- Centre for Agricultural Bioinformatics, ICAR-Indian Agricultural Statistics Research Institute, PUSA, New Delhi, India
Cereals are the most important food crops and are considered key contributors to global food security. Loss due to abiotic stresses in cereal crops is limiting potential productivity in a significant manner. The primary reasons for abiotic stresses are abrupt temperature, variable rainfall, and declining nutrient status of the soil. Varietal development is the key to sustaining productivity under influence of multiple abiotic stresses and must be studied in context with genomics and molecular breeding. Recently, advances in a plethora of Next Generation Sequencing (NGS) based methods have accelerated the enormous genomic data generation associated with stress-induced transcripts such as microarray, RNAseq, Expressed Sequenced Tag (ESTs), etc. Many databases related to microarray and RNA-seq based transcripts have been developed and profusely utilized. However, an abundant amount of transcripts related to abiotic stresses in various cereal crops arising from EST technology are available but still remain underutilized in absence of a consolidated database. In this study, an attempt has been made with a primary goal to integrate, analyse, and characterise the available resources of ESTs responsive to abiotic stresses in major cereals. The developed CerealESTdb presents a customisable search in two different ways in the form of searchable content for easy access and potential use. This database comprises ESTs from four major cereal crops, namely rice (Oryza sativa L.), wheat (Triticum aestivum L.), sorghum (Sorghum bicolour L.), and maize (Zea mays L.), under a set of abiotic stresses. The current statistics of this cohesive database consists of 55,826 assembled EST sequences, 51,791 predicted genes models, and their 254,609 gene ontology terms including extensive information on 1,746 associated metabolic pathways. We anticipate that developed CerealESTdb will be helpful in deciphering the knowledge of complex biological phenomena under abiotic stresses to accelerate the molecular breeding programs towards the development of crop cultivars resilient to abiotic stresses. The CerealESTdb is publically available with the URL http://cabgrid.res.in/CerealESTDb.
Introduction
To provide more than nine billion people by 2050 with essential nutrients and energy in their everyday diet, food production must be increased by 70% with reduced fertilizer inputs (Wani et al., 2021; Kumar et al., 2018; Balyan et al., 2016). Crop productivity suffers due to multiple abiotic stresses during the crop life cycle. The important four cereal crops, namely rice (Oryza sativa L.), wheat (Triticum aestivum L.), sorghum (Sorghum bicolour L.), and maize (Zea mays L.) show sensitivity to a wide range of abiotic stress viz., abscisic acid (ABA), cold, drought, heat, and salt (Askari-Khorasgani et al., 2021; Cramer et al., 2011). In crop plants, mitigation mechanisms against these environmental stresses are complex biological processes. Although, there is a surge of biological information on plant responses to abiotic stresses, the understanding of the molecular mechanisms involved is still enigmatic (Zhang et al., 2021; Wani et al., 2018; Gahlaut et al., 2016). The mining of candidate genes and gene families responsive to multiple abiotic stresses from whole-genome sequencing data facilitates the molecular understanding of the complex cellular and molecular processes in a significant manner. Also, functional and structural annotations of genes and gene families will provide insight into the complex interactions regulating stress responses (Takahashi et al., 2020; Atkinson et al., 2013). Recently, advances in high throughput omics technologies have accelerated the identification and characterization of novel genes and their biological functions in a tissue-specific manner. At the genome and transcriptome level, the pathway reconstruction will play a principal role in quantifying and characterising the genotype to phenotype relationship (Gupta et al., 2020; Zhang et al., 2020; Feist and Palsson, 2008). In this ongoing genomic era, a huge amount of genomic data have been generated and analysed to provide key information leading to the development of crop cultivars resilient to abiotic stresses (Alter et al., 2015). This includes various transcriptomic data of gene expression such as Expressed Sequence Tags (EST), microarray, RNAseq etc. (Vincent et al., 2013; Zeng and Extavour, 2012). Whlie the era of Next Generation Sequencing (NGS) generates a huge amount of transcriptome data, previously generated EST resources still hold an important position. EST sequence has been defined as a short genomic sub-sequence and is important for the identification of gene transcripts under different environmental conditions. It can provide a means of accessing the gene space of almost any organism (Bouck and Vision, 2007; Nagaraj et al., 2007). EST libraries are a cost-effective tool and can serve as a starting point for the development of effective molecular genetic markers, such as gene-linked microsatellites, single nucleotide polymorphisms, etc. However, these EST resources are too scattered to provide insight into the biological process including the involvement of genes/regulatory elements and their functional role in responding to different abiotic stresses. Though the process of generation of EST data is historical, simple, cost-effective, and abundantly available it largely remains underutilised due to the scarce availability of proper databases. Efforts were made in the past by several research groups related to the development of EST resources in cereals such as Gramene (Jaiswal, 2011), cerealsDB (Wilkinson et al., 2012), Wheat Genome (Lai et al., 2012), MaizeGDB (Lawrence et al., 2004), Plant Stress Gene Database (Prabha et al., 2011), and Uniprot (Apweiler et al., 2004) summarized in Table 1.
