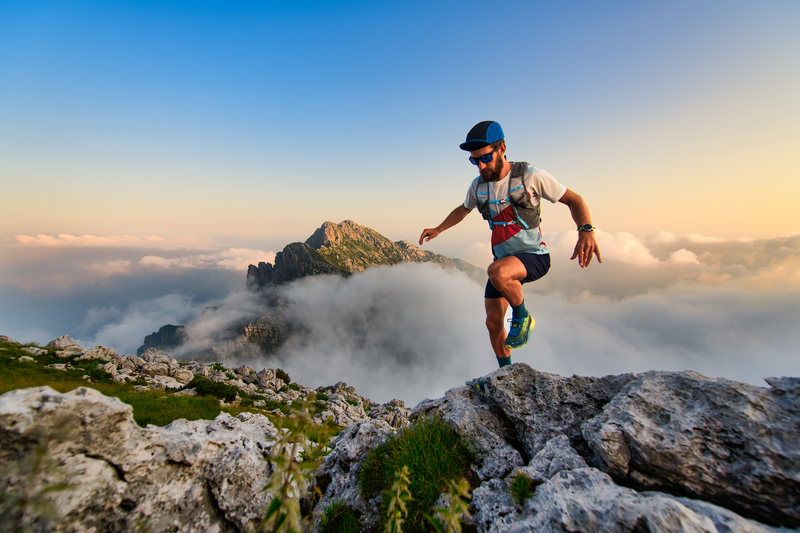
94% of researchers rate our articles as excellent or good
Learn more about the work of our research integrity team to safeguard the quality of each article we publish.
Find out more
ORIGINAL RESEARCH article
Front. Genet. , 19 July 2022
Sec. Cancer Genetics and Oncogenomics
Volume 13 - 2022 | https://doi.org/10.3389/fgene.2022.838624
This article is part of the Research Topic Genetic targets for cancer immunotherapy View all 14 articles
Pyroptosis, defined as programmed cell death, results in the release of inflammatory mediators. Recent studies have revealed that pyroptosis plays essential roles in antitumor immunity and immunotherapy efficacy. Long noncoding RNAs (lncRNAs) are involved in a variety of biological behaviors in tumor cells, although the roles and mechanisms of lncRNAs in pyroptosis are rarely studied. Our study aimed to establish a novel pyroptosis-related lncRNA signature as a forecasting tool for predicting prognosis and ascertaining immune value. Based on lung adenocarcinoma (LUAD) patients from The Cancer Genome Atlas (TCGA), we performed Pearson’s correlation analysis to identify pyroptosis-related lncRNAs. After differentially expressed gene analysis and univariate Cox regression analysis, we selected prognosis-related and differentially expressed lncRNAs. Finally, we performed multivariate Cox regression analysis to establish the three pyroptosis-related lncRNA signature. Kaplan–Meier (KM) survival analyses and receiver operating characteristic (ROC) curves indicated the excellent performance for predicting the prognosis of LUAD patients. At the same time, we applied multidimensional approaches to further explore the functional enrichment, tumor microenvironment (TME) landscape, and immunotherapy efficacy among the different risk groups. A nomogram was constructed by integrating risk scores and clinical characteristics, which was validated using calibrations and ROC curves. Three lncRNAs, namely, AC090559.1, AC034102.8, and AC026355.2, were involved in this signature and used to classify LUAD patients into low- and high-risk groups. Overall survival time (OS) was higher in the low-risk group than in the high-risk group, which was also validated in our LUAD cohort from Shandong Provincial Hospital. TME landscape analyses revealed that a higher abundance of infiltrating immune cells and a greater prevalence of immune-related events existed in the low-risk group. Meanwhile, higher expression of immune checkpoint (ICP) genes, higher immunophenoscore (IPSs), and greater T cell dysfunction in the low-risk group demonstrated a better response to immunotherapy than the high-risk group. Combined with predictions from the Tumor Immune Dysfunction and Exclusion (TIDE) website, we found that LUAD patients in the low-risk group significantly benefited from programmed cell death 1 (PD-1) and cytotoxic T-lymphocyte–associated protein 4 (CTLA4) immune checkpoint blockade (ICB) therapy compared with those in the high-risk group. Furthermore, drug susceptibility analysis identified potential sensitive chemotherapeutic drugs for each risk group. In this study, a novel three pyroptosis-related lncRNA signature was constructed, which could accurately predict the immunotherapy efficacy and prognosis in LUAD patients.
Lung cancer is currently the leading cause of cancer-related death worldwide, and its 5-year survival rate varies from 4% to 17% depending on stage and regional differences (Hirsch et al., 2017; Siegel et al., 2021). Lung adenocarcinoma (LUAD), as the most common subtype of lung cancer, accounts for approximately 40% of lung cancer cases (Denisenko et al., 2018). Despite the tremendous progress in a variety of treatment strategies, the survival rate of LUAD remains low. Therefore, there is an urgent need of developing accurate and reliable biomarkers for effective prognosis prediction of LUAD.
Pyroptosis is a novel programmed inflammatory cell death mediated by gasdermin proteins (GSDMs) (Yu P. et al., 2021; Deets and Vance, 2021). The activation of caspases-1/4/5/11 by inflammasomes mediates the cleavage of GSDMs, which results in the rupture of cell membranes and release of intracellular proinflammatory substances such as interleukin-1 β (IL-1 β) and interleukin-18 (IL-18). This is followed by a strong inflammatory response that is triggered in the immune microenvironment (Rathinam and Fitzgerald, 2016). In cancer, the complex effects of pyroptosis are dependent on genetic characteristics, which vary across different tissues. Several studies have reported that pyroptosis can suppress tumorigenesis, and even if only a few tumor cells undergo pyroptosis, a strong inflammatory response is triggered to recruit immune cells and enhance T cell-mediated antitumor immunity (Wang et al., 2020; Zhang et al., 2020). Another study has reported that pyroptosis also creates a tumor microenvironment (TME), which was a requirement of tumor growth (Zhang et al., 2021). Nevertheless, the mechanisms of action and effects of pyroptosis are still largely unknown in LUAD.
Long noncoding RNAs (lncRNAs) are transcripts longer than 200 nucleotides that are encoded by the genome but usually not translated into proteins (Bhan et al., 2017). Recent studies have reported that lncRNAs can regulate pyroptosis in a variety of ways such as targeting microRNAs (miRNA) and directly or indirectly binding to pyroptosis-associated proteins (Evavold, Hafner-Bratkovic et al., 2021). As lncRNAs can remain stable in the blood, which is easily collected from patients, they have good prospects as prognostic or predictive markers that are radiosensitive, chemosensitive, and sensitive to target therapy (Chen et al., 2021).
With the development of next-generation sequencing, various biomarkers have been identified to construct signatures for subgroup classification and prognosis prediction (Seijo et al., 2019; Lazzari et al., 2020). However, due to the lack of effective subgroup classification and prognostic prediction models, LUAD patients remain to be undertreated. Therefore, the construction of accurate subgroup classification and prognostic prediction models is urgently needed to guide clinicians on chemotherapy and immunotherapy.
