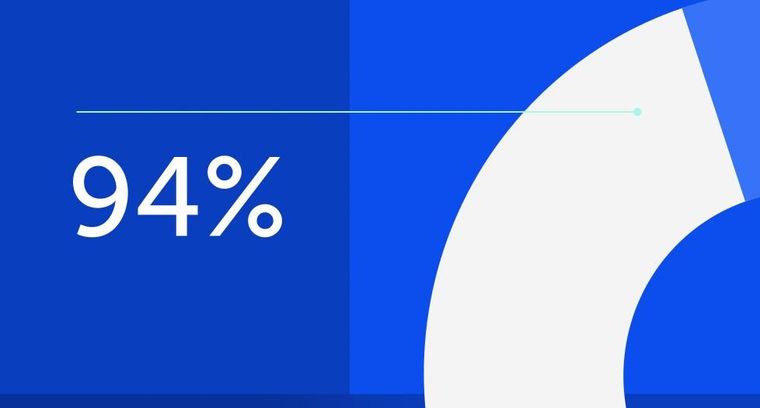
94% of researchers rate our articles as excellent or good
Learn more about the work of our research integrity team to safeguard the quality of each article we publish.
Find out more
ORIGINAL RESEARCH article
Front. Genet., 16 May 2022
Sec. Livestock Genomics
Volume 13 - 2022 | https://doi.org/10.3389/fgene.2022.849399
This article is part of the Research TopicTraditional and Up-to-date Genomic Insights into Domestic Animal DiversityView all 19 articles
There is an increasing understanding of the possible regulatory role of long non-coding RNAs (LncRNA). Studies on livestock have mainly focused on the regulation of cell differentiation, fat synthesis, and embryonic development. However, there has been little study of skeletal muscle of domestic animals and the potential role of lncRNA. In this study, the transcriptome numbers of longissimus muscle of different beef cattle (Shandong black catle and Luxi catlle) were used to construct muscle related lncRNAs-miRNA-mRNA interaction network through bioinformatics analysis. This is helpful to clarify the molecular mechanism of bovine muscle development, and can be used to promote animal husbandry and improve animal husbandry production. According to the screening criteria of |FC|≧2 and q < 0.05, a total of 1,415 transcripts (of which 480 were LncRNAs) were differentially expressed (q < 0.05) in the different breeds. Further, we found that the most differentially expressed LncRNAs were found on chromosome 9, in which the differentially expressed LncRNAs targeted 1,164 protein coding genes (MYORG, Wnt4, PAK1, ADCY7,etc) (upstream and downstream<50 Kb). In addition, Pearson’s correlation coefficients of co-expression levels indicated a potential trans regulatory relationship between the differentially expressed LncRNAs and 43844 mRNAs (r > 0.9). The identified co-expressed mRNAs (MYORG, Dll1, EFNB2, SOX6, MYOCD, and MYLK3) are related to the formation of muscle structure, and enriched in muscle system process, strained muscle cell differentiation, muscle cell development, striated muscle tissue development, calcium signaling, and AMPK signaling. Additionally, we also found that some LncRNAs (LOC112444238, LOC101903367, LOC104975788, LOC112441863, LOC112449549, and LOC101907194) may interact with miRNAs related to cattle muscle growth and development. Based on this, we constructed a LncRNAs-miRNA-mRNA interaction network as the putative basis for biological regulation in cattle skeletal muscle. Interestingly, a candidate differential LncRNA (LOC104975788) and a protein-coding gene (Pax7) contain miR-133a binding sites and binding was confirmed by luciferase reporter assay. LOC104975788 may combined miR-133a competitively with Pax7, thus relieving the inhibitory effect of miR-133a on Pax7 to regulate skeletal muscle development. These results will provide the theoretical basis for further study of LncRNA regulation and activity in different cattle breeds.
As an important economic trait that affects the production efficiency of beef cattle, meat production traits are a research focus in the field of beef cattle genetics and breeding. The analysis of the molecular regulation mechanisms of muscle growth can facilitate cattle breeding. Local cattle breeds in China typically present reduced growth, but increased meat quality when compared to imported cattle. These differences may be related to differences in skeletal muscle development among different breeds (Xu et al., 2018). The growth and development of beef cattle skeletal muscle can be affected by a variety of regulatory factors. Previous work mainly focused on contributions from DNA, mRNA, and miRNA, but regulatory effects of long non-coding RNA (LncRNA) on the growth and development of beef skeletal muscle remain poorly understood (Zhang et al., 2007; Dylan et al., 2008; Xu et al., 2020).
Previous studies reported contributions of LncRNA in the process of skeletal muscle proliferation and differentiation. Liu identified, mapped, and determined the global and skeletal muscle expression patterns of 7,188 LncRNAs, finding that these LncRNAs had similar open reading frames and expression levels as those of other mammalian LncRNAs (Sun et al., 2016; Yu-ying et al., 2017). Subsequently, Yue identified a highly expressed LncRNA-YYW in muscle tissue (Yue et al., 2017). Microarray analysis showed that LncRNA-YYW positively regulated the expression of growth hormone-1 and its downstream genes AKT1 and PIK3CD in bovine myoblasts, and promoted myoblast proliferation (Dylan et al., 2008; Billerey et al., 2014). Cai found that lnc-ORA interacted with IGF2BP2 to inhibit the PI3K/AKT signaling pathway, thereby inhibiting muscle production and inducing skeletal muscle atrophy (Cai et al., 2021). Studies had shown that LncMD could be used as a competitive endogenous RNA (ceRNA) to compete with IGF2 and bound miR-125b to weaken its inhibitory effect on IGF2, and promoted the differentiation of bovine skeletal muscle satellite cells (Sun et al., 2016). Linc-MD1 had been shown to be a competitive endogenous RNA for miR-133 and miR-135 targets during myoblast differentiation. These transcription factors activated muscle-specific gene expression to control the time of muscle differentiation (Marcella Davide et al., 2011). Lnc-31 maintained the proliferation of myoblasts and counteracts differentiation (Dacia et al., 2018). The active regulation of Rock1 translation by lnc-31 was of great significance to the control of myogenesis (Ballarino et al., 2015). These results suggest that LncRNA may regulate the growth and development of beef skeletal muscle and meat quality. Although LncRNAs lack coding capacity, many LncRNAs act in various biological processes, serving as an additional level of genomic regulation.
The first embryo transfer calf in China was obtained from a vitrified frozen somatic cell cloned embryo, which combined Japan black cattle, Luxi cattle, and Bohai black cattle. The new generation core breeding group of this new breed, Shandong black cattle, was established by the filial generation. The new generation core breeding group hybridized with Shandong black cattle, and the second generation group of Japan black cattle (3/4) was crossed with Luxi cattle (1/4) to produce the new generation (3/4) × Bohai black cattle (1/4). Ideal black cattle and Shandong black cattle were selected from the second-generation group for cross-cross fixation. In this stage, several good lines were cross-bred with distant relatives, and then selected and the excellent lines were preserved. After four generations, this line was finally bred into a new Shandong black cattle variety matching line and used as a bull. In 2015, the Shandong black cattle variety was approved by the National Animal and Poultry Genetic Resources Committee as a new population and successfully established as a new cultivated variety in China (Lu, 2015; Liu et al., 2018).
