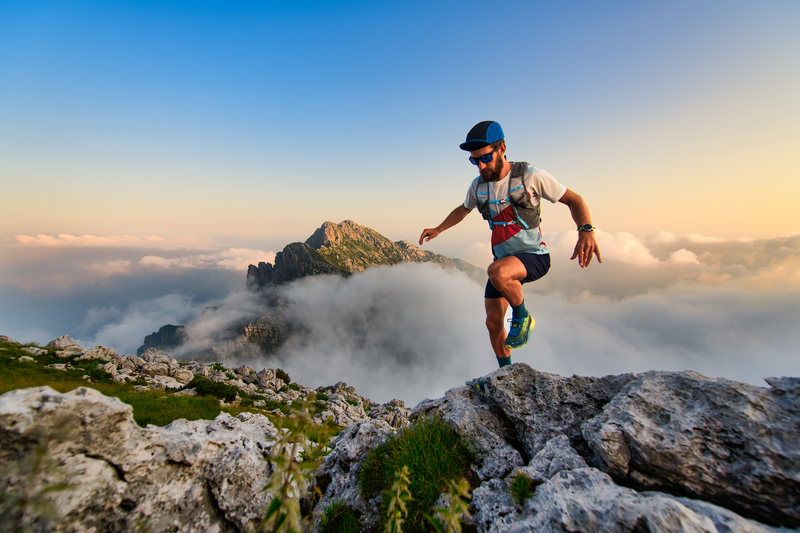
95% of researchers rate our articles as excellent or good
Learn more about the work of our research integrity team to safeguard the quality of each article we publish.
Find out more
ORIGINAL RESEARCH article
Front. Genet. , 25 March 2022
Sec. Genomics of Plants and the Phytoecosystem
Volume 13 - 2022 | https://doi.org/10.3389/fgene.2022.845602
This article is part of the Research Topic Legume Breeding in Transition: Innovation and Outlook View all 28 articles
Peanut is one of the most important cash crops with high quality oil, high protein content, and many other nutritional elements, and grown globally. Cultivated peanut (Arachis hypogaea L.) is allotetraploid with a narrow genetic base, and its genetics and molecular mechanisms controlling the agronomic traits are poorly understood. Here, we report a comprehensive genome variation map based on the genotyping of a panel of 178 peanut cultivars using Axiom_Arachis2 SNP array, including 163 representative varieties of different provinces in China, and 15 cultivars from 9 other countries. According to principal component analysis (PCA) and phylogenetic analysis, the peanut varieties were divided into 7 groups, notable genetic divergences between the different areas were shaped by environment and domestication. Using genome-wide association study (GWAS) analysis, we identified several marker-trait associations (MTAs) and candidate genes potentially involved in regulating several agronomic traits of peanut, including one MTA related with hundred seed weight, one MTA related with total number of branches, and 14 MTAs related with pod shape. This study outlines the genetic basis of these peanut cultivars and provides 13,125 polymorphic SNP markers for further distinguishing and utility of these elite cultivars. In addition, the candidate loci and genes provide valuable information for further fine mapping of QTLs and improving the quality and yield of peanut using a genomic-assisted breeding method.
Cultivated peanut (Arachis hypogaea. L) is one of the most important oil crops and cash crops in the world. In 2019, the total production of peanuts was approximately 48.8 million tons (http://www.fao.org). Peanuts are full of high-quality vegetable oil, protein, more than 30 essential vitamins, and many other nutrients, and is part of a balanced diet for human health. Peanuts are widely planted in India, China, United States, Argentina, Australia, and Brazil. In the past 20 years, the average yield of peanut increased from 1.95 t/ha in 1999 to 3.3 t/ha in 2019 in the above six countries (http://www.fao.org). However, peanut is often grown on marginal soils with lesser inputs and usually intercropped with cereals in many countries such as China and India, the top two countries with the largest peanut harvest area. At present, almost all peanut varieties are developed through conventional breeding methods, marker-assisted selection (MAS) technology was only used in a few traits in the peanut breed program, such as oleic acid content, and root-knot nematode resistance (Chu et al., 2011; Shasidhar et al., 2020). The average yield of peanut is significantly lower than that of the staple food crop, rice, and corn. Peanut germplasm resources have a narrow genetic background. It has been difficult to get significant improvement of the yield and quality through traditional cross-breeding. In the future, MAS will be an important alternative approach for increasing the yield and improving the quality of peanut.
Compared to the conventional breeding approach, MAS technology can significantly accelerate breeding process and improve breeding efficiency by increasing the genetic gains per selection cycles (Collard and Mackill, 2007; Varshney et al., 2013). The utility of MAS is becoming more and more popular in crop breeding programs. For example, in wheat, hundreds of resistance (R) genes to powdery mildew, leaf rust, and stripe rust have been mapped (Pinto da Silva et al., 2018; Qureshi et al., 2018; Shah et al., 2018), and many of them have been successfully used to improve the resistance of wheat through MAS. For MAS technology, identification of quantitative trait loci (QTL) or genes, and development of the closely linked markers is necessary. With the availability of genome sequence information and high-throughput genotyping technologies, genome-wide association study (GWAS) has become a powerful way to identify the tightly linked markers and QTLs from the genome, superseding the traditional QTL mapping method from the structured populations derived from two parents (Pujar et al., 2020). GWAS has been successfully used in identifying the QTLs and the key genes related with the complex traits on peanut. (Gangurde et al., 2020). In peanut, the markers associated with oil, protein, oleic acid, and linoleic acid through a preliminary GWAS analysis with 120 simple sequence repeat (SSR) and transposable element (TE) markers have been reported (Zhang et al., 2020b). Recently, using genotyping-by-sequencing based SNP markers, 79 loci significantly associated for the six yield-related traits were also reported (Zhou et al., 2021).