Therefore, an attempt has been made to develop a user friendly, searchable, and interactive database of assembled and annotated ESTs for major cereal crops i.e., maize, rice, sorghum, and wheat along with associated genes and metabolic pathways involved in abiotic stresses viz., ABA, cold, drought, heat and salt. In order to develop this database, these abiotic stresses related ESTs of considered cereal crops were collected from various sources and assembled. Subsequently, genes were identified and putative genes were predicted and further, these genes were functionally annotated using bioinformatics tools (Bhati et al., 2016; Chaduvula et al., 2015; Bhati et al., 2014). Partial amplification of nine identified candidate genes related to salinity stress response genes was standardised and carried out in Oryza sativa var. indica IR64 (Bhati et al., 2016), and 12 candidate genes were selected for salinity stress and amplified in nine indigenous Sorghum bicolor genotypes (Chaduvula et al., 2015). Moreover, information associated with various metabolic pathways involving these genes was identified and collected. This extracted and derived information was stored in relational database management systems (RDBMS) and made available through a searchable web interface as CerealESTdb which is unique in terms of its contents and features. This database provides various search options to retrieve the desired information in a few clicks and selections. This database contains 51,791 genes, 254,609 gene ontology terms, and 1,746 pathways. The primary goal of this database is to serve as a central data repository of processed and annotated EST resources of major cereal crops under abiotic stress conditions. The database is accessible from http://cabgrid.res.in/CerealESTDb.
Materials and Methods
Source of Data and Functional Annotation
The EST sequences (ESTs) were searched and downloaded from National Centre for Biotechnology Information (NCBI) (www.ncbi.nlm.nih.gov) by using various combinations of terms crop name, the scientific name of crop, and stress name for four important cereal crops viz., maize, rice, sorghum, and wheat and five abiotic stresses viz., abscisic acid (ABA), cold, drought, heat, and salt stresses (Figure 1). These EST sequences were re-checked with the writing of suitable Perl scripts and filtered out all non-related EST sequences. The remaining EST sequences were also masked to eliminate the incorrect part in these sequences and then finally assembled using EGAssembler (https://www.genome.jp/tools/egassembler/) by considering the default parameter settings and the similarity-based clustering was set to 80 percent overlap identity (Masoudi-Nejad et al., 2006). These assembled EST-contigs were then translated to reading frames. The BLASTx algorithm was applied against the protein sequence database (NCBI nr) for the identification of potential translated products (Altschul et al., 1990). The homologous EST-contigs with annotated proteins that exists in the nr database were selected for functional annotations based on maximum E-value (1E−3) and the minimum alignment size of high scoring pair (HSP) length of 33. Blast2GO v 2.5 was employed to perform the functional analysis of the EST-contigs (Conesa and Gotz, 2008; Gotz et al., 2008). Further, the EST-contigs were categorized according to the GO vocabularies into three categories i.e. molecular function, biological process, and cellular component. These EST-contigs were mapped to the Kyoto Encyclopedia of Genes and Genomes (KEGG) database (Kanehisa and Goto, 2000) and attained the corresponding metabolic pathways. A flowchart depicting the pipeline for above-described process is presented in Figure 2.
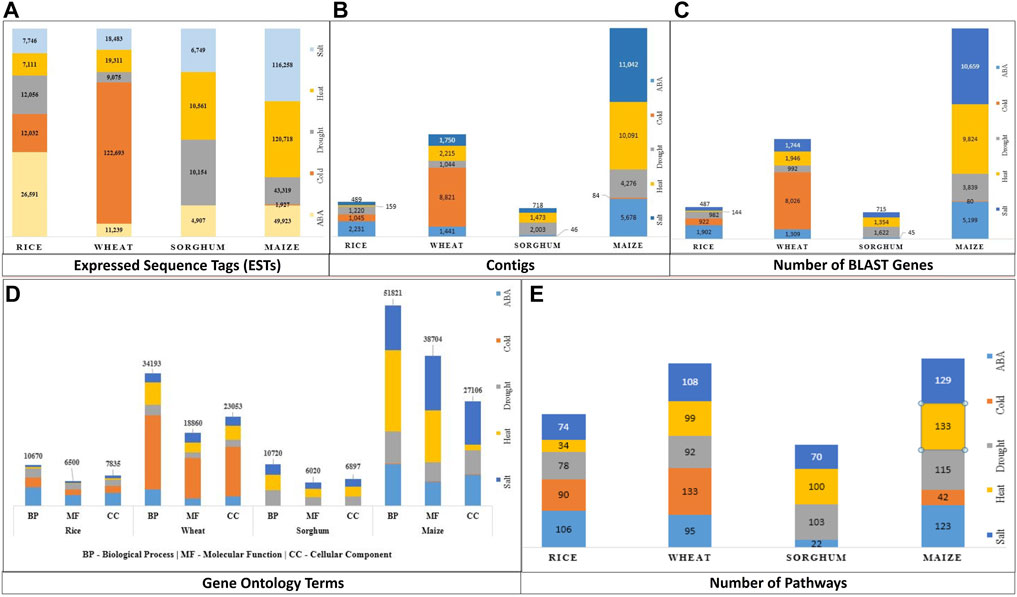
FIGURE 1. Data Summary—(A) ESTs, (B) Annotated contigs along with their corresponding (C) BLAST genes, (D) GO term, and (E) Pathway (GO: Gene Ontology, BP, Biological process; MF, Molecular Function; CC, Cellular Components).
Software Architecture and Database Design
The CerealESTDb has been implemented using standard 3-tier web architecture (Lee et al., 2009) followed to develop this web application. Different components of CerealESTDb architecture are presented in Figure 3. Access to this database has been provided by developing an end-user interface using HyperText Markup Language (HTML), cascading style sheets (CSS), and javascript. The search request will be submitted to a web server i.e., apache-tomcat, and processed by corresponding servlets created using Java Server Pages (JSP) and javascript. These servlets fetched the data from the developed RDBMS in MySQL using Java Database Connectivity (JDBC) technology to process and send the response to the end user’s web browser.
The database has been implemented using MySQL and contained different information related to crop, stress, gene name, gene ontology, enzyme, and pathway. The design of the database has followed the normalization procedure of a relational database management system to maintain data consistency and avoid data redundancy in the database. The database comprises tables for crop, stress, contigs, gene ontology, enzyme, pathway, contigs_pathway, and pathway_enzyme. The entity-relationship (ER) diagram of this database is mentioned in Supplementary Figure S1.