In recent years, there has been growing research of LncRNA, but most work has mainly focused on the effects of neurologic diseases, tumors, embryonic development, and cell differentiation in human and mouse, with little research on domestic animals. The growth and development of beef cattle skeletal muscle are highly regulated processes. Although the contributions of DNA, mRNA, and miRNA to this regulation have been studied, the extent to which LncRNA regulates beef skeletal muscle growth and development remains poorly understood. To investigate the potential contribution of LncRNA, RNA samples were prepared from longissimus dorsi muscle tissues of Shandong black cattle (hybrid offspring) and Luxi Cattle (the first maternal generation). RNA-seq technology was used to identify LncRNA transcripts and their genomic characteristics, and LncRNAs differentially expressed in skeletal muscle tissues in Shandong black cattle and Luxi cattle were determined. Using these data, we constructed an interaction network of LncRNA-miRNA-mRNA and muscle development which can guide future breeding efforts.
The methods used in this study were performed in accordance with the guidelines of Good Experimental Practices adopted by the Institute of Animal Science (Qingdao Agricultural University, Qingdao, China). All surgical procedures involving cattle were performed according to the approved protocols of the Biological Studies Animal Care and Use Committee, Shandong Province, China (Cao et al., 2014).
Shandong black cattle(three)and Luxi cattle(three)were used in this study. Cattle were fed three times and received approximately equal amounts of green coarse feed and concentrate feed everyday according to standard NY5127—2002 pollution-free feeding management of beef cattle. They were raised in the same environment. At the age of 18 months, three healthy male beef cattle in each group were randomly selected for this study. The selected cattle had no scratches, scars, and scabs on their bodies, with no fat deposits in the internal organs or abdomen. No disease was found during examination, and all physiological and biochemical indexes were normal. Longissimus dorsi muscle samples were collected and immediately frozen in liquid nitrogen for RNA extraction. The cattle used in the experiment were euthanized as follows: first, a 1–3% sodium pentobarbital solution was prepared with physiological saline, and then intravenously injected. The injection dose is 90–135 mg/kg.
In order to better observe the histological morphology of muscle, we performed HE staining and fast/slow muscle staining. Paraffin sections were prepared from muscle tissue fixed with 4% paraformaldehyde. The HE staining protocol was performed as described previously (Liu et al., 2020). Briefly, dewaxing, covering with water, Hematoxylin staining, washing with water, 5% acetic acid differentiation, eosin staining, dehydration, natural drying, sealing, and image acquisition were performed.
Tissue sections were placed in a box filled with EDTA antigen repair buffer (Purchased from Shanghai Beyotime Biotechnology Co., Ltd) (ph 8.0) for antigen repair. After natural cooling, the slides were washed in PBS (pH7.4) with three ashes of 5 minutes each. BSA was added for blocking, and then the antibody was added, followed by DAPI to stain the nucleus. An autofluorescence quenching agent was added to the slices for 5 min and the samples were then washed with running water for 10 min. After natural drying, the film was sealed with an anti-fluorescence quenching sealing agent. Finally, the slices were observed under the fluorescence microscope and images were collected.
Image-Pro Plus software was used to count the images and measure the surface area. SPSS software was used for statistical analysis to determine significant differences.
Total RNA samples were isolated using TRIzol reagent (Invitrogen, Carlsbad, CA, United States) according to the manufacturer’s instructions. RNA degradation and contamination were monitored on 1% agarose gels. RNA purity was checked using a NanoPhotometer® spectrophotometer (IMPLEN, Los Angeles, CA, United States). RNA concentration was measured using a Qubit® RNA Assay Kit in a Qubit® 2.0 Fluorometer (Life Technologies, Carlsbad, CA, United States). RNA integrity was assessed using a RNA Nano 6000 Assay Kit in a Bioanalyzer 2,100 system (Agilent Technologies, Santa Clara, CA, United States). Only samples with RNA Integrity Number (RIN) scores >8 were used for sequencing, which is different from the previous studies (Liu et al., 2020). The different RIN values are due to the different requirements for RNA quality when constructing different data sets. A total of 3 μg RNA per sample was used as the input material for RNA library preparation. This study used Illumina hiseq xten sequencing platform.
The original sequencing data were processed by Fastp software (v0.23.2) (Chen et al., 2018) with parameters “-Q 20 -P90” with disjointing sequence and low-quality sequence. Clean data were obtained by removing reads containing adapters, reads containing over 10% of poly(N), and low-quality reads (>50% of the bases had Phred quality scores ≤10) from the raw data. All downstream analyses were based on the high quality clean data. The Bos taurus genome reference genome and gene model annotation files were downloaded from the NCBI database (CHIR_1.0, NCBI) (Mahmoudi et al., 2020). An index of the reference genome was built using Bowtie v2.0.6 (Cai et al., 2015; Cai et al., 2018) and paired-end clean reads were aligned to the reference genome using HISAT v2-2.1.0 (Yuan et al., 2019). The mapped reads from each library were assembled with Cufflinks v2.2.1 (Andersson, 2009), using Cufflinks with ‘min-frags-per-transfrag = 0’ and’–library-typefr-firststrand’, and other parameters set as default.
Stringtie (Pertea et al., 2015; Kovaka et al., 2019) was used to sort reads into different classes and then generate a map for each class. Based on the length of LncRNA, and the characteristics of non-protein coding sequences, we established strict screening conditions to screen LncRNA as follows:
(1) Transcripts equal to or longer than 200 bp in length, and containing two or more exons;
(2) Transcript read coverage of at least five reads;
(3) No transcripts of known mRNA or other specific non-coding RNAs (rRNA, tRNA, snoRNA, or snRNA). This screening was based on gffcompare (http://ccb.jhu.edu/software/stringtie/gff.shtml) using the same species annotation file;
(4) According to the class in the comparison result Code information (“U”, “I”, “X”) was used to screen potential lincrna and intronic LncRNA anti-sense LncRNA Coding potential prediction screening:
To further assess if the identified transcripts are LncRNAs, a variety of coding potential analysis software was integrated, including CNCI analysis, CPC analysis, Pfam protein domain analysis, and CPAT analysis (only for animals) (Langfelder et al., 2008; Jing et al., 2010; Luo et al., 2017; Ito et al., 2018). The transcripts identified as non-coding by several methods were the final potential LncRNA dataset.
Comparison of raw counts data (Wang et al., 2010) for different genes is a very effective tool for quantitative estimation of gene expression based on RNA-Seq data. This method can eliminate the influence of gene length and sequencing quantity on the determination of gene expression levels. The calculated gene expression values allow direct comparison of gene expression differences among different varieties. Here, we used DEseq (Anders and Huber, 2010) to analyze the differential expression between the treatment group with the reference group, and selected |Log2ratio| ≥2 and q < 0.05 genes as indicative of significant differential expression. The numbers of up-regulated and down-regulated genes were also obtained.
