- 1Genetic Metabolic Unit, Department of Pediatrics, Advanced Pediatric Centre, Post Graduate Institute of Medical Education & Research, Chandigarh, India
- 2Allergy Immunology Unit, Department of Pediatrics, Advanced Pediatric Centre, Post Graduate Institute of Medical Education & Research, Chandigarh, India
Kawasaki disease (KD) is a common childhood systemic vasculitis with a special predilection for coronary arteries. Even after more than five decades of the initial description of the disease, the etiology of KD remains an enigma. This transcriptome data re-analysis study aimed to elucidate the underlying pathogenesis of KD using a bioinformatic approach to identify differentially expressed genes (DEGs) to delineate common pathways involved in KD. Array datasets from the Gene Expression Omnibus database were extracted and subjected to comparative meta-analysis for the identification of prominent DEGs. Fifteen hub genes with high connectivity were selected from these DEGs (IL1B, ITGAM, TLR2, CXCL8, SPI1, S100A12, MMP9, PRF1, TLR8, TREM1, CD44, UBB, FCER1G, IL7R, and FCGR1A). Of these 15 genes, five genes (CXCL8, FCGR1A, IL1B, TLR2, and TLR8) were found to be involved in neutrophil degranulation. To gain further insight into the molecular mechanism, a protein–protein network was established. Significantly enriched pathways based on the above-mentioned genes were mainly centered on biological regulation and signaling events. In addition, the pathway analysis also indicated that the majority of the DEGs in KD were enriched in systemic lupus erythematosus, suggesting a strong interplay between immunological and genetic factors in the pathogenesis of KD. These findings could significantly aid in identifying therapeutic targets and understanding KD biosignatures to design a biomarker panel for early diagnosis and severity of the disease.
Introduction
Kawasaki Disease (KD) is an acute childhood febrile illness predominately affecting children below 5 years. It is a systemic vasculitis with a special predilection for coronary arteries (Newburger et al., 2004). It is now recognized as the most common cause of acquired heart disease in children in the developed world (Banday et al., 2021). The incidence of the KD ranges from 10 to 25 per 100,000 children below five years in North America and Europe. The highest incidence of KD is reported in children of Japanese ancestry; 350 per 100,000 children below 5 (Singh et al., 2015). Approximately 1% of all Japanese children would develop KD by the age of 10. Although many centers in India are now diagnosing children with KD on a regular basis, the vast majority of patients are perhaps still going undiagnosed and unreported (Singh et al., 2015). KD has the potential to cause severe complications and significant morbidity and mortality. We have reported that the mortality rate in our cohort has been 0.8%. This is significantly higher than mortality figures of ≤0.04% reported in developed countries (Singh et al., 2016).
Even after more than five decades of the initial description of the disease, the diagnosis of KD is still clinical and based on a constellation of clinical features. There is no pathognomonic laboratory feature. Differential diagnosis of KD includes several febrile illnesses in children, including viral infections (e.g., measles, Epstein Barr virus, and adenovirus), scarlet fever, toxic shock syndrome, and drug reactions like Stevens-Johnson syndrome or serum sickness. Clinical symptoms of KD include persistent fever for more than five days, rash, swelling of the dorsum of hands and feet, red strawberry tongue, conjunctival nonsuppurative injection, cervical lymphadenopathy, periungual peeling, and diffuse mucosal inflammation (McCrindle et al., 2017; CDC, 2020). Children with scarlet fever do not have lip changes and eye changes, which are present in KD. While rash in scarlet fever is characteristically sandpaper, KD can have a pleomorphic rash. Elevated antistreptolysin O (ASO) titer, positive throat culture for β hemolytic streptococcal group A, and brisk response to antimicrobials are other characteristic features of scarlet fever. Similarly, one can differentiate KD from other common differentials based on the constellation of clinical features (Jindal et al., 2019). Standard of care involves high-dose of intravenous immunoglobulin (IVIG) along with oral aspirin in the acute phase of the disease, which helps to resolve inflammation and minimize the risk of coronary arteries abnormalities (CAAs) (Oates-Whitehead et al., 2003). Approximately 10%–20% of children with KD doses did not respond to first-line therapy and persisted in having inflammation, known as IVIG resistant KD. Despite timely treatment, 3%–5% of patients with KD can develop CAAs. In patients having IVIG resistant KD, the chances of development of CAAs remain high (Ogata et al., 2013; Skochko et al., 2018; Brogan et al., 2020). KD remains the most common cause of acquired heart disease among children in Japan, North America, and Europe (Banday et al., 2021).
Multiple theories regarding the etiology of KD have been hypothesized such as infectious theory, infectious plus autoimmunity, RNA virus theory, and superantigen theory. However, none of them has been independently able to provide an exact mechanism for the initiation and progression of pathogenic mechanisms in KD (Ramphul and Mejias, 2018; Nakamura et al., 2019). The present consensus strongly indicates a complex interplay of infectious triggers in children with a genetic predisposition of KD, followed by an abnormal immune response (Dietz et al., 2017; Nakamura et al., 2019).
Over the last few years, high-throughput platforms such as microarray have emerged as potent tools to detect differential gene expression profiles and have been recognized as significant clinical approaches with efficient diagnosis at the molecular level, prognostic prediction, stratification of patients, and identification of therapeutic targets. To date, quite a number of genes have been examined in KD, which were usually selected based on information regarding their function in inflammation, immune response, and other biological mechanisms. Recently, gene expression profiling studies have been conducted to understand the pathophysiology of KD (Hoang et al., 2014; Dietz et al., 2017; Wu et al., 2019; Rahmati et al., 2020; Gao et al., 2021); however, the prognostic power of identified genes still remains unclear. Therefore, understanding the pathogenesis of KD is essential for identifying novel pathways that can be targeted for therapy. The current study was conducted to find out the most important hub genes among the known ones.
In our recent study, we explored the role of epigenetic factors in modulating the gene expression in KD (Sharma et al., 2021). Transcriptomic profiles in KD can reveal perturbations caused by inflammation or infection, which could help to delineate the pathogenesis of KD. In the present study, we extracted a large-scale expression dataset of a similar microarray-based platform to investigate the pathogenesis of KD. Using an integrated transcriptomic approach, we identified differentially expressed genes (DEGs) in KD. The underlying pathways were recognized using functional annotation and via constructing protein–protein interaction (PPI) network. This data from the present study could help to conceptualize the molecular events underpinning KD and identify diagnostic biosignature and design a biomarker panel.
Materials and Methods
Microarray Data
Data of microarray samples with suitable gene expression was obtained from the NCBI Gene Expression Omnibus (GEO) database (http://www.ncbi.nlm.nih.gov/geo/) (Edgar et al., 2002). Using the keywords “Kawasaki Disease and Homo sapiens,” a thorough search of the GEO database was performed. Furthermore, the ARGEOS web tool (https://ar-geos.org) was also used for the selection of datasets from various public databases. After a systematic review, we included GSE73461, GSE63881, GSE73463, and GSE68004, GEO datasets that belong to the same array platform GPL10558. The PRISMA checklist was followed for this study (Supplementary Figure S1).
Differentially Expressed Genes Screening
For microarray expression data analysis ExAtlas meta-analysis software was used (Sharov et al., 2015). The four GEO datasets included in the present study were extracted from the GEO database. Data normalization was carried out using the quantile method. From the sample file, unpaired samples were removed, followed by the generation of the gene expression matrix file. Using correlation of log10-transformed expression level with other data, the quality of individual samples and level of global standard deviation were evaluated with respect to a set of pre-selected housekeeping genes. Low-quality samples were removed from the datasets.