In the last decade, advances in high throughput sequencing and bioinformatics technologies provided a good platform for peanut genome research including marker development and trait mapping as well as development of molecular breeding products (Zhao et al., 2017; Han et al., 2018; Luo et al., 2018; Bertioli et al., 2019; Zhuang et al., 2019; Ma et al., 2020; Pandey et al., 2020; Zhao et al., 2020). The availability of large-scale genomic resources was used for identifying a large number of genome-wide SNPs, and high-throughput genotyping platforms like 48 K SNP array (Axiom_Arachis2, version II) (Clevenger et al., 2018) and 58K SNP array (Pandey et al., 2017). The 48 K SNP array is also used for GWAS analysis for 96 peanut genotypes and revealed that current Korean genetic resources lacked variability compared to US mini-core genotypes (Nabi et al., 2021). Zhang et al. reported the identification of 36 QTLs related with the 13 nutrient elements and 46 QTLs related with leaf spots resistance using the SNP array based GWAS analysis for 120 mini-core germplasms (Zhang H. et al., 2019; Zhang et al., 2020a). All these studies have successfully discovered loci associated with the agronomic traits of peanut.
In this study, we analyzed 20 phenotypic traits of 178 peanut cultivars from diverse origins in China and 9 other countries. We analyzed the genetic divergences of these peanut cultivars and identified a number of significant genetic loci related to phenotypic traits, which will be helpful for further fine mapping and genomic-assisted breeding.
For genome-wide association study, a set of 178 peanut varieties (of which 119 varieties represented varieties from 13 provinces and regions including Shandong, Henan, Guangdong, and Fujian; 41 featured germplasm resources preserved in laboratories, and 18 were exotic, i.e., imported from resources abroad) were selected based on phenotypic characteristics, including plant height, number of total branches, seed and pod size, protein, etc. The botanical information for the selected Chinese varieties was derived from available monographs (Feng, 1987; Yu, 2008) and an online database (http://www.peanutdata.cn). The 178 peanut varieties consisted of var. hypogaea, var. vulgaris, var. fastigiata, var. hirsuta, and irregular type varieties. The detailed information of each sample was also listed on the Supplementary File S1.
The test materials used were planted in the Jiyang Agricultural Planting base in Jinan City in the summer for 3 consecutive years from 2018 to 2020. The field experiment is a completely random design and adopts conventional cultivation management (Wan, 2003). At harvest, there are three peanut plants randomly selected from each peanut variety and the main stem height, lateral branch angle, total number of branches, pod length, seed length, linoleic acid content, and other traits were measured. The phenotypic data obtained were analyzed using Excel data analysis tools for descriptive statistics and normal distribution test, and Origin software was used for drawing.
DNA was extracted from 15-day-old seedlings using Plant Genome Extraction Kit (Beijing, China), following the manufacturer’s instructions (https://www.tiangen.com/). The DNA was visualized in agarose gel containing Super GelRed (US Everbright Inc., Suzhou, China), and then quality and concentration were determined using Nanodrop™ 2000 spectrophotometer (Thermo Scientific, Shanghai, China). The second-generation 48K SNP array of peanut was used to obtain genotyping data of 178 materials (Clevenger et al., 2018). SNPs with low call rates were removed with selection criteria of missing data rate (>10%) and minor allele frequency (<5%). Only high-quality SNPs were selected for further analysis. Reference genome builds were acquired from arahy (https://peanutbase.org/peanut_genome).
The phylogenetic tree was constructed based on the SNPs identified above by maximum likelihood (ML) method in IQ-tree v1.6.12 (Minh et al., 2020) (http://www.iqtree.org/), which was visualized with ITOL software (Letunic and Bork, 2021) (https://itol.embl.de/). The bootstrap values were calculated with 1000 replicates. The population structure of the 178 samples was first evaluated using PCA by the GCTA package and later using Admixture v1.3.0 (Alexander et al., 2009). We used the default parameters in Admixture to test the number of ancestral populations (K) with a cross-validation (CV) process, and the one with minimum CV error calculated was selected as best K value (http://software.genetics.ucla.edu/admixture/admixture-manual.pdf) (Alexander et al., 2009), which was visualized in R script next.
The TASSEL v5.2.1 software was used for the genome-wide association study (GWAS) analysis of 7 aforementioned yield and quality related traits with the high-quality SNPs (Bradbury et al., 2007). Both generalized linear model (GLM) and mixed linear model (MLM) were used to determine MTAs. In general, the GLM model focuses on the SNP effects, which only contains the fixed effects such as population structure and genotype, and the MLM model additionally adds random effects (kinship matrix) to correct for the cryptic relatedness. The Q-Q plots were used for selecting the best model of each trait. The Bonferroni-corrected p-value was used for mining the trait-related genome regions, and the markers that p-value of 0.05/13,125 (the total number of SNPs) or less were defined as significant. Based on the loci of MTAs, we used the online software - genome browser of peanut (https://www.peanutbase.org/gbrowse_peanut1.0) to screen the trait-related candidate genes among the trait-related regions.
Based on 3 years’ evaluation data, we observed a large phenotypic variability among 178 peanut cultivars for all the traits studied. Phenotype identification and statistical analysis showed that the 178 peanut genotypes displayed wide ranges of phenotypic variation for most of the agronomic traits. For example, the length of the lateral branch, the height of the main stem, and the angle of the lateral branch are important factors for determining the peanut plant type, which varied from 44.2 to 106.6 cm, 82.8–34.2 cm, and 30–90°, respectively (Table 1). Besides, many traits related to yield and quality as well as significant variation included the weight and the length of seed and the number of branches with the pod, and the latter varied from average 2.6 to 12 (Table 1). Moreover, the testa color also had high variation in these peanut varieties, and the seeds with pink, red, black, white, and variegation are included. In addition, many quality traits are also varied, including the content of oleic acid and linoleic acid (Table 1). Most of these traits accord with normal distributions indicating these traits could be quantitative traits (Figure 1).
