- 1Division of Human Genetics, Department of Pathology, University of Cape Town, Medical School Cape Town, Cape Town, South Africa
- 2Institute of Infectious Disease and Molecular Medicine, University of Cape Town, Cape Town, South Africa
- 3Department of Mathematics and Computer Science, University of Kinshasa, Kinshasa, Congo
- 4Centre for Bioinformatics, Department of Informatics, University of Oslo, Oslo, Norway
Findings resulting from whole-genome sequencing (WGS) have markedly increased due to the massive evolvement of sequencing methods and have led to further investigations such as clinical actionability of genes, as documented by the American College of Medical Genetics and Genomics (ACMG). ACMG’s actionable genes (ACGs) may not necessarily be clinically actionable across all populations worldwide. It is critical to examine the actionability of these genes in different populations. Here, we have leveraged a combined WES from the African Genome Variation and 1000 Genomes Project to examine the generalizability of ACG and potential actionable genes from four diseases: high-burden malaria, TB, HIV/AIDS, and sickle cell disease. Our results suggest that ethnolinguistic cultural groups from Africa, particularly Bantu and Khoesan, have high genetic diversity, high proportion of derived alleles at low minor allele frequency (0.0–0.1), and the highest proportion of pathogenic variants within HIV, TB, malaria, and sickle cell diseases. In contrast, ethnolinguistic cultural groups from the non-Africa continent, including Latin American, Afro-related, and European-related groups, have a high proportion of pathogenic variants within ACG than most of the ethnolinguistic cultural groups from Africa. Overall, our results show high genetic diversity in the present actionable and known disease-associated genes of four African high-burden diseases, suggesting the limitation of transferability or generalizability of ACG. This supports the use of personalized medicine as beneficial to the worldwide population as well as actionable gene list recommendation to further foster equitable global healthcare. The results point out the bias in the knowledge about the frequency distribution of these phenotypes and genetic variants associated with some diseases, especially in African and African ancestry populations.
Introduction
NGS analysis contributed to the improvement of patient treatment and clinical care. This development has bridged the gap between healthcare and genomics. Furthermore, variant calling is an important aspect of genomics studies as polymorphism information can be used to influence the discovery of actionable pathogenic variants and therefore impact important clinical decisions. Currently, the definition of actionable pathogenic variants varies among scholars (Bope et al., 2019).
The Clinical Genome Resource (ClinGen) presents actionability as clinically prescribed interventions to a genetic disorder that is effective for prevention, lowered clinical burden or delay for a clinical disease, or improved clinical treatments and outcomes in a previously undiagnosed adult (Hunter et al., 2016). On the other hand, the 100,000 Genomes Project protocol presents actionable genes as variants that can significantly prevent (or result in illness or disability that is clinically significant, severely life-threatening, and clinically actionable) disease morbidity and mortality, if identified before symptoms become apparent. However, in any case, the classification of variants to be clinically actionable or not dependent and can only emerge during the process of seeking ethical approval for the study (Hunter et al., 2016).
Overall, in the current literature and most annotation databases, the classification of pathogenicity differs (Sherry et al., 2001; Wang et al., 2010; Landrum et al., 2016; McLaren et al., 2016). Dorschner et al. (1016) leveraged exome data of European and African populations to dissect actionable pathogenic variants, and the result shows that actionable pathogenic variants were disproportionate between European and African populations with an estimated frequency of approximately 3.4 and 1.2%, respectively. This indicates a deficit in the identification or categorization of pathogenic variants in African populations. A similar study conducted by Amendola et al. (2015) also confirmed the findings of Dorschner et al. (1016). One approach to define actionability is to combine many annotation pipelines during filtering and prioritization of mutations, in which casting vote can be applied respectively to allow better prediction of the targeted variant (Lebeko et al., 2017; Bope et al., 2019). Furthermore, on top of ethical approval, the ancestral/derived minor allele frequency of the variants, segregation evidence, and the number of patients affected with the variants and their status as a de novo mutation can highly be considered.