For statistical analysis, ExAtlas uses the algorithm applied in the NIA Array Analysis (Zhou et al., 2019). The significance of gene expression change was assessed by false discovery rate (FDR) instead of p-values.
Standard Meta-Analysis
In the pairwise comparison section of ExAtlas, one of the KD expression profiles was added as a sample for examination, and for baseline control, its adjacent comparative pair was added. Then, more gene expression profile pairs were added using the meta-analysis section. Furthermore, to perform the meta-analysis, a random-effect model was used to take into account the variance of heterogeneity between the studies. For each, gene symbol analysis was performed, and their effect was represented in terms of combined log-10 ratios and fold changes. FDR <0.05, p-value ≤0.05 and change of ≥2-folds in gene expression were considered significant.
Protein–Protein Interaction Network Construction and Hub Gene Identification
Protein–protein interaction (PPI) network analysis was performed using the Search Tool for the Retrieval of Interacting Genes (STRING) database (https://string-db.org/). To assess possible PPI correlations, previously identified DEGs were mapped to the STRING database, followed by the extraction of PPI pairs. Cytoscape software v3.9.0 (https://cytoscape.org/) with the CytoHubba plugin was then employed to visualize the PPI network. In our study, the top 15 genes were considered as hub genes.
Pathway Enrichment Analysis
The biological processes that are involved with the DEGs, along with the functional enrichment analysis, were studied using the BINGO app (Maere et al., 2005) of Cytoscape. A hypergeometric test was carried out using Benjamini and Hochberg FDR correction. The Gene Ontology (GO) biological process was selected as the ontology file for executing enrichment analyses. Furthermore, the Kyoto Encyclopedia of Genes and Genomes (KEGG) pathway (Kanehisa and Goto, 2000) enrichment analysis was performed using the online tool Database for Annotation, Visualization, and Integrated Discovery (DAVID) (Version 6.8; https://david.ncifcrf.gov/home.jsp) (Huang et al., 2009).
Results
A systematic search of the studies was carried out up to December 2021. The search with the keywords “Kawasaki Disease” in Homo sapiens and “expression profiling by array” resulted in 13 microarray gene expression datasets (Table 1). We have combined those studies which implement the same methodology/platform and where >2 datasets were available. From 13 data sets, we have chosen four datasets which belong to the same platform GPL10558 (Table 2).
Meta-Analysis
The meta-analysis identified overall 74 differentially over-expressed and 52 repressed genes in KD compared to the matched adjacent control samples. A list of the top 25 upregulated genes and 25 downregulated genes is given in Tables 3 and 4.
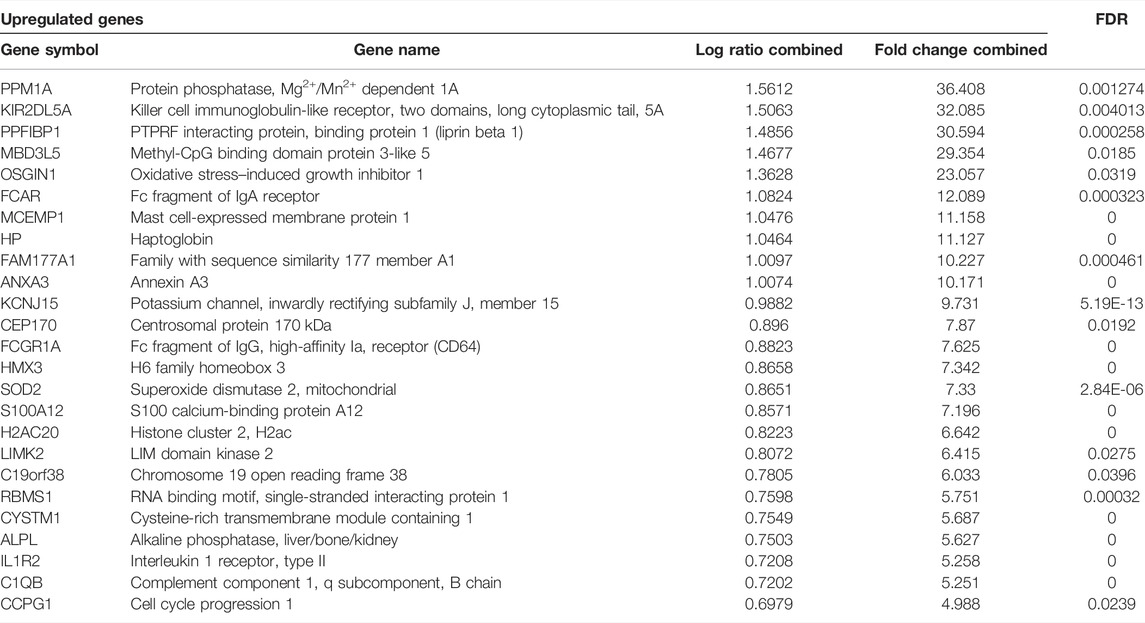
TABLE 3. Top 25 upregulated genes of the microarray meta-analyses along with their fold change values.
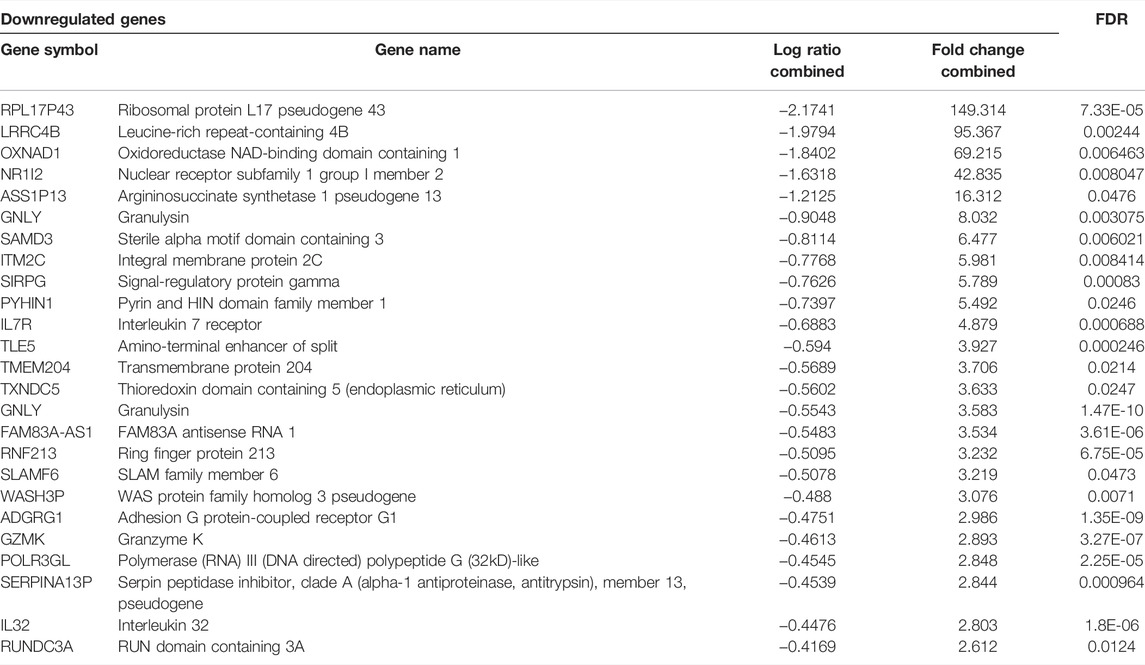
TABLE 4. Top 25 downregulated genes of the microarray meta-analyses along with their fold change values.