LncRNA can act on target genes by Cis or Trans. Cis effect refers to LncRNA acting on adjacent target genes (Cabili et al., 2011). To predict the cis-regulatory genes of LncRNA, we screened the 50 kb of sequence upstream and downstream of LncRNA and looked for potential target genes. The coexpression relationship between LncRNA and mRNA was described by Trans. The basic principle of transaction is that the function of LncRNA has nothing to do with the position of the coding gene,but it is related to the protein-coding genes it coexpressed. According to the correlation coefficient between LncRNA and mRNA expression (correlation coefficient cor ≥ 0.9); WGCNA(Weighted correlation network analysis) was used to predict the target genes of lncRNA for cluster analysis and functional enrichment analysis of LncRNA target genes. p-value < 0.05 was set as the significance threshold.
GO (gene ontology) (Young et al., 2010) can be used for enrichment analysis of target genes with differential expression and Goseq (Young et al., 2010) was used to analyze the target genes of differentially expressed LncRNA. A value of p < 0.05 is considered as significant enrichment of differentially expressed genes.
The KEGG database (Kanehisa and Goto, 2000) describes advanced functions and utility of biological systems such as cells, organisms, and ecosystems (http://www.genome.jp/kegg/). We used KOBAS V3.0 software (Bu et al., 2021) to detect the enrichment of LncRNA target genes differentially expressed in the KEGG pathway.
As competing endogenous RNAs (ceRNA), LncRNA can act as a sponge to adsorb miRNA and affect mRNA expression. Therefore, to further understand the function of LncRNA in the growth and development of skeletal muscle, we used TargetScan (http://www.targetscan.org/vert_71/) miRanda ((http://www.microrna.org/microrna/home.do) and PITA qtar (https://genie.weizmann.ac.il/pubs/mir07/mir07_dyn_data.html) software to predict the differentially expressed miRNAs(Sequencing has been completed) adsorbed by LncRNA and downstream-regulated mRNA. Online miRNA binding site prediction software (RNA22: http://cbcsrv.watson.ibm.com/rna22.html and RNAhybird ()) predicted potential interaction of miRNA with LncRNA. We then constructed an LncRNA-miRNA-mRNA interaction network map using Cytoscape version 3.5.1 (Shannon et al., 2003).
Cells were seeded in 96-well plates at a density of 5 × 103 cells (HEK-293T) per well, 24 h before transfection. The cells were co-transfected with a mixture of 50 ng Firefly luciferase (FL) reporter vectors, 5 ng Renilla luciferase (RL) reporter vectors (pRL-TK), and miRNA mimics at the indicated concentration. The miRNA mimics were obtained from Life Technologies. After 48 h, the luciferase activity was measured with a dual luciferase reporter assay system using the psiCHECK-2 vector (Promega, Madison, WI). The LOC104975788 and LOC536229 (Pax7) sequences were separately cloned into the reporter gene vector (psiCHECK-2) to synthesize the predicted miRNA mimics and control. The potential binding target of each miRNA was cloned into the 3′UTR region of r-luciferase (hrluc), and then co-transfected with the miRNA to determine the activity of R-Luciferase. F-Luciferase (hluc +) was used as an internal reference to correct for differences in transfection efficiency between different samples. The miRNA mimics and psicheck-LOC104975788 or psicheck-LOC536229 (Pax7) were co-transfected into 293T cells. The expression level of reporter genes was detected using a multi-functional enzyme labeling instrument, and the miRNAs that exhibited down-regulated reporter gene expression were further screened.
The reaction system (20 μl) for the RT-PCR reaction consisted of the following: 1 μl of template cDNA, 10 μl each of the upstream and downstream primers, and 5 ml (5 × 1 ml vials) of RNase-free water. The thermal cycling procedure was as follows: 94°C for 10 min, 94°C for 30 s, 60°C for 30 s, and 72°C for 40 s, with 40 cycles12. The expression of GAPDH was calculated by the 2-△△CT method. Primers used for qRT-PCR as shown in Supplementary Table S1.
To investigate the potential regulatory effects of LncRNA on the growth and development of beef skeletal muscle, we analyzed samples from muscles of different breeds of beef cattle.
First, we obtained samples from Shandong black cattle and Luxi cattle and performed fluorescence staining sections of fast and slow muscle fibers (Figure 1A) and HE staining (Figure 1B). The results showed significant differences in muscle fibers of longissimus dorsi muscles between Shandong black cattle and Luxi cattle. IPP(Image-Pro Plus) software analysis showed that the average area of muscle fibres of Shandong black cattle was significantly larger than that of Luxi cattle (p < 0.05), with significant differences in the muscle fibre density and the ratio of fast-twitch fibers to slow-twitch fibers and slow-twitch fibers to muscle fiber area (p < 0.05), but not in other muscle fibre properties (p > 0.05) (Table 1).
FIGURE 1. Fast/slow muscle fluorescence staining and paraffin section HE staining of muscle tissue. (A) Green represents slow muscle fiber, red represents fast muscle fiber, and blue represents the nucleus of the muscle cell. (B) Red represents a single muscle fibre, Blue represents a nucleus.
TABLE 1. Comparison of muscle fibre characteristics and growth characteristics of different breeds of cattle.
RNA was extracted from longissimus dorsi muscle samples of Shandong black cattle and Luxi cattle and sequenced. Totals of 105345396 and 126735736 reads were obtained from Shandong black cattle and Luxi cattle, respectively, with 101145946 and 122463284 mapped reads. In the data for Luxi cattle longissimus dorsi muscle, the percentages of exons, introns, and genes were 43.26%, 41.34%, and 15.4%, respectively, with 38.16%, 48.3%, and 13.54%, respectively, for Shandong black cattle longissimus dorsi muscle tissue, as shown in Figure 2.
FIGURE 2. The relative expression and the genome distribution of potential transcripts and clean reads. (A) The new transcripts were relatively expressed in longissimus dorsi muscle of Shandong black cattle and Luxi cattle, and the density distribution map was made after taking logarithm of 2 as the base. The abscissa was log2 (FPKM +0.0001), and the ordinate was gene density. Different colors represent different samples. (B) The results showed that the genomic distribution of clean data of longissimus dorsi muscle tissue of Shandong black cattle and Luxi cattle was genome distribution; the abscissa was each sample, and the ordinate was the ratio of the sequence number of exon, intron and intergenic region between the reference genome.
A total of 20104 transcripts were obtained from the two libraries, including 18859 and 18976 mRNAs for Luxi cattle and Shandong black cattle, respectively. According to the screening criteria of |FC|≧2 and q < 0.05, 1,415 differentially expressed mRNA (Supplementary Table S2) were found, with 939 mRNAs significantly up-regulated and 476 down-regulated in Luxi cattle. The 92 mRNAs were specifically expressed in cattle, with 30 and 62 mRNAs specifically expressed in Shandong black cattle and Luxi Cattle, respectively (Supplementary Table S2). The top 40 genes with significant differential expression in the two breeds in cattle are listed in Supplementary Table S3, and suggest genes that could be used for the functional comparison and analysis of differences among varieties.
