analysis and visualization using the Gene Ontology (GO) and Kyoto Encyclopedia of Genes and Genomes (KEGG) databases, with an FDR <0.05 required for significance. Heat maps of the gene expression profiles were generated using R packages.
Validation by Quantitative Real-Time PCR
To validate the reliability of the RNA-Seq analysis, we performed quantitative real-time PCR (qRT-PCR) using ten randomly selected genes. The primers were designed using Primer Premier v.5.0 software (Premier Biosoft International, Palo Alto, CA, United States). We synthesized the first-strand cDNA using a TransScript One-Step gDNA Removal and cDNA Synthesis SuperMix Kit (TransGen Biotech, Beijing, China). We conducted the qRT-PCR on an ABI StepOnePlus Real-Time PCR System (Applied Biosystems, Foster City, CA, United States) using SYBR Premix Ex Taq (TaKaRa, Dalian, China). We used Actin1 (Os03g0718100) as an internal standard to calculate the relative expression level and determined expression values based on the relative quantitative method (Livak and Schmittgen, 2001).
Data Availability Statement
The datasets presented in this study can be found in National Center for Biotechnology Information (NCBI) BioProject database under accession number PRJNA663252.
Author Contributions
YZ and YL conceived and designed the study. WL, FH, and YL performed the RNA-Seq analysis and the lncRNA discovery. WL, WQ, CZ, TC, and CW constructed the NILs, performed phenotypic analysis and the qRT-PCR experiment. WL and YL wrote the manuscript. All authors read and approved the manuscript.
Funding
This work was supported by the National Natural Science Foundation of China (31771761, 31901485), Key Laboratory of Jiangsu Province for Agrobiology (JKLA2022-ZD01) and China Agriculture Research System (CARS-01-67).
Conflict of Interest
The authors declare that the research was conducted in the absence of any commercial or financial relationships that could be construed as a potential conflict of interest.
Publisher’s Note
All claims expressed in this article are solely those of the authors and do not necessarily represent those of their affiliated organizations, or those of the publisher, the editors and the reviewers. Any product that may be evaluated in this article, or claim that may be made by its manufacturer, is not guaranteed or endorsed by the publisher.
Supplementary Material
The Supplementary Material for this article can be found online at: https://www.frontiersin.org/articles/10.3389/fgene.2022.857143/full#supplementary-material
Supplementary Figure S1 | Quantitative analysis of 10 selected DEGs in RNA-Seq. Each bar represents SD; S, 526 young panicle 1–3 mm; M, young panicle 4–6 mm; L, young panicle 8–10 mm.
References
Chen, S., Zhou, Y., Chen, Y., and Gu, J. (2018). Fastp: an Ultra-fast All-In-One FASTQ Preprocessor. Bioinformatics 34, i884–i890. doi:10.1093/bioinformatics/bty560
Fan, C., Xing, Y., Mao, H., Lu, T., Han, B., Xu, C., et al. (2006). GS3, a Major QTL for Grain Length and Weight and Minor QTL for Grain Width and Thickness in rice, Encodes a Putative Transmembrane Protein. Theor. Appl. Genet. 112, 1164–1171. doi:10.1007/s00122-006-0218-1
Fan, C., Yu, S., Wang, C., and Xing, Y. (2009). A Causal C-A Mutation in the Second Exon of GS3 Highly Associated with rice Grain Length and Validated as a Functional Marker. Theor. Appl. Genet. 118 (118), 465–472. doi:10.1007/s00122-008-0913-1
Gao, X., Zhang, X., Lan, H., Huang, J., Wang, J., and Zhang, H. (2015). The Additive Effects of GS3 and qGL3 on rice Grain Length Regulation Revealed by Genetic and Transcriptome Comparisons. BMC Plant Biol. 15, 156. doi:10.1186/s12870-015-0515-4