In this study, we aimed to develop a prognostic risk model based on pyroptosis-related lncRNAs in LUAD patients. Based on LUAD patients from The Cancer Genome Atlas (TCGA), we constructed and validated a prognostic risk model to accurately predict the prognosis and overall survival (OS) of LUAD patients, which consisted of three pyroptosis-related lncRNAs. The risk score was significantly associated with tumor-infiltrating immune cells, immune function, and immunotherapy response. Our study revealed the potential connection and mechanism of action between pyroptosis, TME, and immunotherapy response.
Gene transcriptome profiling data, mutation data, and corresponding clinical information of LUAD patients were downloaded from (https://portal.gdc.cancer.gov/). Fragments per kilobase of exon model per million mapped fragments (FPKM) were converted to
A total of 45 LUAD patient specimens were recruited at Shandong Provincial Hospital, Shandong, China. The biomedical research ethic committee of Shandong Provincial Hospital approved this study (SWYX: NO. 2021-433).
We identified 33 pyroptosis-related genes from the literature, as listed in Supplementary Table S1 (Deets and Vance, 2021; Liu et al., 2021; Ye et al., 2021). The R software (R, vision 4.1.0) package “limma” (Ritchie et al., 2015) was used to determine the differently expressed pyroptosis-related genes with the absolute log2-fold change (
Pyroptosis-related lncRNAs were extracted using Pearson’s correlation analysis between pyroptosis-related genes and lncRNAs (correlation coefficient >0.60, p < 0.001, and false-discovery rate (FDR) < 0.05).
We extracted differently expressed pyroptosis-related lncRNAs with an
The Kaplan–Meier plotter (https://kmplot.com/analysis/) was used to analyze the impact of co-expressed mRNAs, while TIMER2.0 (Li et al., 2020) (http://timer.cistrome.org/) and gene expression profiling integrative analysis (Tang et al., 2017) (GEPIA, http://gepia.cancer-pku.cn/) were used for differential expression analysis of co-expressed mRNAs.
According to the prognostic-related lncRNAs, multivariate Cox regression analysis was performed to identify the best prognostic signature, which consisted of three lncRNAs, namely, AC090559.1, AC026355.2, and AC034102.8. We calculated the risk score as follows: risk score = (−0.2603×AC090559.1) + (−0.0974×AC026355.2) + (−0.9235×AC034102.8). Based on the median of risk score, the LUAD patients from the TCGA database were divided into high- and low-risk groups for further analysis. Subsequently, risk score distribution maps, survival status maps, and lncRNA expression heat maps were plotted. Kaplan–Meier analysis was applied to compare the overall survival (OS) of the two groups. The time receiver operating characteristic (ROC) curve was plotted using the “timeROC” package with R software, which was used to evaluate the predictive capability of the risk model. The area under the curve (AUC) of the constructed risk model in predicting the OS of LUAD patients was compared with several previously published lncRNA signatures, including the ferroptosis-related lncRNA signature of Lu (Lu et al., 2021), hypoxia-associated lncRNA signature of Shao (Shao et al., 2021), autophagy-related lncRNA signature of Liu (Liu and Yang, 2021), and six-lncRNA-based prognostic signatures of Yang (Yang et al., 2021).
The prognostic significance of the risk score and other clinical characteristics was evaluated by using univariate and multivariate Cox regression analyses. A nomogram was established to predict the 1-, 3-, and 5-year OS, which consisted of the risk score, age, and stage. Calibration curves were plotted to assess the accuracy of the risk model.
GO enrichment analysis was applied to investigate the potential pathways of the differentially expressed pyroptosis genes using “org.Hs.eg.db,” “clusterProfiler,” and “enrichplot” modules within the R package. By applying the “GSVA” tool, GSVA enrichment analysis was performed based on hallmark gene sets extracted from the MSigDB database (Hänzelmann et al., 2013).
Single-sample gene set enrichment analysis (ssGSEA) was performed to compare the difference in abundance of 28 types of infiltrating immune cells, 13 immune functions, and 13 other tumor-related biological processes, which were extracted from previous articles (Şenbabaoğlu et al., 2016; Charoentong et al., 2017; Mariathasan et al., 2018). In addition, we used computational methods to assess the infiltrating immune cells, including the TIMER (Li et al., 2020), CIBERSORT (Chen et al., 2018), quanTIseq (Finotello et al., 2019), MCP-counter (Becht et al., 2016), xCell (Aran et al., 2017), EPIC (Racle et al., 2017), and TIDE (Jiang et al., 2018) algorithms. Immune score and tumor purity were calculated using the “ESTIMATE” tool within the R package (Chakraborty and Hossain, 2018). In addition, correlation analysis was performed using the correlation heat map tool in HiPlot (https://hiplot.com.cn), a comprehensive web platform for scientific data visualization.
The immunophenoscore (IPS) was obtained from The Cancer Immunome Atlas (https://tcia.at/). Information on the dysfunction and exclusion of infiltrating cytotoxic T lymphocytes (CTLs) was downloaded from the Tumor Immune Dysfunction and Exclusion (TIDE) website (http://tide.dfci.harvard.edu/). In addition, TIDE was used to evaluate patients who received a benefit or no benefit from ICB therapy through the comprehensive biomarkers of the ICB response in different groups (Fu et al., 2020).
Following the manufacturer’s protocol, total RNA was extracted from clinical specimens using AG RNAex Pro Reagent (Accurate Biotechnology (Hunan) Co., Ltd., China). The Evo M-MLVRT Master Mix kit (Accurate Biotechnology (Hunan) Co., Ltd., China) was used for reverse transcription to obtain cDNA. Relative gene expression was detected using the SYBR Premix Ex Tap kit (Accurate Biotechnology (Hunan) Co., Ltd., China) and normalized to the expression using 18S. The primers are listed in Supplementary Table S1.
Student’s t test was used to compare the differences between the two groups. Pearson’s correlation test was used for correlation analysis. Survival analysis was performed by the Kaplan–Meier method and compared with the log-rank test. All statistical analyses were performed using R software (vision 4.1.0), and p < 0.05 was considered statistically significant.
We identified 33 pyroptosis-related genes and performed differential gene expression analysis between LUAD and normal lung tissues. A total of 15 genes, namely, AIM2, CASP4, CASP8, GSDME, NLRP7, GPX4, CASP6, TIRAP, GSDMD, PLCG1, GSDMA, GSDMC, GSDMB, CASP3, and PJVK were highly expressed in LUAD, while the expression of IL1B, IL6, NLRP3, IL18, PYCARD, TNF, CASP1, PRKACA, NLRP1, CASP5, NOD1, ELANE, and NLRC4 was decreased (Figure 1A). Subsequently, we developed a panorama of the somatic mutations of pyroptosis-related genes. A total of 30.48% of the samples had mutations, and the three genes with the highest mutation rates were NLRP3 (11%), NLRP7 (5%), and NLRP2 (4%) (Figure 1B). Figures 1C and D show the frequency of the CNV alterations of the 33 pyroptosis-related genes and their locations on the chromosome. The frequency of copy number mutations in pyroptosis-related genes was greater than the frequency of copy number deletions. To clarify the functions of the pyroptosis-related genes, we conducted GO functional enrichment analyses. Apart from the pyroptosis pathway, pyroptosis-related genes were also enriched in defense response to bacterium, regulation of interleukin-1 or interleukin-1β production, and inflammasome complex (Figure 1E).