In this study, we provide a broad assessment of the possible actionability of variants known to be associated with the top four burden African diseases and a list of actionable genes from the American College of Medical Genetics and Genomics (ACMG) using WGS data of 20 worldwide ethnolinguistic cultural groups. This work aims to 1) perform variant join calling on publicly available data from the African Genome Variation and the 1000 Genomes Project to examine the evolutionary variation of pathogenic mutation; 2) perform disease-gene population structure; and 3) examine the heterozygosity ratio, the proportion of ancestral/derived alleles, and the distribution of minor allele frequencies based on selected known disease-genes from four predominant African burden diseases, HIV/AIDS, malaria, TB, and sickle cell disease, and a set of known actionable genes across 20 worldwide ethnolinguistic cultural groups. These diseases have uniquely shaped ethnolinguistic culturally specific groups and continental-specific genomic variations, and therefore offer unprecedented opportunities to map disease genes.
Our results in support with previous findings indicate higher genetic diversity in ethnolinguistic cultural groups from Africa, based on four African burden diseases and associated actionable genes. The results suggest the limitation of transferability or generalizability and support the use of personalized medicine as beneficial to each worldwide population or ethnolinguistic cultural group. In addition, our results point out the bias in the knowledge about the frequency distribution of these phenotypes and genetic variants associated with some diseases, especially in African and African ancestry populations, suggesting further examination of actionable gene lists to improve equitable global healthcare.
Results
Based on the initial sample description of populations and country labels and leveraging the population culture and ethnolinguistic information (Gudykunst and Schmidt, 1987; Michalopoulos, 2012), we grouped 4,932 samples from their country labels into 20 independent ethnolinguistic cultural groups (Supplementary Table S1) and performed an independent joint call (see Materials and Methods), resulting in 90, 641, and 235 curated polymorphisms. We leveraged the dbSNPS database in extracting SNPs associated with 77, 50, 75, 460, and 114 genes known to be associated with tuberculosis, malaria, sickle cell disease, HIV, and ACMG’s actionable genes, respectively (Table 1), to examine the generalizability and actionability of these disease-associated genes from 20 worldwide ethnolinguistic cultural groups.
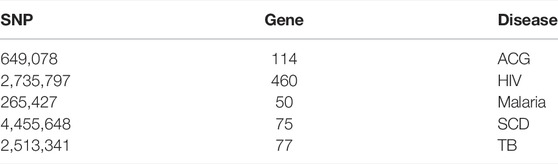
TABLE 1. Number of SNPs after quality control (QC) in each group of genes associated with HIV, TB, SCD, malaria, and actionable genes.
Disease and Actionable Gene-Specific Population Structure
To better characterize the genetic relatedness, we first conducted principal component analysis (PCA) on whole-genome SNPs across all these 20 ethnolinguistic cultural groups (Supplementary Figure S1). Regardless of ethnolinguistic cultural groups, the results in Supplementary Figure S1 show a clear separation between African, European, Indian, and Eastern Asian groups. Second, based on the extracted disease-specific SNPs of different diseases, among these 20 different worldwide ethnolinguistic cultural groups (Materials and Methods), we performed principal component analysis (PCA). This PCA produces a set of orthogonal axes for which the remaining variances in the data are maximized by each successive dimension. Supplementary Table S2 illustrates the genetics distance (Fst) based on disease-specific variants among these 20 ethnolinguistic cultural groups. We present our gene-specific population structure results for HIV (Figure 1A), TB (Figure 1B), malaria (Figure 1C), sickle cell anemia (Figure 1D), and ACG (Figure 1E). Our results show that HIV variation is observed among Bantu, African–American, Khoesan, and Afro-related ethnolinguistic cultural groups, while the European group is clustering together (Figure 1A). Most ethnolinguistic cultural groups from Africa have the highest HIV gene-specific frequency (Figure 1A), confirming that HIV infection has a high incidence or prevalence among ethnolinguistic cultural groups from Africa compared to other ethnolinguistic cultural groups. Moreover, a variation in HIV-specific genes shows little overlap between/within