- 1Équipe Génomique, Bioinformatique et Chimie Moléculaire (EA 7528), Conservatoire National des Arts et Métiers, HESAM Université, Paris, France
- 2Chanel R&T, Department of Skin Knowledge and Women Beauty, Pantin, France
- 3Department of Dermatology, Medical University of Vienna, Vienna, Austria
- 4Christian Doppler Laboratory for Skin Multimodal Analytical Imaging of Aging and Senescence (SKINMAGINE), Medical University of Vienna, Vienna, Austria
- 5Plate-Forme Post-Génomique P3S, Hôpital Pitié-Salpêtrière, Paris, France
- 6Department of Dermatology, Hôpital Henri Mondor and EA 7379 EPIDERM, Créteil, France
Skin aging is an ineluctable process leading to the progressive loss of tissue integrity and is characterized by various outcomes such as wrinkling and sagging. Researchers have identified impacting environmental factors (sun exposure, smoking, etc.) and several molecular mechanisms leading to skin aging. We have previously performed genome-wide association studies (GWAS) in 502 very-well characterized French women, looking for associations with four major outcomes of skin aging, namely, photoaging, solar lentigines, wrinkling, and sagging, and this has led to new insights into the molecular mechanisms of skin aging. Since individual SNP associations in GWAS explain only a small fraction of the genetic impact in complex polygenic phenotypes, we have made the integration of these genotypes into the reference Kegg biological pathways and looked for associations by the gene set enrichment analysis (GSEA) approach. 106 pathways were tested for association with the four outcomes of skin aging. This biological pathway analysis revealed new relevant pathways and genes, some likely specific of skin aging such as the WNT7B and PRKCA genes in the “melanogenesis” pathway and some likely involved in global aging such as the DDB1 gene in the “nucleotide excision repair” pathway, not picked up in the previously published GWAS. Overall, our results suggest that the four outcomes of skin aging possess specific molecular mechanisms such as the “proteasome” and “mTOR signaling pathway” but may also share common molecular mechanisms such as “nucleotide excision repair.”
Introduction
Aging is an ineluctable process leading to the progressive loss of tissue integrity. Skin aging is the most obvious feature of this process and can be characterized by several outcomes such as wrinkling, sagging, and pigmented spots (Raschke and Elsner, 2010). A great effort has been made to determine environmental factors and molecular mechanisms involved in skin aging. The environment greatly impacts skin aging (Guyuron et al., 2009), especially UV exposure that induces a particular phenotype of skin aging called photoaging (Rabe et al., 2006; Yaar and Gilchrest, 2007). However, in this environment-dependent context, genetic factors may also exert a modulatory role. Indeed, heritability studies performed on Caucasian twin subjects suggest a genetic component to the variation of skin aging features that support the use of genome-wide analysis studies (GWAS) on skin aging: 55% for facial wrinkling, 41% for pigmented spots, and 61% for sagging eyelids (Gunn et al., 2009; Jacobs et al., 2014). Over the last 3 years, several GWAS focusing on skin aging have been published and several genes associated with skin aging have been identified, some of which have revealed new molecular mechanisms involved in skin aging: a first GWAS identified an association between the STXBP5L gene and facial photoaging (Le Clerc et al., 2013), a GWAS focusing on sagging eyelids highlighted the possible role of the TGIF1 gene (Jacobs et al., 2014), two GWAS performed on skin youthfulness unraveled, respectively, the KCND2, DIAPH1, and EDEM1 genes (Chang et al., 2014) and the MC1R genes (Liu et al., 2016), and a GWAS identified associations between the four skin color genes MC1R, IRF4, BNC2, and RALY/ASIP and facial pigmented spots (Jacobs et al., 2015; Jacobs et al., 2015), whereas a second GWAS focusing on facial solar lentigines emphasized the possible role of the HLA region in their occurrence (Laville et al., 2016).
However, it has now been well established that individual SNP associations in GWAS do not represent the whole impact of genetics in complex polygenic phenotypes such as skin aging (Maher, 2008) because of the lack of power and multiple testing. These individual SNP associations only pick up specific loci with very strong influence on the phenotype, and they often stop short of providing solid biological mechanisms. To overcome these limitations, strategies that integrate biological knowledge have been developed to look for new associations buried under the significance threshold (Wang et al., 2010a). One of the most popular approaches is to look at the level of biological pathways associated with a phenotype, using the gene set enrichment analysis (GSEA) (Wang et al., 2007). Biological pathways are defined as a set of interacting molecules and reactions between entities (nucleic acid, protein, complex, etc.) forming a biological network and have been classified in categories. For example, “melanogenesis” and “glycolysis” are biological pathways belonging to the “metabolism” category. In the GSEA approach, a pathway is represented by a set of genes involved in a particular biological network. The underlying concept is that various genes involved in the same biological pathway may contain genetic variants, each having a moderate effect that will not be picked up using the GWAS significance threshold. Identifying associations between a complex trait and biological pathways will, thus, be of great interest because it will provide more functional insights to understand the underlying molecular mechanisms and, as a consequence, likely provide further therapeutic or diagnostic targets for specific aspects of skin aging.