Guo, H., Mendrikahy, J. N., Xie, L., Deng, J., Lu, Z., Wu, J., et al. (2017). Transcriptome Analysis of Neo-Tetraploid rice Reveals Specific Differential Gene Expressions Associated with Fertility and Heterosis. Sci. Rep. 7, 40139. doi:10.1038/srep40139
Han, L., Mu, Z., Luo, Z., Pan, Q., and Li, L. (2019). New lncRNA Annotation Reveals Extensive Functional Divergence of the Transcriptome in maize. J. Integr. Plant Biol. 61, 394–405. doi:10.1111/jipb.12708
Hernandez-Nistal, J., Martin, I., Labrador, E., and Dopico, B. (2010). The Immunolocation of XTH1 in Embryonic Axes during Chickpea Germination and Seedling Growth Confirms its Function in Cell Elongation and Vascular Differentiation. J. Exp. Bot. 61, 4231–4238. doi:10.1093/jxb/erq223
Huang, R., Jiang, L., Zheng, J., Wang, T., Wang, H., Huang, Y., et al. (2013). Genetic Bases of rice Grain Shape: So many Genes, So Little Known. Trends Plant Sci. 18, 218–226. doi:10.1016/j.tplants.2012.11.001
Jan, A., Yang, G., Nakamura, H., Ichikawa, H., Kitano, H., Matsuoka, M., et al. (2004). Characterization of a Xyloglucan Endotransglucosylase Gene that Is Up-Regulated by Gibberellin in Rice. Plant Physiol. 136, 3670–3681. doi:10.1104/pp.104.052274
Kang, Y.-J., Yang, D.-C., Kong, L., Hou, M., Meng, Y.-Q., Wei, L., et al. (2017). CPC2: a Fast and Accurate Coding Potential Calculator Based on Sequence Intrinsic Features. Nucleic Acids Res. 45, W12–W16. doi:10.1093/nar/gkx428
Kawahara, Y., de la Bastide, M., Hamilton, J. P., Kanamori, H., McCombie, W. R., Ouyang, S., et al. (2013). Improvement of the Oryza Sativa Nipponbare Reference Genome Using Next Generation Sequence and Optical Map Data. Rice 6, 4. doi:10.1186/1939-8433-6-4
Ke, S., Liu, X.-J., Luan, X., Yang, W., Zhu, H., Liu, G., et al. (2018). Genome-wide Transcriptome Profiling Provides Insights into Panicle Development of rice (Oryza Sativa L.). Gene 675, 285–300. doi:10.1016/j.gene.2018.06.105
Kumar, L., and Futschik, M. E. (2007). Mfuzz: A Software Package for Soft Clustering of Microarray Data. Bioinformation 2, 5–7. doi:10.6026/97320630002005
Lee, J. H., Ryu, H.-S., Chung, K. S., Posé, D., Kim, S., Schmid, M., et al. (2013). Regulation of Temperature-Responsive Flowering by MADS-Box Transcription Factor Repressors. Science 342, 628–632. doi:10.1126/science.1241097
Li, A., Zhang, J., and Zhou, Z. (2014). PLEK: A Tool for Predicting Long Non-coding RNAs and Messenger RNAs Based on an Improved K-Mer Scheme. BMC Bioinformatics 15, 1–10. doi:10.1186/1471-2105-15-311
Li, H., Handsaker, B., Wysoker, A., Fennell, T., Ruan, J., Homer, N., et al. (2009). The Sequence Alignment/Map Format and SAMtools. Bioinformatics 25, 2078–2079. doi:10.1093/bioinformatics/btp352
Liu, Q., Han, R., Wu, K., Zhang, J., Ye, Y., Wang, S., et al. (2018). G-protein βγ Subunits Determine Grain Size through Interaction with MADS-Domain Transcription Factors in rice. Nat. Commun. 9, 852. doi:10.1038/s41467-018-03047-9
Livak, K. J., and Schmittgen, T. D. (2001). Analysis of Relative Gene Expression Data Using Real-Time Quantitative PCR and the 2−ΔΔCT Method. Methods 25, 402–408. doi:10.1006/meth.2001.1262
Love, M. I., Huber, W., and Anders, S. (2014). Moderated Estimation of Fold Change and Dispersion for RNA-Seq Data with DESeq2. Genome Biol. 15, 550. doi:10.1186/s13059-014-0550-8
Lv, Y., Hu, F., Zhou, Y., Wu, F., and Gaut, B. S. (2019). Maize Transposable Elements Contribute to Long Non-coding RNAs that Are Regulatory Hubs for Abiotic Stress Response. BMC Genomics 20, 864. doi:10.1186/s12864-019-6245-5
Mao, H., Sun, S., Yao, J., Wang, C., Yu, S., Xu, C., et al. (2010). Linking Differential Domain Functions of the GS3 Protein to Natural Variation of Grain Size in rice. Proc. Natl. Acad. Sci. 107, 19579–19584. doi:10.1073/pnas.1014419107