ethnolinguistic cultural groups. The first principal component (PC) separates the European-related ethnolinguistic cultural group cluster and the African-related ethnolinguistic cultural cluster from one end to the other with the Afro-Asiatic ethnolinguistic cultural groups, the African–American, and one part of the Latin-Americans in the middle. The second principal component separates the European-related ethnolinguistic cultural cluster and the East Asian ethnolinguistic cultural group from one end to the other with the United Kingdom/United States–Indian group, the South Asian, and one part of the Latin-American ethnolinguistic cultural group in the middle. We also observe a cline between each axis. The dispersion of samples of HIV-specific genes along the lines suggests the existence of an admixture which may have occurred between ethnolinguistic cultural groups located on the same line and added to a strong local adaptation of HIV-specific genes among ethnolinguistic cultural groups located in the middle of each cline. One interesting observation is the intersection of the Latin-American ethnolinguistic cultural group with the Afro-Asiatic ethnolinguistic cultural groups on one side and the United Kingdom/United States–Indian and South Asian ethnolinguistic cultural groups on the other side which may indicate either a possible existence of HIV-specific actionable genes overlapping between these mentioned populations or a differing effect of these genes across these ethnolinguistic cultural groups. As for HIV, a variation in TB-specific genes was observed among Bantu and Khoesan and Afro-related ethnolinguistic cultural groups (Figure 1B), while European groups are clustering together, except in North European (explaining the known high incidence of TB in Central and North Europe). As the same observation for TB is similar to HIV, then the same comment applies for TB as well. Malaria-specific worldwide ethnolinguistic cultural groups’ genetic structure (Figure 1C) shows that ethnolinguistic cultural groups from Africa and African–American ethnolinguistic cultures are still separated from the rest of the other ethnolinguistic cultural groups. United Kingdom/United States–Indians and Afro-related, Latin-American, and all Europeans are clustering together based on malaria-specific genes, low prevalence, and/or absence of malaria in their geographic regions, indicating that the malaria-specific genes found in one of these aforementioned populations may not be found in the other population. East/South Asians are clustering apart from ethnolinguistic cultural clusters from Africa and Europe continents. While it is known that malaria has a high prevalence among African and Asian populations, the separate cluster between them may indicate different patterns of linkage disequilibrium, geographic location, and genetic variation in malaria-specific genes. As expected, since malaria and sickle cell disease are known to be genetically correlated, similar results for Malaria are observed with sickle-cell disease-specific genes (Figure 1D). The population structure on ACG-specific genes reveals that Africa and European-related ethnolinguistic cultural groups, East-Asian ethnolinguistic cultural groups, and United Kingdom/United States–Indian and South Asian ethnolinguistic cultural groups are separated and clustered in three different clusters (Figure 1E). We observed that African–American and Afro-related ethnolinguistic cultural groups are in the convex of these three clusters (Figure 1E), justifying that they are the result of the admixture of these ethnolinguistic cultural groups considered geographic ancestral populations. In addition, Latin-America is close to European and South Asian clusters, as seen from the results of the admixture, and they are mainly in the convex between East-Asian, South-Asian, and European groups, and a bit distant to the ethnolinguistic cultural groups from Africa. This result indicates that the transferability or generatability of the actionability of these ACG genes may have differing effects across 20 worldwide ethnolinguistic cultural groups.
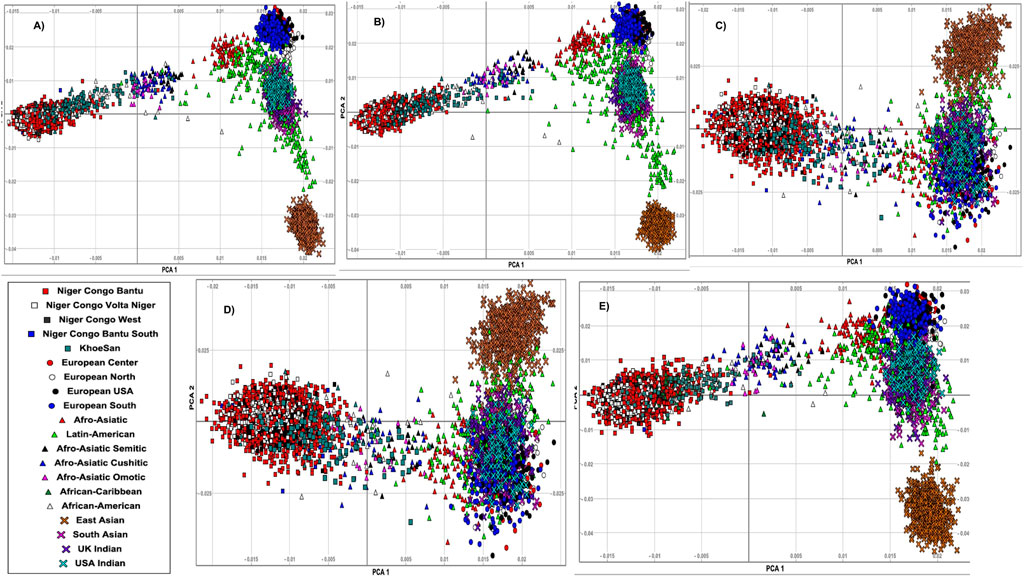
FIGURE 1. Principal component analysis (PCA) of genes associated with (A) HIV-specific, (B) TB-specific, (C) malaria-specific, (D) sickle cell disease-specific, and (E) ACG-specific SNPs and plots of the first and second eigenvectors for 20 ethnolinguistic cultural groups.