In this work, we used a set of 502 well-characterized Caucasian women from the SU.VI.MAX cohort that were genotyped with the Illumina HumanOmni1-Quad BeadChips leading to 795,063 SNPs after quality control. We looked for new associations at the level of biological pathways for four major outcomes of skin aging, namely, photoaging, solar lentigines, wrinkling, and sagging. For each outcome, we performed a classical GWAS using a regression adjusted for various confounding factors, and we then treated the data in the GSEA pipeline to look for pathway associations.
Materials and Methods
Study Design and Population
This population was previously described in detail (Le Clerc et al., 2013). Briefly, in the autumn/winter of 2002–2003, 570 out of the 2,257 middle-aged women living in the Paris area from the SU.VI.MAX cohort (Hercberg et al., 1998) agreed to participate in a research study on skin aging and provided informed consent. The protocol was approved by the Hospital Medicals Ethics Committee of Paris-Cochin (CCPPRB no. 706) and the “Commission Nationale de l’Informatique et des Libertés” (CNIL no. 334641). The study was conducted according to the Declaration of Helsinski Principles. Each participant completed a self-administered questionnaire related to lifetime sun exposure behavior, and three standardized high-resolution digital images (2,008 × 3,032 pixels) of the face were taken under normalized lighting conditions (one frontal view of the face and one of each profile), using a Kodak DCS 760 digital camera with a 105 mm camera lens (Kodak, Paris, France). Moreover, a blood sample was collected from each woman.
Outcome Variables: Phenotype Analyzed
After image acquisition, several skin aging features were assessed by a dermatologist. The Photoaging was evaluated using a scale developed by Larnier et al., (1994), as described in a previous study (Le Clerc et al., 2013). Wrinkles were visually graded by a dermatologist on different areas of the face using a scale from photographs and then a global wrinkling score on the face was computed using principal component analysis and linear regression. The same methodology has been applied to obtain a global score of sagging and a global score of solar lentigines on the face. The photoaging scale and the three outcome scores were analyzed in this study.
Covariates Used for the Statistical Analysis
Several characteristics susceptible to play a role in the onset of the different outcomes analyzed were taken into account: age (in years), body mass index (BMI; in kg.m−2), smoking habits (never, former, and current), and hormonal status (nonmenopausal, menopausal with hormone replacement therapy, and menopausal without hormone replacement therapy). BMI was categorized as underweight or normal (BMI < 25 kg m−2), overweight (25 ≤ BMI < 30 kg m−2), or obese (BMI ≥ 30 kg m−2) according to the World Health Organization (WHO) recommendations (WHO, 1995) Moreover, lifetime sun exposure intensity was estimated by a score based on data collected by a self-reported questionnaire (Ezzedine et al., 2013). The design, validation, and description of this score have been described previously (Guinot et al., 2001).
Genotyping Method
The genotyping method has already been described in detail (Le Clerc et al., 2013). The 529 women were genotyped using Illumina Infinium HumanOmni1-Quad BeadChips (Illumina, San Diego, CA) that contain 1,140,419 markers, and a sample of 250 ng of ADN by individual was used to obtain genotypes. For the analysis, we considered only SNPs, consequently excluding the copy-number variations that represented 91,706 markers on the HumanOmni1-Quad BeadChips. Moreover, 2,182 SNPs located on the Y chromosome were removed.
Quality Control
The quality control steps have been described in a previous study (Le Clerc et al., 2013). Briefly, nine samples with a call rate (percentage of SNPs genotyped by sample) of <95% in the Illumina clusters were removed. The SNPs with a call frequency (percentage of samples genotyped by SNP) of <99% were reclustered and, after that, samples with a call rate of <98% were deleted. In total, after these quality control steps, 56,479 SNPs with a call frequency of <98% (2% of missing data) were excluded. The Hardy–Weinberg equilibrium analysis was performed for each SNP by using an exact statistical test implemented in the PLINK software (Purcell et al., 2007). Thus, 3,866 SNPs, which were not in the Hardy–Weinberg equilibrium (p < 1 × 10–3), were rejected. Finally, we removed 191,123 SNPs with minor allele frequency <1% to avoid errors of genotyping, leaving a total of 795,063 SNPs.
Identification of Population Stratification
To correct for possible population stratification, genotypes were analyzed using EIGENSTRAT utility of the EIGENSOFT package version 4.2 (Price et al., 2006). The two first pass with the EIGENSTRAT software pointed out 18 outliers, who were removed from further analyses. Then, a third pass without outliers was performed to determine the Eigen vectors. In the statistical analysis, we used the top two Eigen vectors as covariates to correct for population substructure in the association analyses.
SNP-Gene and Gene-Pathway Mappings
The 795,063 SNPs were assigned to genes according to their physical position in the hg19 build using ANNOVAR (Wang et al., 2010b). To take into account promoting and regulatory regions, the genes were enlarged 10 kb upstream and downstream. One hundred eighty six KEGG pathways were downloaded from MSigDB (Subramanian et al., 2005). To reduce bias inherent to the different number of genes in the pathways, we only analyzed pathways composed of at least 20 genes and 200 genes maximum. Finally, 364,087 SNPs were mapped in 20,161 genes gathered in 160 pathways.