FIGURE 1. Frequency distribution of 178 peanut cultivars for 20 traits. MSH, main stem height; LBL, lateral branch length; TNB, total number of branches; PBN, pod-bearing branches number; PNP, pod number per plant; LBA, lateral branch angle; HPW, hundred pod weight; HSW, hundred seed weight; PL, pod length; PW, pod width; SL, seed length; SW, seed width; PT, peel thickness; FPN, filled pods number; OAC, oleic acid content; LAC, linoleic acid content; BAC, behenic acid content; AAC, arachidic acid content; PAC, palmitic acid content; SAC, stearic acid content.
The Axiom_Arachis2 48 K SNP array was used for genotyping the 178 peanut varieties (Nabi et al., 2021). A total of 34,712 SNPs were excluded based on filtering criterion: (1) SNPs with missing data rate (>10%) and (2) minor allele frequency (<5%). After filtration, 13,125 (27.43%) high quality SNPs were obtained (Supplementary File S2). On an average 4.69 SNPs/Mb were found distributed on 20 peanut chromosomes (Arahy.01 to Arahy.20) ranging from 3.74 SNPs/Mb to 7.84 SNPs/Mb (Table 2; Figure 2A). The maximum number of SNPs (800) were found on chromosome Arahy.14, followed by Arahy.01 (797) and Arahy.19 (788). As the smallest chromosome of the peanut genome, Arahy.08 contains only 340 SNPs. The density on chromosome Arahy.07 was the highest density (7.84 SNPs/Mb), while that on chromosome Arahy.12 was the lowest density (3.74 SNPs/Mb) (Table 2).
FIGURE 2. Distribution and types of SNPs. (A) Distribution and density of SNPs in 20 peanut chromosomes. The horizontal axis shows the length of the chromosome (Mb), and the vertical axis represents 20 chromosomes. The shades of assorted color represent the SNP density on corresponding loci. (B) Frequency of several types of SNPs.
A total of six types of SNPs were observed including “T/C”, “A/G”, “A/C”, “T/G”, “C/G”, and “A/T”. We found that “T/C” is the most abundant type of SNPs, accounting for 39.43% of the total SNPs, followed by “A/G” which accounted for 39.05% of the total SNPs (Figure 2B). The “A/C” and “T/G” account for 10.71 and 10.37% of the total SNPs, respectively (Figure 2B). The “C/G” accounts for 0.29% of the total SNPs. The “A/G” is the least type of the SNPs, accounting for only 0.15% of the total SNPs (Figure 2B).
The population structure of the panel of peanut varieties was first investigated with the assessment of K value (Figure 3A), followed by validation via PCA (Figure 3B). The magnitude of CV error suggested that the best K (number of groups) was seven in the model-based group analysis. Based on their genotypes, the peanut panel could be divided into seven groups, group 1 (G1) to group 7 (G7), and the number of peanut varieties per group ranged from 5 to 72. The G1 containing 72 peanut varieties is the biggest group, followed by G6 which contains 53 peanut varieties (Figures 3C,D). Furthermore, the population structure and phylogenetic analysis results also suggested the presence of two subgroups of G1 (G1-1) and G2 (G2-1) (Figures 3C,D). The groups exhibited geographic distribution patterns, and the peanut varieties derived from the same planted areas of origin were usually in the same group. Most of the varieties originating from northern provinces including Shandong, Henan, and Hebei Provinces belong to G1, while the varieties originating from the southern provinces (Fujian, Guangdong, and Guangxi Provinces) were grouped into G6 (Figure 3E).
FIGURE 3. Population structure and genetic diversity of the 178 peanut varieties. (A) Cross-validation value of each K ranging from 1 to 10. (B) The PCA analysis of the total accessions. Each dot represents one variety. (C) Population structure. Each variety is represented with a single vertical line, and the color represents ancestry. (D) Phylogenetic trees constructed by the maximum likelihood method. (E) Geographical distribution of total varieties.
Based on the Q-Q plot analysis, GLM was selected as the best model for GWAS signals among five traits: total number of branches, oil patch, peel thickness, main stem height and testa color; while the MLM was used for the other two traits: hundred seed weight (HSW) and pod shape (Supplementary Figure S1).
Hundred seed weight and the total number of branches are important agronomic traits related to peanut yield. Under the threshold of -log10 > 5.4, MTA related to HSW was detected on chromosome 16 (Figure 4A). In addition, the associated SNPs were identified. The SNP_Chr16:146387758 is located on the 5′UTR of the gene Ahy. 9SIV6F which encodes an unknown function protein. SNP_Chr16:146400676 and SNP_Chr16: 146397542 were all located in the gene region of Ahy.4TTF80, and the latter was in the exon of this gene. Function annotation showed that Ahy.4TTF80 encodes an ABC-2 type transporter (Figure 4A). A previous study has shown that the ABC-2 type transporter protein was related with increasing size of plant seed and content of fat stored within the seed (Kim et al., 2013). For total number of branches, 1 MAT was detected in a 1.79-Mb region of chromosome 5 (97,904,713 to 98,975,592 bp), and 9 associated SNPs were enriched (Figure 4B). Among them, SNP_Chr05:98904713 is in the intron of Ahy.N1NJX0, which is annotated as a calmodulin-binding transcription activator 2-like isoform X1. The other 8 SNPs were all located on intergenic regions (Figure 4B).
FIGURE 4. GWAS signals for hundred seed weight (A) and total number of branches (B) of peanut. The significance level is log10 (0.05/13125) = 5.4 (the gray horizontal line). The characteristic analysis of functional genes in the screening intervals is shown below each Manhattan plot.
