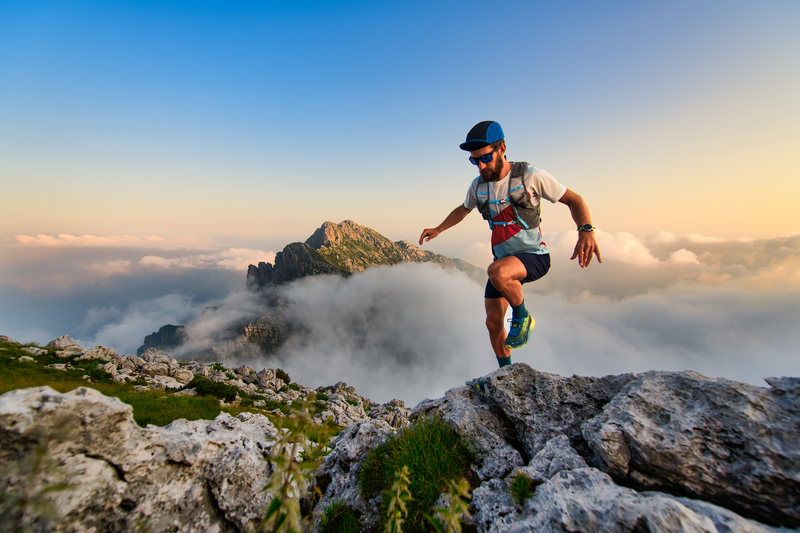
94% of researchers rate our articles as excellent or good
Learn more about the work of our research integrity team to safeguard the quality of each article we publish.
Find out more
ORIGINAL RESEARCH article
Front. Genet. , 27 May 2022
Sec. Computational Genomics
Volume 13 - 2022 | https://doi.org/10.3389/fgene.2022.838220
This article is part of the Research Topic Identifying Genetics-Based Mechanisms and Treatments for Neurodevelopmental and Psychiatric Disorders Through Data Integration View all 6 articles
Glaucoma is the leading cause of irreversible blindness globally; hence, relevant clinical biomarkers are necessary to enable diagnosis, early detection, and development of novel therapies. The differentially expressed genes were annotated and visualized using Gene Ontology and Kyoto Encyclopedia. In addition, a competitive endogenous ribonucleic acids network was constructed using Cytoscape, which explained the regulation of gene expression in glaucoma. The CIBERSORT algorithm was employed to analyze the immune microenvironment. We validated that the core genes could predict glaucoma occurrence and development and identified potential molecular mechanism pathways, which were associated with immune infiltration and participated in endogenous regulation networks. Our data may partially explain the pathogenesis of glaucoma and they provide potential theoretical support for targeted therapy.
Glaucoma, the leading cause of irreversible blindness globally, is commonly regarded as a neurodegenerative disease with a multifactorial origin, resulting in progressive visual field loss and blindness (Beykin et al., 2021). It is estimated that by 2040, the number of patients with glaucoma will have increased from the prevailing 60 million to 111.8 million (Tham et al., 2014). Primary open-angle glaucoma (POAG) is the most prevalent glaucoma subtype. POAG combined with irreversible optic neuropathy keep increasing the global burden of glaucoma because of unsatisfactory clinical diagnosis and treatment thus far (Zimprich et al., 2020). Currently, the accurate diagnosis and detection of POAG at an early stage is difficult. Without efficient instruments to help estimate the screening and diagnosis, progression monitoring, and response to treatment, all diagnoses and detections are based on the clinician’s experience. Therefore, glaucoma-related biomarkers must be identified for clinical testing to enable early diagnosis and detection of disease progression. This will assist in clinical examinations, enhance early diagnosis, and improve early phase clinical trial design, allowing for timely intervention. Therefore, the emergence of gene therapy studies, regulation of gene expression, and gene networks aiming at different pathways involved in glaucoma pathogenesis are high-priority landmarks for ophthalmic research. Recent studies have demonstrated that long non-coding ribonucleic acids (lncRNAs), which do not encode proteins and are >200-nt-long RNAs, can mediate the pathogenesis of glaucoma (Yao et al., 2020) (Kong et al., 2021). According to existing reports, lncRNAs regulate gene expression, protein modification, cell differentiation, immune response, and other critical pathological processes (Jiang et al., 2019). Furthermore, some of these functions have been identified in neurodegenerative diseases such as Alzheimer’s disease (Zhang and Wang, 2021). The sponge hypothesis was proposed to suggest that lncRNAs suppressed miRNA interactions with mRNAs, thereby affecting the translation of protein-coding genes (Dhamija and Menon, 2018). Therefore, lncRNAs are called competing endogenous RNAs (ceRNAs), and the lncRNA–microRNA (miRNA)–messenger RNA (mRNA) regulation network is called the ceRNA network. In this study, we established the ceRNA network of core genes in glaucoma to describe the interactions of these RNAs.