Meng, Q., Li, X., Zhu, W., Yang, L., Liang, W., Dreni, L., et al. (2017). Regulatory Network and Genetic Interactions Established by OsMADS34 in rice Inflorescence and Spikelet Morphogenesis. J. Integr. Plant Biol. 59, 693–707. doi:10.1111/jipb.12594
Miao, J., Yang, Z., Zhang, D., Wang, Y., Xu, M., Zhou, L., et al. (2019). Mutation of RGG 2 , Which Encodes a Type B Heterotrimeric G Protein γ Subunit, Increases Grain Size and Yield Production in rice. Plant Biotechnol. J. 17, 650–664. doi:10.1111/pbi.13005
Moumeni, A., Satoh, K., Venuprasad, R., Serraj, R., Kumar, A., Leung, H., et al. (2015). Transcriptional Profiling of the Leaves of Near-Isogenic rice Lines with Contrasting Drought Tolerance at the Reproductive Stage in Response to Water Deficit. BMC Genomics 16, 1110. doi:10.1186/s12864-015-2335-1
Pertea, M., Kim, D., Pertea, G. M., Leek, J. T., and Salzberg, S. L. (2016). Transcript-level Expression Analysis of RNA-Seq Experiments with HISAT, StringTie and Ballgown. Nat. Protoc. 11, 1650–1667. doi:10.1038/nprot.2016.095
Si, L., Chen, J., Huang, X., Gong, H., Luo, J., Hou, Q., et al. (2016). OsSPL13 Controls Grain Size in Cultivated rice. Nat. Genet. 48, 447–456. doi:10.1038/ng.3518
Sun, S., Wang, L., Mao, H., Shao, L., Li, X., Xiao, J., et al. (2018). A G-Protein Pathway Determines Grain Size in rice. Nat. Commun. 9, 851. doi:10.1038/s41467-018-03141-y
Tariq, R., Wang, C., Qin, T., Xu, F., Tang, Y., Gao, Y., et al. (2018). Comparative Transcriptome Profiling of Rice Near-Isogenic Line Carrying Xa23 under Infection of Xanthomonas Oryzae Pv. Oryzae. Ijms 19, 717. doi:10.3390/ijms19030717
Trapnell, C., Williams, B. A., Pertea, G., Mortazavi, A., Kwan, G., van Baren, M. J., et al. (2010). Transcript Assembly and Quantification by RNA-Seq Reveals Unannotated Transcripts and Isoform Switching during Cell Differentiation. Nat. Biotechnol. 28, 511–515. doi:10.1038/nbt.1621
Uozu, S., Tanaka-Ueguchi, M., Kitano, H., Hattori, K., and Matsuoka, M. (2000). Characterization of XET-Related Genes of Rice. Plant Physiol. 122, 853–860. doi:10.1104/pp.122.3.853
Wang, J., Zhang, Q., Wang, Y., Huang, J., Luo, N., Wei, S., et al. (2019). Analysing the rice Young Panicle Transcriptome Reveals the Gene Regulatory Network Controlled by TRIANGULAR HULL1. Rice 12, 6. doi:10.1186/s12284-019-0265-2
Wang, S., Li, S., Liu, Q., Wu, K., Zhang, J., Wang, S., et al. (2015). The OsSPL16-GW7 Regulatory Module Determines Grain Shape and Simultaneously Improves rice Yield and Grain Quality. Nat. Genet. 47, 949–954. doi:10.1038/ng.3352
Wang, S., Wu, K., Yuan, Q., Liu, X., Liu, Z., Lin, X., et al. (2012). Control of Grain Size, Shape and Quality by OsSPL16 in rice. Nat. Genet. 44, 950–954. doi:10.1038/ng.2327
Wang, Y., Wang, Y., and Deng, D. (2019). Multifaceted Plant G Protein: Interaction Network, Agronomic Potential, and beyond. Planta 249, 1259–1266. doi:10.1007/s00425-019-03112-7
Wang, Y., Xiong, G., Hu, J., Jiang, L., Yu, H., Xu, J., et al. (2015). Copy Number Variation at the GL7 Locus Contributes to Grain Size Diversity in rice. Nat. Genet. 47, 944–948. doi:10.1038/ng.3346
Wu, W., Liu, X., Wang, M., Meyer, R. S., Luo, X., Ndjiondjop, M.-N., et al. (2017). A Single-Nucleotide Polymorphism Causes Smaller Grain Size and Loss of Seed Shattering during African rice Domestication. Nat. Plants 3, 17064. doi:10.1038/nplants.2017.64
Xia, D., Zhou, H., Liu, R., Dan, W., Li, P., Wu, B., et al. (2018). GL3.3, a Novel QTL Encoding a GSK3/SHAGGY-like Kinase, Epistatically Interacts with GS3 to Produce Extra-long Grains in Rice. Mol. Plant 11, 754–756. doi:10.1016/j.molp.2018.03.006