The oil patch is the spot in the seed coat of the peanut (Figure 5A). The presence of an oil patch will affect peanut quality; however, the genetic and molecular mechanism of an oil patch are unclear. GWAS analysis showed that 6 SNPs were associated with the oil patch, and all of them were in a 3.88-Mb region of chromosome 5 ranging from chr.05:111.93–115.81 (Figure 5B). SNP_Chr05:111936057 is on the exon of Arahy.7X9WBQ, which encodes a peroxidase superfamily protein. Pod shape, peel thickness, and testa color are also the important appearance traits of peanut. Pod shape is one of the important characteristics for the classification of peanut. A total of 14 MTAs were detected for pod shape, and distributed in Chr2, Chr3, Chr5, Chr8, Chr10, Chr12, Chr13, Chr14, Chr15, Chr16, Chr17, Chr18, and Chr20 (Figure 6A). Among them, the most significant association loci were detected on Chr8 and Chr18 (Figure 6A). For peel thickness, one MAT was detected on a 3.72 -Mb region of chromosome 2 (Chr2:86.18–89.45 Mb) (Figure 6B). For the main stem height, only one SNP was identified on chromosome 6 (Figure 6C).
FIGURE 5. GWAS signals for oil patch (spots) of peanut. (A) Peanut cultivars without an oil patch (LH14) and with an oil patch (JH3). (B) Manhattan plot. The characteristic analysis for one gene encoding peroxidase superfamily protein is shown below.
FIGURE 6. GWAS signals for (A) pod shape, (B) peel thickness, and (C) main stem height, related to the appearance of peanut.
The seed coat (testa) is an important trait of peanut which is not only as an important protective barrier for peanut seed against the pathogen, but also important for health nutrition such as anthocyanins and procyanidins. Testa color is also a complex trait which is controlled by at least 12 genes (Branch, 2011). In this study, the color of the 178 peanut genotypes displayed significant variations, including pink, red, black, purple, white, and variegation (Figure 7A). GWAS analysis showed that the associated SNPs were detected in most of the chromosomes except to Chr.01 and Chr.07 (Figure 7B). Among them, the SNP_AX-177640068 of chr.10 is only 222 kb to the gene AhTc1, one of the key gene controlling black testa identified previously (Zhao et al., 2020). The SNP_AX-176811136 in chr.03 is close to AhRt1 locus contributing to red testa of peanut (Chen et al., 2021) (Figure 7B).
FIGURE 7. Manhattan plots showing significant marker-trait associations for testa color of peanut. (A) Peanuts with different testa color, and its (B) GWAS signal. The characteristic analysis for one gene encoding lysosomal cystine transporter is shown below.
GWAS is a useful method for identifying linked loci and candidate genes by analyzing the association between the genotypes and the phenotypes of individuals in a population. Whole genome resequencing (WGS), high-density SNP array, and target genome resequencing (TGS) are the popular methods for acquiring the high throughput genotyping. WGS is with the maximum coverage across the genome, however, it is more expensive. TGS is a low-cost method that relies on the sequencing of target regions of the genome. Recently, several TGS methods have been developed and used in GWAS analysis, including genotyping by sequencing (GBS), restriction-site associated DNA sequencing (RAD-seq) and specific-locus amplified fragment sequencing (SLAF) (Zhang S. et al., 2019; Dodia et al., 2019; Wang et al., 2019; Yu et al., 2020; Jadhav et al., 2021). The SNP array is a low-cost and wide coverage method. The commercial high-density SNP array chips have developed in many crops, such as 660K of wheat (Hassan et al., 2021), 90K of rice (Yang et al., 2020), and 55K of maize (Li et al., 2021). In peanut, the available genome resource was used for identifying a large number of genome-wide SNPs, and large-scale 58 K SNP array (Axiom_Arachis) (Clevenger et al., 2017; Pandey et al., 2017) and 48K SNP array (Axiom_Arachis2) (Clevenger et al., 2018) have been developed. Axiom_Arachis2 containing 47,837 SNPs is the second generation of peanut gene chip which has been successfully used for genetic diversity analysis and identification of QTLs related with the nutrient elements and leaf spots resistance of peanut (Zhang H. et al., 2019; Zhang et al., 2020a). However, the Axiom_Arachis2 was developed prior to the release of a cultivated peanut genome. The positions of these SNPs were according to the genomes of wild type diploid peanut species, A. duranensis and A. ipaensis. In this study, we used Axiom_Arachis2 to genotype 178 cultivars of peanut, and all of them are tetraploid cultivated peanut. Thus, we first remapped the probe sequences with the genome of cultivated peanut Tifrunner and updated the position information of the SNPs. In total, 45,608 SNPs were mapped in the 20 chromosomes of the peanut genomes and 2229 SNPs were mapped in the scaffolds. In the past few years, the SNP array has shown great potential for mapping the traits on peanut, and the updated position information of these SNPs will provide important references for future utility of the peanut SNP array (Pandey et al., 2021).