Proportion of Pathogenic Polymorphisms Within Disease-Associated Genes
Ethnolinguistic cultural groups from Africa including Bantu and Latin-American and Afro-related groups have a considerable high proportion of pathogenic variants in these HIV-specific genes (Figure 2A). We observe that the Khoesan ethnolinguistic cultural group has a high proportion of pathogenic variants within TB-specific genes (Figure 2B). Latin-American, Afro-Asiatic, and African ancestry (African diaspora)-related ethnolinguistic cultural groups have a high proportion of pathogenic variants (Figure 2B). The low proportion of pathogenic variants is observed across all malaria-specific genes in Bantu, Afro-Asiatic, and Latin-American ethnolinguistic cultural groups (Figure 2C); however, except for toll-like receptor 9 (TLR9), FREM3, IL4, ICAM-1, and nitric oxide synthase 1 (neuronal), the Bantu-related ethnolinguistic cultural groups and Latin-Americans have a high proportion of pathogenic variants (Figure 2C). Bantu, Afro-related ethnolinguistic cultural groups, and Latin America have a similar low proportion of pathogenic variants in most of the sickle cell disease-specific genes, except in MY O 7B, CPS1, COL6A3, MTRR, SLC22A5, ABCC1, and RPL3L (Figure 2D). We observed a considerable high proportion of pathogenic variants within ACG-specific genes from ethnolinguistic cultural groups out of the African continent including Latin America, Afro-Asiatic, and European-related ethnolinguistic cultural groups (Figure 2E), while few genes show a high proportion of pathogenic variants in Niger-Bantu and African–American groups (Figure 2E).
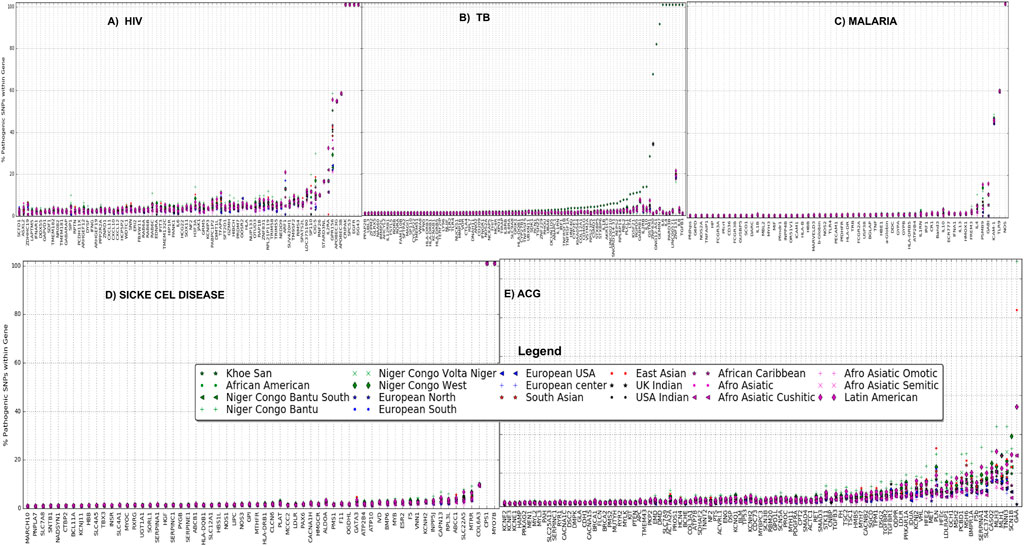
FIGURE 2. Proportion of pathogenic variants within (A) HIV-specific, (B) TB-specific, (C) malaria-specific, (D) sickle cell disease-specific, and (E) ACG-specific (actionable genes) genes among all 20 ethnolinguistic cultural groups.