FIGURE 1. Landscape of expression, genetic variation, and functional enrichment of pyroptosis-related genes in LUAD. (A) Expression of pyroptosis-related genes between normal tissues and LUAD tissues (Wilcoxon test, *p < 0.05; **p < 0.01; ***p < 0.001; ns, not statistically significant). (B) Landscape of mutation profiles in LUAD patients from the TCGA cohort. (C) CNV frequency of 33 pyroptosis-related genes. (D) Location of CNV alternation of pyroptosis-related genes in the chromosome. The red dots represent more samples with increased copy number gains than samples with copy number losses, while the blue dots are the opposite. The black dot means the two are equal. (E) Enrichment analysis of GO biological process, cellular component, and molecular function. LUAD, lung adenocarcinoma; CNV, copy number variation.
We first performed correlation analysis (correlation coefficients >0.60, p < 0.001, FDR<0.05) between 14,057 lncRNAs and 33 pyroptosis-related genes in LUAD samples, and 1,070 pyroptosis-related lncRNAs were identified. Subsequently, 320 differently expressed pyroptosis-related lncRNAs were identified and exhibited in a volcano map after differential expression analysis (Figure 2A). Finally, 17 lncRNAs related to LUAD prognosis were identified after applying univariate COX regression analysis (Table 1). A heat map was plotted to show the differential expression of the 17 lncRNAs between normal and tumor tissues (Figure 2B). The forest map indicated that the hazard ratios of these 17 lncRNAs were all <1, suggesting that they were protective factors for prognosis (Figure 2C). In addition, we used Cytoscope to construct a co-expression network for the 17 pyroptosis-related lncRNAs and two corresponding genes (Figure 2D). The correlation scores between NLRC4, SCAF11, and 17 lncRNAs are shown in Figure 2E, suggesting that these lncRNAs may perform functions through NLRC4 and SCAF11. Next, we analyzed the impact of NLRC4 and SCAF11 on survival by applying the Kaplan–Meier plotter, which indicated that high expression was significantly associated with high overall survival (Supplementary Figure S1A). TIMER2.0 and GEPIA were used for differential gene expression analysis, and the results showed a significant downregulation of NLRC4 in LUAD but no change in SCAF11 (Supplementary Figure S1B–D).
FIGURE 2. Identification and characteristics of pyroptosis-related lncRNAs. (A) Volcano plot showing the differently expressed pyroptosis-related lncRNAs. (B) Heat map visualizes the differential expression of prognostic pyroptosis-related lncRNAs between normal and LUAD. (C) Forest plot showing the result of univariate Cox regression analysis for screening prognosis-related lncRNAs (p < 0.05). (D) Interaction network of the 17 prognostic prognosis-related lncRNAs–mRNAs. (E) Visualization of prognosis-related lncRNA–mRNA correlation. ***p < 0.001, LUAD, lung adenocarcinoma.
We subsequently performed multivariate COX regression analysis on the previously obtained 17 lncRNAs and three lncRNAs were identified (Figure 3A). A risk score was established based on the multivariate regression coefficients (Table 2). According to the median risk score, LUAD patients were divided into high- and low-risk groups. Most pyroptosis-related genes were significantly upregulated in the low-risk group, suggesting a more active involvement of pyroptosis (Figure 3B). By contrast, survival analysis revealed that patients in the low-risk group showed a better prognosis, indicating that pyroptosis was associated with survival advantages (Figure 3C). Principal component analysis (PCA) indicated significant distinction in transcription profiles between the two groups (Figure 3D). The risk score, survival status, and lncRNA expression are shown in Figure 3E, revealing that mortality was significantly related to risk score. Moreover, a higher risk score was significantly related to advanced stage (Figure 3F). These results indicated that a lower risk score was associated with active pyroptosis and better clinical outcome. The areas under the curves (AUCs) of the 1-year, 3-year, and 5-year ROC curve were 0.775, 0.730, and 0.705, respectively, revealing a high accuracy in the prognosis prediction of the risk model (Figure 3G). Compared with four published lncRNA signatures, the risk model we constructed had higher accuracy in predicting 1-, 3-, and 5-year survival for TCGA–LUAD patients (Figure 3H).
FIGURE 3. Construction of risk model and clinical correlation of high- and low-risk groups. (A) Three lncRNAs were identified in multivariate Cox regression analysis for model construction. (B) Differential expression of pyroptosis-related genes between high- and low-risk groups (Wilcoxon test, *p < 0.05; **p < 0.01; ***p < 0.001). (C) Kaplan–Meier curve of high- and low-risk groups. (D) PCA of high and low-risk groups. (E) Risk curve based on the risk score of each sample. Scatterplot showing the survival status of LUAD patients. Heat map showing the expression of identified lncRNAs in high- and low-risk groups. (F) Relationship between tumor stage and risk score. (G) Time-dependent ROC curves of OS at 1, 3, and 5 years. (H) Comparison of the risk model with four published lncRNA signatures. OS, overall survival; PCA, principal component analysis.
After incorporating the risk scores and clinical features, univariate and multivariate COX regression analyses were performed, and the results indicated that the risk score could serve as an independent factor affecting the survival of LUAD patients, similar to stage and age (Figures 4A,B). To better predict the 1-, 3-, and 5-year OS of LUAD patients, we constructed a nomogram by incorporating risk score, age, and stage (Figure 4C), and calibration curves were constructed to assess the accuracy of nomogram (Figure 4D).
FIGURE 4. Construction and validation of a nomogram. Univariate (A) and multivariate (B) Cox regression analyses of age, gender, stage, and risk score. (C) Nomogram for predicting the OS of LUAD patients at 1, 3, and 5 years. (D) Calibration curves of the nomogram for OS prediction at 1, 3, and 5 years. OS, overall survival; LUAD, lung adenocarcinoma.
To further understand the significance of the risk score, we conducted GSVA analysis. Several immune-related pathways and events, including interferon (IFN) gamma/alpha response, IL-6–JAK-STAT3 signaling, allograft rejection, and inflammatory response, were upregulated in the low-risk group, while in the high-risk group, metabolic and cancer-promoting pathways, such as oxidative phosphorylation, glycolysis, MYC signaling, E2F signaling, and MTORC1 signaling, were activated (Figure 5A).
FIGURE 5. Differences in landscape of the TME between high- and low-risk groups. (A) GSVA enrichment analysis of tumor hallmark pathways. (B–E) Differences in immune-related functions, TME infiltrating immune cells, other tumor-related functions, and HLA-related gene expression in the high- and low-risk groups. (F) Comparison of tumor purity, immune score, stromal score, and ESTIMATE score between high- and low-risk groups. TME, tumor microenvironment, *p < 0.05; **p < 0.01; ***p < 0.001; ns, not statistically significant.