Statistical Analysis
We first performed GWAS on each of the four outcomes as described in a previous work (Le Clerc et al., 2013). Briefly, for each outcome, the association between each SNP and the outcome was measured using a linear regression with a genotypic model adjusted on the first two Eigenstrat principal components and the potential confounding factors (smoking habits, BMI, hormonal status, lifetime sun exposure intensity, and age) using the PLINK software (Purcell et al., 2007). Then, we looked for associations between the biological pathways and each outcome by performing the gene set enrichment analysis (GSEA) implemented in the GenGen software suite (Wang et al., 2007; 2010a). 5,000 p-value permutations were performed for the normalization of the enrichment score. The significance and correction for multiple tests were based on the 5,000 permutations and finally assessed using the false discovery rate (FDR). Pathways with an FDR below 0.05 were considered as significantly associated with the outcome analyzed and pathways with an FDR between 0.05 and 0.25 were considered as of interest. Additionally, we investigated pathways having FDR <0.25 by looking at their top ranking genes, and for that, we picked up the genes of these pathways for which the associated p values were < 0.01.
Enrichment Analysis
According to the classification of the KEGG pathways, the pathways are assigned to six categories: “environmental information processing,” “cellular processes,” “metabolism,” “organismal system,” “genetic information processing,” and “human disease.” For each outcome, we looked for an enrichment of significant pathways (FDR < 0.25) in the six categories compared to the distribution of these categories in the whole KEGG database. We used a Fisher’s exact test based on hypergeometric distributions to compare the distribution of the significant pathways in each category with the distribution of the pathways of the whole KEGG database in these categories.
Results
We looked for pathway associations using the GSEA pipeline based on 160 pathways selected from KEGG database (Kanehisa et al., 2017) and the four outcomes of skin aging: photoaging, solar lentigines, wrinkling, and sagging. We first selected the pathways with an FDR < 0.05, and then, the pathways with an FDR < 0.25 in common with two or more outcomes.
For the four phenotypes analyzed, five pathways reached the 0.05 FDR threshold. The wrinkling phenotype was associated with the “nucleotide excision repair” (P = 2 × 10−4, FDR = 0.02) and “proteasome” (P = 8 × 10−4, FDR = 0.04) pathways. For these two pathways selected by GSEA, it was interesting to look at their top ranked genes (with associated p values < 0.01), they were, respectively, XPC, MNAT1, and DDB1, and PSMB3, PSMA1, PSMC2, PSMD2, PSMB5, and PSMD4 (Supplementary Table S1). The sagging outcome exhibited three pathways with an FDR below 0.05: the “amino sugar and nucleotide sugar metabolism” pathway (P = 2 × 10−4, FDR = 0.04), the “mTOR signaling pathway” (P = 8 × 10−4, FDR = 0.05), and the “nucleotide excision repair” pathway (P = 8 × 10−4, FDR = 0.05). The top ranked genes of these pathways are also presented in Supplementary Table S1. Interestingly, the sagging and wrinkling phenotypes were both associated with “nucleotide excision repair,” and the only top ranked gene in common was DDB1. No pathway association with an FDR below 0.05 was found for the photoaging or solar lentigine phenotypes.
We then looked for pathways exhibiting an FDR < 0.25, in common with two or more outcomes (Figure 1, Supplementary Tables S2–S5). Eight pathways were shared between two phenotypes: “arrhythmogenic right ventricular cardiomyopathy (ARVC),” “long-term potentiation,” “mTOR signaling pathway,” “phosphatidylinositol signaling system,” “regulation of actin cytoskeleton,” “ribosome,” “thyroid cancer,” and “vasopressin regulated water reabsorption.” No pathway exhibited association with the four aging phenotypes, but three pathways were associated with three phenotypes: “melanogenesis” (Supplementary Figure S1) with photoaging, lentigines, and sagging, and “primary immunodeficiency” (Supplementary Figure S2) and “nucleotide excision repair” with photoaging, sagging, and wrinkles. For the top ranked genes (p value < 0.01) of “primary immunodeficiency,” no gene was found in common for the three outcomes. In contrast, the DDB1 gene for “nucleotide excision repair” and the WNT7B and PRKCA genes for “melanogenesis” were associated with the three outcomes. We further investigated the top-ranked genes of all these pathways in common between phenotypes. Interestingly, for the melanogenesis pathway, the top-ranked genes encoded proteins having identical functions or interacting with each other (Supplementary Table S6; Supplementary Figure S1).
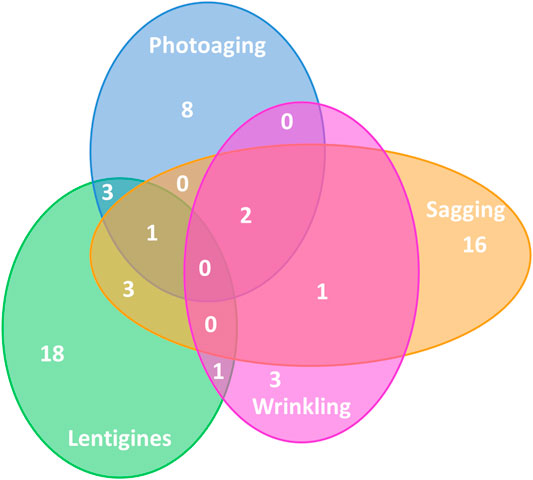
FIGURE 1. Distribution of significant pathways in KEGG database categories. The four circles represent the most significant pathways (FDR < 0.25) for the four outcomes. The number of pathways is indicated in the corresponding area with a total of 14 significant pathways for photoaging, 7 for wrinkles, 23 for sagging, and 25 for lentigines.