As an important index to evaluate seed size, HSW has been one of the hotspots in peanut genetics and QTL mapping. In this study, the HSW of the 178 peanut genotypes displayed wide ranges of variation, ranging from 29.5 to 113.0 g. Our results also showed that HSWs displayed variation within different groups. The G1 represented the varieties from Shandong, Henan, and Hebei Provinces. The average HSW of G1 is 85.1 g, which is significantly heavier than that of G6 (average HSW 66.0 g), in which most of the varieties come from southern provinces of China including Fujian, Guangdong, and Guangxi Provinces. Previous studies have reported many QTLs related with HSW, which is distributed in chr02, 03, 05, 07, 08, 12, 13, 14, 16, 17, and 18. Among them, QTLs in chr16 could be detected at least from four populations, including Fuchuandahuasheng × ICG 637, ZH16 × sd-H1, Zhonghua 16× J11, and Huayu 36 × 6–13, explaining up to 35.39% of the phenotypic variation (Huang et al., 2015; Wang et al., 2018; Mondal and Badigannavar, 2019; Zhang et al., 2019). In this study, the MTAs related with HSW were also detected in chromosome 16, and associated with the candidate gene Ahy.4TTF80, which encodes an ABC-2 type transporter. In tomato, the natural variation of the ABC transporter gene was associated with the seed size (Orsi and Tanksley, 2009). In rice, the ABC transporter gene, OsABCG18 controls the shootward transport of cytokinin and is related with the grain yield of rice (Zhao et al., 2019). We found that there are two SNP substitutes in the Ahy.4TTF80 gene, which provided an important clue for further fine mapped and revealed the key genes controlling the seed size of peanut. Besides, oil patch and pod shape are important agronomic traits of peanut. The oil patch and pod shape affect the appearance and commodity value. However, the physiology and genetics of them are rarely studied. In this study, we identified three MTAs related with the oil patch and pod shape. The details of those selected MTAs were shown in Supplementary Figure S3. These MTAs and candidate genes offer the opportunity to further study the molecular mechanism and improve these traits through the MAS approach.
Cultivated peanut is allotetraploid (AABB, 2n = 4 × = 40), derived from a hybridization event between A. duranensis (AA, 2n = 2 × = 20) and A. ipaensis (BB, 2n = 2 × = 20) about 3500 years ago (Kochert et al., 1996; Lavia et al., 2011). The molecular marker analysis demonstrated that cultivated peanut possesses a narrow genetic base (Halward et al., 1992), and some elite germplasm lines were overused in the peanut breeding program. For example, a previous study showed that more than 70% of peanut cultivars in China derived from two germplasms, Fuhuasheng and Shitouqi, directly or indirectly (Liao, 2004). In this study, a pedigree survey of these 120 peanut germplasms from 13 provinces and regions in China showed that 83 were from Fuhuasheng, accounting for 69.17%, and 42 were from Shitouqi, accounting for 35.00%. Thus, analysis of the genetic relationship between different germplasm resources is especially important for further designing hybrid combinations. In this study, we constructed the phylogenetic tree of these peanut germplasms through the 13,125 polymorphic SNPs. The results suggested that the geographical distribution is not exactly consistent with the genetic relationship among Chinese indigenous peanut breeds, which might be due to the exchange of germplasm resources across China (Figure 3). For example, Yueyou551 (SAAS_015) is classified into G1, however, it is a cultivar from the southern region of China. Pedigree analysis showed that the Yueyou551 derived from the cross-combination of Yueyou 22 and Yueyou 431, and the latter derived from the cross-combination of Shitouqi and Fuhuasheng. Fuhuasheng is a very typical elite peanut germplasm in the north of China. Besides, another two cultivars from the south, Tianfu3 (SAAS_128) and Guihua36 (SAAS_99), also have a close relationship with Fuhuasheng and is classified into G1. In addition, we found two peanut cultivars from Indonesia and one germplasm from Zambia (PI268586) are closely related to peanut varieties in south China, and classified into G6, which might be due to the exchange of germplasms between China and other countries. These results provide an important reference for further use of these germplasms.
In this study, we analyzed 20 phenotypic traits of 178 peanut germplasms and genotyped them using the 48 K Axiom_Arachis2 SNP array. We analyzed the genetic diversity of these cultivars and identified a number of MTAs related to different traits. The candidate SNPs and candidate genes for these MTAs are helpful for further fine mapping and improving the quality and yield of peanut via a molecular breeding method.
The datasets presented in this study can be found in online repositories. The names of the repository/repositories and accession number(s) can be found below: NCBI's GEO database, accession number GSE197103.
XW, CZ, RT, and CM conceived and designed the experiments. HZ, XZ, JM, HH, and KZ performed the experiments. CZ, RT, HX, and HZ analyzed data. CZ, RT, HX, HZ, and MT wrote and revised the manuscript. All authors read and approved the manuscript.
This research is supported by Key Research and Development Project of Shandong Province (2020LZGC001), Shandong Province Natural Science Foundation (ZR2020MC105), National Natural Science Foundation of China (32072090, 31861143009, and 31801301), agricultural scientific and technological innovation project of Shandong Academy of Agricultural Sciences (CXGC2021B05, CXGC2021B24, and CXGC2021A30), High-level Foreign Experts Introduction Program (GL20200123001), and Taishan Scholar Project of Shandong Province (ts20190964).
The authors declare that the research was conducted in the absence of any commercial or financial relationships that could be construed as a potential conflict of interest.
All claims expressed in this article are solely those of the authors and do not necessarily represent those of their affiliated organizations, or those of the publisher, the editors, and the reviewers. Any product that may be evaluated in this article, or claim that may be made by its manufacturer, is not guaranteed or endorsed by the publisher.