We investigated the differences in various immune-related functions, immune-infiltrating cells, and other tumor-related functions between high- and low-risk groups using ssGSEA. As expected, the low-risk group, with a higher level of pyroptosis, was more involved in immune-related functions such as antigen-presenting cell (APC) co-stimulation/inhibition, inflammation, cytolytic activity, human leukocyte antigen (HLA) function, T cell co-stimulation/inhibition, and type I/II IFN responses, indicative of active immune functions (Figure 5B). Consistently, the higher abundance of most infiltrating immune cells existed in the low-risk group, including activated B cells, activated CD8+ T cells, dendritic cells, eosinophils, MDSCs, and macrophages (Figure 5C, Supplementary Figure S2). In addition, several tumor-related pathways and events, including immune checkpoint, angiogenesis, antigen processing machinery, and CD8+ T effector function, were also upregulated in the low-risk group. By contrast, DNA damage repair, mismatch repair, nucleotide excision repair, and DNA replication were upregulated in the high-risk group, which may play roles in genome stability and LUAD progression (Figure 5D). In addition, HLA-related genes were significantly upregulated in the low-risk group, indicative of antigen presentation (Figure 5E). Finally, tumor purity, immune score, ESTIMATE score, and stromal score were calculated using the “estimate” tool within the R package (Figure 5F). Significantly upregulated immune and stromal scores were features of the low-risk group, while the high-risk group was characterized by higher tumor purity.
Considering the significant differences in the TME landscape, we identified several ICPs and performed differential expression analysis between low- and high-risk groups. As shown in Figure 6A, all 39 selected ICPs were upregulated in the low-risk group, indicating the potential benefit of ICI therapy. Furthermore, we used IPS obtained from TIDE as a predictor of the response to anticytotoxic T lymphocyte antigen-4 (CTLA-4) and antiprogrammed cell death protein 1 (anti-PD-1) antibodies (Charoentong et al., 2017). The results showed a higher IPS level in the low-risk group, revealing a better response to combined PD1 and CTLA4 blockade therapy or PD1 monotherapy (Figures 6B,C). Considering the upregulation of immune checkpoints and infiltration of Treg cells in the low-risk group, which could suppress the effect of CD8+ T cells, we further investigated the status of T cells in the TME. Consistently, the low-risk group was characterized by higher T cell dysfunction and lower exclusion, suggesting a potential advantage to ICI therapy (Figures 6D,E). To further investigate the response to ICIs, we utilized the TIDE website to predict the “responder” and “nonresponders” of PD1 and CTLA4 blockade therapy in TCGA–LUAD patients, which were constructed by integrating TIDE score, IFNG, MSI, MDSC, CAFs, and other published modules. We found that 44% of patients in the low-risk group were identified as responders to PD1 and CTLA4 blockade therapy, while only 31% in the high-risk group were classified as responders (Figure 6F). In further analysis, only 4% of patients in the low-risk group showed no benefit from ICB compared with 13% in the high-risk group (Figure 6G). In addition, patients who were not responders or showed no benefit from PD1 and CTLA4 blockade therapy had significantly higher risk scores (Figures 6H,I).
FIGURE 6. Prediction of response to ICB therapy. (A) The expression of ICPs between high- and low-risk groups (Wilcox test, *p < 0.05; **p < 0.01; ***p < 0.001). The response to combined PD1 and CTLA4 blockade therapy (B) and PD1 monotherapy (C) between high- and low- risk groups. Differences in T cell status, including T cell dysfunction (D) and T cell exclusion (E), in high and low risk groups. (F–I) Prediction of the “responder” and “no benefit” of PD1 and CTLA4 blockade therapy in TCGA- LUAD patients from TIDE website. ICB immune checkpoint blockade, ICP immune checkpoint, PD1 programmed cell death protein 1, TCGA The Cancer Genome Atlas, LUAD lung adenocarcinoma.
In conclusion, the low-risk group could be clarified as an immune “hot” phenotype, with a high abundance of infiltrating immune cells and better efficacy for PD1 and CTLA4 blockade therapy, while the high-risk group represented the immune “cold” phenotype which was characterized by less sensitivity to ICB.
To explore whether the risk score affected immune function through NLRC4 or SCAF11, we performed a correlation analysis between NLRC4 or SCAF11 and infiltrating immune cells. The heat map showed that lncRNA AC090559.1 and its corresponding mRNA NLRC4 were closely correlated with infiltrating immune cells, suggesting that AC090559.1 may exert its regulatory function by targeting NLRC4 (Supplementary Figure S3). The scatter plots of NLRC4, SCAF11, and various infiltrating immune cells downloaded from TIMER indicated that NLRC4 was highly correlated with a variety of immune cells (Supplementary Figure S4). Finally, we predicted the sensitivity of several common chemotherapy drugs in high- and low-risk groups. The high-risk group was more sensitive to doxorubicin, sorafenib, docetaxel, and erlotinib but less sensitive to gefitinib (Supplementary Figure S5).
We detected the relative expression levels of the three lncRNAs in LUAD specimens by using qRT-PCR. The risk score was subsequently calculated, and the patients were divided into high- and low-risk groups based on the median value. The overall survival of the low-risk group was significantly better, consistent with previous results (Figure 7A). The AUC values of 3 year, 5 year and 7 year were 0.599, 0.671, and 0.704, respectively (Figure 7B). By correlation analysis of the three identified lncRNAs and immune checkpoint genes in LUAD specimens, we found a strong correlation between risk score and CTLA4, indicative of a better response to anti-CTLA4 immunotherapy (Figures 7C,D).
FIGURE 7. Validation of risk score using clinical specimens of LUAD patients. (A) Kaplan–Meier curve of high- and low-risk groups in clinical LUAD patients. (B) Time-dependent ROC curves of OS at 1, 3, and 5 years. (C) The correlation between 3 identified lncRNAs, risk score and immune checkpoints. LUAD, lung adenocarcinoma; OS, overall survival.
In recent years, the development of immunotherapy and chemotherapy has brought about a paradigm shift in the treatment of LUAD patients. However, only some patients can benefit from immunotherapy due to drug resistance (Boumahdi and de Sauvage, 2020). Furthermore, it is still difficult to identify patients who may benefit from immunotherapy. Therefore, it is important to identify novel therapeutic targets.