For each outcome, we performed an enrichment analysis by comparing the distribution of significant pathways (with FDR < 0.25) in the six categories of KEGG (“environmental information processing,” “cellular processes,” “metabolism,” “organismal system,” “genetic information processing,” and, “human disease”) to the pathway distribution in the categories in the whole KEGG database. Figure 2 illustrates this analysis showing the ratio (R) of the percentage of significant pathways in a category found for each outcome to the percentage of pathways in that category over the whole KEGG database. Using Fisher’s exact test based on hypergeometric distributions, we have computed the statistical significance of the enrichments observed in Figure 2 (see Methods). For wrinkles, “genetic information processing” pathways were over-represented (R = 4.98, p = 1.49 × 10–2). “Organismal system” was over-represented for solar lentigines with nearly half (42.31%, see Supplementary Figure S3) of the associated pathways in this category (R = 2.25, p = 2.3 × 10–3, Figure 2). Moreover, 44.6% of the pathways of the category “organismal system” found for the solar lentigines phenotype belonged to the sub-category, “immune system,” whereas photoaging, sagging, and wrinkling phenotypes obtained, respectively, 0, 16.6, and 0% of associated pathways in this sub-category (data not shown). Finally, the “metabolism” category is underrepresented for the lentigine outcomes compared to the KEGG database with R = 0.31 and p = 2.1 × 10–3 (Figure 2).
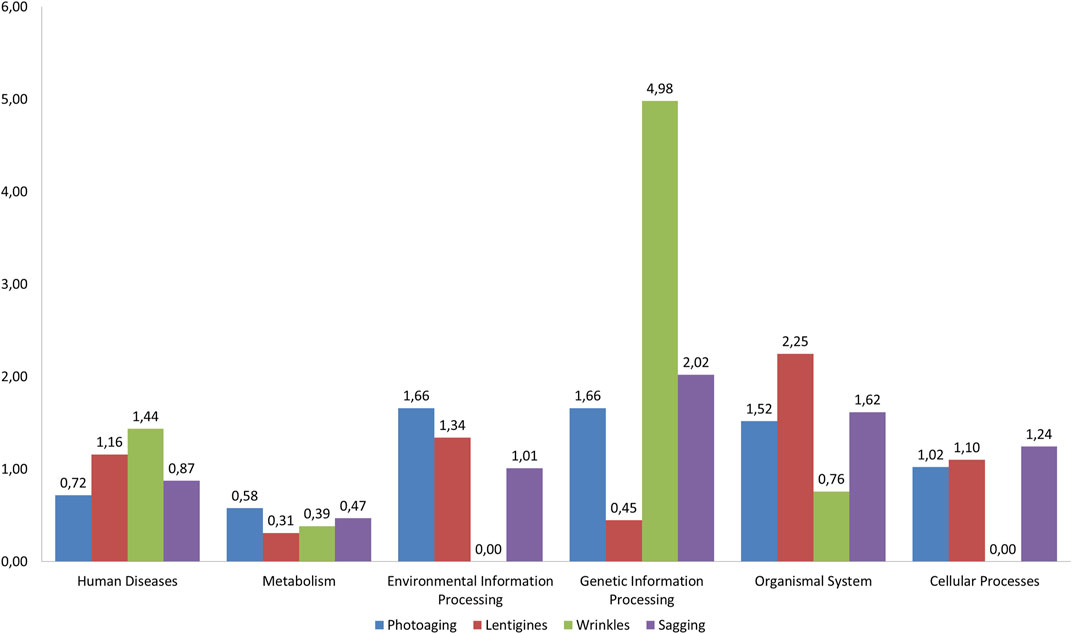
FIGURE 2. Diagram of pathways of interest for the four outcomes. For each phenotype, the most significant pathways (FDR < 25%) have been considered. There were 14 pathways for photoaging, 7 pathways for wrinkles, 23 pathways for sagging, and 26 for lentigines (see Figure 1). These pathways were assigned to one of the six KEGG categories (shown in abscissa of the figure). The ratio (R) of the percentage of significant pathways in a category for each phenotype to the percentage of pathways in a category for KEGG was computed (shown in the Y-axis).
Discussion
We have performed a pathway-based genome-wide association study on four skin aging outcomes, namely, photoaging, solar lentigines, sagging, and wrinkling. The pathway-based approach looks for associations with a set of genes involved in the same biological function (i.e., pathway). Therefore, by taking into account prior biological knowledge, this approach leads to a more comprehensive integration of underlying biological mechanisms than the usual GWAS single SNP approach. We first investigated the results for individual outcomes and then looked for shared pathways, in order to underline specific and shared mechanisms.