User Interface of CerealESTDb
The CerealESTDb comprises of Home, About, Search, Advance Search, Important Link, Contact Us, and Help. The “Home” page describes the database and its possible use in a molecular breeding program. The “About” page describes the process workflow used for extracting and processing the data, starting from collection of data to annotation of the genes and finally populating into the database. Various search options provide the facility to extract the stored information in two ways namely, “Search” (Supplementary Figure S2A) and “Advance Search” (Supplementary Figure S2B).
Several options have been provided in the web interface to enable a fast and efficient search for the end-user to retrieve the desired results with and meet the varied requirements of end users in terms of selecting various attributes. The users have the option to enter the free text in the search box or select the required values from dropdown lists. After selecting or entering the values for various input boxes, the users will click on the “Submit” button to obtain the required results. After clicking on the “Submit” button, the selected and entered values are converted into suitable Structured Query Language (SQL) format and fetched the results from the database and delivered the results in easy to use format to the end users’ browser window.
Results and Discussion
Out of 610,893 ESTs downloaded for abiotic stresses viz., ABA, cold, drought, heat, and salt across all four crops viz., maize, rice, sorghum, and wheat, only 9% were assembled into contigs (55,826 in number). ESTs for cold stress in sorghum were not assembled because EGAssembler requires a minimum of 64 EST sequences and there were only 40 ESTs for cold stress in sorghum. There were a total of 51,791 genes that were annotated by BLAST out of 55,826 contigs across all the abiotic stresses. The total number of the assembled contigs and corresponding annotated BLAST genes against the nr database of NCBI, GO terms, and pathways are shown in Figure 1. The options to select the desired attributes have also been provided to the users and avoided the unnecessary attributes from the database that save the query response time too. Some of the important attribute names are selected by default and users have the option to either select or ignored other attributes, if needed. These results can further be combined with identified GO terms and associated pathways if any. The identified pathways will be helpful for retrieving the enzymatic information and hyperlink to pathways that can be further visualized for additional information or relationships. The users can also download the result in.xls format to the user’s desktop for later use. The above description is summarized in Figure 4 for a better understating of the retrieval process.
Stress-wise annotated genes are also represented using pie charts for the cereal crops under study (Figure 5A). Figure 5B shows the percentage of GO terms in different crops across these five abiotic stresses. The GO terms were further classified into three categories, biological process (BP), molecular function (MF), and cellular component (CC). The number of biological process-related GO terms are largest for each stress in all crops, followed by cellular component and molecular function, except in salt stress of maize, rice, and wheat.
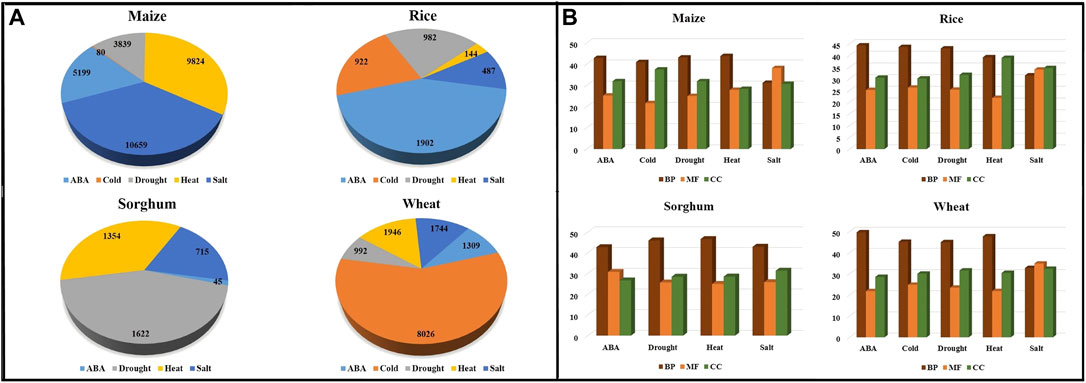
FIGURE 5. Distribution of (A) annotated contigs across different abiotic stresses in the crops. (B) GO terms—Biological Process (BP), Molecular Function (MF), and Cellular Component (CC) across abiotic stress in crops.
Many plant genes have their expression modulated by stress conditions. The common genes in all crops and conditions were auxin-binding protein/auxin response factor (Guo et al., 2018), CCT motif transcription factor (Li and Xu, 2017), heat-shock proteins (Jacob et al., 2017; Shanker el at., 2012), Ser/Thr protein kinase (Islam et al., 2013), ribosomal proteins (Moin et al., 2017), and zinc-finger proteins (Zang et al., 2016). The small auxin upregulated RNA (SAUR) is a plant-specific gene family of auxin-responsive genes. As evident from previous reports, the overexpression of these SAUR genes contributed to the tolerance to drought and salt in Arabidopsis (Guo et al., 2018). Auxin-response factor proteins have been well studied in crop plants for their direct involvement in response to multiple stresses (Stortenbeker and Bemer, 2019). The heat-shock proteins play key roles in protecting plant cells or tissues from various abiotic stresses (Wen et al., 2017). Other common genes such as 14-3-3 genes, cytochrome c/b/p450, ubiquitin-like protein, etc., have been identified in the present study. The 14-3-3 genes are involved in various abiotic stresses of different plant species such as rice (Chen et al., 2006; Chen et al., 2019), Populus (Tian et al., 2015), Phaseolus vulgaris L. (Li et al., 2015), and Hevea brasiliensis (Yang et al., 2014), as reported by previous studies.