Protein–Protein Interaction Network
The PPI network analysis was performed to look for the physical and functional links of proteins encoded by the identified DEGs in KD. A confidence score cut-off >0.9 was considered to construct the PPI network (Figure 1).
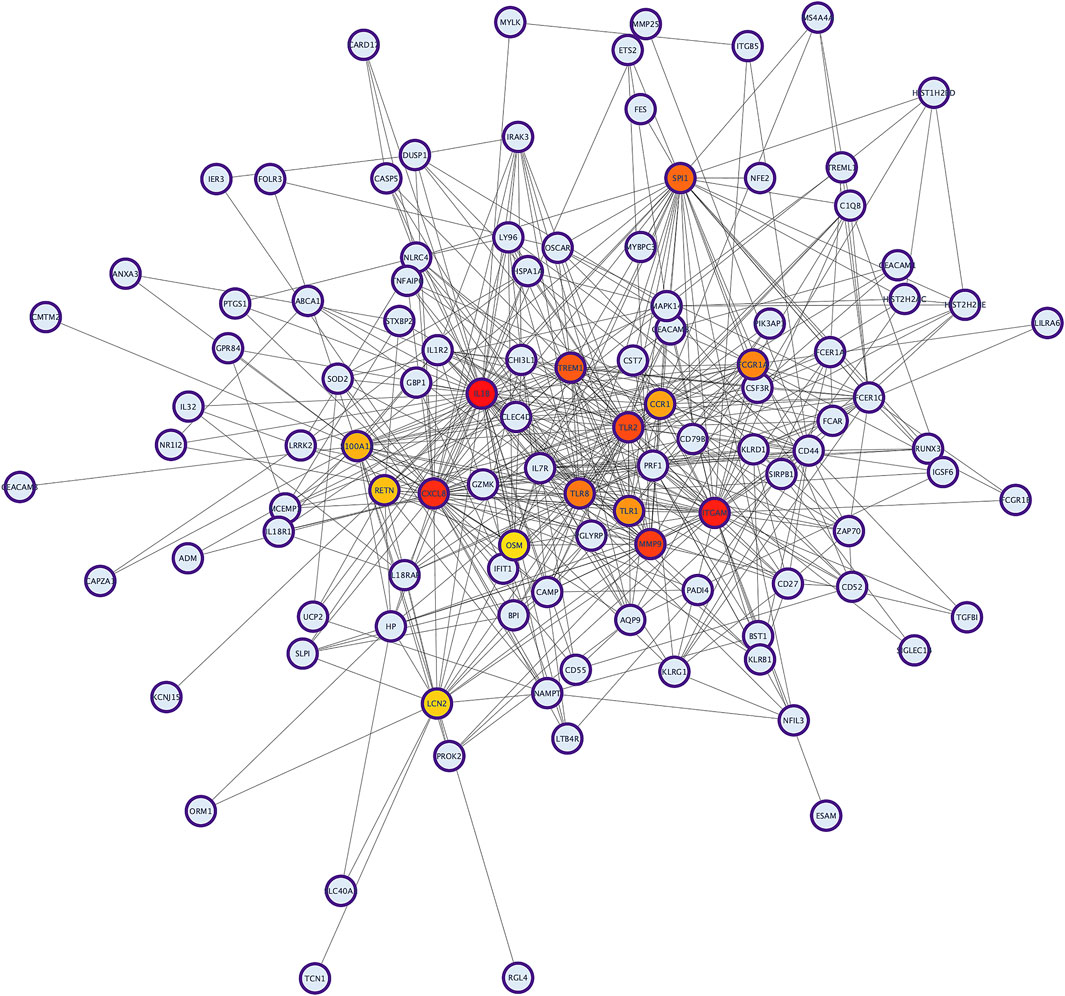
FIGURE 1. The protein–protein interaction (PPI) network analysis of differentially expressed genes in KD. The network was constructed by Cytoscape based on the PPI correlations from the STRING database.
To identify hub proteins in the PPI network, the degree of each node in the network was calculated by using the Network Analyzer tool of Cytoscape software. The degree of a node is defined as the number of edges connected to the node. Nodes with higher degrees play a crucial role in the organization of the PPI network, and therefore they might be more crucial and relevant than non-hub genes (Vallabhajosyula et al., 2009). In this study, nodes with degrees >15 are considered to indicate “hub proteins” and are presented in Table 5. Interleukin 1-Beta (IL1β) had the highest degree of node (54), followed by Integrin αM (ITGAM) and Toll-like receptor-2 (TLR2), with their degrees of nodes being 44 and 42, respectively.
Pathway Enrichment Analyses
The BINGO plugin of Cytoscape was used to perform Gene Ontology (GO) functional enrichment analyses (Figure 2). Yellow nodes are significantly over-represented, while the white nodes are not significantly over-represented and are included only to show the yellow nodes in reference to the GO hierarchy. The size of a node is proportional to the number of query genes that are annotated to the corresponding GO category. The top 20 GO categories based on their respective node sizes, which are significantly over-represented in our study, are listed in Table 6. Among these significantly over-represented categories, the highest node size was reported for the biological regulation pathway followed by a response to stimulus and signaling. It is interesting to find that 25 genes (ANXA3, BPI, C1QB, CCR1, CD55, CLEC4D, CXCL8, FCGR1A, IL18R1, IL18RAP, IL1B, IL32, LCN2, LILRA6, LTB4R, LY96, PGLYRP1, PRDX2, RAB27A, SH2D1A, TLR1, TLR2, TLR8, TREML1, and TRIM25) were found to be common in top 5 pathways. This shows the importance of these genes in the flow of information in reference to the pathophysiology of the diseases. Of these 25 genes, five genes are common in between hub genes; CXCL8, FCGR1A, IL1B, TLR2, and TLR8.