The main results for the wrinkles were the “nucleotide excision repair” and “proteasome” pathways. These two pathways are directly related to the maintenance of DNA and protein integrity, and are thus highly relevant, since accumulation of genetic damage throughout life (López-Otín et al., 2013; Moskalev et al., 2013), impairment of protein homeostasis, and proteostasis (Powers et al., 2009; López-Otín et al., 2013) play a key role in aging and also in skin cancer, the ultimate step of skin aging. The investigation of the top-ranked genes of the “nucleotide excision repair” pathway yielded relevant biological evidence: XPC and DDB1 are involved in Xedoderma pigmentosum (XP), which is a pigmentary skin disorder characterized by solar hypersensitivity of the skin with dermal atrophy and high predisposition for developing cutaneous melanoma and squamous cell carcinoma of the skin (Li et al., 1993; Puumalainen et al., 2016). The symptom of XP reflects a deficiency of the “nucleotide excision repair” pathway in the maintenance of the genome stability in human skin (Marteijn et al., 2014), and could be used as a model of accelerated photoaging, emphasizing a putative role of XPC and DDB1. In contrast, no gene in the “proteasome” pathway was directly linked with aging, although deeper investigation of this pathway is of interest.
Regarding the sagging phenotype, the “amino sugar and nucleotide sugar metabolism” pathway yielded the best FDR and the three most associated genes were PMM2, GFPT2, and GMDS. PMM2 is involved in a metabolism disorder linked with abnormal fat distribution and skin wrinkling (Kouwenberg et al., 2014). A decrease in the expression of the GFPT2 and GMDS genes is observed with aging (Craig et al., 2015). Additionally, sagging was also associated with the “nucleotide excision repair” pathway, pointing out several genes, namely DDB1 and RAD23B, which are both involved in XP (Kapetanaki et al., 2006; Petruseva et al., 2009), and ERCC8 associated with the Cokayne syndrome (Ridley et al., 2005), another disease characterized by sun hypersensitivity and progeroid appearance, but without an association with skin cancer. Finally, sagging was associated with the “mTOR signaling pathway.” Strikingly, the inhibition of the mTOR pathway has been experimentally linked to an extension of lifespan in animal models and protects against a growing list of age-related pathologies (Johnson et al., 2013).
Three pathways were commonly associated with three outcomes. In detail, the “melanogenesis” pathway was associated with photoaging, solar lentigines, and sagging, whereas the “nucleotide excision repair” and “primary immunodeficiency” pathways were associated with photoaging, sagging, and wrinkling. Among the top-ranked genes, three genes were shared by three outcomes: DDB1 belonging to the “nucleotide excision repair” pathway, and WNT7B and PRKCA belonging to “melanogenesis.” The DDB1 gene encodes a protein belonging to the UV–DDB (ultraviolet radiation–DNA damage-binding protein) complex, which directly binds to UV-radiation-induced lesions and functions as an auxiliary damage-recognition factor (Marteijn et al., 2014). Hence, DDB1 defect/modulation might be involved in photoaging, sagging, and wrinkling in response to UV exposure. The WNT7B gene is a member of the WNT gene family, known to encode proteins that have been implicated in oncogenesis and in several developmental processes, including the regulation of cell fate and patterning during embryogenesis. The PRKCA gene encodes a protein kinase, belonging to the PKC family, known to phosphorylate a wide variety of protein targets and involved in diverse cellular signaling pathways. Interestingly, for the “melanogenesis” pathway common for three outcomes, the top-ranked genes of the three outcomes encoded proteins with identical functions or interacting with each other. For example, we found several genes encoding adenylate cyclases, and they contribute to the same function in the pathway (Supplementary Table S6). A functional investigation of these proteins and their role in the “melanogenesis” pathway could explain the differences between the three outcomes and lead to new insights into their mechanisms of action.
Beyond analyzing the pathways one by one, it seems important to have a global overview of these pathways and shared mechanisms as skin aging is probably a complex process involving a combination of pathways. Strikingly, the “metabolism” category was poorly represented in the four outcomes (especially when compared with its KEGG distribution). In contrast, we observed an over-representation of pathways from the “genetic information processing” category associated with wrinkles (compared with KEGG). This observation may recall the influence of UV exposure on aging for the induction of DNA damage and development of wrinkles (Moskalev et al., 2013). Finally, “organismal system” was the most represented category for lentigines (Supplementary Figure S3, Figure 2). For the solar lentigines phenotype, the subclass “immune system” represented 45.4% of the pathways from “organismal system” (five pathways), underlying a putative involvement of the immune system for the solar lentigines in accordance with previous reports (Aoki et al., 2007; Laville et al., 2016).
The pathway-based approach is powerful but has a few limitations. For this analysis, we used the pathways from the KEGG database, and since the understanding of human gene function is incomplete and some genes are not yet characterized, the curated pathways of the database may not be fully representative of all the biological networks. Additionally, in GSEA, the best SNP (weaker p value from the GWAS analysis) is chosen to represent a gene, and this approach is limitative knowing that several variants in the same gene may contribute to the overall association signal. However, some of these limitations have been overcome by several studies (Segrè et al., 2010; Mei et al., 2016; Marczyk et al., 2021).
In conclusion, this is the first pathway-based analysis on skin aging performed on a cohort of 502 very-well-characterized women. Our study points to specific and/or shared highly relevant pathways in photoaging, solar lentigines, wrinkling, and sagging phenotypes. Results of this work may help to further identify diagnostic or therapeutic targets for specific skin aging aspects. Interestingly, some of the identified pathways may be shared by aging features that involve other organs. As for any large-scale study, a replication in other cohorts will be needed to confirm these results.