Xu, R., Li, N., and Li, Y. (2019). Control of Grain Size by G Protein Signaling in rice. J. Integr. Plant Biol. 61, 533–540. doi:10.1111/jipb.12769
Yan, S., Zou, G., Li, S., Wang, H., Liu, H., Zhai, G., et al. (2011). Seed Size Is Determined by the Combinations of the Genes Controlling Different Seed Characteristics in rice. Theor. Appl. Genet. 123, 1173–1181. doi:10.1007/s00122-011-1657-x
Ying, J.-Z., Ma, M., Bai, C., Huang, X.-H., Liu, J.-L., Fan, Y.-Y., et al. (2018). TGW3, a Major QTL that Negatively Modulates Grain Length and Weight in Rice. Mol. Plant 11, 750–753. doi:10.1016/j.molp.2018.03.007
Yu, G., Wang, L.-G., Han, Y., and He, Q.-Y. (2012). clusterProfiler: an R Package for Comparing Biological Themes Among Gene Clusters. OMICS: A J. Integr. Biol. 16, 284–287. doi:10.1089/omi.2011.0118
Zha, M., Imran, M., Wang, Y., Xu, J., Ding, Y., and Wang, S. (2019). Transcriptome Analysis Revealed the Interaction Among Strigolactones, Auxin, and Cytokinin in Controlling the Shoot Branching of rice. Plant Cel Rep 38, 279–293. doi:10.1007/s00299-018-2361-y
Zhang, W., Sun, P., He, Q., Shu, F., and Deng, H. (2018). Transcriptome Analysis of Near-Isogenic Line Provides Novel Insights into Genes Associated with Panicle Traits Regulation in rice. PLoS ONE 13, e0199077. doi:10.1371/journal.pone.0199077
Zhang, X., Wang, J., Huang, J., Lan, H., Wang, C., Yin, C., et al. (2012). Rare Allele of OsPPKL1 Associated with Grain Length Causes Extra-large Grain and a Significant Yield Increase in rice. Proc. Natl. Acad. Sci. 109, 21534–21539. doi:10.1073/pnas.1219776110
Zhang, Y.-C., and Chen, Y.-Q. (2013). Long Noncoding RNAs: New Regulators in Plant Development. Biochem. Biophysical Res. Commun. 436, 111–114. doi:10.1016/j.bbrc.2013.05.086
Zhang, Y.-C., Liao, J.-Y., Li, Z.-Y., Yu, Y., Zhang, J.-P., Li, Q.-F., et al. (2014). Genome-wide Screening and Functional Analysis Identify a Large Number of Long Noncoding RNAs Involved in the Sexual Reproduction of rice. Genome Biol. 15, 512. doi:10.1186/s13059-014-0512-1
Zhang, Y. D., Zheng, J., Liang, Z. K., Liang, Y. L., Peng, Z. H., and Wang, C. L. (2015). Verification and Evaluation of Grain QTLs Using RILs from TD70 X Kasalath in rice. Genet. Mol. Res. 14, 14882–14892. doi:10.4238/2015.November.18.53
Zhang, Y., Zhang, Z., Sun, X., Zhu, X., Li, B., Li, J., et al. (2019). Natural Alleles of GLA for Grain Length and Awn Development Were Differently Domesticated in rice Subspecies Japonica and Indica. Plant Biotechnol. J. 17, 1547–1559. doi:10.1111/pbi.13080
Keywords: rice, GS3, NIL, panicle, g-protein, hormones
Citation: Liang W, Hu F, Qi W, Zhao C, Chen T, Wang C, Lv Y and Zhang Y (2022) Comprehensive Transcriptome Analysis of GS3 Near-Isogenic Lines During Panicle Development in Rice (Oryza sativa L.). Front. Genet. 13:857143. doi: 10.3389/fgene.2022.857143
Received: 18 January 2022; Accepted: 15 February 2022;
Published: 01 March 2022.
Edited by:
Xiaoming Song, North China University of Science and Technology, ChinaReviewed by:
Wei Xuan, Nanjing Agricultural University, ChinaYi Han, Anhui Agricultural University, China
Copyright © 2022 Liang, Hu, Qi, Zhao, Chen, Wang, Lv and Zhang. This is an open-access article distributed under the terms of the Creative Commons Attribution License (CC BY). The use, distribution or reproduction in other forums is permitted, provided the original author(s) and the copyright owner(s) are credited and that the original publication in this journal is cited, in accordance with accepted academic practice. No use, distribution or reproduction is permitted which does not comply with these terms.
*Correspondence: Yuanda Lv, THlkMDUyN0AxMjYuY29t; Yadong Zhang, emhhbmd5ZEBqYWFzLmFjLmNu