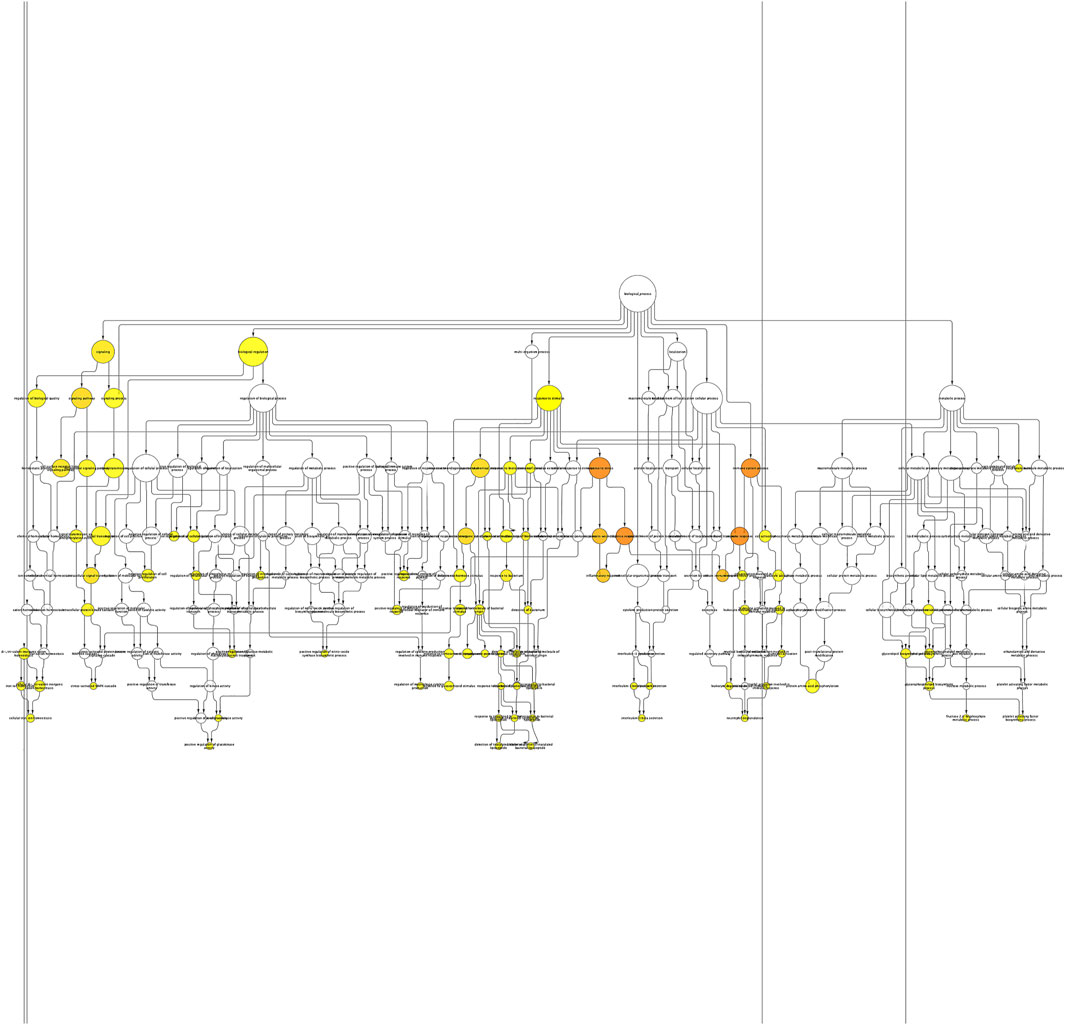
FIGURE 2. Enrichment network of the shared DEGs based on biological processes. Biological process network of differentially expressed genes of KD patients using the BINGO app of Cytoscape. Large nodes indicate more genes involved, and the size of a node is proportional to the number of targets in the GO category. Yellow nodes indicate the genes playing a significant role in KD: p-value < 0.05.
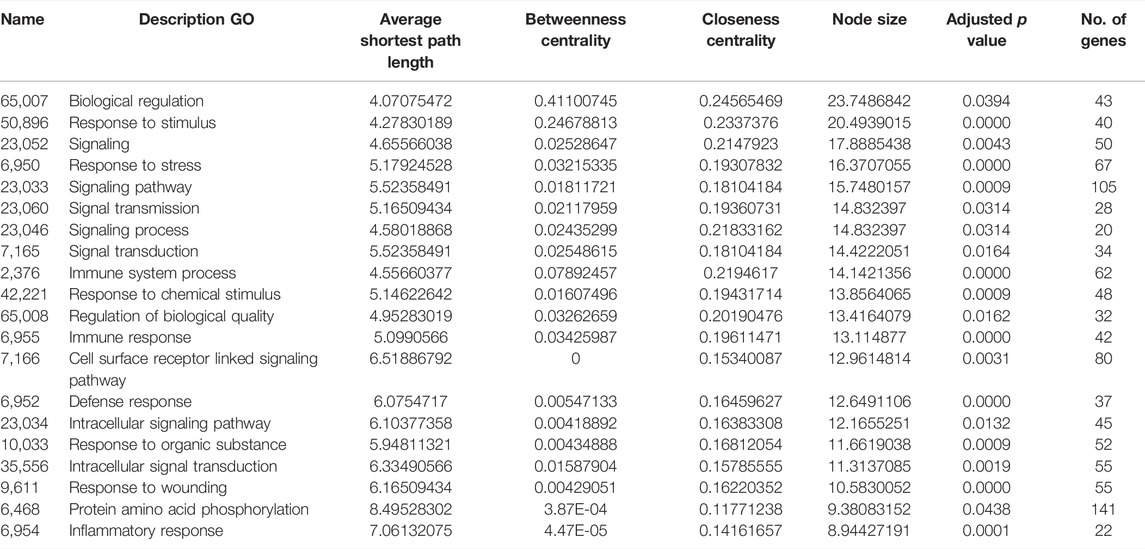
TABLE 6. List of top 20 significantly overrepresented GO categories derived from the BINGO analysis output, based on our data. The list has been arranged in descending order of node size.
Gene Set Overlap for Upregulated Genes
As shown in Figure 3 and Table 7, the analysis identified significantly overrepresented categories (FDR<0.05) of GO molecular function for the upregulated genes. These include “neutrophil degranulation,” “tertiary granule membrane,” and “IgG binding.” Among them, “neutrophil degranulation” and “tertiary granule membrane” were the most significantly enriched biological processes (Figure 3), while most of the proteins encoded by the upregulated genes (number = 50) were found to be located in the “neutrophil degranulation” (Table 7).
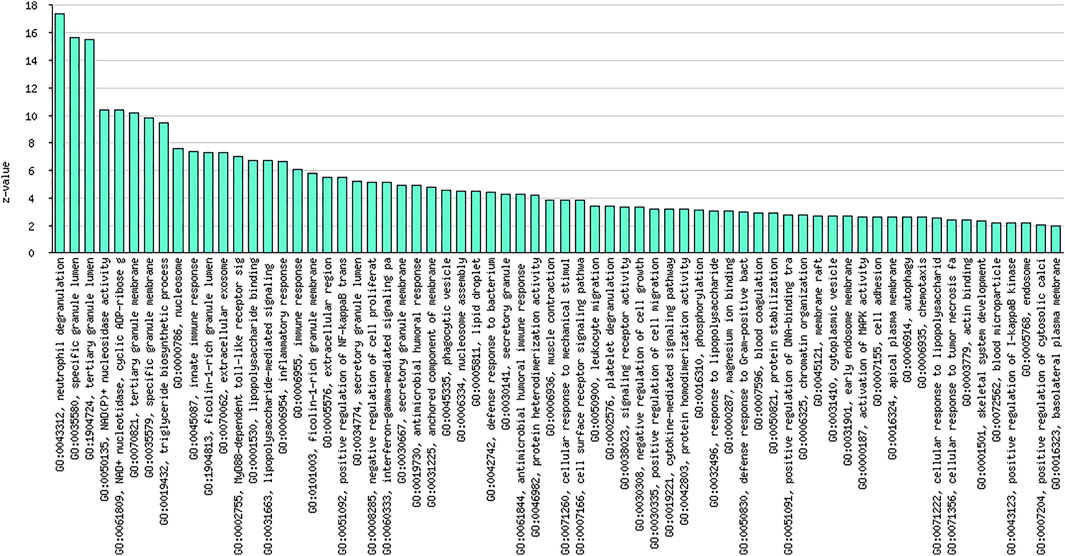
FIGURE 3. Graphical representation of significantly overrepresented individual categories of GO molecular function for the upregulated genes.
Kyoto Encyclopedia of Genes and Genomes Pathway Analysis
KEGG pathway analysis indicated that the majority of the DEGs in KD was enriched in systemic lupus erythematosus (SLE) (Table 8).
Discussion
Despite recent advancements in clinical research, the exact etiology of KD is still poorly understood, and there is no laboratory test for confirmation of diagnosis, especially when the clinical presentation is incomplete or atypical. As a result, early diagnosis of KD may be different to establish. Growing evidence from the immunological, molecular, genetic, and epigenetic studies reveal that epidemiology might differ based on the infectious trigger for KD, especially in some children with a predilection based on genetic factors (Agarwal and Agrawal, 2017; McCrindle et al., 2017; Noval Rivas and Arditi, 2020; Sharma et al., 2021). In such individuals, a putative primary immune response may be activated in mucosal lymphoid tissues. This is then followed by activation of a cytokine cascade resulting in an uncontrolled systemic immune response. Inflammation of medium-sized vessels (especially the coronary arteries) probably results from the extravasation of immune cells in the sub-endothelium. Involvement of coronary arteries results in the development of coronary artery abnormalities (CAAs) (Senzaki, 2006). Therefore, early diagnosis of KD is essential for management decisions in children with KD.