Pyroptosis is a type of GSDM-mediated programmed cell death that is accompanied by the release of damaged-associated molecular pattern (DAMP) and mature IL-1 proteins, which can lead to recruitment of immune cells (Rathinam and Fitzgerald, 2016; Liu et al., 2021). Pyroptosis plays distinct roles in tumor microenvironments, and thus, tumorigenesis. On the one hand, long-term chronic inflammation induced by pyroptosis can stimulate and promote tumorigenesis. On the other hand, pyroptosis can also alter the abundance of infiltrating immune cells and transform “cold” tumors into “hot” tumors, which could enhance the antitumor immune response (Du et al., 2021; Zhang et al., 2021). Moreover, pyroptosis plays important roles in activating antitumor immunity. Granzyme A, which is released by CTLs and natural killer cells, can directly cleave GSDMB to mediate tumor cell pyroptosis (Zhou et al., 2020). Meanwhile, the inflammatory response triggered by pyroptosis in a small proportion of tumor cells can trigger a strong antitumor immune response (Wang et al., 2020). Similarly, GSDME also converts apoptosis, a noninflammatory programmed cell death, into pyroptosis, and exerts antitumor functions while enhancing CTL and natural killer cell infiltration (Zhang et al., 2020). Therefore, we speculate that pyroptosis can improve the prognosis and efficacy of immunotherapy in LUAD patients. In addition, abnormally expressed lncRNAs in tumors can be detected in plasma, urine, or saliva specimens, which indicates that they have potential value as biomarkers of lncRNAs (Chandra Gupta and Nandan Tripathi, 2017). Therefore, we aimed to develop a pyroptosis-related lncRNA-based signature to accurately predict the immunotherapy efficacy and prognosis of LUAD patients.
In this study, we first extracted pyroptosis-related genes from previously published articles, and 17 prognostic-related lncRNAs were identified after correlation analysis, differential expression analysis, and univariate Cox regression analysis. After multivariate regression analysis, we developed a risk model consisting of three pyroptosis-related lncRNAs. Based on the median risk score, we divided patients into high- and low-risk groups and analyzed the differences in prognosis and immune microenvironment. ROC curves were used to verify the accuracy of the risk score. In addition, 39 LUAD patients from Shandong Provincial Hospital were used as a test cohort to verify the risk score.
The risk score served as an independent factor, which was significantly associated with stage and survival. A better prognosis was observed in the low-risk group, accompanied by highly expressed pyroptosis-related genes. Consistent with previous studies, active pyroptosis was associated with better prognosis. GSVA enrichment analysis showed that a greater inflammatory response existed in the low-risk group, which involved interferon γ/α and IL6/JAK/STAT3 pathways, allograft rejection, complement, and inflammatory response. By contrast, the high-risk group was more related to MYC, E2F, MTORC1, and glycolytic pathways, which are essential for tumorigenesis. Similarly, the abundance of infiltrating immune cells was generally higher in the low-risk group. Considering the results of ssGSEA (Figure 5C) and correlation analysis (Supplementary Figure S3), we noticed that the significantly enriched infiltrating immune cells were mainly associated with inflammation and antigen presentation (eosinophils, mast cells, monocytes, macrophages, immature dendritic cells, and activated dendritic cells) and immunomodulatory events (regulatory T cell and various T helper cells) in the low-risk group. As shown in Figure 5E, HLA function was significantly activated in the low-risk group, further confirming antigen presentation.
Interestingly, activated CD8+ T cells highly infiltrated the low-risk group, accompanied by the high abundance of immunosuppressive cells such as Treg and MDSCs (Figure 5C). Considering simultaneous T cell co-stimulation and co-inhibition, we examined whether T cells could exercise their conventional function of antitumor immunity in the low-risk group (Figure 5B). As such, we analyzed the dysfunction and exclusion of T cells, which revealed significant T cell dysfunction in the low-risk group (Figures 6D,E). A previous study has reported that a broad spectrum of dysfunctional states can exist in intratumoral T cells, which essentially blocks the durable clinical benefits of patients (Thommen and Schumacher, 2018). The upregulation of ICPs mediated by PD-1 was the main manifestation of T cell dysfunction, accompanied by a variety of other inhibitory factors such as Treg cell infiltration, cytokine production, and metabolic stress (Speiser et al., 2016; Zarour, 2016; Gajewski et al., 2013). According to previous reports on the simultaneous blockade of PD-1, other inhibitory receptors such as CTLA-4, Tim-3, Lag-3, and TIGIT have been shown to reactivate dysfunctional T cells and provide benefit from ICBs, which was also verified in our results (Figures 6F–I).
Therefore, the low-risk group was considered an immune “hot” phenotype, indicative of a beneficial response to immunotherapy. Alternatively, the high-risk group was identified as an immune “cold” phenotype, whose efficacy of immunotherapy was poor.
In our study, the constructed risk score consisted of only three pyroptosis-related lncRNAs, namely, AC090559.1, AC034102.8, and AC026355.2, which have been rarely mentioned in previous studies. Nevertheless, AC090559.1 was also identified as an independent risk factor related to autophagy and ferroptosis, suggesting that it may be a key regulator in programmed cell death (Guo et al., 2021; Wu et al., 2021). We also found that AC090559.1 may have a potential regulatory relationship with NLRC4. NLRC4 is the core component of NLRC4 inflammasomes, which was composed of a trigger (e.g., cytosolic flagellin), sensor (NAIP), nucleator (NLRC4), adapter (ASC), and effector (CASP1) (Duncan and Canna, 2018). The activation of NLRC4 inflammasomes can activate caspase-1, which cleaves pro-IL-1β and pro-IL-18 and simultaneously cleaves and activates gasdermin-D, thereby activating pyroptosis (Kay et al., 2020). Similar to pyroptosis, the effects of NLRC4 depend on the type and genomic background of the tumor. Continuously aberrant activation of chronic inflammation mediated by NLRC4 can promote the malignant progression of tumor cells. A previous study revealed that obesity-associated NLRC4 inflammasomes mediated IL-1β release, which promotes the growth of breast cancer by triggering VEGF production and angiogenesis (Kolb et al., 2016). Similarly, in nonalcoholic fatty liver, IL-1 signaling promoted metastasis (Ohashi et al., 2019). Consistent with our research, NLRC4 could also suppress tumor development while inducing antitumor immunity. In another study, Flagrp170, an artificially designed immunomodulator, showed protective antitumor immunity in an NLRC4-dependent manner (Yu X. et al., 2021). Sutterwala et al. demonstrated that NLRC4 enhanced inflammation in tumor-associated macrophages (TAMs) in a noninflammasome-dependent manner and the antimelanoma effects of IFN-γ produced by CD4 + and CD8 + T cells (Janowski et al., 2016). Moreover, NLRC4/NAIP5 also participated in the antigen recognition of flagellin-expressing tumor cells to facilitate antigen presentation to T cells, thereby activating CD4+ and CD8+ T cells and exerting antitumor effects (Garaude et al., 2012). However, studies on NLRC4 in lung cancer are still limited.
We found that NLRC4 was highly associated with immune cells such as activated/immature/plasmacytoid dendritic cells, γ delta T cells, MDSCs, macrophages, natural killer cells, regulatory T cells, T follicular helper cells, and type 1 T helper cells. Interestingly, AC090559.1 showed a similar effect compared with NLRC4 in the aforementioned immune cells (Supplementary Figure S3), suggesting that AC090559.1 may affect the extent of pyroptosis and the abundance of immune cells through NLRC4. Here, we performed correlation analysis of the three identified lncRNAs and several immune checkpoints and observed that these three lncRNAs were highly correlated with CTLA4 expression, suggesting that the risk model may reveal more significant effects on CTLA4 blockade; regardless of this, there were some limitations. Presently, there are few studies supporting the mechanisms of action of NLRC4 and AC090559.1, and thus, further studies are needed to clarify these mechanisms.