Gene Ontology (GO) is an international standardized classification system of gene function that includes three categories: biological process (BP), molecular function (MF), and cell composition (CC). We performed GO enrichment analysis on 1,415 differentially expressed genes (Figures 3A,B), and the results showed 2,662 items (p < 0.05), with 388 were enriched in cell composition (CC), 361 in molecular function (MF), and 1913 in biological process (BP) in up-regulated genes (Shandong black cattle as a reference group). The enrichment results of down-regulated genes showed 1883 items in total (p < 0.05), with 251 enriched in cell composition (CC), 247 in molecular function (MF), and 1,385 in biological process (BP). In the muscle regulation system, muscle contraction is significantly enriched in GO terms, indicating that some differential genes may participate in muscle development and function and other life activities. Muscle-related GO terms are shown in Table 2.
FIGURE 3. Functional analysis of differential genes. (A,B) The results of Go cluster enrichment analysis for up-regulated and down-regulated genes: the horizontal axis represents the name of Go entry, and the vertical axis represents the number of genes enriched in the Go entry. (C,D). Up-regulated genes and down-regulated genes were analyzed by Go cluster analysis. The horizontal axis represents the name of the sample group, and the vertical axis represents the KEGG biological pathway. Each point indicates the enrichment degree of the KEGG entry, and the closer the color to green, the higher the enrichment degree. The size of each dot indicates the number of genes enriched in the KEGG entry. The larger the dot, the more genes are enriched in the KEGG entry, and vice versa.
Different genes coordinate with each other to perform their biological functions in organisms. We next performed KEGG pathway analysis of the differentially expressed genes to identify the related signal transduction and metabolic pathways (Figures 3C,D). The results showed enrichment of 939 up-regulated genes in 243 pathways (p < 0.05), and enrichment of 476 down-regulated genes in 239 pathways, including metabolism, disease, cell communication and endocrine regulation (Supplementary Table S4). The enriched pathways (p < 0.05) included some signaling pathways related to skeletal muscle growth, development, and function (Table 3), such as the Wnt signaling pathway, the calcium signal transduction pathway, gap junction, the Hippo signaling pathway, the AMPK signaling pathway, and the thyroid hormone synthesis pathway.
A total of 8,427 LncRNAs were found in the small RNA Library of longissimus dorsi muscle of Shandong black cattle and Luxi cattle. Of these, 3,498 LncRNAs were annotated in non-coding regions and the remaining 4,929 LncRNAs were in regions that are not annotated. According to the screening criteria of |FC|≧2 and q < 0.05, 480 differentially expressed LncRNAs were found, with 245 and 235 LncRNAs significantly up-regulated and down-regulated in Luxi Cattle compared to the levels in Shandong black cattle (Supplementary Table S5). A total of 92 LncRNAs were only expressed in one cattle breed, with 30 specific LncRNAs in Shandong black cattle and 62 specific LncRNAs in Luxi cattle (Supplementary Table S5). These breed-specific longissimus dorsi muscle LncRNAs included eight annotated LncRNAs in Shandong black cattle and 13 annotated LncRNAs in Luxi cattle, as shown in Table 4. The LncRNAs specifically expressed in Shandong black cattle and Luxi cattle, the first 40 unexplained LncRNAs and annotated LncRNAs are listed in Supplementary Table S6.
We constructed a Circos diagram to visually display the distribution of differential LncRNAs and differential mRNAs (Figure 4A). The results showed that most differentially expressed LncRNAs mapped to chromosome 9, with others mapping to chromosomes 3, 1, 11, and 7. Pearson correlation coefficient analysis of differentially expressed LncRNA and differential mRNA genes (Figure 4B) showed 43844 pairs of co-expressed LncRNAs and differential mRNA genes with correlation coefficient >0.9 (Supplementary Table S7). Based on genomic location, 387 differentially expressed LncRNAs were selected for further study.
FIGURE 4. (A). Circos map of differentially expressed genes and LncRNAs on cattle chromosomes. The outer ring is the diagram of autosomal distribution of the species; the first and second circles show the distribution of differentially expressed genes on chromosomes, with red lines indicating up-regulation, green lines indicating down-regulation, and the higher the column, the more differentially expressed genes in this region; the fourth and fifth circles show the distribution of LncRNAs on chromosomes, with the same expression pattern as genes. The internal lines indicate the corresponding relationship between LncRNAs and genes coexpressed by TOP 500. (B). The Pearson correlation coefficient heat map of differential LncRNAs and mRNAs: Red represents positive correlation and blue represents negative correlation.
LncRNAs can regulate the expression of adjacent mRNAs. We analyzed the LncRNAs and protein-coding genes by analyzing mRNAs within 50 kb of LncRNAs. The results showed that 387 of the differentially expressed LncRNAs targeted 1,164 genes. In these predictions, one LncRNA targeted multiple mRNAs and many LncRNAs targeted the same mRNA (Supplementary Table S8). Of these, LOC112448071, LOC112444635, LOC112445963, LOC104975064, LOC101903261, LOC535280, and LOC521224 can target genes related to muscle development, including MYORG, Wnt4, PAK1, and ADCY7.
The potential target genes of LncRNA were next subjected to GO and KEGG analysis (Figure 5). We found 130 significantly enriched GO items (p < 0.05), with five related to the regulation of muscle development (Table 5). The 40 most enriched terms include multicellular organization development, single multicellular organization process, and multicellular organic process (Supplementary Table S9). The GO terms related to muscle development are listed in Table 5. KEGG analysis showed enrichment of 1,164 potential target genes in 299 pathways, with the 40 most significantly enriched pathways listed in Supplementary Table S9. Some of these are related to muscle development (Table 6), including the calcium signaling pathway, the AMPK signaling pathway, the cGMP-PKG signaling pathway, and the PPAR signaling pathway.
FIGURE 5. Analysis results of potential target genes GO (A) and KEGG (B) of LncRNA differentially expressed in beef cattle muscle growth and development. The legend is the same as Figure 3.
We used Targetscan (Agarwal et al., 2015), Miranda (Enright et al., 2003), and PITA qtar software (Li et al., 2018) to predict the target gene relationships between mRNA and miRNA, and used Miranda and PITA qtar to predict the target gene relationships between miRNA and LncRNA. LncRNA can function as ceRNA to regulate the expression of downstream genes at the post-transcriptional level by binding miRNAs. We selected several miRNAs related to muscle development (including miR-1, miR-133, miR-206, miR-181, miR-21, and miR-23a) to predict and screen downstream-regulated mRNA using Targetscan and Miranda software. We then constructed an LncRNA-miRNA-mRNA interaction network diagram using Cytoscape version 3.5.1 (Figure 6). LncRNAs that may be involved in the development of beef cattle muscle are summarized in Table 7.
FIGURE 6. LncRNA-miRNA-mRNA network interaction diagram. LncRNA-miRNA-mRNA interaction network diagram related to muscle, green represents miRNA, orange represents mRNA, purple represents LncRNA.
We next looked for potential miRNA binding sites of LOC104975788 and found potential miR-133a and miR-103 binding sites. Online miRNA binding site prediction software (RNA22 and RNAhybird) predicted potential interaction of miR-133a with LOC104975788 and LOC536229(Pax7). The results showed that both LOC104975788 and LOC536229 (Pax7) contained miR-133a binding sites.