Distribution of Gene-Specificity in SNP Frequencies
We observed variations in the distribution of MAF at rare variants within MAF bin 0.0–0.05 among these 20 ethnolinguistic cultural groups in four African burden diseases (Supplementary Figures S2A–D) and ACMG’s actionable genes (Supplementary Figure S2E). BTNL2, MOS, CDSN, USP18, MCM8, OAS1, COG4, CCL3L1, HLA-G, HLA-E, STT3A, TMED2, and USP18 have HIV gene-specificity in SNP frequencies ranging between 5 and 15% (Figure 3A) and those ethnolinguistic cultural groups from Africa have the highest. A total of 33 genes have TB gene-specificity in SNP frequencies between 5 and 20% of which all ethnolinguistic cultural groups from Africa have the highest (Figure 3B), suggesting that these genes may harbor common effects and contributions to TB among African ethnolinguistic cultural groups. The distribution of malaria gene-specificity in SNP frequencies from Figure 3C suggests that four genes include GYPB, FCGR2A, IL13, and FREM3 with gene-specificity ranging between 4 and 15%, while all sickle cell disease-related genes (Figure 3D) show low gene-specificity in SNP frequencies ranging between 0.1 and 0.3% among all 20 ethnolinguistic cultural groups, but all ethnolinguistic cultural groups from Africa have the highest frequencies. The distribution of ACG-gene-specificity in SNP frequencies in Figure 3E indicates that all ACG genes have gene-specificity in SNP frequencies lower than 0.4% in all 20 ethnolinguistic cultural groups. However, the gene-specificity in SNP frequencies from most of the ethnolinguistic cultural groups from Africa are higher than those from non-African ethnolinguistic cultural groups, supporting a potential difference effect and contribution of these actionable genes among worldwide ethnolinguistic cultural groups. Supplementary Table S3 shows the details of gene-specificity in SNP frequencies of these ACG and disease burdens across all these 20 ethnolinguistic cultural groups.
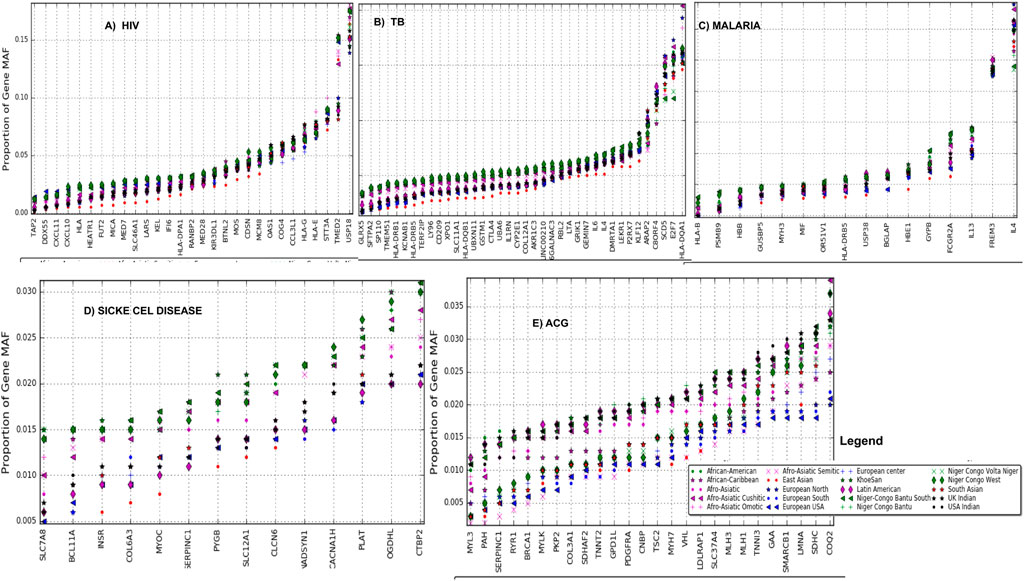
FIGURE 3. Gene-specificity in SNP minor allele frequency: the distribution of the minor allele frequency at the gene level (A) HIV, (B) TB, (C) malaria, (D) sickle cell disease, and (E) ACG (actionable genes) among all ethnolinguistic cultural groups.