In conclusion, we constructed a prognostic model based on pyroptosis-related lncRNAs. In addition to prognosis prediction, this model was significantly associated with pyroptosis extent and immune phenotype. We believe that these lncRNAs may serve as new targets for inducing pyroptosis, and stimulating pyroptosis-mediated antitumor immunity may provide new insights for the treatment of LUAD patients.
The original contributions presented in the study are included in the article/Supplementary Material; further inquiries can be directed to the corresponding author.
Written informed consent was obtained from the individuals for the publication of any potentially identifiable images or data included in this article. Our research obtained approval from the Biomedical Research Ethic Committee of Shandong Provincial Hospital (SWYX:NO. 2021-433).
JD contributed to design and supervised the study and correspondence. Co-authors JL and QL collected and analyzed the data and completed the manuscript. HS, YL, YW, and GW helped search for references and offered guidelines of statistical methods. All authors have read and approved the final version to be published.
This study is supported by the Natural Science Foundation of Shandong Province (grant number ZR2019PH002) and Clinical Medicine Science and Technology Innovation Plan of Jinan (grant number 202019058).
The authors declare that the research was conducted in the absence of any commercial or financial relationships that could be construed as a potential conflict of interest.
All claims expressed in this article are solely those of the authors and do not necessarily represent those of their affiliated organizations, or those of the publisher, the editors, and the reviewers. Any product that may be evaluated in this article, or claim that may be made by its manufacturer, is not guaranteed or endorsed by the publisher.
We express our sincere thanks to the LUAD patients from Shandong Provincial Hospital, TCGA–LUAD for providing data. We thank openbiox community and HiPlot team (https://hiplot.com.cn) for providing technical assistance and valuable tools for data analysis and visualization.
The Supplementary Material for this article can be found online at: https://www.frontiersin.org/articles/10.3389/fgene.2022.838624/full#supplementary-material.
Supplementary Figure S1 | Expression and prognosis analysis of NLRC4 and SCAF11/SIP1. (A) KM curves of NLRC4 and SCAF11/SIP1 in LUAD patients obtained from the KMPlot. Differential expression of NLRC4 and SCAF11 in GEPIA websites (B) and TIMER2.0 (C). NLRC4 nod-like receptor family CARD domain containing 4, SCAF11/SIP1 SR-related CTD associated factor 11, LUAD lung adenocarcinoma.
Supplementary Figure S2 | Differential abundance of infiltrating immune cells between high- and low-risk groups in LUAD. TME: tumor microenvironment, LUAD: lung adenocarcinoma.
Supplementary Figure S3 | Correlation analysis between risk score, interacted mRNAs, identified lncRNAs, and TME infiltrating immune cells. TME: tumor microenvironment.
Supplementary Figure S4 | Correlation between NLRC4 or SCAF11 and TME immune cell infiltration in LUAD from TIMER website. NLRC4: nod-like receptor family CARD domain containing 4, SCAF11: SR-related CTD associated factor 11, TME: tumor microenvironment, LUAD lung adenocarcinoma.
Supplementary Figure S5 | Prediction of drug sensitivity.
Aran, D., Hu, Z., and Butte, A. J. (2017). xCell: Digitally Portraying the Tissue Cellular Heterogeneity Landscape. Genome Biol. 18 (1), 220. doi:10.1186/s13059-017-1349-1
Becht, E., Giraldo, N. A., Lacroix, L., Buttard, B., Elarouci, N., Petitprez, F., et al. (2016). Estimating the Population Abundance of Tissue-Infiltrating Immune and Stromal Cell Populations Using Gene Expression. Genome Biol. 17 (1), 218. doi:10.1186/s13059-016-1070-5
Bhan, A., Soleimani, M., and Mandal, S. S. (2017). Long Noncoding RNA and Cancer: A New Paradigm. Cancer Res. 77 (15), 3965–3981. doi:10.1158/0008-5472.can-16-2634
Boumahdi, S., and de Sauvage, F. J. (2020). The Great Escape: Tumour Cell Plasticity in Resistance to Targeted Therapy. Nat. Rev. Drug Discov. 19 (1), 39–56. doi:10.1038/s41573-019-0044-1
Chakraborty, H., and Hossain, A. (2018). R Package to Estimate Intracluster Correlation Coefficient with Confidence Interval for Binary Data. Comput. Methods Programs Biomed. 155, 85–92. doi:10.1016/j.cmpb.2017.10.023
Chandra Gupta, S., and Nandan Tripathi, Y. (2017). Potential of Long Non-Coding RNAs in Cancer Patients: From Biomarkers to Therapeutic Targets. Int. J. Cancer 140 (9), 1955–1967. doi:10.1002/ijc.30546
Charoentong, P., Finotello, F., Angelova, M., Mayer, C., Efremova, M., Rieder, D., et al. (2017). Pan-Cancer Immunogenomic Analyses Reveal Genotype-Immunophenotype Relationships and Predictors of Response to Checkpoint Blockade. Cell Rep. 18 (1), 248–262. doi:10.1016/j.celrep.2016.12.019
Chen, B., Khodadoust, M. S., Liu, C. L., Newman, A. M., and Alizadeh, A. A. (2018). Profiling Tumor Infiltrating Immune Cells with CIBERSORT. Methods Mol. Biol. 1711, 243–259. doi:10.1007/978-1-4939-7493-1_12
Chen, Y., Zitello, E., Guo, R., and Deng, Y. (2021). The Function of LncRNAs and Their Role in the Prediction, Diagnosis, and Prognosis of Lung Cancer. Clin. Transl. Med. 11 (4), e367. doi:10.1002/ctm2.367
Deets, K. A., and Vance, R. E. (2021). Inflammasomes and Adaptive Immune Responses. Nat. Immunol. 22 (4), 412–422. doi:10.1038/s41590-021-00869-6
Denisenko, T. V., Budkevich, I. N., and Zhivotovsky, B. (2018). Cell Death-Based Treatment of Lung Adenocarcinoma. Cell Death Dis. 9 (2), 117. doi:10.1038/s41419-017-0063-y
Du, T., Gao, J., Li, P., Wang, Y., Qi, Q., Liu, X., et al. (2021). Pyroptosis, Metabolism, and Tumor Immune Microenvironment. Clin. Transl. Med. 11 (8), e492. doi:10.1002/ctm2.492