In the last decade, several studies have been conducted to identify the genetic markers for KD; however, a consistent molecular marker having a significant prognostic value has not yet been determined. Furthermore, it is known that despite treatment, approximately 3%–5% of patients with KD would still develop CAAs. Therefore, understanding the pathogenesis of KD is essential for identifying novel pathways that can be targeted for therapy. The current study used an integrated transcriptomic analysis approach to identify DEGs with significant expression change in KD. These molecular biomarkers may be used as a novel multifunctional biomarker panel for diagnosis and therapeutic targets in patients with KD.
Recently, several studies have been conducted on gene expression and genome-wide association in patients with KD. However, there is no consensus on specific genes or biological pathways responsible for the pathogenesis of KD (Popper et al., 2007; Burgner et al., 2009; Onouchi et al., 2012; Xie et al., 2018; Chen et al., 2020; Johnson et al., 2021). The present study aims to pin down specific genes using large-scale datasets on microarray profiles from the GEO database and with the aid of bioinformatics provide key hub genes. Genome-wide expression data in whole-blood samples of KD patients and in controls were obtained using four different GSE datasets with the same array platform, and an integrated bioinformatic analysis was performed. As a result, 126 shared DEGs were screened out and these were mainly enriched in immune and inflammatory responses. The identified 15 hub genes were all significantly upregulated in KD. After careful inspection, we found that five of the 15 hub genes, including ITGAM, MMP9, S100A12, TLR2, and FCER1G, were involved in the top GO item neutrophil degranulation.
The top 10 hub genes from the analyzed data sets in our study were IL-1β, ITGAM, TLR2, CXCL8, SPI1, S100A12, MMP9, PRF1, TLR8, and TREM1. Of these, IL-1β had the highest degree of a node (Lau et al., 2008). IL-1β is a potent proinflammatory cytokine that has been responsible for chronic inflammatory conditions such as cardiovascular disease, coronary artery lesions, and vasculopathy, relevant to the pathogenesis of KD (Lee et al., 2012). Enhanced circulating levels of IL-1β have been reported in KD patients as compared to controls (Hoang et al., 2014; Porritt et al., 2020). Upregulated IL-1β expression is associated with IVIG resistance (Weng et al., 2010; Fu et al., 20192019). Studies have shown that administration of IL-1 receptor antagonist (anakinra) in the KD mice model effectively prevents the development of coronary artery aneurysm, vasculitis, and myocarditis (Lee et al., 2012; Wakita et al., 2016). Based on the genetic and transcriptomic studies and evidence from the mice-model studies, anakinra is undergoing clinical trials (clinicaltrials.gov: NCT02179853) for the treatment of patients with KD.
The KEGG pathway analysis indicated that most DEGs were enriched in SLE and may thus be immune response-associated genes. While overlap of SLE and KD has been occasionally reported in the literature, this is extremely unusual. Both KD and SLE are immune-mediated disorders characterized by distinctive clinical features. However, some clinical findings are common to both conditions. These include fever, lymphadenopathy, arthritis or arthralgia, ocular and mucosal manifestations, rash, and multisystemic involvement. In addition, there appears to be a strong interplay between immunological and genetic factors in the pathogenesis of both KD and SLE (Guleria et al., 2020; Saez-de-Ocariz et al., 2020). ITGAM (Integrin αM) was one such gene with the second highest degree of nodes (44) in our analysis. ITGAM is associated with SLE (Han et al., 2009) and is found to be upregulated in KD patients with vasculopathy (Hom et al., 2008; Reindel et al., 2013). ITGAM, also known as CD11b, belongs to the integrin family and is involved in the regulation of neutrophils, monocyte activation, adhesion, and migration to damaged endothelium associated extracellular matrix. Moreover, studies have shown increased ITGAM levels in peripheral blood and upregulated protein expression in patients with KD (Reindel et al., 2013; Wu et al., 2019).
Immune system activation is one of the major pathogenic mechanisms of KD. These pathways play a crucial role in the innate immune response by recognizing pathogen-associated molecular patterns (PAMPs) of infectious agents. Huang et al. (2009) found that mRNA levels of TLR2 and TLR8 were significantly elevated in patients with KD as compared to controls. Furthermore, after treatment with IVIG therapy, the level of TLR mRNA expression in KD patients was decreased. Studies have shown that augmented expression of TLR2 on CD14+ monocytes is observed in patients with KD and in coronary arteritis mice model, suggesting their role in immunopathogenesis (Rosenkranz et al., 2005; Lin et al., 2012; Mitsui et al., 2014). Therefore, these TLRs may be used as inflammatory biomarkers for the identification of patients with KD.
CXCL2, also known as interleukin-8 (IL8), is a member of the CXC chemokine family, known to possess tumorigenic properties. It is involved in proinflammatory activities, such as neutrophil degranulation, amelioration of adhesion molecule expression on neutrophils’ surfaces and directional migration of neutrophils (Brat et al., 2005). In the acute phase of KD, IL-8 is overexpressed in mononuclear cells and polymorphonuclear neutrophils of patients with KD as compared to controls (Lin et al., 1992; Asano and Ogawa, 2001). Furthermore, Asano et al. showed that IL-8 protein and the neutrophil chemoattractant activity within plasma were increased in the acute phase of KD and were significantly elevated following IVIG therapy (Asano and Ogawa, 2001).
Spi-1 proto-oncogene (SPI1) is a protein-coding gene mainly involved in the activation, differentiation, activation, and migration of macrophages or B cells. This gene was also identified as one of the hub genes in a study by Gao et al. (2021). The role of the SPI1 gene is yet not explored in KD.
Activation of TREM-1 (triggering receptor expressed on myeloid cells-1), trigger receptor is found to be associated with the pathogenesis of KD. Zhao et al. have shown that serum soluble TREM-1 protein concentrations were significantly higher in the acute phase of KD as compared to controls, indicating its involvement in vasculitis and CAAs in patients with KD (Zhao and Wang, 2016). Our results confirm that the expression of the TREM gene was significantly upregulated in patients with KD.
S100 calcium-binding protein A12 (S100A12) belongs to the S100 protein family. Serum SAA00A12 levels were significantly higher in patients with KD, and the levels declined significantly after treatment with IVIG (Foell et al., 2003; Abe et al., 20051950; Armaroli et al., 2019; Lech et al., 2019; Gao et al., 2021). Furthermore, expression of S100A12 increased on the surface of circulating endothelial cells of patients with KD and persisted for a longer duration in KD patients with CAAs, indicating the role of S100A12 in the development of CAAs. In addition, S100A12 stimulates monocytes to produce IL-8, which in turn induces coronary artery endothelial cell dysfunction (Armaroli et al., 2019). Li et al. have shown that IVIG therapy and S100A12 antibody had similar effects on reducing neutrophil infiltration (Lin et al., 1992). Data from these studies highlight the potential of S100A12 as an imperative biomarker for monitoring patients with KD. Despite this, detailed mechanisms through which S100A genes regulate the pathogenesis of KD have not yet been well studied. We hope that our results will enhance our understanding of the role of the S100A gene family in the pathogenesis of KD.