Nine differentially expressed LncRNAs and five differentially expressed mRNAs were verified by qRT-PCR. The qRT-PCR results (Figures 7D,E) confirmed differential expression of these LncRNAs and mRNAs.
FIGURE 7. Map of binding of bta-miR-133a with LOC104975788 and LOC536229 (Pax7) target site (A). Luciferase reporter assay showed significantly lower luciferase activity of miR-133a co transfected with the psi-LOC112448162 vector compared to the control group (p < 0.01). The luciferase activity of miR-133a co-transfected with psi-LOC536229 (Pax7) vector was also significantly decreased relative to the control Q19 group (p < 0.01) (B, C). These results indicate that both LOC104975788 and LOC536229 (Pax7) contain miR-133a binding sites. Nine differentially expressed LncRNAs and five differentially expressed mRNAs were verified by qRT-PCR (D, E).
The muscle fiber is the basic unit of muscle, and the type of muscle fiber greatly shapes muscle characteristics (Steinbacher et al., 2006). There were obvious differences in appearance in the muscle fibres isolated from longissimus dorsi muscles of Shandong black cattle and Luxi cattle. The average muscle fiber area of Shandong black cattle was significantly larger than that of Luxi cattle (p < 0.05), and the muscle fiber diameter was smaller than that of Luxi cattle. There was a significant positive correlation between muscle fiber area and carcass traits. Muscle fiber diameter is closely related to meat quality and taste and thicker muscle fiber diameter corresponds to decreased muscle tenderness. There is a negative correlation between muscle fiber diameter and muscle fiber density (Zhou, 2010). The amount of slow muscle fiber affects sarcomere length and has an important impact on meat quality (Hendricks et al., 1971). There were significant differences in the muscle fiber density, the ratio of fast-twitch fibers/slow-twitch fibers, and weight (p < 0.05) in this study. These differences may be key factors leading to the differences in meat production performance and meat quality of the two breeds of cattle after birth, which was also the research basis of this study to explore the underlying molecular regulatory mechanism.
With low conservation of LncRNA sequences among species, bioinformatics methods are required to screen and identify LncRNAs. This type of analysis is based on transcript length, the number of exons, and the coding potential. In this study, transcriptome data of skeletal muscle of Shandong black cattle and Luxi cattle were generated and analyzed. In our studies, 1,415 transcripts were found to be differentially expressed, with 939 and 476 transcripts were significantly up-regulated and down-regulated in Luxi cattle (Shandong black cattle as a reference group), respectively. Further, 19 GO items and 14 regulatory pathways related to muscle development were screened by hierarchical GO and KEGG cluster analysis. Many genes involved in the regulation of muscle development were identified, including myosin protein family genes (MYH1, MYH3, MYH4, MYH8, and MYL3), myogenic regulatory factor family genes (MY O 10 and MY O 5B), Homeobox family genes (HOXC10, HOXC11, and HOXD9), Troponin T family genes (TNNT2 and TNNT3) and some regulatory transcription factors (WNT4 and TNNT2). It is worth noting that three LncRNAs (LOC107133268, LOC112445823, LOC104969184) are directly enriched into muscle regulation items. Further target gene prediction analysis shows that LncRNAs may participate in the regulation of muscle development through bta-miR-2892 (LOC107133268), bta-miR-2360 and bta-miR-2449 (LOC112445823). The specific regulatory mechanism needs to be further verified and analyzed.
Compared with studies in human (Federica et al., 2019) and other model organisms (James et al., 2015), there has been limited identification and characterization of beef LncRNAs, especially ones related to skeletal muscle development, with most studies of cattly focused on the identification of genes and miRNAs (Xue et al., 2013; Peters et al., 2017). In this study, we identified 8,427 multiple exon LncRNAs in beef skeletal muscle, and 480 differentially expressed LncRNAs were identified. More LncRNAs were detected in this study than previously reported in goats (Sun et al., 2013; Ying-hui et al., 2019). Fifteen randomly selected differentially expressed transcripts were verified by qPCR, and the results were consistent with the results of RNA sequencing. In conclusion, these results confirm the reliability of the identification of LncRNAs (Langfelder et al., 2008).
Although LncRNAs can act on gene sites far from their chromosomal location (Cohen et al., 2000), genes in close proximity on a chromosome may participate in the same cellular metabolic pathways and have similar biological functions (Leonardo et al., 2011). Therefore, the distribution of differentially expressed LncRNAs on chromosomes and the linkage differential expression of nearby genes may have biological significance that can help us to determine the function of a gene. The most differentially expressed LncRNAs were found on chromosome 9, followed by chromosomes 3, 1, 11, and 7. In co-expression analysis, we detected 387 differentially expressed LncRNA transcripts related to protein-coding genes according to the expression correlation coefficient values (r > 0.9), and predicted 1,164 target genes. GO analysis showed 20 GO terms related to the regulation of genes involved in muscle development. KEGG analysis showed enrichment of 1,164 potential target genes in 299 pathways, with some related to muscle development, such as the calcium signaling pathway, the AMPK signaling pathway, the cGMP - PKG signaling pathway, and the PPAR signaling pathway. Interestingly, we also found MYORG, Dll1, EFNB2, SOX6, PROX1, MYOCD, NEBL and MYLK3 genes, annotated as related to muscle development. Overall, LncRNAs may play a regulatory role in skeletal muscle biological development through cis or trans mechanisms.
At the post transcriptional level, LncRNA binds miRNA competitively with mRNA for protein-coding genes, thus relieving the inhibitory effect of miRNA on protein-coding genes to promote expression of these genes (Kartha and Subramanian, 2014). We analyzed the expression patterns of LncRNAs differentially expressed among different breeds, and constructed an LncRNA-miRNA-mRNA interaction network related to skeletal muscle development. A total of 52 LncRNAs related to muscle development were identified, with nine up-regulated and five down-regulated. All of these LncRNAs contain one or more putative miRNA binding sites with 48 LncRNAs predicted to interact with one to two miRNAs related to muscle development, and other LncRNAs predicted to interact with more than three miRNAs, such as LOC525506, LOC540051, LOC514189. With the miRNA seed sequences, LncRNAs can bind to miRNA to act as a sponge, preventing miRNA from binding to its target mRNA. As a classic example, M. Cesana (Marcella Davide et al., 2011) confirmed that linc-MD1, a long non-coding RNA specifically expressed during myoblast differentiation, regulates the expression of muscle specific transcription regulators MAML1 and MEF2C through miR-133 and miR-135. In particular, LOC525506 may be involved in the development of beef skeletal muscle by regulating miR-1, miR-23a, miR-378, let-7, miR-483, and miR-21. This is the most predicted LncRNA, but little is known about its expression and function. Both LOC112447073 and LOC104975788 are involved in the skeletal muscle development of beef cattle by interaction with miR-103. More interestingly, some target genes of LOC112447073, LOC104975788, and LOC101903367 directly regulate the development of muscle fiber and maintain the stability and development of muscle, such as miR-103, miR-133a, miR-145, MEF2C, myocardin, and Pax7 (Richard et al., 2008; Mathew et al., 2011). Choi identified 11 LncRNAs in bovine transcripts by studying skeletal muscle and adipose tissue of Korean cattle, with four related to muscle function (Jae et al., 2018). These data provide new insight into the role of LncRNA in muscle development and enrich the existing LncRNA mammalian skeletal muscle resources.