The maximum number of GO terms is pertaining to response to stress/defense response in all abiotic stresses for all considered plant species. Moreover, some GO terms are specific to the abiotic stress like Abscisic acid stimulus/signalling/recovery, drought response, response to heat, salt, and cold.
Further, there were 1,746 KEGG pathways associated with the annotated genes in all crops for these five stresses. The sugar metabolism pathway is the most common pathway in all cereal crops under study. Sugars are chemically active biomolecules and are involved in crucial Physico-chemical mechanisms such as photosynthesis, respiration, seed germination, flowering, senescence, and so forth. Therefore, modulating sugar composition or concentration in plants may improve their responses or adaptation to abiotic stress (Gangola and Ramadoss, 2018). The other common pathways were carbon fixation in photosynthetic organisms, purine metabolism, and metabolic pathways. Evidence suggests that certain purine metabolites like allantoin and allantoate, products of purine metabolism contribute to stress tolerance in plants (Casartelli et al., 2019; Watanabe et al., 2014).
The user interface of this database has been developed to provide access to the compiled resources in the form of CerealESTDb and important links have been made for Home, About CerealESTDb, Search, Advance Search, Important Links, Contact Us, and Help. There are two types of search options developed in the user interface in the form of “Search” and “Advanced Search”. The first option allows the user to select crop or stress or a combination of crop and associated stress (Figure 6A).
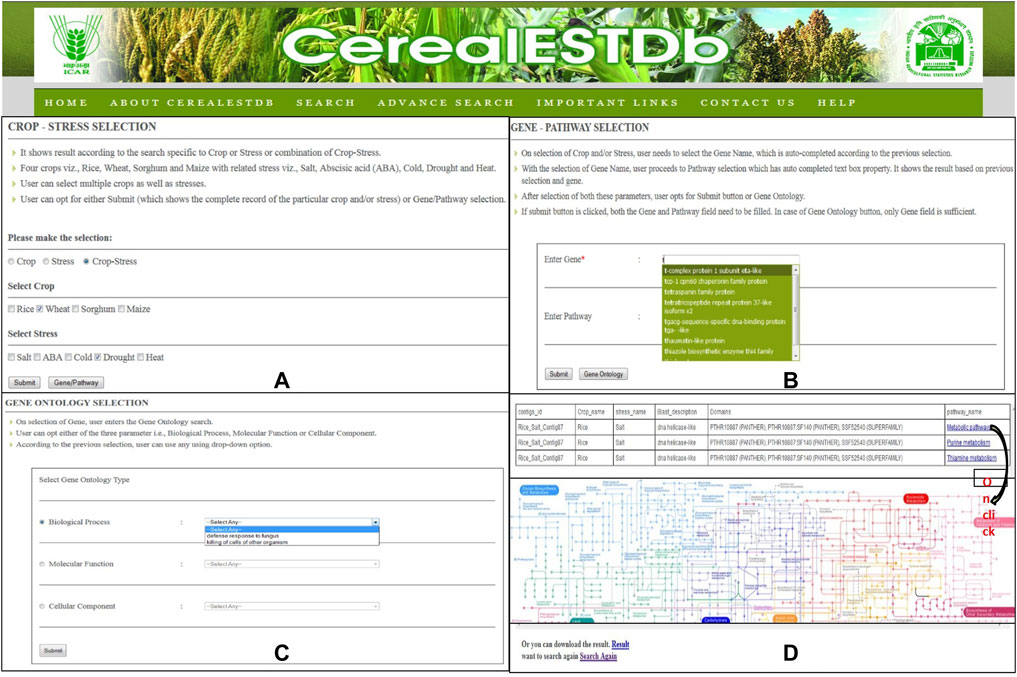
FIGURE 6. Details of Search Options in CerealESTDb (A) Crop-Stress Selection page with options of select either crop or stress or combination of both (B) Gene-Pathway Selection page with mandatory field Gene and optional field Pathway (C) Gene Ontology Selection page, to select either of the three options of Biological Process or Molecular Function or Cellular Component (D) Final Result page after going through all the three selection pages with pathway image (on click).
The users have the option to either click on “Submit” or “Genes/Pathway”. If a user clicks on “Submit,” it will further ask users to select the list of attributes or parameters from available attributes (i.e., Crop Name, Stress Name, Gene Name, Sequence, Sequence length, e-value, similarity, and domains). After selecting the desired attributes, the required information will be displayed to the users and this information can also be downloaded and exported in.xls format for further analysis. Another option is to select genes and corresponding pathways for selected Crop/Stress/Crop-Stress and further choose the required attributes for additional information of the selected combination (Figure 6B). After selecting the gene/pathway option, it is compulsory to enter the gene name to proceed to the next level of search to retrieve the desired results.
Further, the users have the option to search by specifying “Gene Ontology” terms related to biological process, molecular function, and cellular component (Figure 6C). The results under each selection will be displayed to the end-user along with a pathway map (Figure 6D). The user can download these results in.xls format and the corresponding pathway map can also be saved as.gif.
The “Advanced Search” option provides users the ability to directly search information from this searchable database by selecting a specific gene name (Figure 7A), pathway name (Figure 7B), or gene ontology type (Figure 7C). The user is then asked to select the combination of crop name and stress names (Figure 7D). The relevant tabular information is displayed to the user which can be downloaded in.xls format (Figure 7E). In order to make the search user-friendly, the gene name, pathway name, and ontology type will be selected through a drop-down menu displayed from the database to the user after typing the first few letters of these in the search options.