Matrix metalloproteinases-9 (MMP-9) has been implicated in the pathogenesis of several disorders, including tumor metastasis, inflammatory disorders, and atherosclerosis (Sakata et al., 2010). The role of MMP-9 in the pathogenesis of KD has also been highlighted. Overexpression of MMPs induces degradation of the extracellular matrix. Takeshita et al. (2001) hypothesized that in patients with KD, activated neutrophils and monocytes produce MMP-9 in large amounts, that upon migration leads to the breakdown of basement membrane that resulting in vascular damage and coronary artery inflammation. This hypothesis was validated in the mice model by Lau et al. (2008). Studies have shown that serum levels of MMP-9 are elevated in KD patients and were found to play an important role in the development of CAAs (Takeshita et al., 2001; Chua et al., 2003; Gavin et al., 2003; Wu et al., 2019). Perforin-1 (PRF1) is one of the major cytolytic proteins of cytolytic granules. One of the main pathways of lymphocyte-mediated cytolysis entails the secretion onto target membranes of cytolytic granules contained in cytolytic effector lymphocytes of T-cell or NK-cell type (https://omim.org/entry/170280). This gene is related to hemophagocytic lymphohistiocytosis (HLH) but has no reports in KD. We assumed that together all these hub genes may play a key role in the pathogenesis of KD. However, future studies need to be conducted to verify this hypothesis.
Conclusion
The integrated transcriptomic approach, along with the bioinformatic analysis used in the present study, helped to reveal deregulated molecular mechanisms explaining the underlying etiology of KD. Furthermore, using data from more than one microarray dataset and their healthy controls helped eliminate the potential influences of clinical, demographic, and environmental factors on transcriptomic analysis. The 10 hub genes may provide clues to understanding the pathogenesis of KD pathogenesis and could be used to design a biomarker panel to monitor patients with KD.
Data Availability Statement
The datasets presented in this study can be found in online repositories. The names of the repository/repositories and accession number(s) can be found in the article/Supplementary Material.
Author Contributions
PS, CB, and AK: data analysis and preparation of the manuscript. RP, RK, AS, and SS: editing and critical review of the manuscript. All authors approved the final version of the manuscript.
Conflict of Interest
The authors declare that the research was conducted in the absence of any commercial or financial relationships that could be construed as a potential conflict of interest.
Publisher’s Note
All claims expressed in this article are solely those of the authors and do not necessarily represent those of their affiliated organizations, or those of the publisher, the editors, and the reviewers. Any product that may be evaluated in this article, or claim that may be made by its manufacturer, is not guaranteed or endorsed by the publisher.
Supplementary Material
The Supplementary Material for this article can be found online at: https://www.frontiersin.org/articles/10.3389/fgene.2022.849834/full#supplementary-material
References
Abe, J., Jibiki, T., Noma, S., Nakajima, T., Saito, H., and Terai, M. (20051950)., 174. Baltimore, Md, 5837–5845. doi:10.4049/jimmunol.174.9.5837Gene Expression Profiling of the Effect of High-Dose Intravenous Ig in Patients with Kawasaki DiseaseJ. Immunol.9
Agarwal, S., and Agrawal, D. K. (2017). Kawasaki Disease: Etiopathogenesis and Novel Treatment Strategies. Expert Rev. Clin. Immunol. 13 (3), 247–258. doi:10.1080/1744666X.2017.1232165
Armaroli, G., Verweyen, E., Pretzer, C., Kessel, K., Hirono, K., Ichida, F., et al. (2019). Monocyte‐Derived Interleukin‐1β as the Driver of S100A12‐Induced Sterile Inflammatory Activation of Human Coronary Artery Endothelial Cells: Implications for the Pathogenesis of Kawasaki Disease. Arthritis Rheumatol. 71 (5), 792–804. doi:10.1002/art.40784
Asano, T., and Ogawa, S. (2001). Expression of IL-8 in Kawasaki Disease. Clin. Exp. Immunol. 122 (3), 514–519. doi:10.1046/j.1365-2249.2000.01395.x
Banday, A. Z., Mondal, S., Barman, P., Sil, A., Kumrah, R., Vignesh, P., et al. (2021). What Lies Ahead for Young Hearts in the 21st Century - Is it Double Trouble of Acute Rheumatic Fever and Kawasaki Disease in Developing Countries? Front. Cardiovasc. Med. 8, 694393. doi:10.3389/fcvm.2021.694393
Brat, D. J., Bellail, A. C., and Van Meir, E. G. (2005). The Role of Interleukin-8 and its Receptors in Gliomagenesis and Tumoral Angiogenesis. Neuro-oncology 7 (2), 122–133. doi:10.1215/S1152851704001061
Brogan, P., Burns, J. C., Cornish, J., Diwakar, V., Eleftheriou, D., Gordon, J. B., et al. (2020). Lifetime Cardiovascular Management of Patients with Previous Kawasaki Disease. Heart 106, 411–420. doi:10.1136/heartjnl-2019-315925
Burgner, D., Davila, S., Breunis, W. B., Ng, S. B., Li, Y., Bonnard, C., et al. (2009). A Genome-wide Association Study Identifies Novel and Functionally Related Susceptibility Loci for Kawasaki Disease. Plos Genet. 5 (1), e1000319. doi:10.1371/journal.pgen.1000319
Cdc, (2020). About Kawasaki Disease. Available at: https://www.cdc.gov/kawasaki/about.html (Accessed January 1, 2022).
Chen, M.-R., Chang, T.-Y., Chiu, N.-C., Chi, H., Yang, K. D., Chang, L., et al. (2020). Validation of Genome-wide Associated Variants for Kawasaki Disease in a Taiwanese Case-Control Sample. Sci. Rep. 10 (1), 11756. doi:10.1038/s41598-020-68673-0
Chua, P. K., Melish, M. E., Yu, Q., Yanagihara, R., Yamamoto, K. S., and Nerurkar, V. R. (2003). Elevated Levels of Matrix Metalloproteinase 9 and Tissue Inhibitor of Metalloproteinase 1 during the Acute Phase of Kawasaki Disease. Clin. Vaccin. Immunol 10 (2), 308–314. doi:10.1128/cdli.10.2.308-314.2003
Dietz, S. M., van Stijn, D., Burgner, D., Levin, M., Kuipers, I. M., Hutten, B. A., et al. (2017). Dissecting Kawasaki Disease: a State-Of-The-Art Review. Eur. J. Pediatr. 176 (8), 995–1009. doi:10.1007/s00431-017-2937-5
Edgar, R., Domrachev, M., and Lash, A. E. (2002). Gene Expression Omnibus: NCBI Gene Expression and Hybridization Array Data Repository. Nucleic Acids Res. 30 (1), 207–210. doi:10.1093/nar/30.1.207