The microenvironment of glaucoma comprises immune cells, extracellular matrix, various growth factors, and inflammatory factors and possesses special physical and chemical characteristics, which significantly affect the diagnosis and clinical treatment sensitivity (Gajewski et al., 2013). The resident immune cells in the retina are implicated in the pathogenesis of various neurodegenerative diseases, including multiple sclerosis and glaucoma, which affects the optical system (Namekata et al., 2019). A study showed that neurodegeneration in glaucoma was associated with immune cell infiltration in the optic nerve head region (Maddineni et al., 2020). Another study primarily tested the function of a complex immune infiltration called complement-mediated inflammation in glaucoma (Harder et al., 2020). The ocular hypertension model showed infiltrating monocytes in pathological glaucoma (Tribble et al., 2020). However, the exact role of immune infiltration in glaucoma is yet to be discovered.
This study used next-generation sequencing data to analyze individual trabecular meshwork (TM) samples from POAG patients. We matched controls to identify RNA expression characteristics and evaluated the potential of these RNAs to serve as biomarkers for POAG diagnosis and prognosis to explore further the underlying molecular mechanisms of core genes affecting glaucoma progression.
The Series Matrix File data files of lncRNA were downloaded from the National Center for Biotechnology Information Gene Expression Omnibus (GEO); GSE138125 comprises the data of four normal samples (n = 4) and four POAG samples (n = 4). GSE27276 comprises the data of nineeen normal samples (n = 19) and seventeen POAG samples (n = 17). The POAG biomarkers identification process is shown in Figure 1. The limma package in R (Version 1.3.959) was applied to analyze the difference between the two datasets using the standard log2FC > 1 with adj.P.Val <0.05. In addition, we reserved differently expressed genes to explore the molecular mechanisms of POAG occurrence and development.
We used GO and KEGG to annotate specific genes in order to identify the biological function and signaling pathways related to the occurrence and development of glaucoma. We defined significant results as Min overlap ≥3 and p ≤ 0.01.
The miRcode database was used to predict the interaction groups of lncRNA–miRNA. miRDB, mirtarbase, and TargetScan were combined to predict the interaction between miRNA and mRNA, and the co-identified targeted mRNAs were selected for further analysis. In addition, by evaluating the interactions between lncRNA–miRNA and mRNA–miRNA, Protein–protein interaction (PPI) network of RNAs was constructed and visualized using Cytoscape.
The RNA-seq data of different POAG patients’ subtypes were processed using the CIBERSORT algorithm (Chen et al., 2018) to estimate the relative infiltration proportions of 22 immune cells. The corrplot R package was used to analyze the relationship of immune cells and assess their interaction’s influence. The vioplot R package was applied to plot the relative number of immune cells and evaluate the influence of differently expressed genes on immune infiltration. Spearman correlation analysis was performed on gene expression level and immune cell numbers, with p < 0.05 considered significant.
Predefined gene sets were assessed using GSEA. Genes were ranked according to the degree of differential expression in lncRNA and mRNA samples, and the predefined gene sets were tested whether they were enriched at the top or bottom of the ranked list. In this study, we used GSEA to compare the difference in signaling pathways between the high- and low-risk groups, exploring a possible molecular mechanism of the difference in the prognosis of patients in the two groups. The substitution frequency was set as 1,000, and the type was set as phenotype. As ssGSEA classifying gene sets with common biological functions, chromosomal localization, and physiological regulation, we obtained immune cells and conducted Spearman correlation analysis with hub genes.
All statistical analyses were conducted in R language (version 3.6). All statistical tests were bilateral, and p < 0.05 indicated a significant difference.
We downloaded eight groups of lncRNA transcriptome data of GSE138125 from GEO, comprising normal (n = 4) and POAG (n = 4) groups. We acquired 36 groups of RNA transcriptome data from GSE27276, comprising normal (n = 19) and POAG (n = 17) groups. First, the limma package was applied to screen the differentially expressed genes between normal and POAG groups with the following conditions: adj.P.Val <0.05 and | Log2FC | > 1. A total of 411 gene expression variations was identified from GSE138125, comprising 143 upregulated genes and 268 downregulated genes (Figures 2A,B). In addition, there were 59 upregulated genes and 108 downregulated genes in the 167 differentially expressed genes of GSE27276 (Figures 2C,D). Subsequently, pathway analysis was performed on these 167 differentially expressed genes, with the results showing that they were mainly enriched in 20 pathways, especially the haptoglobin–hemoglobin complex and epidermis development pathway (Figure 3).
FIGURE 2. Heatmaps and Volcano Plots of GSE138125 and GSE27276. GSE138125 was a lncRNA transcriptome dataset. We screened a total of 411 differential genes, including 143 up-regulated genes and 268 down-regulated genes (A,B). We also downloaded mRNA transcriptome data from GSE27276, there was 59 up-regulated genes and 108 down-regulated genes in all the 167 differential genes (C,D).