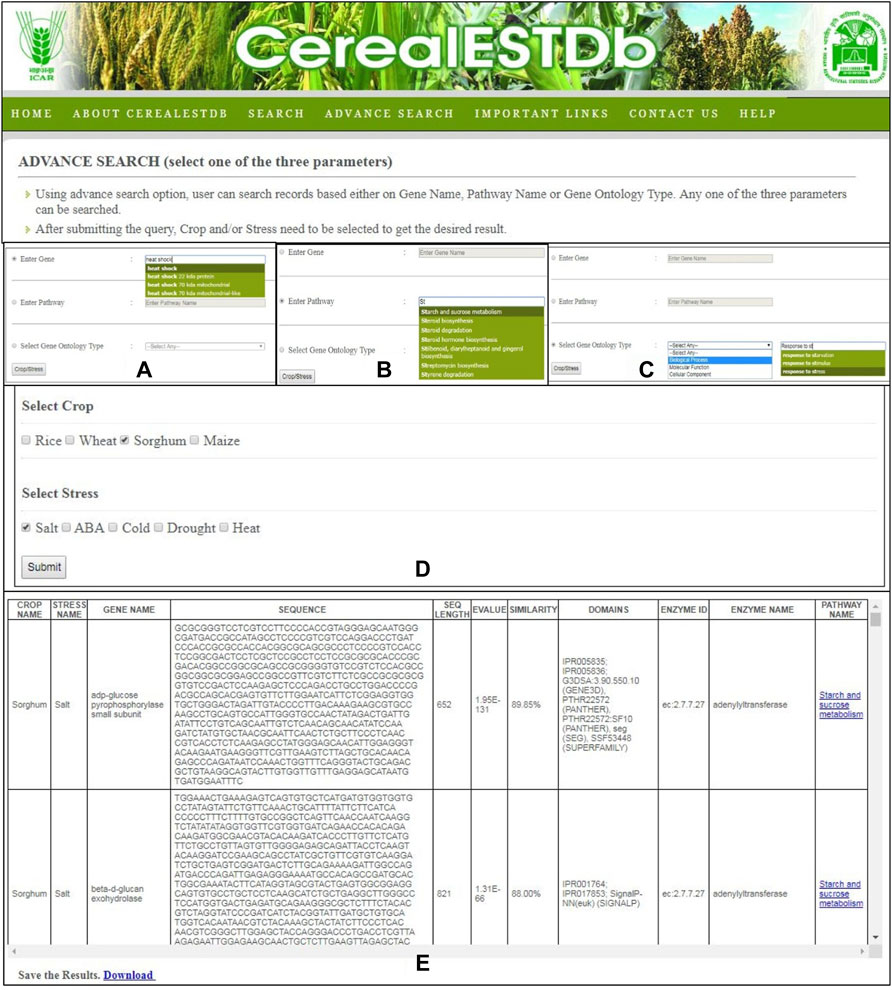
FIGURE 7. Details of Advance Search options of CerealESTDb. The search could be done through three options (A) Gene name search or (B) Pathway name search or (C) Gene ontology term search, to select either of the three options of Biological Process or Molecular Function or Cellular Component and their corresponding values (D) Common Crop-Stress Selection page with options of select either crop or stress or combination of both (E) Final Result page after selecting either of the three options.
“Important Link” helps the user find the web pages of relevant organizations and databases. “Contact Us” gives contact details of research team members and “Help” provides steps and flow of various search routes of this database.
The features of the CerealESTDb are:
a. User-friendly, searchable, and interactive data resource for searching a set of genomic information associated with response to abiotic stresses in cereal crops.
b. Provides a unique platform for interactive retrieval of annotated genes, GO terms, and corresponding generated metabolic pathways.
c. Provides alternate ways to explore 51,791 genes, 254,609 GO terms, and 1,746 generated metabolic pathways
d. Provides a facility to users to export results in.xls format for further annotations.
The few advantages of the present database are given below:
a. The developed database will provide the desired information related to search queries even without knowing anything specific to search query criteria and also facilitate the users through selection.
b. In this database, an effort has been made to collect various ESTs related to four important cereal crops across five specific abiotic stresses. A pipeline has been developed to mine the genes/proteins and that can also be applied in other plant species to annotate the ESTs and gene models.
c. The present database provides extensive information on metabolic pathways and extracted GO information with the help of BLAST2Go tool specific to particular abiotic stress i.e. ABA, cold, drought, heat, or salt-related to considered cereal crops.
Conclusion
The CerealESTdb, a user-friendly, searchable, and interactive database has been developed with a primary goal to provide information on assembled and annotated ESTs from four major crop plants, namely wheat, rice, maize, and sorghum under multiple environmental stresses including cold, heat, drought, and salt stress as well as on the application of ABA. This cohesive database will also provide extensive information about genes, ontology, and corresponding metabolic pathways related to these ESTs. The CerealESTdb also facilitates users to access a description of the biological functions of genes involved in more than one related abiotic stress. This database will help in providing new solutions to molecular biologists and plant breeders for accelerating their efforts to develop abiotic stress-resistant cultivars without compromising the nutritional quality through introgression and gene pyramiding. With further progress in NGS methods and the availability of genome sequences data in the public domain, we hope that statistics of CerealESTdb will eventually grow by two-to-three folds in upcoming years.
Data Availability Statement
The original contributions presented in the study are included in the article/Supplementary Materials, further inquiries can be directed to the corresponding author.
Author Contributions
All authors contributed to study design. JB did the data collection, data analysis, and data interpretation. KC, SL, JB, AS, and PP designed the database. SK, KC and JB wrote the manuscript draft. All authors contributed to discussing and writing the final text.
Funding
This research was funded by the project “Network Project for Agricultural Bioinformatics and Computational Biology under CABin Scheme,” ICAR and project “Modeling Network of Gene Responses to Abiotic Stress in Rice,” funded by NASF, ICAR.