The Supplementary Material for this article can be found online at: https://www.frontiersin.org/articles/10.3389/fgene.2022.845602/full#supplementary-material
Alexander, D. H., Novembre, J., and Lange, K. (2009). Fast Model-Based Estimation of Ancestry in Unrelated Individuals. Genome Res. 19, 1655–1664. doi:10.1101/gr.094052.109
Bertioli, D. J., Jenkins, J., Clevenger, J., Dudchenko, O., Gao, D., Seijo, G., et al. (2019). The Genome Sequence of Segmental Allotetraploid Peanut Arachis H. Nat. Genet. 51, 877–884. doi:10.1038/s41588-019-0405-z
Bradbury, P. J., Zhang, Z., Kroon, D. E., Casstevens, T. M., Ramdoss, Y., and Buckler, E. S. (2007). TASSEL: Software for Association Mapping of Complex Traits in Diverse Samples. Bioinformatics 23, 2633–2635. doi:10.1093/bioinformatics/btm308
Branch, W. D. (2011). First 100 Years - Inheritance of Testa Color in Peanut (Arachis HypogaeaL.). Crop Sci. 51, 1–4. doi:10.2135/cropsci2010.06.0349
Chen, H., Chen, X., Xu, R., Liu, W., Liu, N., Huang, L., et al. (2021). Fine-Mapping and Gene Candidate Analysis for AhRt1, a Major Dominant Locus Responsible for Testa Color in Cultivated Peanut. Theor. Appl. Genet. 134, 3721–3730. doi:10.1007/s00122-021-03924-w
Chu, Y., Wu, C. L., Holbrook, C. C., Tillman, B. L., Person, G., and Ozias-Akins, P. (2011). Marker-Assisted Selection to Pyramid Nematode Resistance and the High Oleic Trait in Peanut. The plant genome 4, 110–117. doi:10.3835/plantgenome2011.01.0001
Clevenger, J., Chu, Y., Chavarro, C., Agarwal, G., Bertioli, D. J., Leal-Bertioli, S. C. M., et al. (2017). Genome-Wide SNP Genotyping Resolves Signatures of Selection and Tetrasomic Recombination in Peanut. Mol. Plant 10, 309–322. doi:10.1016/j.molp.2016.11.015
Clevenger, J. P., Korani, W., Ozias-Akins, P., and Jackson, S. (2018). Haplotype-Based Genotyping in Polyploids. Front. Plant Sci. 9, 564. doi:10.3389/fpls.2018.00564
Collard, B. C. Y., and Mackill, D. J. (2007). Marker-Assisted Selection: An Approach for Precision Plant Breeding in the Twenty-First century. Phil. Trans. R. Soc. B 363, 557–572. doi:10.1098/rstb.2007.2170
Dodia, S. M., Joshi, B., Gangurde, S. S., Thirumalaisamy, P. P., Mishra, G. P., Narandrakumar, D., et al. (2019). Genotyping-by-Sequencing Based Genetic Mapping Reveals Large Number of Epistatic Interactions for Stem Rot Resistance in Groundnut. Theor. Appl. Genet. 132, 1001–1016. doi:10.1007/s00122-018-3255-7
Gangurde, S. S., Wang, H., Yaduru, S., Pandey, M. K., Fountain, J. C., Chu, Y., et al. (2020). Nested-Association Mapping (NAM)-Based Genetic Dissection Uncovers Candidate Genes for Seed and Pod Weights in Peanut (Arachis Hypogaea). Plant Biotechnol. J. 18, 1457–1471. doi:10.1111/pbi.13311
Halward, T., Stalker, T., LaRue, E., and Kochert, G. (1992). Use of Single-Primer DNA Amplifications in Genetic Studies of Peanut (Arachis hypogaea L.). Plant Mol. Biol. 18, 315–325. doi:10.1007/BF00034958
Han, S., Yuan, M., Clevenger, J. P., Li, C., Hagan, A., Zhang, X., et al. (2018). A SNP-Based Linkage Map Revealed QTLs for Resistance to Early and Late Leaf Spot Diseases in Peanut (Arachis Hypogaea L.). Front. Plant Sci. 9, 1012. doi:10.3389/fpls.2018.01012
Hassan, M. A., Yang, M., Rasheed, A., Tian, X., Reynolds, M., Xia, X., et al. (2021). Quantifying Senescence in Bread Wheat Using Multispectral Imaging from an Unmanned Aerial Vehicle and QTL Mapping. Plant Physiol. 187, 2623–2636. doi:10.1093/plphys/kiab431
Huang, L., He, H., Chen, W., Ren, X., Chen, Y., Zhou, X., et al. (2015). Quantitative Trait Locus Analysis of Agronomic and Quality-Related Traits in Cultivated Peanut (Arachis H L.). Theor. Appl. Genet. 128, 1103–1115. doi:10.1007/s00122-015-2493-1
Jadhav, M. P., Gangurde, S. S., Hake, A. A., Yadawad, A., Mahadevaiah, S. S., Pattanashetti, S. K., et al. (2021). Genotyping-by-Sequencing Based Genetic Mapping Identified Major and Consistent Genomic Regions for Productivity and Quality Traits in Peanut. Front. Plant Sci. 12, 668020. doi:10.3389/fpls.2021.668020
Kim, S., Lee, Y., Nishida, I., Yamaoka, Y., and Ono, H. (2013). Composition Containing Gene Encoding Abc Transporter Proteins for Increasing Size of Plant Seed and Content of Fat Stored within Seed. US, US20130283481 A1.
Kochert, G., Stalker, H. T., Gimenes, M., Galgaro, L., Lopes, C. R., and Moore, K. (1996). RFLP and Cytogenetic Evidence on the Origin and Evolution of Allotetraploid Domesticated Peanut, Arachis Hypogaea (Leguminosae). Am. J. Bot. 83, 1282–1291. doi:10.1002/j.1537-2197.1996.tb13912.x
Lavia, G. I., Ortiz, A. M., Robledo, G., Fernández, A., and Seijo, G. (2011). Origin of Triploid Arachis Pintoi (Leguminosae) by Autopolyploidy Evidenced by FISH and Meiotic Behaviour. Ann. Bot. 108, 103–111. doi:10.1093/aob/mcr108
Letunic, I., and Bork, P. (2021). Interactive Tree of Life (iTOL) V5: An Online Tool for Phylogenetic Tree Display and Annotation. Nucleic Acids Res. 49, W293–W296. doi:10.1093/nar/gkab301
Li, S., Zhang, C., Yang, D., Lu, M., Qian, Y., Jin, F., et al. (2021). Detection of QTNs for Kernel Moisture Concentration and Kernel Dehydration Rate before Physiological Maturity in maize Using Multi-Locus GWAS. Sci. Rep. 11, 1764. doi:10.1038/s41598-020-80391-1
Liao, B. (2004). Present Situation and prospect of Peanut Genetic Improvement in China. J. Agr. Sci. Tech-Iran. 6, 60–62.