The second to eighth bases of the sequence at the 5 ′end of miRNA is called the seed sequence. Complete complementarity of this sequence and that of the target gene indicates the potential for binding of the target gene by miRNA (Lewis et al., 2005; Andrew et al., 2007). We predicted the miRNA binding sites of functional LncRNAs that may be related to skeletal muscle development. Using a double luciferase test, we found a recognition site of bta-mir-133a in the sequence of LOC104975788 and a binding site for bta-mir-133a in the downstream target gene Pax7. We speculate that LOC104975788 may be involved in the regulation of skeletal muscle development by competitively inhibiting the expression of the target gene Pax7 through binding of bta-miR-133a. Future work should test this proposed regulatory mechanism.
The expression patterns of LncRNAs in skeletal muscle of Shandong black cattle and Luxi cattle were elucidated by RNA sequence analysis, and the LncRNAs that may be involved in the skeletal muscle development of beef cattle were identified. The results allowed construction of interaction networks of LncRNAs-miRNA-mRNA regulated by muscle biology. We speculate that LOC104975788 may be involved in the regulation of skeletal muscle development by competitively inhibiting the expression of the target gene Pax7 through binding of bta-miR-133a.
The datasets generated during and/or analysed during the current study are available from the corresponding author on reasonable request. The RNA sequencing data has been uploaded to the NCBI GEO database, and can be retrieved using the following accession numbers: GSM4904154, GSM4904155, 623 GSM4904156, GSM4904157, GSM4904158, GSM4904159.
The animal study was reviewed and approved by Biological Studies Animal Care and Use Committee, Shandong Province, China.
YD, XB, KY, RL and MH designed the study. RL and MH conducted the experiment. RL, MH, XL and XB performed and collected the data. RL analyzed the data and wrote the manuscript. All authors read and approved the final manuscript.
This work was supported by Shandong modern agricultural industry technology system cattle industry innovation team (668-2216030). The team participated in the collection and analysis of data.
The authors declare that the research was conducted in the absence of any commercial or financial relationships that could be construed as a potential conflict of interest.
All claims expressed in this article are solely those of the authors and do not necessarily represent those of their affiliated organizations, or those of the publisher, the editors, and the reviewers. Any product that may be evaluated in this article, or claim that may be made by its manufacturer, is not guaranteed or endorsed by the publisher.
The authors would like to thank Shanghai Sangon Biotechnology Co., Ltd. and Beijing AnnoroadGene Co., Ltd. for their assistance in the original data processing and related bioinformatics analysis for RNA-sequencing and GC-MS. The authors also thank Liu for her meticulous revision of language. More importance, special thanks to Yue Liu for his contribution to data processing. Additionally, the authors would like to particularly thank her parents and Dong, for their continuing love and support.
The Supplementary Material for this article can be found online at: https://www.frontiersin.org/articles/10.3389/fgene.2022.849399/full#supplementary-material
DEGs, differentially expressed genes; KEGG, Kyoto Encyclopedia of Genes and Genomes; GO, The Gene Ontology; LncRNA, Long noncoding RNA; miRNA, MicroRNA (miRNA) is a class of noncoding single stranded RNA molecules with a length of about 22 nucleotides encoded by endogenous genes; mRNA, Messenger RNA (mRNA) that encodes proteins.
Agarwal, V., Bell, G. W., Nam, J. W., and Bartel, D. P. (2015). Predicting Effective microRNA Target Sites in Mammalian mRNAs. eLife 12, e05005. doi:10.7554/eLife.05005
Anders, S., and Huber, W. (2010). Differential Expression Analysis for Sequence Count Data. Genome Biol. 11, R106. doi:10.1186/gb-2010-11-10-r106
Andersson, L. (2009). Genome-wide Association Analysis in Domestic Animals: a Powerful Approach for Genetic Dissection of Trait Loci. Genetica 136 (2), 341–349. doi:10.1007/s10709-008-9312-4
Andrew, G., Kyle, K. F., Wendy, K. J., Philip, G., Lee, P. L., and David, P. B. (2007). Micro RNA Targeting Specificity in Mammals: Determinants beyond Seed Pairing. Mol. Cel 27, 91–105. doi:10.1016/j.molcel.2007.06.017
Ballarino, M., Cazzella, V., D'Andrea, D., Grassi, L., Bisceglie, L., Cipriano, A., et al. (2015). Novel Long Noncoding RNAs (lncRNAs) in Myogenesis: a miR-31 Overlapping lncRNA Transcript Controls Myoblast Differentiation. Mol. Cel Biol 35 (4), 728–736. doi:10.1128/mcb.01394-14
Billerey, C., Boussaha, M., Esquerré, D., Rebours, E., Djari, A., Meersseman, C., et al. (2014). Identification of Large Intergenic Non-coding RNAs in Bovine Muscle Using Next-Generation Transcriptomic Sequencing. BMC genomics 15 (1), 499. doi:10.1186/1471-2164-15-499
Bu, D., Luo, H., Huo, P., Wang, Z., Zhang, S., He, Z., et al. (2021). KOBAS-i: Intelligent Prioritization and Exploratory Visualization of Biological Functions for Gene Enrichment Analysis. Nucleic Acids Res. 49, W317–W325. doi:10.1093/nar/gkab447
Cabili, M. N., Trapnell, C., Goff, L., Koziol, M., Tazon-Vega, B., Regev, A., et al. (2011). Integrative Annotation of Human Large Intergenic Noncoding RNAs Reveals Global Properties and Specific Subclasses. Genes Dev. 25, 1915–1927. doi:10.1101/gad.17446611
Cai, R., Zhang, Q., Wang, Y., Yong, W., Zhao, R., and Pang, W. (2021). Lnc-ORA Interacts with microRNA-532-3p and IGF2BP2 to Inhibit Skeletal Muscle Myogenesis. J. Biol. Chem. 296, 100376. doi:10.1016/j.jbc.2021.100376
Cai, W., Li, C., Liu, S., Zhou, C., Yin, H., Song, J., et al. (2018). Genome Wide Identification of Novel Long Non-coding RNAs and Their Potential Associations with Milk Proteins in Chinese Holstein Cows. Front. Genet. 9, 281. doi:10.3389/fgene.2018.00281
Cai, Y., He, J., and Zhang, D. (2015). Long Noncoding RNA CCAT2 Promotes Breast Tumor Growth by Regulating the Wnt Signaling Pathway. Onco Targets Ther. 8, 2657–2664. doi:10.2147/OTT.S90485
Cao, T., Shi, L. G., and Zhang, L. L. (2014). Comparative Study on Fetal Muscle Fiber of Wuzhishan Pig and Changbai Pig during 65d Gestation. J. Anim. Ecol. 35, 37–40.