Conflict of Interest
The authors declare that the research was conducted in the absence of any commercial or financial relationships that could be construed as a potential conflict of interest.
The reviewer UK declared a past co-authorship with the author AK to the handling editor.
Publisher’s Note
All claims expressed in this article are solely those of the authors and do not necessarily represent those of their affiliated organizations, or those of the publisher, the editors and the reviewers. Any product that may be evaluated in this article, or claim that may be made by its manufacturer, is not guaranteed or endorsed by the publisher.
Supplementary Material
The Supplementary Material for this article can be found online at: https://www.frontiersin.org/articles/10.3389/fgene.2022.842868/full#supplementary-material
Supplementary Figure S1 | Entity-Relationship Diagram
Supplementary Figure S2 | Step-wise options followed in (A) simple Search page and (B) Advance Search page
References
Alter, S., Bader, K. C., Spannagl, M., Wang, Y., Bauer, E., Schön, C.-C., et al. (2015). DroughtDB: an Expert-Curated Compilation of Plant Drought Stress Genes and Their Homologs in Nine Species. Database 2015. doi:10.1093/database/bav046
Altschul, S. F., Gish, W., Miller, W., Myers, E. W., and Lipman, D. J. (1990). Basic Local Alignment Search Tool. J. Mol. Biol. 215 (3), 403–410. doi:10.1016/S0022-2836(05)80360-2
Apweiler, R., Bairoch, A., Wu, C. H., Barker, W. C., Boeckmann, B., Ferro, S., et al. (2004). UniProt: the Universal Protein Knowledgebase. Nucleic Acids Res. 32 (Suppl. l_1), 115D–119D. doi:10.1093/nar/gkh131
Askari-Khorasgani, O., Rehmani, M. I. A., Wani, S. H., and Kumar, A. (2021). Osmotic Stress. In Handbook of Plant and Crop Physiology, 4. New York: CRC Press, 445–464. doi:10.1201/9781003093640
Atkinson, N. J., Lilley, C. J., and Urwin, P. E. (2013). Identification of Genes Involved in the Response of Arabidopsis to Simultaneous Biotic and Abiotic Stresses. Plant Physiol. 162 (4), 2028–2041. doi:10.1104/pp.113.222372
Balyan, H. S., Gahlaut, V., Kumar, A., Jaiswal, V., Dhariwal, R., Tyagi, S., et al. (2016). Nitrogen and Phosphorus Use Efficiencies in Wheat: Physiology, Phenotyping, Genetics, and Breeding. Plant Breed. Rev. 40, 167–234. doi:10.1002/9781119279723.ch4
Bhati, J., Chaduvula K, P., Rai, A., Gaikwad, K., Soma Marla, S., and Kumar, S. (2016). In-silico Prediction and Functional Analysis of Salt Stress Responsive Genes in rice (Oryza Sativa). J. Rice Res. 4 (164), 2. doi:10.4172/2375-4338.1000164
Bhati, J., Chaduvula, P. K., Rani, R., Kumar, S., and Rai, A. (2014). In-silico Prediction and Functional Analysis of Salt Stress Responsive Genes in maize (Zea mays). Eur. J. Mol. Biol. Biochem. 1, 151–157.
Bouck, A., and Vision, T. (2007). The Molecular Ecologist's Guide to Expressed Sequence Tags. Mol. Ecol. 16 (5), 907–924. doi:10.1111/j.1365-294x.2006.03195.x
Casartelli, A., Melino, V. J., Baumann, U., Riboni, M., Suchecki, R., Jayasinghe, N. S., et al. (2019). Opposite Fates of the Purine Metabolite Allantoin under Water and Nitrogen Limitations in Bread Wheat. Plant Mol. Biol. 99 (4), 477–497. doi:10.1007/s11103-019-00831-z
Chaduvula, P. K., Bhati, J., Rai, A., Gaikwad, K., Marla, S. S., Elangovan, M., et al. (2015). In-silico' Expressed Sequence Tag Analysis in Identification and Characterization of Salinity Stress Responsible Genes in 'Sorghum Bicolor. Aust. J. Crop Sci. 9 (9), 799–806.