Luo, H., Pandey, M. K., Khan, A. W., Guo, J., Wu, B., Cai, Y., et al. (2018). Discovery of Genomic Regions and Candidate Genes Controlling Shelling Percentage Using QTL‐seq Approach in Cultivated Peanut (Arachis Hypogaea L.). Plant Biotechnol. J. 17, 1248–1260. doi:10.1111/pbi.13050
Ma, J., Zhao, Y., Chen, H., Fu, C., Zhu, L., Zhou, X., et al. (2020). Genome-Wide Development of Polymorphic Microsatellite Markers and Their Application in Peanut Breeding Program. Electron. J. Biotechnol. 44, 25–32. doi:10.1016/j.ejbt.2020.01.004
Minh, B. Q., Schmidt, H. A., Chernomor, O., Schrempf, D., Woodhams, M. D., von Haeseler, A., et al. (2020). Corrigendum to: IQ-TREE 2: New Models and Efficient Methods for Phylogenetic Inference in the Genomic Era. Mol. Biol. Evol. 37, 2461. doi:10.1093/molbev/msaa131
Mondal, S., and Badigannavar, A. M. (2019). Identification of Major Consensus QTLs for Seed Size and Minor QTLs for Pod Traits in Cultivated Groundnut (Arachis Hypogaea L.). 3 Biotech. 9, 347. doi:10.1007/s13205-019-1881-7
Nabi, R. B. S., Cho, K.-S., Tayade, R., Oh, K. W., Lee, M. H., Kim, J. I., et al. (2021). Genetic Diversity Analysis of Korean Peanut Germplasm Using 48 K SNPs 'Axiom_Arachis' Array and its Application for Cultivar Differentiation. Sci. Rep. 11, 16630. doi:10.1038/s41598-021-96074-4
Orsi, C. H., and Tanksley, S. D. (2009). Natural Variation in an ABC Transporter Gene Associated with Seed Size Evolution in Tomato Species. Plos Genet. 5, e1000347. doi:10.1371/journal.pgen.1000347
Pandey, M. K., Agarwal, G., Kale, S. M., Clevenger, J., Nayak, S. N., Sriswathi, M., et al. (2017). Development and Evaluation of a High Density Genotyping 'Axiom_Arachis' Array with 58 K SNPs for Accelerating Genetics and Breeding in Groundnut. Sci. Rep. 7, 40577. doi:10.1038/srep40577
Pandey, M. K., Gangurde, S. S., Sharma, V., Pattanashetti, S. K., Naidu, G. K., Faye, I., et al. (2021). Improved Genetic Map Identified Major QTLs for Drought Tolerance- and Iron Deficiency Tolerance-Related Traits in Groundnut. Genes 12 (1), 37. doi:10.3390/genes12010037
Pandey, M. K., Pandey, A. K., Kumar, R., Nwosu, C. V., Guo, B., Wright, G. C., et al. (2020). Translational Genomics for Achieving Higher Genetic Gains in Groundnut. Theor. Appl. Genet. 133, 1679–1702. doi:10.1007/s00122-020-03592-2
Pinto da Silva, G. B., Zanella, C. M., Martinelli, J. A., Chaves, M. S., Hiebert, C. W., McCallum, B. D., et al. (2018). Quantitative Trait Loci Conferring Leaf Rust Resistance in Hexaploid Wheat. Phytopathology 108, 1344–1354. doi:10.1094/PHYTO-06-18-0208-RVW
Pujar, M., Gangaprasad, S., Govindaraj, M., Gangurde, S. S., Kanatti, A., and Kudapa, H. (2020). Genome-Wide Association Study Uncovers Genomic Regions Associated with Grain Iron, Zinc and Protein Content in Pearl Millet. Sci. Rep. 10, 19473. doi:10.1038/s41598-020-76230-y
Qureshi, N., Bariana, H., Kumran, V. V., Muruga, S., Forrest, K. L., Hayden, M. J., et al. (2018). A New Leaf Rust Resistance Gene Lr79 Mapped in Chromosome 3BL from the Durum Wheat Landrace Aus26582. Theor. Appl. Genet. 131, 1091–1098. doi:10.1007/s00122-018-3060-3
Shah, L., Rehman, S., Ali, A., Yahya, M., Riaz, M. W., Si, H., et al. (2018). Genes Responsible for Powdery Mildew Resistance and Improvement in Wheat Using Molecular Marker-Assisted Selection. J. Plant Dis. Prot. 125, 145–158. doi:10.1007/s41348-017-0132-6
Shasidhar, Y., Variath, M. T., Vishwakarma, M. K., Manohar, S. S., Gangurde, S. S., Sriswathi, M., et al. (2020). Improvement of Three Popular Indian Groundnut Varieties for Foliar Disease Resistance and High Oleic Acid Using SSR Markers and SNP Array in Marker-Assisted Backcrossing. Crop J. 8, 1–15. doi:10.1016/j.cj.2019.07.001
Varshney, R. K., Mohan, S. M., Gaur, P. M., Gangarao, N. V. P. R., Pandey, M. K., Bohra, A., et al. (2013). Achievements and Prospects of Genomics-Assisted Breeding in Three Legume Crops of the Semi-Arid Tropics. Biotechnol. Adv. 31, 1120–1134. doi:10.1016/j.biotechadv.2013.01.001
Wang, J., Yan, C., Li, Y., Li, C., Zhao, X., Yuan, C., et al. (2019). GWAS Discovery of Candidate Genes for Yield-Related Traits in Peanut and Support from Earlier QTL Mapping Studies. Genes 10, 803. doi:10.3390/genes10100803
Wang, Z., Huai, D., Zhang, Z., Cheng, K., Kang, Y., Wan, L., et al. (2018). Development of a High-Density Genetic Map Based on Specific Length Amplified Fragment Sequencing and its Application in Quantitative Trait Loci Analysis for Yield-Related Traits in Cultivated Peanut. Front. Plant Sci. 9, 827. doi:10.3389/fpls.2018.00827
Yang, Y., Xu, X., Zhang, M., Xu, Q., Feng, Y., Yuan, X., et al. (2020). Genetic Basis Dissection for Eating and Cooking Qualities of Japonica rice in Northeast China. Agronomy 10, 423. doi:10.3390/agronomy10030423
Yu, B., Jiang, H., Pandey, M. K., Huang, L., Huai, D., Zhou, X., et al. (2020). Identification of Two Novel Peanut Genotypes Resistant to Aflatoxin Production and Their SNP Markers Associated with Resistance. Toxins 12, 156. doi:10.3390/toxins12030156
Yu, S. (2008). Chinese Peanut Cultivar and Pedigree. Shanghai: Shanghai Scientific Technology Press.