Gene-Specific in Proportion of Derived Alleles and Relationship Between Derived and Ethnolinguistic Cultural-Specific Minor Allele Frequency
Derived alleles are more often minor alleles (<50% allele frequency) and associated with risk than ancestral alleles (32). As for the variation observed in the distribution of MAF at rare variants at low ethnolinguistic and cultural-specific minor allele frequencies (ranging between 0.0 and 0.1, Supplementary Figure S3), high variation in the proportion of derived alleles can be observed in HIV (Supplementary Figure S3A), TB (Supplementary Figure S3B), malaria (Supplementary Figure S3C), and sickle cell disease (Supplementary Figure S3D), and a set of actionable genes (Supplementary Figure S3E) across all ethnolinguistic cultural groups from Africa compared to the rest of the other ethnolinguistic cultural groups, and that most of the ethnolinguistic cultural groups from Africa have the highest proportion of derived alleles in the range of minor allele frequency bin (0.0–0.1) (Supplementary Figure S3A), indicating that different mutations and possible selections occurred in rare variants within genes associated with these four African burden diseases, and ACMG’s actionable genes play critical roles and that ethnolinguistic and cultural-specific risk alleles may differentially contribute to the phenotypic variations and clinical outcomes.
To obtain gene-specific proportions of derived alleles, derived allele frequencies were aggregated for all SNPs associated with each of these disease-specific genes (see Materials and Methods). For all African burden diseases including HIV (Figure 4A), TB (Figure 4B), malaria (Figure 4C), and sickle cell diseases (Figure 4D), we observe that Latin America and most of Afro-Asiatic, Bantu, and Khoesan ethnolinguistic cultural groups have a considerable and consistently high proportion of gene-specific derived alleles. We observe a consistent high ACG-gene-specific allele in Latin America and most Afro-related ethnolinguistic cultural groups following most of European-related ethnolinguistic cultural groups (Figure 4E), while a low ACG-gene-specific allele is observed in most of African ethnolinguistic cultural groups. One can expect actionable genes to have a high proportion of derived alleles; however, this is not the case for most of African ethnolinguistic cultural groups, indicating that the current ACG genes were primarily tailored for non-African ethnolinguistic cultural groups. A full list of the ethnolinguistic and cultural gene-specific proportions of derived alleles based on genes associated with these four African burden diseases and ACMG’s actionable genes can be found in Supplementary Table S4.
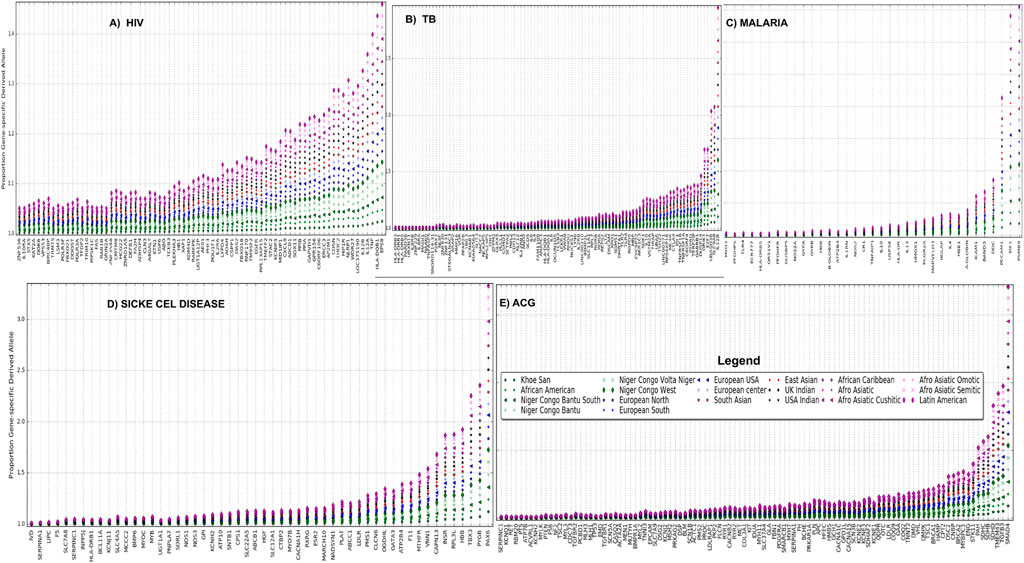
FIGURE 4. (A) HIV, (B) TB, (C) malaria, (D) sickle cell disease, and (E) ACG gene-specific proportion of derived alleles across 20 worldwide ethnolinguistic cultural groups.