Data Availability Statement
The original contributions presented in the study are included in the article/Supplementary Material, further inquiries can be directed to the corresponding authors.
Ethics Statement
The studies involving human subjects were reviewed and approved by the Hospital Medicals Ethics Committee of Paris-Cochin (CCPPRB no. 706) and the “Commission Nationale de l’Informatique et des Libertés” (CNIL no. 334641). The patients provided their written informed consent to participate in this study.
Author Contributions
RJ, JL, LE and FG measured and prepared the phenotypes. MR, VL, CC, TL, WC and SL prepared the genotypes. MR, VL, and SL performed the pathway analyses. MR, J-LS, SL, and JL performed the statistical analyses. SL, JL, KE and J-FZ performed the interpretation of the results. JL, FM, ET and J-FZ designed the study. MR, SL, J-LS, KE and J-FZ wrote the manuscript. All the authors have read and corrected the manuscript.
Conflict of Interest
The authors declare that the research was conducted in the absence of any commercial or financial relationships that could be construed as a potential conflict of interest.
Publisher’s Note
All claims expressed in this article are solely those of the authors and do not necessarily represent those of their affiliated organizations, or those of the publisher, the editors, and the reviewers. Any product that may be evaluated in this article, or claim that may be made by its manufacturer, is not guaranteed or endorsed by the publisher.
Acknowledgments
The authors gratefully acknowledge the SU.VI.MAX cohort who kindly collaborated to perform this genomic study. They also gratefully acknowledge the financial support of the Federal Ministry for Digital and Economic Affairs of Austria and the National Foundation for Research, Technology, and Development of Austria to the Christian Doppler Laboratory for Skin Multimodal Imaging of Aging and Senescence.
Supplementary Material
The Supplementary Material for this article can be found online at: https://www.frontiersin.org/articles/10.3389/fgene.2022.836581/full#supplementary-material
Abbreviations
FDR, false discovery rate; GSEA, gene set enrichment analysis; GWAS, genome-wide association studies; SNP, single-nucleotide polymophirsm; UV–DDB, ultraviolet radiation–DNA damage-binding protein; and XP, xedoderma pigmentosum.
References
Aoki, H., Moro, O., Tagami, H., and Kishimoto, J. (2007). Gene Expression Profiling Analysis of Solar Lentigo in Relation to Immunohistochemical Characteristics. Br. J. Dermatol. 156, 1214–1223. doi:10.1111/j.1365-2133.2007.07830.x
Chang, A. L. S., Atzmon, G., Bergman, A., Brugmann, S., Atwood, S. X., Chang, H. Y., et al. (2014). Identification of Genes Promoting Skin Youthfulness by Genome-wide Association Study. J. Invest. Dermatol. 134, 651–657. doi:10.1038/jid.2013.381
Craig, T., Smelick, C., Tacutu, R., Wuttke, D., Wood, S. H., Stanley, H., et al. (2015). The Digital Ageing Atlas: Integrating the Diversity of Age-Related Changes into a Unified Resource. Nucleic Acids Res. 43, D873–D878. doi:10.1093/nar/gku843
Ezzedine, K., Mauger, E., Latreille, J., Jdid, R., Malvy, D., Gruber, F., et al. (2013). Freckles and Solar Lentigines Have Different Risk Factors in Caucasian Women. J. Eur. Acad. Dermatol. Venereol. 27, e345–e356. doi:10.1111/j.1468-3083.2012.04685.x
Guinot, C., Latreille, J., Malvy, D., Preziosi, P., Galan, P., Hercberg, S., et al. (2001). Use of Multiple Correspondence Analysis and Cluster Analysis to Study Dietary Behaviour: Food Consumption Questionnaire in the SU.VI.MAX. Cohort. Eur. J. Epidemiol. 17, 505–516. doi:10.1023/a:1014586129113
Gunn, D. A., Rexbye, H., Griffiths, C. E. M., Murray, P. G., Fereday, A., Catt, S. D., et al. (2009). Why Some Women Look Young for Their Age. PLoS One 4, e8021. doi:10.1371/journal.pone.0008021
Guyuron, B., Rowe, D. J., Weinfeld, A. B., Eshraghi, Y., Fathi, A., and Iamphongsai, S. (2009). Factors Contributing to the Facial Aging of Identical Twins. Plast. Reconstr. Surg. 123, 1321–1331. doi:10.1097/PRS.0b013e31819c4d42
Hercberg, S., Galan, P., Preziosi, P., Roussel, A. M., Arnaud, J., Richard, M. J., et al. (1998). Background and rationale behind the SU.VI.MAX Study, a prevention trial using nutritional doses of a combination of antioxidant vitamins and minerals to reduce cardiovascular diseases and cancers. SUpplementation en VItamines et Minéraux AntioXydants Study. Int. J. Vitam Nutr. Res. 68, 3–20.