Zhang, H., Chu, Y., Dang, P., Tang, Y., Jiang, T., Clevenger, J. P., et al. (2020a). Identification of QTLs for Resistance to Leaf Spots in Cultivated Peanut (Arachis Hypogaea L.) through GWAS Analysis. Theor. Appl. Genet. 133, 2051–2061. doi:10.1007/s00122-020-03576-2
Zhang, H., Li Wang, M., Dang, P., Jiang, T., Zhao, S., Lamb, M., et al. (2020b). Identification of Potential QTLs and Genes Associated with Seed Composition Traits in Peanut (Arachis Hypogaea L.) Using GWAS and RNA-Seq Analysis. Gene 769, 145215. doi:10.1016/j.gene.2020.145215
Zhang, H., Wang, M. L., Schaefer, R., Dang, P., Jiang, T., and Chen, C. (2019). GWAS and Coexpression Network Reveal Ionomic Variation in Cultivated Peanut. J. Agric. Food Chem. 67, 12026–12036. doi:10.1021/acs.jafc.9b04939
Zhang, S., Hu, X., Miao, H., Chu, Y., Cui, F., Yang, W., et al. (2019). QTL Identification for Seed Weight and Size Based on a High-Density SLAF-Seq Genetic Map in Peanut (Arachis Hypogaea L.). BMC Plant Biol. 19, 537. doi:10.1186/s12870-019-2164-5
Zhao, C., Qiu, J., Agarwal, G., Wang, J., Ren, X., Xia, H., et al. (2017). Genome-Wide Discovery of Microsatellite Markers from Diploid Progenitor Species, Arachis Duranensis and A. Ipaensis, and Their Application in Cultivated Peanut (A. Hypogaea). Front. Plant Sci. 8, 1209. doi:10.3389/fpls.2017.01209
Zhao, J., Yu, N., Ju, M., Fan, B., Zhang, Y., Zhu, E., et al. (2019). ABC Transporter OsABCG18 Controls the Shootward Transport of Cytokinins and Grain Yield in rice. J. Exp. Bot. 70, 6277–6291. doi:10.1093/jxb/erz382
Zhao, Y., Ma, J., Li, M., Deng, L., Li, G., Xia, H., et al. (2020). Whole-Genome Resequencing-Based QTL-Seq Identified AhTc1 Gene Encoding a R2R3-MYB Transcription Factor Controlling Peanut Purple Testa Colour. Plant Biotechnol. J. 18, 96–105. doi:10.1111/pbi.13175
Zhou, X., Guo, J., Pandey, M. K., Varshney, R. K., Huang, L., Luo, H., et al. (2021). Dissection of the Genetic Basis of Yield-Related Traits in the Chinese Peanut Mini-Core Collection through Genome-Wide Association Studies. Front. Plant Sci. 12, 637284. doi:10.3389/fpls.2021.637284
Keywords: peanut, GWAS, SNP array, molecular markers, agronomic traits
Citation: Zhao H, Tian R, Xia H, Li C, Li G, Li A, Zhang X, Zhou X, Ma J, Huang H, Zhang K, Thudi M, Ma C, Wang X and Zhao C (2022) High-Density Genetic Variation Map Reveals Key Candidate Loci and Genes Associated With Important Agronomic Traits in Peanut. Front. Genet. 13:845602. doi: 10.3389/fgene.2022.845602
Received: 30 December 2021; Accepted: 22 February 2022;
Published: 25 March 2022.
Edited by:
Ahmed Sallam, Assiut University, EgyptReviewed by:
Sunil S. Gangurde, University of Georgia, United StatesCopyright © 2022 Zhao, Tian, Xia, Li, Li, Li, Zhang, Zhou, Ma, Huang, Zhang, Thudi, Ma, Wang and Zhao. This is an open-access article distributed under the terms of the Creative Commons Attribution License (CC BY). The use, distribution or reproduction in other forums is permitted, provided the original author(s) and the copyright owner(s) are credited and that the original publication in this journal is cited, in accordance with accepted academic practice. No use, distribution or reproduction is permitted which does not comply with these terms.
*Correspondence: Xingjun Wang, eGluZ2p1bndAaG90bWFpbC5jb20=; Chuanzhi Zhao, Y2h1YW56aGl6QDEyNi5jb20=
†These authors have contributed equally to this work and share first authorship
Disclaimer: All claims expressed in this article are solely those of the authors and do not necessarily represent those of their affiliated organizations, or those of the publisher, the editors and the reviewers. Any product that may be evaluated in this article or claim that may be made by its manufacturer is not guaranteed or endorsed by the publisher.
Research integrity at Frontiers
Learn more about the work of our research integrity team to safeguard the quality of each article we publish.