FIGURE 3. The enrichment of total 167 differential genes from GSE27276. The results of pathway analysis showed that differential genes were mainly enriched in 20 pathways, especially in haptoglobin hemoglobin complex and epidermis development (A,B).
We used miRcode database (http://www.mircode.org/) to predict the miRNA targets of 411 different lncRNAs, and the results showed that there were 1,317 lncRNA-related targeted miRNAs, providing 1,317 pairs of lncRNA–miRNA. We used TargetScan, miRDB, and mirtarbase databases to predict miRNA-related target mRNAs, and 1,935 pairs of miRNA–mRNA were obtained. Subsequently, all the mRNAs from 1,935 miRNA–mRNA relationship pairs were intersected with 167 differentially expressed mRNAs, reserving eight pairs of miRNA–mRNA. Then, the eight pairs of miRNA–mRNA and 1,317 pairs of lncRNA–miRNA were intersected, and finally, 64 pairs of lncRNA–miRNA–mRNA were obtained.
PPI network of 64 pairs of lncRNA–miRNA–mRNA was generated and visualized using Cytoscape to analyze the competitive endogenous network of key genes in lncRNA and miRNA. Figure 4A shows the competitive endogenous network diagram of ceRNA. We calculated the connectivity degree of each gene with hub genes, defined as mRNAs with top five grades, including FLOT2, ANKH, DLEU1, DDIT4, and CSRP2.
FIGURE 4. ceRNA network of differential genes and the relationship between core genes and immune infiltration. PPI network of 64 pairs of lncRNA-miRNA-mRNA relationship was generated, and then visualized by Cytoscape (A) and mRNAs with top five degree were defined as core genes, including FLOT2, ANKH, DLEU1, DDIT4 and CSRP2. Violin plot demonstrated that Plasma cells and Dendritic cells activated were significantly reduced in patients comparing with control group, while NK cells activated and Monocytes were increased remarkably (B). (C) showed the relative percentage of 22 type of immune cells in each sample. We found that core genes were all closed related to the content of immune cells as we prospected (D). In brief, ANKH was positively correlated with T cells CD4 memory resting and was negatively correlated with Dendritic cells activated and Plasma cells. CSRP2 was positively associated with Dendritic cells activated, NK cells resting and Plasma cells. DDIT was positively associated with NK cells resting and Plasma cells. There was a negative correlation between DLEU1 and Macrophages M1. FLOT2 had a positive relation with B cells naïve, Dendritic cells activated and T cells follicular helper, while having a negative relation with Macrophages M2.
By analyzing the relationship between hub genes and immune infiltration in POAG, the potential molecular mechanism of hub genes influencing glaucoma progression was further explored. The violin plot demonstrated that plasma cell and activated dendritic cell (DC) numbers were significantly reduced in patients compared to those in the control group. By contrast, natural killer cells (NK) were activated, and monocyte counts were increased remarkably (Figure 4B). Figure 4C shows the relative percentage of 22 types of immune cells in each sample. We observed that core genes were closely related to the immune cell counts as expected (Figure 4D). In brief, ANKH expression was positively correlated with memory resting CD4 T cell count and was negatively correlated with activated DC and plasma cell counts. CSRP2 expression was positively associated with activated DC, resting NK cell, and plasma cell counts. DDIT expression was positively associated with resting NK cell and plasma cell counts. There was a negative correlation between DLEU1 expression and M1 macrophage counts. FLOT2 expression had a positive relation with naïve B cell, activated DC, and T follicular helper cell counts, whereas it was negatively correlated with M2 macrophage counts.
According to the receiver operating characteristic curve used for diagnostic efficacy verification, the higher the area under the curve (AUC) value, the better the predictive efficiency. The results showed that the AUC values of ANKH, CSRP2, DDIT4, DLEU1, and FLOT2 hub genes were in 0.969 (0.925–1.000), 0.791 (0.624–0.958), 0.836 (0.695–0.977), 0.511 (0.315–0.707), and 0.867 (0.743–0.991), respectively (Figures 5A–E), while the AUC values of these combined-five genes approached to 1 (Figure 6A). The results suggest that the ANKH, CSRP2, DDIT4 and FLOT2 can efficiently predict POAG occurrence and development separately. Moreover, the five hub genes impacted more effectively as a combined clinical biomarker.
FIGURE 5. ROC curves and GSEA analyses of hub genes. The AUCs of each gene were ANKH-AUC: 0.969 (0.925–1.000), CSRP2-AUC: 0.791 (0.624–0.958), DDIT4-AUC: 0.836 (0.695–0.977), DLEU1-AUC: 0.511 (0.315–0.707) and FLOT2-AUC: 0.867 (0.743–0.991) individually (A–E). GSEA analysis showed that ANKH enriched in AXON GUIDANCE, GLYCOSAMINOGLYCAN BIOSYNTHESIS CHONDROITIN SULFATE and MATURITY ONSET DIABETES OF THE YOUNG. CSRP2 enriched in GLYCOSAMINOGLYCAN BIOSYNTHESIS CHONDROITIN SULFATE and GLYCOSAMINOGLYCAN BIOSYNTHESIS HEPARAN SULFATE. DDIT4 enriched in MTOR SIGNALING PATHWAY and O GLYCAN BIOSYNTHESIS. DLEU1 enriched in CHEMOKINE SIGNALING PATHWAY and GLYCOSPHINGOLIPID BIOSYNTHESIS LACTO AND NEOLACTO SERIES. FLOT2 enriched in BIOSYNTHESIS OF UNSATURATED FATTY ACIDS and DRUG METABOLISM OTHER ENZYMES (F–J).