Duncan, J. A., and Canna, S. W. (2018). The NLRC4 Inflammasome. Immunol. Rev. 281 (1), 115–123. doi:10.1111/imr.12607
Evavold, C. L., Hafner-Bratkovič, I., Devant, P., D’Andrea, J. M., Ngwa, E. M., Boršić, E., et al. (2021). Control of Gasdermin D Oligomerization and Pyroptosis by the Ragulator-Rag-mTORC1 Pathway. Cell 184 (17), 4495–4511. doi:10.1016/j.cell.2021.06.028
Finotello, F., Mayer, C., Plattner, C., Laschober, G., Rieder, D., Hackl, H., et al. (2019). Molecular and Pharmacological Modulators of the Tumor Immune Contexture Revealed by Deconvolution of RNA-Seq Data. Genome Med. 11 (1), 34. doi:10.1186/s13073-019-0638-6
Fu, J., Li, K., Zhang, W., Wan, C., Zhang, J., Jiang, P., et al. (2020). Large-Scale Public Data Reuse to Model Immunotherapy Response and Resistance. Genome Med. 12 (1), 21. doi:10.1186/s13073-020-0721-z
Garaude, J., Kent, A., van Rooijen, N., and Blander, J. M. (2012). Simultaneous Targeting of Toll- and Nod-Like Receptors Induces Effective Tumor-Specific Immune Responses. Sci. Transl. Med. 4 (120), 120ra16. doi:10.1126/scitranslmed.3002868
Guo, Y., Qu, Z., Li, D., Bai, F., Xing, J., Ding, Q., et al. (2021). Identification of a Prognostic Ferroptosis-Related lncRNA Signature in the Tumor Microenvironment of Lung Adenocarcinoma. Cell Death Discov. 7 (1), 190. doi:10.1038/s41420-021-00576-z
Hänzelmann, S., Castelo, R., and Guinney, J. (2013). GSVA: Gene Set Variation Analysis for Microarray and RNA-Seq Data. BMC Bioinforma. 14, 7. doi:10.1186/1471-2105-14-7
Hirsch, F. R., Scagliotti, G. V., Mulshine, J. L., Kwon, R., Curran, W. J., Wu, Y.-L., et al. (2017). Lung Cancer: Current Therapies and New Targeted Treatments. Lancet 389 (10066), 299–311. doi:10.1016/s0140-6736(16)30958-8
Janowski, A. M., Colegio, O. R., Hornick, E. E., McNiff, J. M., Martin, M. D., Badovinac, V. P., et al. (2016). NLRC4 Suppresses Melanoma Tumor Progression Independently of Inflammasome Activation. J. Clin. Invest. 126 (10), 3917–3928. doi:10.1172/jci86953
Jiang, P., Gu, S., Pan, D., Fu, J., Sahu, A., Hu, X., et al. (2018). Signatures of T Cell Dysfunction and Exclusion Predict Cancer Immunotherapy Response. Nat. Med. 24 (10), 1550–1558. doi:10.1038/s41591-018-0136-1
Kay, C., Wang, R., Kirkby, M., and Man, S. M. (2020). Molecular Mechanisms Activating the NAIP‐NLRC4 Inflammasome: Implications in Infectious Disease, Autoinflammation, and Cancer. Immunol. Rev. 297 (1), 67–82. doi:10.1111/imr.12906
Kolb, R., Phan, L., Borcherding, N., Liu, Y., Yuan, F., Janowski, A. M., et al. (2016). Obesity-Associated NLRC4 Inflammasome Activation Drives Breast Cancer Progression. Nat. Commun. 7, 13007. doi:10.1038/ncomms13007
Lazzari, C., Bulotta, A., Cangi, M. G., Bucci, G., Pecciarini, L., Bonfiglio, S., et al. (2020). Next Generation Sequencing in Non-Small Cell Lung Cancer: Pitfalls and Opportunities. Diagn. (Basel) 10 (12), 1092. doi:10.3390/diagnostics10121092
Li, T., Fu, J., Zeng, Z., Cohen, D., Li, J., Chen, Q., et al. (2020). TIMER2.0 for Analysis of Tumor-Infiltrating Immune Cells. Nucleic Acids Res. 48 (W1), W509–W514. doi:10.1093/nar/gkaa407
Liu, B., and Yang, S. (2021). A Five Autophagy-Related Long Non-Coding RNA Prognostic Model for Patients with Lung Adenocarcinoma. Int. J. General Med. 14, 7145–7158. doi:10.2147/ijgm.s334601
Liu, X., Xia, S., Zhang, Z., Wu, H., and Lieberman, J. (2021). Channelling Inflammation: Gasdermins in Physiology and Disease. Nat. Rev. Drug Discov. 20 (5), 384–405. doi:10.1038/s41573-021-00154-z
Lu, L., Liu, L.-P., Zhao, Q.-Q., Gui, R., and Zhao, Q.-Y. (2021). Identification of a Ferroptosis-Related LncRNA Signature as a Novel Prognosis Model for Lung Adenocarcinoma. Front. Oncol. 11, 675545. doi:10.3389/fonc.2021.675545
Mariathasan, S., Turley, S. J., Nickles, D., Castiglioni, A., Yuen, K., Wang, Y., et al. (2018). TGFβ Attenuates Tumour Response to PD-L1 Blockade by Contributing to Exclusion of T Cells. Nature 554 (7693), 544–548. doi:10.1038/nature25501
Mayakonda, A., Lin, D.-C., Assenov, Y., Plass, C., and Koeffler, H. P. (2018). Maftools: Efficient and Comprehensive Analysis of Somatic Variants in Cancer. Genome Res. 28 (11), 1747–1756. doi:10.1101/gr.239244.118
Ohashi, K., Wang, Z., Yang, Y. M., Billet, S., Tu, W., Pimienta, M., et al. (2019). NOD‐Like Receptor C4 Inflammasome Regulates the Growth of Colon Cancer Liver Metastasis in NAFLD. Hepatology 70 (5), 1582–1599. doi:10.1002/hep.30693
Racle, J., de Jonge, K., Baumgaertner, P., Speiser, D. E., and Gfeller, D. (2017). Simultaneous Enumeration of Cancer and Immune Cell Types from Bulk Tumor Gene Expression Data. Elife 6, e26476. doi:10.7554/eLife.26476
Rathinam, V. A. K., and Fitzgerald, K. A. (2016). Inflammasome Complexes: Emerging Mechanisms and Effector Functions. Cell 165 (4), 792–800. doi:10.1016/j.cell.2016.03.046
Ritchie, M. E., Phipson, B., Wu, D., Hu, Y., Law, C. W., Shi, W., et al. (2015). Limma Powers Differential Expression Analyses for RNA-Sequencing and Microarray Studies. Nucleic Acids Res. 43 (7), e47. doi:10.1093/nar/gkv007
Seijo, L. M., Peled, N., Ajona, D., Boeri, M., Field, J. K., Sozzi, G., et al. (2019). Biomarkers in Lung Cancer Screening: Achievements, Promises, and Challenges. J. Thorac. Oncol. 14 (3), 343–357. doi:10.1016/j.jtho.2018.11.023
Şenbabaoğlu, Y., Gejman, R. S., Winer, A. G., Liu, M., Van Allen, E. M., de Velasco, G., et al. (2016). Tumor Immune Microenvironment Characterization in Clear Cell Renal Cell Carcinoma Identifies Prognostic and Immunotherapeutically Relevant Messenger RNA Signatures. Genome Biol. 17 (1), 231. doi:10.1186/s13059-016-1092-z
Shao, J., Zhang, B., Kuai, L., and Li, Q. (2021). Integrated Analysis of Hypoxia-Associated lncRNA Signature to Predict Prognosis and Immune Microenvironment of Lung Adenocarcinoma Patients. Bioengineered 12 (1), 6186–6200. doi:10.1080/21655979.2021.1973874