Chen, S., Zhou., Y., Chen, Y., and Gu, J. (2018). Fastp: an Ultra-fast All-In-One FASTQ Preprocessor. Bioinformatics 34 (17), i884–i890. doi:10.1093/bioinformatics/bty560
Cohen, B. A., Mitra, R. D., Hughes, J. D., and Church, G. M. (2000). A Computational Analysis of Whole-Genome Expression Data Reveals Chromosomal Domains of Gene Expression. Nat. Genet. 26, 183–186. doi:10.1038/79896
Dacia, D., Alessio, C., Monica, B., Julie, M., Davide, M., Johannes, D., et al. (2018). Irene, B. The Long Non-coding RNA Lnc-31 Interacts with Rock1 mRNA and Mediates its YB-1-dependent Translation. Cel Rep. 23 (3), 733–740. doi:10.1016/j.celrep.2018.03.101
Dylan, S., Katarzyna, G., Tina, R., Svetlana, O., Thomas, B., Tamas, D., et al. (2008). Specific Requirements of MRFs for the Expression of Muscle Specific microRNAs, miR-1, miR-206 and miR-133. Dev. Biol. 321 (2), 491–499. doi:10.1016/j.ydbio.2008.06.019
Enright, A. J., John, B., Gaul, U., Tuschl, T., Sander, C., and Marks, D. S. (2003). MicroRNA Targets in Drosophila. Genome Biol. 5 (1), R1. doi:10.1186/gb-2003-5-1-r1
Federica, A., Vijay, A. R., Andrei, E. M., Sivapriya, K. V., and Anthony, T. V. (2019). Long Noncoding RNAs in Host-Pathogen Interactions. Trends Immunol. 40, 492–510. doi:10.1016/j.it.2019.04.001
Hendricks, H. B., Lafferty, D. T., Aberle, E. D., Judge, M. D., and Forrest, J. C. (1971). Relation of Porcine Muscle Fiber Type and Size to Postmortem Shortening. Anim. Sci. 32, 57–61. doi:10.2527/jas1971.32157x
Ito, E. A., Katahira, I., Vicente, F. F. d. R., Pereira, L. F. P., and Lopes, F. M. (2018). BASiNET-BiologicAl Sequences NETwork: a Case Study on Coding and Non-coding RNAs Identification. Nucleic Acids Res. 46 (16), e96. doi:10.1093/nar/gky462
Jae, Y. C., Donghyun, S., Hyun, J. L., and Jae, D. O. (2018). Comparison of Long Noncoding RNA between Muscles and Adipose Tissues in Hanwoo Beef Cattle. Anim. Cell Syst. official Publ. Zoolog. Soc. Korea 23, 1–9. doi:10.1080/19768354.2018.1512522
James, A. B., Ernst, J. W., John, S. M., John, L. R., and Guy, B. (2015). Mechanisms of Long Non-coding RNAs in Mammalian Nervous System Development, Plasticity, Disease, and Evolution. Neuron 88, 861–877. doi:10.1016/j.neuron.2015.09.045
Jing, Z., Toshiro, K. O., Johnny, T. K., Yuya, O., Daniel, J. G., and Kavitha, S. (2010). Genome-wide Identification of Polycomb-Associated RNAs by RIP-Seq. Mol. Cel 40, 939–953. doi:10.1016/j.molcel.2010.12.011
Kanehisa, M., and Goto, S. K. E. G. G. (2000). KEGG: Kyoto Encyclopedia of Genes and Genomes. Nucleic Acids Res. 128 (1), 27–30. doi:10.1093/nar/28.1.27
Kartha, R. V., and Subramanian, S. (2014). Competing Endogenous RNAs (ceRNAs): New Entrants to the Intricacies of Gene Regulation. Front. Genet. 5, 8. doi:10.3389/fgene.2014.00008
Kovaka, S., Zimin, A. V., Pertea, G. M., Razaghi, R., Salzberg, S. L., and Pertea, M. (2019). Transcriptome Assembly from Long-Read RNA-Seq Alignments with StringTie2. Genome Biol. 20, 278. doi:10.1186/s13059-019-1910-1
Langfelder, P., Zhang, B., and Horvath, S. (2008). Defining Clusters from a Hierarchical Cluster Tree: The Dynamic Tree Cut Package for R. Bioinform 24, 719–720. doi:10.1093/bioinformatics/btm563
Leonardo, S., Laura, P., Yvonne, T., Lev, K., and Pier, P. P. (2011). A ceRNA Hypothesis: the Rosetta Stone of a Hidden RNA Language? Cell 146, 353–358. doi:10.1016/j.cell.2011.07.014
Lewis, B. P., Burge, C. B., and Bartel, D. P. (2005). Conserved Seed Pairing, Often Flanked by Adenosines, Indicates that Thousands of Human Genes Are MicroRNA Targets. Cell 120, 15–20. doi:10.1016/j.cell.2004.12.035
Li, D., Bao, P., Yin, Z., Sun, L., Feng, J., He, Z., et al. (2018). Exploration of the Involvement of LncRNA in HIV-Associated Encephalitis Using Bioinformatics. PeerJ 6, e5721. doi:10.7717/peerj.5721
Liu, R., Liu, X., Bai, X., Xiao, C., and Dong, Y. (2020). Different Expression of Lipid Metabolism-Related Genes in Shandong Black Cattle and Luxi Cattle Based on Transcriptome Analysis[J]. Scientific Rep. 10, 1. doi:10.1038/s41598-020-79086-4
Liu, R., Liu, X., Bai, X., Xiao, C., and Dong, Y. (2018). Screening of Skeletal Muscle Differential Genes Based on Transcriptome. North China Agric. J. 33, 64–72. doi:10.3389/fvets.2022.831519
Lu, W. (2015). Feeding and Management Techniques of Luxi Cattle in Fattening Period. Beijing Agric. 25 (2015), 130–131.