FIGURE 6. ROC curve of combined clinical biomarker and ssGSEA analyses of hub genes. The AUC of the combined clinical biomarker reached to 1 (A). Hub genes were all related to immune cells (B). FLOT2 was closely related to CCR, HLA, pDCs, Tfh, Th1_cells, Th2_cells, Type_I_IFN_Reponce and Type_II_IFN_Reponce. DLEU1 was related to Macrophages, T_cell_co.stimulation, TIL and Type_II_IFN_Reponce. DDIT4 was relative with Check. point, Netrophils, T_cell_co.inhibition, T_cell_co.stimulation and TIL all negatively. CSRP2 was closely related to aDCs, Check. point, DCs, T_cell_co.stimulation and Type_II_IFN_Reponce. ANKH was closely related to aDCs, Check. point, DCS, HLA, Mast_cells, Netrophils, Parainflammation, T_cell_co.stimulation, Tfh, Th2_cells,TIL, Type_I_IFN_Reponce and Type_II_IFN_Reponce.
We investigated specific signaling pathways related to hub genes and explored the potential molecular mechanism of POAG progression. The enrichment results are shown in Figures 5F–J. GSEA showed that ANKH was enriched in axon guidance, glycosaminoglycan biosynthesis chondroitin sulfate, and maturity-onset diabetes of the young. CSRP2 was enriched in glycosaminoglycan, chondroitin sulfate, glycosaminoglycan, and heparan sulfate biosynthesis. DDIT4 was enriched in mammalian target of rapamycin signaling pathway and O-glycan biosynthesis. DLEU1 was enriched in the chemokine signaling pathway, glycosphingolipid biosynthesis and lacto and neolacto series. FLOT2 was enriched in the biosynthesis of unsaturated fatty acids, drug metabolism, and other enzymes.
We performed ssGSEA analysis with Spearman correlation analysis between five core genes and ssGSEA immune cells. The results showed hub genes were all related to immune cells (Figure 6B). FLOT2 was closely related to CCR, HLA, pDCs, Tfh, Th1_cells, Th2_cells, Type_I_IFN_Reponce and Type_II_IFN_Reponce. DLEU1 was related to Macrophages, T_cell_co.stimulation, TIL and Type_II_IFN_Reponce. DDIT4 was relative with Check. point, Netrophils, T_cell_co.inhibition, T_cell_co.stimulation and TIL all negatively. CSRP2 was closely related to aDCs, Check. point, DCs, T_cell_co.stimulation and Type_II_IFN_Reponce. ANKH was closely related to aDCs, Check. point, DCS, HLA, Mast_cells, Netrophils, Parainflammation, T_cell_co.stimulation, Tfh, Th2_cells,TIL, Type_I_IFN_Reponce and Type_II_IFN_Reponce.
In this study, we constructed a ceRNA network in TM from patients with POAG and identified five core genes as potential biomarkers by screening and validating next-generation sequencing datasets. In recent years, ceRNA has attracted a lot of attention from academic groups. It is represented as a new view of the regulated gene expression module. ceRNA regulation is more sophisticated and complicated than miRNA regulation, which involves more RNA molecules, such as mRNA, pseudogenes of the coding gene, long non-coding RNAs, miRNA, and lncRNA. The miRcode (http://www.mircode.org/) is a public database used to query the relationship between lncRNAs and miRNAs. It covers the complete GENCODE-annotated transcriptome, including non-coding RNA genes over 10,000 in length. By intersecting the identified lncRNA–miRNA and miRNA–mRNA pairs, we constructed the ceRNA network. After calculating the degree of each gene, mRNAs with top five degrees were identified as core genes. In addition, the analysis of core genes and immune infiltration showed a strong correlation with immune cell counts. In addition, the five core genes showed a significant difference between patients and controls with regards to AUC, suggesting that they could predict POAG occurrence and development. We further explored the underlying molecular mechanisms of glaucoma progression, and the result showed 12 pathways were enriched, mainly related to immunity and metabolism. Moreover, ssGSEA showed hub genes were all highly correlated with immune cells. Thus, we assume that the core genes have potential clinical utility as POAG diagnostic markers based on immune infiltration.