Foell, D., Ichida, F., Vogl, T., Yu, X., Chen, R., Miyawaki, T., et al. (2003). S100A12 (EN-RAGE) in Monitoring Kawasaki Disease. The Lancet 361 (9365), 1270–1272. doi:10.1016/S0140-6736(03)12986-8
Fu, L. Y., Qiu, X., Deng, Q. L., Huang, P., Pi, L., Xu, Y., et al. (20192019). The IL-1B Gene Polymorphisms Rs16944 and Rs1143627 Contribute to an Increased Risk of Coronary Artery Lesions in Southern Chinese Children with Kawasaki Disease. J. Immunol. Res. 2019, 1–7. doi:10.1155/2019/4730507
Gao, S., Ma, W., Lin, X., Huang, S., and Yu, M. (2021). Identification of Key Genes and Underlying Mechanisms in Acute Kawasaki Disease Based on Bioinformatics Analysis. Med. Sci. Monit. 27, e930547. doi:10.12659/MSM.930547
Gavin, P. J., Crawford, S. E., Shulman, S. T., Garcia, F. L., and Rowley, A. H. (2003). Systemic Arterial Expression of Matrix Metalloproteinases 2 and 9 in Acute Kawasaki Disease. Atvb 23 (4), 576–581. doi:10.1161/01.atv.0000065385.47152.fd
Guleria, S., Jindal, A., Pilania, R., Bhattarai, D., Kaur, A., Naganur, S., et al. (2020). An Uncommon Overlap of Two Common Rheumatological Disorders. Lupus 29 (9), 1121–1125. doi:10.1177/0961203320930101
Han, S., Kim-Howard, X., Deshmukh, H., Kamatani, Y., Viswanathan, P., Guthridge, J. M., et al. (2009). Evaluation of Imputation-Based Association in and Around the Integrin- -M (ITGAM) Gene and Replication of Robust Association between a Non-synonymous Functional Variant within ITGAM and Systemic Lupus Erythematosus (SLE). Hum. Mol. Genet. 18 (6), 1171–1180. doi:10.1093/hmg/ddp007
Hoang, L. T., Shimizu, C., Ling, L., Naim, A. N. M., Khor, C. C., Tremoulet, A. H., et al. (2014). Global Gene Expression Profiling Identifies New Therapeutic Targets in Acute Kawasaki Disease. Genome Med. 6 (11), 541. doi:10.1186/s13073-014-0102-6
Hom, G., Graham, R. R., Modrek, B., Taylor, K. E., Ortmann, W., Garnier, S., et al. (2008). Association of Systemic Lupus Erythematosus withC8orf13-BLKandITGAM-ITGAX. N. Engl. J. Med. 358 (9), 900–909. doi:10.1056/NEJMoa0707865
Huang, D. W., Sherman, B. T., and Lempicki, R. A. (2009). Systematic and Integrative Analysis of Large Gene Lists Using DAVID Bioinformatics Resources. Nat. Protoc. 4 (1), 44–57. doi:10.1038/nprot.2008.211
Jindal, A. K., Pilania, R. K., Prithvi, A., Guleria, S., and Singh, S. (2019). Kawasaki Disease: Characteristics, Diagnosis, and Unusual Presentations. Expert Rev. Clin. Immunol. 15 (10), 1089–1104. doi:10.1080/1744666X.2019.1659726
Johnson, T. A., Mashimo, Y., Wu, J.-Y., Yoon, D., Hata, A., Kubo, M., et al. (2021). Association of an IGHV3-66 Gene Variant with Kawasaki Disease. J. Hum. Genet. 66, 475–489. doi:10.1038/s10038-020-00864-z2
Kanehisa, M., and Goto, S. (2000). KEGG: Kyoto Encyclopedia of Genes and Genomes. Nucleic Acids Res. 28 (1), 27–30. doi:10.1093/nar/28.1.27
Lau, A. C., Duong, T. T., Ito, S., and Yeung, R. S. M. (2008). Matrix Metalloproteinase 9 Activity Leads to Elastin Breakdown in an Animal Model of Kawasaki Disease. Arthritis Rheum. 58 (3), 854–863. doi:10.1002/art.23225
Lech, M., Guess, J., Duffner, J., Oyamada, J., Shimizu, C., Hoshino, S., et al. (2019). Circulating Markers of Inflammation Persist in Children and Adults with Giant Aneurysms after Kawasaki Disease. Circ. Genomic Precision Med. 12 (4), e002433. doi:10.1161/CIRCGEN.118.002433
Lee, Y., Schulte, D. J., Shimada, K., Chen, S., Crother, T. R., Chiba, N., et al. (2012). Interleukin-1β Is Crucial for the Induction of Coronary Artery Inflammation in a Mouse Model of Kawasaki Disease. Circulation 125 (12), 1542–1550. doi:10.1161/CIRCULATIONAHA.111.072769
Lin, C.-Y., Lin, C.-C., Hwang, B., and Chiang, B. (1992). Serial Changes of Serum Interleukin-6, Interleukin-8, and Tumor Necrosis Factor Alpha Among Patients with Kawasaki Disease. J. Pediatr. 121 (6), 924–926. doi:10.1016/s0022-3476(05)80343-9
Lin, I.-C., Kuo, H.-C., Lin, Y.-J., Wang, F.-S., Wang, L., Huang, S.-C., et al. (2012). Augmented TLR2 Expression on Monocytes in Both Human Kawasaki Disease and a Mouse Model of Coronary Arteritis. PloS one 7 (6), e38635. doi:10.1371/journal.pone.0038635
Maere, S., Heymans, K., and Kuiper, M. (2005). BiNGO: a Cytoscape Plugin to Assess Overrepresentation of Gene Ontology Categories in Biological Networks. Bioinformatics 21 (16), 3448–3449. doi:10.1093/bioinformatics/bti551
McCrindle, B. W., Rowley, A. H., Newburger, J. W., Burns, J. C., Bolger, A. F., Gewitz, M., et al. (2017). Diagnosis, Treatment, and Long-Term Management of Kawasaki Disease: a Scientific Statement for Health Professionals from the American Heart Association. Circulation 135, e927–e999. doi:10.1161/CIR.0000000000000484
Mitsui, K., Yusa, T., Miyazaki, S., Ohara, A., and Saji, T. (2014). Increased TLR2 and TLR4 Expression in Peripheral Neutrophils Isolated from Kawasaki Disease. Pediatr. Allergy Immunol. Pulmonology 27 (1), 24–29. doi:10.1089/ped.2013.0315
Nakamura, A., Ikeda, K., and Hamaoka, K. (2019). Aetiological Significance of Infectious Stimuli in Kawasaki Disease. Front. Pediatr. 7, 244. doi:10.3389/fped.2019.00244
Newburger, J. W., Takahashi, M., Gerber, M. A., Gewitz, M. H., Tani, L. Y., Burns, J. C., et al. (2004). Diagnosis, Treatment, and Long-Term Management of Kawasaki Disease. Circulation 110 (17), 2747–2771. doi:10.1161/01.CIR.0000145143.19711.78
Noval Rivas, M., and Arditi, M. (2020). Kawasaki Disease: Pathophysiology and Insights from Mouse Models. Nat. Rev. Rheumatol. 16 (7), 391–405. doi:10.1038/s41584-020-0426-0
Oates-Whitehead, R. M., Baumer, J. H., Haines, L., Love, S., Maconochie, I. K., Gupta, A., et al. (2003). Intravenous Immunoglobulin for the Treatment of Kawasaki Disease in Children. Cochrane database Syst. Rev. 2021 (4), CD004000. doi:10.1002/14651858.CD004000
Ogata, S., Shimizu, C., Franco, A., Touma, R., Kanegaye, J. T., Choudhury, B. P., et al. (2013). Treatment Response in Kawasaki Disease Is Associated with Sialylation Levels of Endogenous but Not Therapeutic Intravenous Immunoglobulin G. PLoS ONE 8, e81448. doi:10.1371/journal.pone.0081448