Jacobs, L. C., Hamer, M. A., Gunn, D. A., Deelen, J., Lall, J. S., van Heemst, D., et al. (2015). A Genome-wide Association Study Identifies the Skin Color Genes IRF4 , MC1R , ASIP , and BNC2 Influencing Facial Pigmented Spots. J. Invest. Dermatol. 135, 1735–1742. doi:10.1038/jid.2015.62
Jacobs, L. C., Liu, F., Bleyen, I., Gunn, D. A., Hofman, A., Klaver, C. C. W., et al. (2014). Intrinsic and Extrinsic Risk Factors for Sagging Eyelids. JAMA Dermatol. 150, 836–843. doi:10.1001/jamadermatol.2014.27
Johnson, S. C., Rabinovitch, P. S., and Kaeberlein, M. (2013). mTOR Is a Key Modulator of Ageing and Age-Related Disease. Nature 493, 338–345. doi:10.1038/nature11861
Kanehisa, M., Furumichi, M., Tanabe, M., Sato, Y., and Morishima, K. (2017). KEGG: New Perspectives on Genomes, Pathways, Diseases and Drugs. Nucleic Acids Res. 45, D353–D361. doi:10.1093/nar/gkw1092
Kapetanaki, M. G., Guerrero-Santoro, J., Bisi, D. C., Hsieh, C. L., Rapic-Otrin, V., and Levine, A. S. (2006). The DDB1-Cul4addb2 Ubiquitin Ligase Is Deficient in Xeroderma Pigmentosum Group E and Targets Histone H2A at UV-Damaged DNA Sites. Proc. Natl. Acad. Sci. 103, 2588–2593. doi:10.1073/pnas.0511160103
Kouwenberg, D., Gardeitchik, T., Mohamed, M., Lefeber, D. J., and Morava, E. (2014). Wrinkled Skin and Fat Pads in Patients with ALG8-CDG: Revisiting Skin Manifestations in Congenital Disorders of Glycosylation. Pediatr. Dermatol. 31, e1–e5. doi:10.1111/pde.12233
Larnier, C., Ortonne, J.-P., Venot, A., Faivre, B., Béani, J.-C., Thomas, P., et al. (1994). Evaluation of Cutaneous Photodamage Using a Photographic Scale. Br. J. Dermatol. 130, 167–173. doi:10.1111/j.1365-2133.1994.tb02895.x
Laville, V., Clerc, S. L., Ezzedine, K., Jdid, R., Taing, L., Labib, T., et al. (2016). A Genome-wide Association Study in Caucasian Women Suggests the Involvement ofHLAgenes in the Severity of Facial Solar Lentigines. Pigment Cel Melanoma Res. 29, 550–558. doi:10.1111/pcmr.12502
Le Clerc, S., Taing, L., Ezzedine, K., Latreille, J., Delaneau, O., Labib, T., et al. (2013). A Genome-wide Association Study in Caucasian Women Points Out a Putative Role of the STXBP5L Gene in Facial Photoaging. J. Invest. Dermatol. 133, 929–935. doi:10.1038/jid.2012.458
Li, L., Bales, E. S., Peterson, C. A., and Legerski, R. J. (1993). Characterization of Molecular Defects in Xeroderma Pigmentosum Group C. Nat. Genet. 5, 413–417. doi:10.1038/ng1293-413
Liu, F., Hamer, M. A., Deelen, J., Lall, J. S., Jacobs, L., van Heemst, D., et al. (2016). The MC1R Gene and Youthful Looks. Curr. Biol. 26, 1213–1220. doi:10.1016/j.cub.2016.03.008
López-Otín, C., Blasco, M. A., Partridge, L., Serrano, M., and Kroemer, G. (2013). The Hallmarks of Aging. Cell 153, 1194–1217. doi:10.1016/j.cell.2013.05.039
Maher, B. (2008). Personal Genomes: The Case of the Missing Heritability. Nature 456, 18–21. doi:10.1038/456018a
Marczyk, M., Macioszek, A., Tobiasz, J., Polanska, J., and Zyla, J. (2021). Importance of SNP Dependency Correction and Association Integration for Gene Set Analysis in Genome-wide Association Studies. Front. Genet. 12, 767358. doi:10.3389/fgene.2021.767358
Marteijn, J. A., Lans, H., Vermeulen, W., and Hoeijmakers, J. H. J. (2014). Understanding Nucleotide Excision Repair and its Roles in Cancer and Ageing. Nat. Rev. Mol. Cel Biol 15, 465–481. doi:10.1038/nrm3822
Mei, H., Li, L., Jiang, F., Simino, J., Griswold, M., Mosley, T., et al. (2016). snpGeneSets: An R Package for Genome-wide Study Annotation. G3 (Bethesda) 6, 4087–4095. doi:10.1534/g3.116.034694
Moskalev, A. A., Shaposhnikov, M. V., Plyusnina, E. N., Zhavoronkov, A., Budovsky, A., Yanai, H., et al. (2013). The Role of DNA Damage and Repair in Aging through the Prism of Koch-like Criteria. Ageing Res. Rev. 12, 661–684. doi:10.1016/j.arr.2012.02.001
Petruseva, I. O., Tikhanovich, I. S., Maltseva, E. A., Safronov, I. V., and Lavrik, O. I. (2009). Photoactivated DNA Analogs of Substrates of the Nucleotide Excision Repair System and Their Interaction with Proteins of NER-Competent HeLa Cell Extract. Biochem. Mosc. 74, 491–501. doi:10.1134/s0006297909050034
Powers, E. T., Morimoto, R. I., Dillin, A., Kelly, J. W., and Balch, W. E. (2009). Biological and Chemical Approaches to Diseases of Proteostasis Deficiency. Annu. Rev. Biochem. 78, 959–991. doi:10.1146/annurev.biochem.052308.114844