Generally, TM in the juxtacanalicular region was mediated to reduce outflow resistance in the conventional outflow pathway and help maintain intraocular pressure (iop) homeostasis (Weinreb et al., 2020). However, induration and reconstruction of TM are involved in the elevated iop often associated with glaucoma. ANKH is the human homolog of the murine ANK gene (Kirsch et al., 2009). ANKH is a multichannel transmembrane protein that transports intracellular pyrophosphate (PPi) to the extracellular milieu, an amino acid comprising 492 base pairs (Mitton-Fitzgerald et al., 2016). It has been demonstrated that the loss of ANKH transporter function in mice led to excessive mineralization because of reduced PPi transport (Ho et al., 2000). Other studies indicated that ANKH took part in chondrocalcinosis articularis (Kumar et al., 2019), fibroblast ossification (He and Dong, 2020), and vascular smooth muscle cell calcification (Chen et al., 2019a), suggesting that ANKH may play a crucial role in the hardening and remodeling of TM cells in POAG patients. This view has been established in studies on POAG identifying novel risk loci (Choquet et al., 2018).
It is universally accepted that the flotillin family is correlated with vesicular invaginations of the plasma membrane and regulation of signal transduction (Zhao et al., 2011). FLOT2 is an oncogene involved in the pathogenic process of several cancers (Liu et al., 2015) (Li et al., 2019). Some researchers believe that FLOT2 is involved in various biological processes, including neuronal differentiation (Bodrikov et al., 2017), adhesion, endocytosis, embryo survival, and phagocytosis, and several signal pathways (Langhorst et al., 2005). In addition, a study on gestational diabetes mellitus showed that the phosphatidylinositol-3-kinase and protein kinase B pathway could be repressed by regulating FLOT2 expression, suggesting FLOT2 inhibition could alleviate insulin resistance and liver gluconeogenesis (Chen et al., 2019b). Thus, FLOT2, which is associated with the prognosis of a variety of diseases, may fail to serve as an independent POAG biomarker, although it has the potential to be an indicator for disease screening, progression, recurrence, and treatment efficacy.
Many factors are necessary for the development of glaucoma, such as aging, exogenous environmental factors, and endogenous factors, and one of the genetic factors that may determine glaucoma pathogenesis is response to hypoxia and nitric oxide (Lin et al., 2014). A recent study demonstrated that glaucoma progression could be accelerated by the interaction between hypoxia-inducible factor (HIF)-1α and vascular endothelial growth factor (Zhou et al., 2021a). CSRP2 encodes the LIM domain protein CSRP2, which participates in regulating organ development and cell differentiation (Weiskirchen et al., 1997), and is widely expressed in various tissues (Jain et al., 1996). Although the precise cellular function of CSRP2 needs further intensive study, it is known that it can be upregulated in hypoxia via HIF-1-mediated transactivation of the CSRP2 promoter (Hoffmann et al., 2018). Thus, CSRP2 may provide prognostic significance and potential therapeutic targets in POAG management.
DDIT4 is a highly conserved protein composed of 232 amino acids encoded by DDIT4. Although the expression of DDIT4 is ubiquitously at low levels in most adult tissues (Shoshani et al., 2002), it can be induced by multiple cellular stresses, such as hypoxia (Brugarolas et al., 2004). Other scholars suggested that DDIT4 was strongly connected to metabolic disorder changes and metabolic functions (Lipina and Hundal, 2016), including its potential contribution to mitochondrial biology. Another study revealed that it might be a potential biomarker for POAG (Zhou et al., 2021b). Therefore, it will be worthwhile to reassess the position of metabolism in glaucoma since more than one gene informs us about metabolic processes, such as the mammalian target of the rapamycin signaling pathway and the role O-glycan biosynthesis, play in this disease (Liu and Prokosch, 2021).
lncRNA DLEU1 is a new research focus in cancer biology. It is regarded as a promising target for biotherapy in numerous tumors because it participates in various biological processes and indispensable role in the pathophysiology of diseases (Song et al., 2020). Furthermore, DLEU1 has been incorporated in the immune prognostic signature in other diseases (Chen et al., 2020), emphasizing its relationship with immune infiltration and the microenvironment. DLEU1 can also be a biomarker of non-cancerous diseases, like ischemic stroke (Fan et al., 2019), due to its involvement in regulating multiple biological processes and pathways. In addition, it offers an option for estimating the progression and therapeutic effect of the disease.
However, our study has some limitations. First, our results need further validation. Research on cellular and animal levels is required to explore how these potential biomarkers work in POAG progression. Second, since there are not many datasets of trabecular meshwork for glaucoma, we were limited by the source of samples. The size of our research was relatively small. In addition, difficulties in obtaining patients’ clinical information made it difficult to combine the study with clinical features.
This study screened, provided, and validated five core genes as clinical biomarkers and potential therapeutic targets for POAG. In addition, this work comprehensively explained POAG biomarkers from an immunological perspective, making them more widely applicable in clinical practice. These results, however, need to be accepted and applied with caution.
Publicly available datasets were analyzed in this study. This data can be found here: GSE138125 GSE27276.
We thank all the patients for providing organization and related-scholars for sharing test result in public. DZ and JW contributed equally to this work.