Chen, F., Li, Q., Sun, L., and He, Z. (2006). The rice 14-3-3 Gene Family and its Involvement in Responses to Biotic and Abiotic Stress. DNA Res. 13 (2), 53–63. doi:10.1093/dnares/dsl001
Chen, Y.-S., David Ho, T.-H., Liu, L., Lee, D. H., Lee, C.-H., Chen, Y.-R., et al. (2019). Sugar Starvation-Regulated MYBS2 and 14-3-3 Protein Interactions Enhance Plant Growth, Stress Tolerance, and Grain Weight in rice. Proc. Natl. Acad. Sci. USA 116 (43), 21925–21935. doi:10.1073/pnas.1904818116
Conesa, A., and Götz, S. (2008). Blast2GO: A Comprehensive Suite for Functional Analysis in Plant Genomics. Int. J. Plant Genomics 2008, 1–12. doi:10.1155/2008/619832
Cramer, G. R., Urano, K., Delrot, S., Pezzotti, M., and Shinozaki, K. (2011). Effects of Abiotic Stress on Plants: a Systems Biology Perspective. BMC Plant Biol. 11, 163. doi:10.1186/1471-2229-11-163
Feist, A. M., and Palsson, B. Ø. (2008). The Growing Scope of Applications of Genome-Scale Metabolic Reconstructions Using Escherichia coli. Nat. Biotechnol. 26 (6), 659–667. doi:10.1038/nbt1401
Gahlaut, V., Jaiswal, V., Kumar, A., and Gupta, P. K. (2016). Transcription Factors Involved in Drought Tolerance and Their Possible Role in Developing Drought Tolerant Cultivars with Emphasis on Wheat (Triticum aestivum L.). Theor. Appl. Genet. 129 (11), 2019–2042. doi:10.1007/s00122-016-2794-z
Gangola, M. P., and Ramadoss, B. R. (2018). “Sugars Play a Critical Role in Abiotic Stress Tolerance in Plants,” in Biochemical, Physiological and Molecular Avenues for Combating Abiotic Stress Tolerance in Plants (Academic Press), 17–38. doi:10.1016/b978-0-12-813066-7.00002-4
Götz, S., García-Gómez, J. M., Terol, J., Williams, T. D., Nagaraj, S. H., Nueda, M. J., et al. (2008). High-throughput Functional Annotation and Data Mining with the Blast2GO Suite. Nucleic Acids Res. 36 (10), 3420–3435. doi:10.1093/nar/gkn176
Guo, Y., Jiang, Q., Hu, Z., Sun, X., Fan, S., and Zhang, H. (2018). Function of the Auxin-Responsive Gene TaSAUR75 under Salt and Drought Stress. Crop J. 6 (2), 181–190. doi:10.1016/j.cj.2017.08.005
Gupta, A., Rico-Medina, A., and Caño-Delgado, A. I. (2020). The Physiology of Plant Responses to Drought. Science 368, 266–269. doi:10.1126/science.aaz7614
Islam, M. R., Hosen, M. I., Zaman, A., and Islam, M. O. (2013). Structural, Functional and Molecular Docking Study to Characterize GMI1 from Arabidopsis thaliana. Interdiscip. Sci. Comput. Life Sci. 5 (1), 13–22. doi:10.1007/s12539-013-0153-1
Jacob, P., Hirt, H., and Bendahmane, A. (2017). The Heat-Shock Protein/chaperone Network and Multiple Stress Resistance. Plant Biotechnol. J. 15 (4), 405–414. doi:10.1111/pbi.12659
Jaiswal, P. (2011). Gramene Database: a Hub for Comparative Plant Genomics. Methods Mol. Biol. 678, 247–275. doi:10.1007/978-1-60761-682-5_18
Kanehisa, M., and Goto, S. (2000). KEGG: Kyoto Encyclopedia of Genes and Genomes. Nucl. Acids Res. 28 (1), 27–30. doi:10.1093/nar/28.1.27
Kumar, A., Pandeya, A., Malik, G., Sharma, M., P., H. K., S., A. K., et al. (2018). A Web Resource for Nutrient Use Efficiency-Related Genes, Quantitative Trait Loci and microRNAs in Important Cereals and Model Plants. F1000Res 7, 673. doi:10.12688/f1000research.14561.1
Lai, K., Berkman, P. J., Lorenc, M. T., Duran, C., Smits, L., Manoli, S., et al. (2012). WheatGenome.info: An Integrated Database and Portal for Wheat Genome Information. Plant Cel Physiol. 53 (2), e2. doi:10.1093/pcp/pcr141
Lawrence, C. J., Dong, Q., Polacco, M. L., Seigfried, T. E., and Brendel, V. (2004). MaizeGDB, the Community Database for maize Genetics and Genomics. Nucleic Acids Res. 32 (Suppl. l_1), 393D–397D. doi:10.1093/nar/gkh011
Lee, D.-Y., Saha, R., Yusufi, F. N. K., Park, W., and Karimi, I. A. (2009). Web-based Applications for Building, Managing and Analysing Kinetic Models of Biological Systems. Brief. Bioinform. 10 (1), 65–74. doi:10.1093/bib/bbn039
Li, R., Jiang, X., Jin, D., Dhaubhadel, S., Bian, S., and Li, X. (2015). Identification of 14-3-3 Family in Common Bean and Their Response to Abiotic Stress. PLoS One 10 (11), e0143280. doi:10.1371/journal.pone.0143280
Li, Y., and Xu, M. (2017). CCT Family Genes in Cereal Crops: A Current Overview. Crop J. 5 (6), 449–458. doi:10.1016/j.cj.2017.07.001
Masoudi-Nejad, A., Tonomura, K., Kawashima, S., Moriya, Y., Suzuki, M., Itoh, M., et al. (2006). EGassembler: Online Bioinformatics Service for Large-Scale Processing, Clustering and Assembling ESTs and Genomic DNA Fragments. Nucleic Acids Res. 34 (Suppl. l_2), W459–W462. doi:10.1093/nar/gkl066
Moin, M., Bakshi, A., Madhav, M. S., and Kirti, P. B. (2017). Expression Profiling of Ribosomal Protein Gene Family in Dehydration Stress Responses and Characterization of Transgenic rice Plants Overexpressing RPL23A for Water-Use Efficiency and Tolerance to Drought and Salt Stresses. Front. Chem. 5, 97. doi:10.3389/fchem.2017.00097
Nagaraj, S. H., Deshpande, N., Gasser, R. B., and Ranganathan, S. (2007). ESTExplorer: an Expressed Sequence Tag (EST) Assembly and Annotation Platform. Nucleic Acids Res. 35 (Suppl. l_2), W143–W147. doi:10.1093/nar/gkm378
Prabha, R., Ghosh, I., and Singh, D. P. (2011). Plant Stress Gene Database: A Collection of Plant Genes Responding to Stress Condition. ARPN J. Sci. Tech. 1, 28–31.