Price, A. L., Patterson, N. J., Plenge, R. M., Weinblatt, M. E., Shadick, N. A., and Reich, D. (2006). Principal Components Analysis Corrects for Stratification in Genome-wide Association Studies. Nat. Genet. 38, 904–909. doi:10.1038/ng1847
Purcell, S., Neale, B., Todd-Brown, K., Thomas, L., Ferreira, M. A. R., Bender, D., et al. (2007). PLINK: a Tool Set for Whole-Genome Association and Population-Based Linkage Analyses. Am. J. Hum. Genet. 81, 559–575. doi:10.1086/519795
Puumalainen, M.-R., Rüthemann, P., Min, J.-H., and Naegeli, H. (2016). Xeroderma Pigmentosum Group C Sensor: Unprecedented Recognition Strategy and Tight Spatiotemporal Regulation. Cell. Mol. Life Sci. 73, 547–566. doi:10.1007/s00018-015-2075-z
Rabe, J. H., Mamelak, A. J., McElgunn, P. J. S., Morison, W. L., and Sauder, D. N. (2006). Photoaging: Mechanisms and Repair. J. Am. Acad. Dermatol. 55, 1–19. doi:10.1016/j.jaad.2005.05.010
Raschke, C., and Elsner, P. (2010). “Skin Aging: A Brief Summary of Characteristic Changes,” in Textbook of Aging Skin. Editors M. A. Farage, K. W. Miller, and H. I. Maibach (Berlin, Heidelberg: Springer), 37–43. doi:10.1007/978-3-540-89656-2_5
Ridley, A. J., Colley, J., Wynford-Thomas, D., and Jones, C. J. (2005). Characterisation of Novel Mutations in Cockayne Syndrome Type A and Xeroderma Pigmentosum Group C Subjects. J. Hum. Genet. 50, 151–154. doi:10.1007/s10038-004-0228-2
Segrè, A. V., Groop, L., Mootha, V. K., Daly, M. J., and Altshuler, D. (2010). DIAGRAM Consortium, MAGIC investigatorsCommon Inherited Variation in Mitochondrial Genes Is Not Enriched for Associations with Type 2 Diabetes or Related Glycemic Traits. Plos Genet. 6, e1001058. doi:10.1371/journal.pgen.1001058
Subramanian, A., Tamayo, P., Mootha, V. K., Mukherjee, S., Ebert, B. L., Gillette, M. A., et al. (2005). Gene Set Enrichment Analysis: a Knowledge-Based Approach for Interpreting Genome-wide Expression Profiles. Proc. Natl. Acad. Sci. 102, 15545–15550. doi:10.1073/pnas.0506580102
Wang, K., Li, M., and Bucan, M. (2007). Pathway-based Approaches for Analysis of Genomewide Association Studies. Am. J. Hum. Genet. 81, 1278–1283. doi:10.1086/522374
Wang, K., Li, M., and Hakonarson, H. (2010a). Analysing Biological Pathways in Genome-wide Association Studies. Nat. Rev. Genet. 11, 843–854. doi:10.1038/nrg2884
Wang, K., Li, M., and Hakonarson, H. (2010b). ANNOVAR: Functional Annotation of Genetic Variants from High-Throughput Sequencing Data. Nucleic Acids Res. 38, e164. doi:10.1093/nar/gkq603
WHO (1995). Physical Status: the Use and Interpretation of Anthropometry. Report of a WHO Expert Committee. World Health Organ. Tech. Rep. Ser. 854, 1–452.
Keywords: SNP, skin, aging, pathway, GWAS
Citation: Rahmouni M, Laville V, Spadoni J-L, Jdid R, Eckhart L, Gruber F, Labib T, Coulonges C, Carpentier W, Latreille J, Morizot F, Tschachler E, Ezzedine K, Le Clerc S and Zagury J-F (2022) Identification of New Biological Pathways Involved in Skin Aging From the Analysis of French Women Genome-Wide Data. Front. Genet. 13:836581. doi: 10.3389/fgene.2022.836581
Received: 15 December 2021; Accepted: 01 March 2022;
Published: 24 March 2022.
Edited by:
Nicholas K. Moschonas, University of Patras, GreeceReviewed by:
Christos C. Zouboulis, Städtische Klinikum Dessau, GermanyJoanna Zyla, Silesian University of Technology, Poland
Copyright © 2022 Rahmouni, Laville, Spadoni, Jdid, Eckhart, Gruber, Labib, Coulonges, Carpentier, Latreille, Morizot, Tschachler, Ezzedine, Le Clerc and Zagury. This is an open-access article distributed under the terms of the Creative Commons Attribution License (CC BY). The use, distribution or reproduction in other forums is permitted, provided the original author(s) and the copyright owner(s) are credited and that the original publication in this journal is cited, in accordance with accepted academic practice. No use, distribution or reproduction is permitted which does not comply with these terms.
*Correspondence: Erwin Tschachler, ZXJ3aW4udHNjaGFjaGxlckBtZWR1bml3aWVuLmFjLmF0; Jean-François Zagury, emFndXJ5QGNuYW0uZnI=
†These authors share first authorship
‡These authors have contributed equally to this work and share last authorship