This study was funded by grant (81470625) and grant (81790641) from National Natural Science Foundation of China.
The authors declare that the research was conducted in the absence of any commercial or financial relationships that could be construed as a potential conflict of interest.
All claims expressed in this article are solely those of the authors and do not necessarily represent those of their affiliated organizations, or those of the publisher, the editors and the reviewers. Any product that may be evaluated in this article, or claim that may be made by its manufacturer, is not guaranteed or endorsed by the publisher.
Beykin, G., Norcia, A. M., Srinivasan, V. J., Dubra, A., and Goldberg, J. L. (2021). Discovery and Clinical Translation of Novel Glaucoma Biomarkers. Prog. Retin. Eye Res. 80, 100875. doi:10.1016/j.preteyeres.2020.100875
Bodrikov, V., Pauschert, A., Kochlamazashvili, G., and Stuermer, C. A. O. (2017). Reggie-1 and Reggie-2 (Flotillins) Participate in Rab11a-dependent Cargo Trafficking, Spine Synapse Formation and LTP-Related AMPA Receptor (GluA1) Surface Exposure in Mouse Hippocampal Neurons. Exp. Neurol. 289, 31–45. doi:10.1016/j.expneurol.2016.12.007
Brugarolas, J., Lei, K., Hurley, R. L., Manning, B. D., Reiling, J. H., Hafen, E., et al. (2004). Regulation of mTOR Function in Response to Hypoxia by REDD1 and the TSC1/TSC2 Tumor Suppressor Complex. Genes Dev. 18 (23), 2893–2904. doi:10.1101/gad.1256804
Chen, B., Khodadoust, M. S., Liu, C. L., Newman, A. M., and Alizadeh, A. A. (2018). Profiling Tumor Infiltrating Immune Cells with CIBERSORT. Methods Mol. Biol. 1711, 243–259. doi:10.1007/978-1-4939-7493-1_12
Chen, B., Zhao, Y., Han, D., Zhao, B., Mao, Y., Cui, Z.-K., et al. (2019). Wnt1 Inhibits Vascular Smooth Muscle Cell Calcification by Promoting ANKH Expression. J. Mol. Cell Cardiol. 135, 10–21. doi:10.1016/j.yjmcc.2019.07.008
Chen, Q., Hu, L., Huang, D., Chen, K., Qiu, X., and Qiu, B. (2020). Six-lncRNA Immune Prognostic Signature for Cervical Cancer. Front. Genet. 11, 533628. doi:10.3389/fgene.2020.533628
Chen, S. H., Liu, X. N., and Peng, Y. (2019). MicroRNA‐351 Eases Insulin Resistance and Liver Gluconeogenesis via the PI3K/AKT Pathway by Inhibiting FLOT2 in Mice of Gestational Diabetes Mellitus. J. Cel Mol Med 23 (9), 5895–5906. doi:10.1111/jcmm.14079
Choquet, H., Paylakhi, S., Kneeland, S. C., Thai, K. K., Hoffmann, T. J., Yin, J., et al. (2018). A Multiethnic Genome-wide Association Study of Primary Open-Angle Glaucoma Identifies Novel Risk Loci. Nat. Commun. 9 (1), 2278. doi:10.1038/s41467-018-04555-4
Dhamija, S., and Menon, M. B. (2018). Non-coding Transcript Variants of Protein-Coding Genes - what Are They Good for? RNA Biol. 15 (8), 1025–1031. doi:10.1080/15476286.2018.1511675
Fan, C. X., Huang, Z. Q., Chen, B. B., Chen, B. J., Wang, Q., Liu, W. D., et al. (2019). Comprehensive Analysis of Key lncRNAs in Ischemic Stroke. Math. Biosci. Eng. 17 (2), 1318–1328. doi:10.3934/mbe.2020066
Gajewski, T. F., Schreiber, H., and Fu, Y.-X. (2013). Innate and Adaptive Immune Cells in the Tumor Microenvironment. Nat. Immunol. 14 (10), 1014–1022. doi:10.1038/ni.2703
Harder, J. M., Williams, P. A., Braine, C. E., Yang, H. S., Thomas, J. M., Foxworth, N. E., et al. (2020). Complement Peptide C3a Receptor 1 Promotes Optic Nerve Degeneration in DBA/2J Mice. J. Neuroinflammation 17 (1), 336. doi:10.1186/s12974-020-02011-z
He, X., and Dong, Y. (2020). Ankylosis Progressive Homolog Upregulation Inhibits Cell Viability and Mineralization during Fibroblast Ossification by Regulating the Wnt/β-catenin S-ignaling P-athway. Mol. Med. Rep. 22 (6), 4551–4560. doi:10.3892/mmr.2020.11576
Ho, A. M., Johnson, M. D., and Kingsley, D. M. (2000). Role of the Mouse Ank Gene in Control of Tissue Calcification and Arthritis. Science 289 (5477), 265–270. doi:10.1126/science.289.5477.265
Hoffmann, C., Mao, X., Brown-Clay, J., Moreau, F., Al Absi, A., Wurzer, H., et al. (2018). Hypoxia Promotes Breast Cancer Cell Invasion through HIF-1α-Mediated Up-Regulation of the Invadopodial Actin Bundling Protein CSRP2. Sci. Rep. 8 (1), 10191. doi:10.1038/s41598-018-28637-x
Jain, M. K., Fujita, K. P., Hsieh, C.-M., Endege, W. O., Sibinga, N. E. S., Yet, S.-F., et al. (1996). Molecular Cloning and Characterization of SmLIM, a Developmentally Regulated LIM Protein Preferentially Expressed in Aortic Smooth Muscle Cells. J. Biol. Chem. 271 (17), 10194–10199. doi:10.1074/jbc.271.17.10194
Jiang, W., Meng, K., and Yang, T. (2019). Long Non-coding RNA PROX1-AS1 Promotes the Proliferation and Migration in Gastric Cancer by Epigenetically Activating FGFR1[J]. Panminerva Med.