Gajewski, T. F., Schreiber, H., and Fu, Y. X. (2013). Innate and Adaptive Immune Cells in Tumor Microenvironment. Nature immunology 14 (10), 1014–1022. doi:10.1038/ni.2703
Siegel, R. L., Miller, K. D., Fuchs, H. E., and Jemal, A. (2021). Cancer Statistics, 2021. CA A Cancer J. Clin. 71 (1), 7–33. doi:10.3322/caac.21654
Speiser, D. E., Ho, P.-C., and Verdeil, G. (2016). Regulatory Circuits of T Cell Function in Cancer. Nat. Rev. Immunol. 16 (10), 599–611. doi:10.1038/nri.2016.80
Tang, Z., Li, C., Kang, B., Gao, G., Li, C., and Zhang, Z. (2017). GEPIA: A Web Server for Cancer and Normal Gene Expression Profiling and Interactive Analyses. Nucleic Acids Res. 45, W98–W102. doi:10.1093/nar/gkx247
Thommen, D. S., and Schumacher, T. N. (2018). T Cell Dysfunction in Cancer. Cancer Cell 33 (4), 547–562. doi:10.1016/j.ccell.2018.03.012
Wang, Q., Wang, Y., Ding, J., Wang, C., Zhou, X., Gao, W., et al. (2020). A Bioorthogonal System Reveals Antitumour Immune Function of Pyroptosis. Nature 579 (7799), 421–426. doi:10.1038/s41586-020-2079-1
Wu, L., Wen, Z., Song, Y., and Wang, L. (2021). A Novel Autophagy‐Related lncRNA Survival Model for Lung Adenocarcinoma. J. Cell Mol. Med. 25 (12), 5681–5690. doi:10.1111/jcmm.16582
Yang, L., Wu, Y., Xu, H., Zhang, J., Zheng, X., Zhang, L., et al. (2021). Identification and Validation of a Novel Six-lncRNA-Based Prognostic Model for Lung Adenocarcinoma. Front. Oncol. 11, 775583. doi:10.3389/fonc.2021.775583
Ye, Y., Dai, Q., and Qi, H. (2021). A Novel Defined Pyroptosis-Related Gene Signature for Predicting the Prognosis of Ovarian Cancer. Cell Death Discov. 7 (1), 71. doi:10.1038/s41420-021-00451-x
Yu, P., Zhang, X., Liu, N., Tang, L., Peng, C., and Chen, X. (2021a). Pyroptosis: Mechanisms and Diseases. Sig Transduct. Target Ther. 6 (1), 128. doi:10.1038/s41392-021-00507-5
Yu, X., Liu, W., Chen, S., Cheng, X., Paez, P. A., Sun, T., et al. (2021b). Immunologically Programming the Tumor Microenvironment Induces the Pattern Recognition Receptor NLRC4-Dependent Antitumor Immunity. J. Immunother. Cancer 9 (1), e001595. doi:10.1136/jitc-2020-001595
Zarour, H. M. (2016). Reversing T-Cell Dysfunction and Exhaustion in Cancer. Clin. Cancer Res. 22 (8), 1856–1864. doi:10.1158/1078-0432.ccr-15-1849
Zhang, H., Meltzer, P., and Davis, S. (2013). RCircos: An R Package for Circos 2D Track Plots. BMC Bioinforma. 14, 244. doi:10.1186/1471-2105-14-244
Zhang, Z., Zhang, Y., and Lieberman, J. (2021). Lighting a Fire: Can We Harness Pyroptosis to Ignite Antitumor Immunity? Cancer Immunol. Res. 9 (1), 2–7. doi:10.1158/2326-6066.cir-20-0525
Zhang, Z., Zhang, Y., Xia, S., Kong, Q., Li, S., Liu, X., et al. (2020). Gasdermin E Suppresses Tumour Growth by Activating Anti-Tumour Immunity. Nature 579 (7799), 415–420. doi:10.1038/s41586-020-2071-9
Zhou, Z., He, H., Wang, K., Shi, X., Wang, Y., Su, Y., et al. (2020). Granzyme A from Cytotoxic Lymphocytes Cleaves GSDMB to Trigger Pyroptosis in Target Cells. Science 368 (6494), eaaz7548. doi:10.1126/science.aaz7548
AUC Area under the curve
APC Antigen-presenting cell
CCR C-C chemokine receptor
ceRNA Competing endogenous RNA
CNV Copy number variations
CTL Cytotoxic lymphocyte
CTLA-4 Cytotoxic T lymphocyte antigen-4
DAMP Damaged-associated molecular pattern
FDR False-discovery rate
FPKM Fragments per kilobase of exon model per million reads mapped
GEPIA Gene Expression Profiling Integrative Analysis
GSDMs Gasdermins
HLA Human leukocyte antigen
HR Hazard ratio
ICB Immune checkpoint blockade
ICP Immune checkpoint
IFN Interferon
IL Interleukin
IPS Immunophenoscore
KM Kaplan–Meier
lncRNA long noncoding RNA
LUAD Lung adenocarcinoma
miRNA MicroRNA
NK cell Natural killer cell
OS Overall survival
PCA Principal component analysis
PCD Programmed cell death
PD-1 Programmed cell death 1
ROC Receiver operating characteristic
ssGSEA Single-sample gene set enrichment analysis
TAM Tumor-associated macrophage
TCGA The Cancer Genome Atlas
TIDE Tumor Immune Dysfunction and Exclusion
TME Tumor microenvironment
Keywords: lung adenocarcinoma, pyroptosis, prognosis, long noncoding RNA, immunotherapy
Citation: Liu J, Liu Q, Shen H, Liu Y, Wang Y, Wang G and Du J (2022) Identification and Validation of a Three Pyroptosis-Related lncRNA Signature for Prognosis Prediction in Lung Adenocarcinoma. Front. Genet. 13:838624. doi: 10.3389/fgene.2022.838624
Received: 18 December 2021; Accepted: 15 June 2022;
Published: 19 July 2022.
Edited by:
Anton A. Buzdin, European Organisation for Research and Treatment of Cancer, BelgiumReviewed by:
Leslie Chavez-Galan, Instituto Nacional de Enfermedades Respiratorias-México (INER), MexicoCopyright © 2022 Liu, Liu, Shen, Liu, Wang, Wang and Du. This is an open-access article distributed under the terms of the Creative Commons Attribution License (CC BY). The use, distribution or reproduction in other forums is permitted, provided the original author(s) and the copyright owner(s) are credited and that the original publication in this journal is cited, in accordance with accepted academic practice. No use, distribution or reproduction is permitted which does not comply with these terms.
*Correspondence: Jiajun Du, ZHVqaWFqdW5Ac2R1LmVkdS5jbg==
†These authors contributed equally to this work and share first authorship
Disclaimer: All claims expressed in this article are solely those of the authors and do not necessarily represent those of their affiliated organizations, or those of the publisher, the editors and the reviewers. Any product that may be evaluated in this article or claim that may be made by its manufacturer is not guaranteed or endorsed by the publisher.
Research integrity at Frontiers
Learn more about the work of our research integrity team to safeguard the quality of each article we publish.