Shanker, A. K., Maddaala, A., Kumar, M. A., Yadav, S. K., Maheswari, M., and Venkateswarlu, B. (2012). In Silico targeted Genome Mining and Comparative Modelling Reveals a Putative Protein Similar to an Arabidopsis Drought Tolerance DNA Binding Transcription Factor in Chromosome 6 of Sorghum Bicolor Genome. Interdiscip. Sci. Comput. Life Sci. 4 (2), 133–141. doi:10.1007/s12539-012-0121-1
Stortenbeker, N., and Bemer, M. (2019). TheSAURgene Family: the Plant's Toolbox for Adaptation of Growth and Development. J. Exp. Bot. 70 (1), 17–27. doi:10.1093/jxb/ery332
Takahashi, F., Kuromori, T., Urano, K., Yamaguchi-Shinozaki, K., and Shinozaki, K. (2020). Drought Stress Responses and Resistance in Plants: from Cellular Responses to Long-Distance Intercellular Communication. Front. Plant Sci. 11, 556972. doi:10.3389/fpls.2020.556972
Tian, F., Wang, T., Xie, Y., Zhang, J., and Hu, J. (2015). Genome-wide Identification, Classification, and Expression Analysis of 14-3-3 Gene Family in Populus. PLoS One 10 (4), e0123225. doi:10.1371/journal.pone.0123225
Vincent, J., Dai, Z., Ravel, C., Choulet, F., Mouzeyar, S., Bouzidi, M. F., et al. (2013)dbWFA: a Web-Based Database for Functional Annotation of Triticum aestivum Transcripts. Database 2013. doi:10.1093/database/bat014
Wani, S. H., Khan, H., Riaz, A., Joshi, D. C., Hussain, W., Rana, M., et al. (2022). “Genetic Diversity for Developing Climate-Resilient Wheats to Achieve Food Security Goals,” in Advances in Agronomy (Academic Press), 255–303. doi:10.1016/bs.agron.2021.08.006
Wani, S. H., Tripathi, P., Zaid, A., Challa, G. S., Kumar, A., Kumar, V., et al. (2018). Transcriptional Regulation of Osmotic Stress Tolerance in Wheat (Triticum aestivum L.). Plant Mol. Biol. 97 (6), 469–487. doi:10.1007/s11103-018-0761-6
Watanabe, S., Matsumoto, M., Hakomori, Y., Takagi, H., Shimada, H., and Sakamoto, A. (2014). The Purine Metabolite Allantoin Enhances Abiotic Stress Tolerance through Synergistic Activation of Abscisic Acid Metabolism. Plant Cel Environ 37 (4), 1022–1036. doi:10.1111/pce.12218
Wen, F., Wu, X., Li, T., Jia, M., Liu, X., Li, P., et al. (2017). Genome-wide Survey of Heat Shock Factors and Heat Shock Protein 70s and Their Regulatory Network under Abiotic Stresses in Brachypodium Distachyon. PLoS One 12 (7), e0180352. doi:10.1371/journal.pone.0180352
Wilkinson, P. A., Winfield, M. O., Barker, G. L., Allen, A. M., Burridge, A., Coghill, J. A., et al. (2012). CerealsDB 2.0: an Integrated Resource for Plant Breeders and Scientists. BMC Bioinformatics 13 (1), 1–6. doi:10.1186/1471-2105-13-219
Yang, Z.-P., Li, H.-L., Guo, D., Tang, X., and Peng, S.-Q. (2014). Identification and Characterization of the 14-3-3 Gene Family in Hevea Brasiliensis. Plant Physiol. Biochem. 80, 121–127. doi:10.1016/j.plaphy.2014.03.034
Zang, D., Li, H., Xu, H., Zhang, W., Zhang, Y., Shi, X., et al. (2016). An Arabidopsis Zinc finger Protein Increases Abiotic Stress Tolerance by Regulating Sodium and Potassium Homeostasis, Reactive Oxygen Species Scavenging and Osmotic Potential. Front. Plant Sci. 7, 1272. doi:10.3389/fpls.2016.01272
Zeng, V., and Extavour, C. G. (20122012). ASGARD: an Open-Access Database of Annotated Transcriptomes for Emerging Model Arthropod Species. Database 2012, bas048. doi:10.1093/database/bas048
Zhang, H., Zhao, Y., and Zhu, J.-K. (2020). Thriving under Stress: How Plants Balance Growth and the Stress Response. Develop. Cel 55, 529–543. doi:10.1016/j.devcel.2020.10.012
Keywords: cereals, abiotic stress, ests, genes, pathways, ontology
Citation: Kumar S, Bhati J, Saha A, Lal SB, Pandey PK, Mishra DC, Farooqi MS, Kumar A, Chaturvedi KK and Rai A (2022) CerealESTDb: A Comprehensive Resource for Abiotic Stress-Responsive Annotated ESTs With Predicted Genes, Gene Ontology, and Metabolic Pathways in Major Cereal Crops. Front. Genet. 13:842868. doi: 10.3389/fgene.2022.842868
Received: 24 December 2021; Accepted: 21 January 2022;
Published: 24 February 2022.
Edited by:
Ramsey Steven Lewis, North Carolina State University, United StatesReviewed by:
Upendra Kumar, Chaudhary Charan Singh Haryana Agricultural University, IndiaRomesh Kumar Salgotra, Sher-e-Kashmir University of Agricultural Sciences and Technology, India
Shumayla, Panjab University, India
Copyright © 2022 Kumar, Bhati, Saha, Lal, Pandey, Mishra, Farooqi, Kumar, Chaturvedi and Rai. This is an open-access article distributed under the terms of the Creative Commons Attribution License (CC BY). The use, distribution or reproduction in other forums is permitted, provided the original author(s) and the copyright owner(s) are credited and that the original publication in this journal is cited, in accordance with accepted academic practice. No use, distribution or reproduction is permitted which does not comply with these terms.
*Correspondence: Sanjeev Kumar, sanjeevbunty@gmail.com
†These authors have contributed equally to this work and share first authorship