Kirsch, T., Kim, H. J., and Winkles, J. A. (2009). Progressive Ankylosis Gene (Ank) Regulates Osteoblast Differentiation. Cells Tissues Organs 189 (1-4), 158–162. doi:10.1159/000151725
Kong, N., Bao, Y., Zhao, H., Kang, X., Tai, X., Chen, X., et al. (2021). Long Noncoding RNA LINC01518 Modulates Proliferation and Migration in TGF-Β1-Treated Human Tenon Capsule Fibroblast Cells through the Regulation of hsa-miR-216b-5P[J]. Neuromolecular Med. doi:10.1007/s12017-021-08662-2
Kumar, P., Sharma, A., Das, S., Srivastava, R., and Gupta, N. (2019). Association of 4-basepair G-To-A Transition in the 5'-untranslated Region of ANKH Gene with Selected Patients of Primary Knee Osteoarthritis: A Cross Sectional Study. J. Fam. Med Prim Care 8 (9), 2937–2941. doi:10.4103/jfmpc.jfmpc_471_19
Langhorst, M. F., Reuter, A., and Stuermer, C. A. (2005). Scaffolding Microdomains and beyond: the Function of Reggie/flotillin Proteins. Cell Mol Life Sci 62 (19-20), 2228–2240. doi:10.1007/s00018-005-5166-4
Li, T., Cao, C., Xiong, Q., and Liu, D. (2019). FLOT2 Overexpression Is Associated with the Progression and Prognosis of Human Colorectal Cancer. Oncol. Lett. 17 (3), 2802–2808. doi:10.3892/ol.2019.9882
Lin, H.-J., Chen, W.-L., Chen, T.-H., Kung, Y.-J., and Wan, L. (2014). Vascular Endothelial Growth Factor -460 C/T BstUI Gene Polymorphism Is Associated with Primary Open Angle Glaucoma. BioMed 4, 4. doi:10.7603/s40681-014-0004-3
Lipina, C., and Hundal, H. S. (2016). Is REDD1 a Metabolic Éminence Grise ? Trends Endocrinol. Metab. 27 (12), 868–880. doi:10.1016/j.tem.2016.08.005
Liu, H., and Prokosch, V. (2021). Energy Metabolism in the Inner Retina in Health and Glaucoma[J]. Int. J. Mol. Sci. 22 (7). doi:10.3390/ijms22073689
Liu, R., Xie, H., Luo, C., Chen, Z., Zhou, X., Xia, K., et al. (2015). Identification of FLOT2 as a Novel Target for microRNA-34a in Melanoma. J. Cancer Res. Clin. Oncol. 141 (6), 993–1006. doi:10.1007/s00432-014-1874-1
Maddineni, P., Kasetti, R. B., Patel, P. D., Millar, J. C., Kiehlbauch, C., Clark, A. F., et al. (2020). CNS Axonal Degeneration and Transport Deficits at the Optic Nerve Head Precede Structural and Functional Loss of Retinal Ganglion Cells in a Mouse Model of Glaucoma. Mol. Neurodegeneration 15 (1), 48. doi:10.1186/s13024-020-00400-9
Mitton-Fitzgerald, E., Gohr, C. M., Bettendorf, B., and Rosenthal, A. K. (2016). The Role of ANK in Calcium Pyrophosphate Deposition Disease. Curr. Rheumatol. Rep. 18 (5), 25. doi:10.1007/s11926-016-0574-z
Namekata, K., Guo, X., Kimura, A., Arai, N., Harada, C., and Harada, T. (2019). DOCK8 Is Expressed in Microglia, and it Regulates Microglial Activity during Neurodegeneration in Murine Disease Models. J. Biol. Chem. 294 (36), 13421–13433. doi:10.1074/jbc.ra119.007645
Shoshani, T., Faerman, A., Mett, I., Zelin, E., Tenne, T., Gorodin, S., et al. (2002). Identification of a Novel Hypoxia-Inducible Factor 1-Responsive Gene, RTP801 , Involved in Apoptosis. Mol. Cel Biol 22 (7), 2283–2293. doi:10.1128/mcb.22.7.2283-2293.2002
Song, C., Zhang, J., Zhao, Z., Yang, Y., Meng, D., Wang, J., et al. (2020). DLEU1: A Functional Long Noncoding RNA in Tumorigenesis. Cpd 26 (15), 1742–1748. doi:10.2174/1381612826666200122145305
Tham, Y.-C., Li, X., Wong, T. Y., Quigley, H. A., Aung, T., and Cheng, C.-Y. (2014). Global Prevalence of Glaucoma and Projections of Glaucoma Burden through 2040. Ophthalmology 121 (11), 2081–2090. doi:10.1016/j.ophtha.2014.05.013