Luo, H., Bu, D., Sun, L., Fang, S., Liu, Z., and Zhao, Y. (2017). Identification and Function Annotation of Long Intervening Noncoding RNAs. Brief Bioinform 18 (5), 789–797. doi:10.1093/bib/bbw046
Mahmoudi, B., Fayazi, J., Roshanfekr, H., Sari, M., and Bakhtiarizadeh, M. R. (2020). Genome-wide Identification and Characterization of Novel Long Non-coding RNA in Ruminal Tissue Affected with Sub-acute Ruminal Acidosis from Holstein Cattle. Vet. Res. Commun. 44 (1), 19–27. doi:10.1007/s11259-020-09769-w
Marcella, C., Davide, C., Ivano, L., Tiziana, S., Olga, S., Mauro, C., et al. (2011). A Long Noncoding RNA Controls Muscle Differentiation by Functioning as a Competing Endoge-Nous RNA. Cell 147 (2), 358–369. doi:10.1016/j.cell.2011.09.028
Mathew, S. J., Hansen, J. M., Merrell, A. J., Murphy, M. M., Lawson, J. A., Hutcheson, D. A., et al. (2011). Connective Tissue Fibroblasts and Tcf4 Regulate Myogenesis. Dev. (Camb. Engl. 138, 371–384. doi:10.1242/dev.057463
Pertea, M., Pertea, G. M., Antonescu, C. M., Chang, T.-C., Mendell, J. T., and Salzberg, S. L. (2015). StringTie Enables Improved Reconstruction of a Transcriptome from RNA-Seq Reads. Nat. Biotechnol. 33 (3), 290–295. doi:10.1038/nbt.3122
Peters, E., van der Linde, S., Vogel, I., Haroon, M., Offringa, C., de Wit, G., et al. (2017). IGF-1 Attenuates Hypoxia-Induced Atrophy but Inhibits Myoglobin Expression in C2C12 Skeletal Muscle Myotubes. Ijms 18, 1889. doi:10.3390/ijms18091889
Richard, D. B., Ariel, J. C., Amy, S. M., and Emanuel, E. S. (2008). Interaction with the IQ3 Motif of Myosin-10 Is Required for Calmodulin-like Protein-dependent Filopodial Extension. Febs Lett. 582, 2377–2381. doi:10.1016/j.febslet.2008.05.051
Shannon, P., Markiel, A., Ozier, O., Baliga, N. S., Wang, J. T., Ramage, D., et al. (2003). Cytoscape: a Software Environment for Integrated Models of Biomolecular Interaction Networks. Genome Res. 13 (11), 2498–2504. doi:10.1101/gr.1239303
Steinbacher, P., Haslett, J. R., Sänger, A. M., and Stoiber, W. (2006). Evolution of Myogenesis in Fish: a sturgeon View of the Mechanisms of Muscle Development. Anat. Embryol. 211, 311–322. doi:10.1007/s00429-006-0082-4
Sun, L., Luo, H., Bu, D., Zhao, G., Yu, K., Zhang, C., et al. (2013). Utilizing Sequence Intrinsic Composition to Classify Protein-Coding and Long Non-coding Transcripts. Nucleic Acids Res. 41, e166. doi:10.1093/nar/gkt646
Sun, X., Li, M., Sun, Y., Cai, H., Lan, X., Huang, Y., et al. (2016). The Developmental Transcriptome Sequencing of Bovine Skeletal Muscle Reveals a Long Noncoding RNA, lncMD, Promotes Muscle Differentiation by Sponging miR-125b. Biochim Biophys Acta. 1863 (11), 2835–2845. doi:10.1016/j.bbamcr.2016.08.014
Wang, L., Feng, Z., Wang, X., Wang, X., and Zhang, X. (2010). DEGseq: an R Package for Identifying Differentially Expressed Genes from RNA-Seq Data. Bioinformatics 26, 136–138. doi:10.1093/bioinformatics/btp612
Xu, J. W., Zheng, L., Li, L. J., Yao, Y. F., Hua, H., Yang, S. Z., et al. (2018). Novel Copy Number Variation of the KLF3 Gene is Associated With Growth Traits in Beef Cattle. Gene 680, 99–104. doi:10.1016/j.gene.2018.08.040
Xu, M., Chen, X., Chen, D., Yu, B., Li, M., He, J., et al. (2020). Regulation of Skeletal Myogenesis by microRNAs. J. Cel Physiol 235 (1), 87–104. doi:10.1002/jcp.28986
Xue, Z., Huang, K., Cai, C., Cai, L., Jiang, C.-y., Feng, Y., et al. (2013). Genetic Programs in Human and Mouse Early Embryos Revealed by Single-Cell RNA Sequencing. Nature 500, 593–597. doi:10.1038/nature12364
Ying-hui, L., Qi, Z., Meng-hua, S., Lu, Z., Li-na, X., Yun-hai, Z., et al. (2019). Comprehensive Analysis of LncRNA Reveals the Temporal-specific Module of Goat Skeletal Muscle Development. Int. J. Mol. Sci. 20 (16), 3950. doi:10.3390/ijms20163950
Young, M. D., Wakefield, M. J., Smyth, G. K., and Oshlack, A. (2010). Gene Ontology Analysis for RNA-Seq: Accounting for Selection Bias. Genome Biol. 11, R14. doi:10.1186/gb-2010-11-2-r14
Yu-ying, L., Xiao-na, C., Hao, S., and Hua-ting, W. (2017). Long Non-coding RNAs in the Regulation of Skeletal Myogenesis and Muscle Diseases. Cancer Lett. 417, 58–64. doi:10.1016/j.canlet.2017.12.015
Yuan, G., Shi-peng, L., Zhen-yu, L., Zi-hui, Z., Fei, W., Yong-zhen, H., et al. (2019). Analysis of Long Non-coding RNA and mRNA Expression Profiling in Immature and Mature Bovine (Bos taurus) Testes. Front. Genet. 10, 646. doi:10.3389/fgene.2019.00646
Yue, Y., Jin, C., Chen, M., Zhang, L., Liu, X., Ma, W., et al. (2017). A lncRNA Promotes Myoblast Proliferation by Up-Regulating GH1. In Vitro Cell.Dev.Biol.-Animal 53 (8), 699–705. doi:10.1007/s11626-017-0180-z
Zhang, S., Knight, T. J., Stalder, K. J., Goodwin, R. N., Lonergan, S. M., and Beitz, D. C. (2007). Effects of Breed, Sex, and Halothane Genotype on Fatty Acid Composition of Pork Longissimus Muscle1. J. Anim. Sci. 85 (3), 583–591. doi:10.2527/jas.2006-239
Keywords: shandong black cattle, luxi cattle, lncRNA, longissimus dorsi, identification
Citation: Liu R, Han M, Liu X, Yu K, Bai X and Dong Y (2022) Genome-Wide Identification and Characterization of Long Non-Coding RNAs in Longissimus dorsi Skeletal Muscle of Shandong Black Cattle and Luxi Cattle. Front. Genet. 13:849399. doi: 10.3389/fgene.2022.849399
Received: 06 January 2022; Accepted: 08 April 2022;
Published: 16 May 2022.
Edited by:
Natalia A Zinovieva, L.K. Ernst Federal Science Center for Animal Husbandry (RAS), RussiaReviewed by:
Juliana Afonso, Animal Biotechnology Laboratory. Embrapa Pecuária Sudeste, BrazilCopyright © 2022 Liu, Han, Liu, Yu, Bai and Dong. This is an open-access article distributed under the terms of the Creative Commons Attribution License (CC BY). The use, distribution or reproduction in other forums is permitted, provided the original author(s) and the copyright owner(s) are credited and that the original publication in this journal is cited, in accordance with accepted academic practice. No use, distribution or reproduction is permitted which does not comply with these terms.
*Correspondence: Yajuan Dong, ZXRjZW50ZXJAMTI2LmNvbQ==
†These authors have contributed equally to this work
Disclaimer: All claims expressed in this article are solely those of the authors and do not necessarily represent those of their affiliated organizations, or those of the publisher, the editors and the reviewers. Any product that may be evaluated in this article or claim that may be made by its manufacturer is not guaranteed or endorsed by the publisher.
Research integrity at Frontiers
Learn more about the work of our research integrity team to safeguard the quality of each article we publish.