Onouchi, Y., Ozaki, K., Ozaki, K., Burns, J. C., Shimizu, C., Terai, M., et al. (2012). A Genome-wide Association Study Identifies Three New Risk Loci for Kawasaki Disease. Nat. Genet. 44 (5), 517–521. doi:10.1038/ng.2220
Popper, S. J., Shimizu, C., Shike, H., Kanegaye, J. T., Newburger, J. W., Sundel, R. P., et al. (2007). Gene-expression Patterns Reveal Underlying Biological Processes in Kawasaki Disease. Genome Biol. 8 (12), R261. doi:10.1186/gb-2007-8-12-r261
Porritt, R. A., Markman, J. L., Maruyama, D., Kocaturk, B., Chen, S., Lehman, T. J. A., et al. (2020). Interleukin-1 Beta-Mediated Sex Differences in Kawasaki Disease Vasculitis Development and Response to Treatment. Atvb 40 (3), 802–818. doi:10.1161/ATVBAHA.119.313863
Rahmati, Y., Mollanoori, H., Kakavandi, N., Nateghian, A., Sayyahfar, S., Babaei, V., et al. (2020). Gene Expression Analysis in Kawasaki Disease; Bioinformatics and Experimental Approach. Inform. Med. Unlocked 20, 100423. doi:10.1016/j.imu.2020.100423
Ramphul, K., and Mejias, S. G. (2018). Kawasaki Disease: a Comprehensive Review. amsad 3, 41–45. doi:10.5114/amsad.2018.74522
Reindel, R., Baker, S. C., Kim, K.-Y., Rowley, C. A., Shulman, S. T., Orenstein, J. M., et al. (2013). Integrins α4 and αM, collagen1A1, and Matrix Metalloproteinase 7 Are Upregulated in Acute Kawasaki Disease Vasculopathy. Pediatr. Res. 73 (3), 332–336. doi:10.1038/pr.2012.185
Rosenkranz, M. E., Schulte, D. J., Agle, L. M. A., Wong, M. H., Zhang, W., Ivashkiv, L., et al. (2005). TLR2 and MyD88 Contribute to Lactobacillus Casei Extract-Induced Focal Coronary Arteritis in a Mouse Model of Kawasaki Disease. Circulation 112 (19), 2966–2973. doi:10.1161/CIRCULATIONAHA.105.537530
Saez-de-Ocariz, M., Pecero-Hidalgo, M. J., Rivas-Larrauri, F., García-Domínguez, M., Venegas-Montoya, E., Garrido-García, M., et al. (2020). A Teenager with Rash and Fever: Juvenile Systemic Lupus Erythematosus or Kawasaki Disease? Front. Pediatr. 8, 149. doi:10.3389/fped.2020.00149
Sakata, K., Hamaoka, K., Ozawa, S., Niboshi, A., Yahata, T., Fujii, M., et al. (2010). Matrix Metalloproteinase-9 in Vascular Lesions and Endothelial Regulation in Kawasaki Disease. Circ. J. 74 (8), 1670–1675. doi:10.1253/circj.cj-09-0980
Senzaki, H. (2006). The Pathophysiology of Coronary Artery Aneurysms in Kawasaki Disease: Role of Matrix Metalloproteinases. Arch. Dis. Child. 91 (10), 847–851. doi:10.1136/adc.2005.087437
Sharma, K., Vignesh, P., Srivastava, P., Sharma, J., Chaudhary, H., Mondal, S., et al. (2021). Epigenetics in Kawasaki Disease. Front. Pediatr. 9, 673294. doi:10.3389/fped.2021.673294
Sharov, A. A., Schlessinger, D., and Ko, M. S. H. (2015). ExAtlas: An Interactive Online Tool for Meta-Analysis of Gene Expression Data. J. Bioinform. Comput. Biol. 13 (6), 1550019. doi:10.1142/S0219720015500195
Singh, S., Bhattad, S., Gupta, A., Suri, D., Rawat, A., and Rohit, M. (2016). Mortality in Children with Kawasaki Disease: 20 Years of Experience from a Tertiary Care centre in North India. Clin. Exp. Rheumatol. 34 (3 Suppl. 97), S129–S133.
Singh, S., Vignesh, P., and Burgner, D. (2015). The Epidemiology of Kawasaki Disease: A Global Update. Arch. Dis. Child. 100, 1084–1088. doi:10.1136/archdischild-2014-307536
Skochko, S. M., Jain, S., Sun, X., Sivilay, N., Kanegaye, J. T., Pancheri, J., et al. (2018). Kawasaki Disease Outcomes and Response to Therapy in a Multiethnic Community: A 10-Year Experience. J. Pediatr. 203, 408–415. doi:10.1016/j.jpeds.2018.07.090
Takeshita, S., Tokutomi, T., Kawase, H., Nakatani, K., Tsujimoto, H., Kawamura, Y., et al. (2001). Elevated Serum Levels of Matrix Metalloproteinase-9 (MMP-9) in Kawasaki Disease. Clin. Exp. Immunol. 125 (2), 340–344. doi:10.1046/j.1365-2249.2001.01608.x
Vallabhajosyula, R. R., Chakravarti, D., Lutfeali, S., Ray, A., and Raval, A. (2009). Identifying Hubs in Protein Interaction Networks. PloS one 4 (4), e5344. doi:10.1371/journal.pone.0005344
Wakita, D., Kurashima, Y., Crother, T. R., Noval Rivas, M., Lee, Y., Chen, S., et al. (2016). Role of Interleukin-1 Signaling in a Mouse Model of Kawasaki Disease-Associated Abdominal Aortic Aneurysm. Atvb 36 (5), 886–897. doi:10.1161/ATVBAHA.115.307072
Weng, K.-P., Hsieh, K.-S., Ho, T.-Y., Huang, S.-H., Lai, C.-R., Chiu, Y.-T., et al. (2010). IL-1B Polymorphism in Association with Initial Intravenous Immunoglobulin Treatment Failure in Taiwanese Children with Kawasaki Disease. Circ. J. 74 (3), 544–551. doi:10.1253/circj.cj-09-0664
Wu, W., You, K., Zhong, J., Ruan, Z., and Wang, B. (2019). Identification of Potential Core Genes in Kawasaki Disease Using Bioinformatics Analysis. J. Int. Med. Res. 47 (9), 4051–4058. doi:10.1177/0300060519862057
Xie, X., Shi, X., and Liu, M. (2018). The Roles of Genetic Factors in Kawasaki Disease: A Systematic Review and Meta-Analysis of Genetic Association Studies. Pediatr. Cardiol. 39 (2), 207–225. doi:10.1007/s00246-017-1760-0
Zhao, J. M., and Wang, Q. (2016). Zhongguo Dang Dai Er Ke Za Zhi = Chinese Journal of Contemporary Pediatrics 18 (6), 522–526. doi:10.7499/j.issn.1008-8830.2016.06.011
Keywords: bioinformatics, biomarkers, hub genes, in-silico analysis, Kawasaki disease, microarray, transcriptomics analysis
Citation: Srivastava P, Bamba C, Pilania RK, Kumari A, Kumrah R, Sil A and Singh S (2022) Exploration of Potential Biomarker Genes and Pathways in Kawasaki Disease: An Integrated in-Silico Approach. Front. Genet. 13:849834. doi: 10.3389/fgene.2022.849834
Received: 06 January 2022; Accepted: 11 April 2022;
Published: 09 May 2022.
Edited by:
Sajad Ahmad Dar, Jazan University, Saudi ArabiaReviewed by:
Arshad Jawed, GE Healthcare Life Sciences, IndiaMohd Wahid, Jazan University, Saudi Arabia
Copyright © 2022 Srivastava, Bamba, Pilania, Kumari, Kumrah, Sil and Singh. This is an open-access article distributed under the terms of the Creative Commons Attribution License (CC BY). The use, distribution or reproduction in other forums is permitted, provided the original author(s) and the copyright owner(s) are credited and that the original publication in this journal is cited, in accordance with accepted academic practice. No use, distribution or reproduction is permitted which does not comply with these terms.
*Correspondence: Priyanka Srivastava, c3JpdmFzdGF2YXByaXlAZ21haWwuY29t