Tribble, J. R., Harder, J. M., Williams, P. A., and John, S. W. M. (2020). Ocular Hypertension Suppresses Homeostatic Gene Expression in Optic Nerve Head Microglia of DBA/2 J Mice. Mol. Brain 13 (1), 81. doi:10.1186/s13041-020-00603-7
Weinreb, R. N., Robinson, M. R., Dibas, M., and Stamer, W. D. (2020). Matrix Metalloproteinases and Glaucoma Treatment. J. Ocul. Pharmacol. Ther. 36 (4), 208–228. doi:10.1089/jop.2019.0146
Weiskirchen, R., Erdel, M., Utermann, G., and Bister, K. (1997). Cloning, Structural Analysis, and Chromosomal Localization of the HumanCSRP2Gene Encoding the LIM Domain Protein CRP2. Genomics 44 (1), 83–93. doi:10.1006/geno.1997.4855
Yao, K., Yu, Y., Li, F., Jin, P., Deng, C., and Zhang, H. (2020). Integrative Analysis of an lncRNA-associated C-ompeting E-ndogenous RNA N-etwork in H-uman T-rabecular M-eshwork C-ells under O-xidative S-tress. Mol. Med. Rep. 21 (3), 1606–1614. doi:10.3892/mmr.2020.10955
Zhang, J., and Wang, R. (2021). Deregulated lncRNA MAGI2-AS3 in Alzheimer's Disease Attenuates Amyloid-β Induced Neurotoxicity and Neuroinflammation by Sponging miR-374b-5p. Exp. Gerontol. 144, 111180. doi:10.1016/j.exger.2020.111180
Zhao, F., Zhang, J., Liu, Y.-S., Li, L., and He, Y.-L. (2011). Research Advances on Flotillins. Virol. J. 8, 479. doi:10.1186/1743-422x-8-479
Zhou, J., Chen, F., Yan, A., and Xia, X. (2021). Role of Mammalian Target of Rapamycin in Regulating HIF-1α and Vascular Endothelial Growth Factor Signals in Glaucoma. Arch. Physiol. Biochem. 127 (1), 44–50. doi:10.1080/13813455.2019.1609996
Zhou, Z., Gao, Z., Yan, W., Zhang, Y., Huang, J., and Xiong, K. (2021). Adenosine A3 Receptor Activated in H2O2 Oxidative Stress of Primary Open-Angle Glaucoma. Ann. Transl Med. 9 (7), 526. doi:10.21037/atm-20-6154
Keywords: glaucoma, POAG, ceRNA network, trabecular meshwork, enrichment analysis
Citation: Zhang D, Wu J, Zhang S and Wu J (2022) Identification of Immune Infiltration-Related ceRNAs as Novel Biomarkers for Prognosis of Patients With Primary Open-Angle Glaucoma. Front. Genet. 13:838220. doi: 10.3389/fgene.2022.838220
Received: 17 December 2021; Accepted: 28 February 2022;
Published: 27 May 2022.
Edited by:
Suhua Chang, Peking University Sixth Hospital, ChinaReviewed by:
Xiaoxu Han, The First Affiliated Hospital of China Medical University, ChinaCopyright © 2022 Zhang, Wu, Zhang and Wu. This is an open-access article distributed under the terms of the Creative Commons Attribution License (CC BY). The use, distribution or reproduction in other forums is permitted, provided the original author(s) and the copyright owner(s) are credited and that the original publication in this journal is cited, in accordance with accepted academic practice. No use, distribution or reproduction is permitted which does not comply with these terms.
*Correspondence: Shenghai Zhang, enNoZWVudEAxNjMuY29t; Jihong Wu, amlob25nd3VAZnVkYW4uZWR1LmNu
†These authors have contributed equally to this work
Disclaimer: All claims expressed in this article are solely those of the authors and do not necessarily represent those of their affiliated organizations, or those of the publisher, the editors and the reviewers. Any product that may be evaluated in this article or claim that may be made by its manufacturer is not guaranteed or endorsed by the publisher.
From Frontiers
Learn more about the work of our research integrity team to safeguard the quality of each article we publish.