Genetic Diversity: Observed and Expected Heterozygosity
Gene diversity consists of two elements including the abundance (or evenness) of the alleles and the number of alleles. The abundance (or evenness) of the alleles and the number of alleles would increase the expected heterozygosity. If an ethnolinguistic cultural group consists of an excess of homozygotes for different alleles, this leads to low-observed heterozygosity. In Figure 5, we observe that ethnolinguistic cultural groups from Africa, particularly Bantus and Khoesan, have the highest gene diversity in HIV, TB, malaria, sickle cell disease, and ACG-associated variants (Supplementary Table S5). This result supports the highest genetic diversity found in individuals and communities across the African continent and that the use of personalized medicine will be beneficial to both the continent and world.
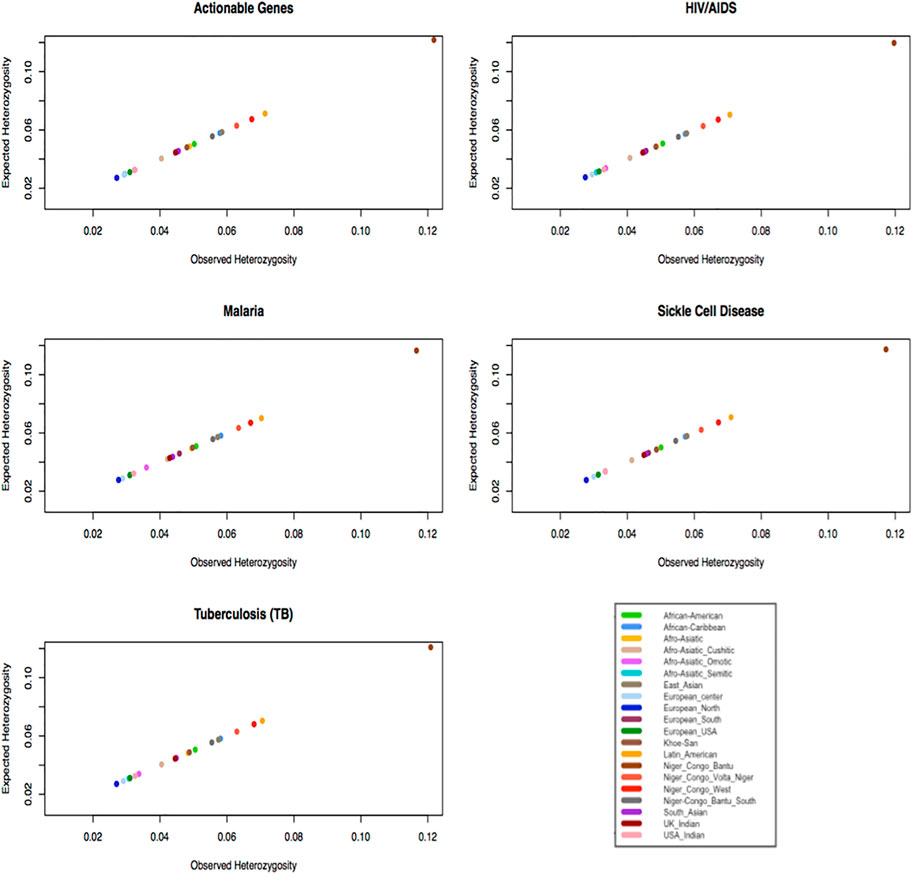
FIGURE 5. Plot expected heterozygosity as a function of observed heterozygosity per gene of specific diseases within ethnolinguistic cultural groups.
Discussion
In this study, we conducted a joint call of 4,932 samples representing 20 worldwide ethnolinguistic cultural groups (Supplementary Table S1), to examine the generalizability and actionability of 77, 50, 75, 460, and 114 genes known to be associated with tuberculosis, malaria, sickle cell disease, HIV, and ACG, respectively (Table 1). To examine the generalizability and actionability of genes, we investigated the distribution of (Bope et al., 2019) gene-specificity in SNP frequencies, (Hunter et al., 2016), gene-specificity in the proportion of derived alleles, and (Sherry et al., 2001) gene-specificity in pathogenic mutations. In addition, population-specific genetic structures and expected heterozygosity were observed in all associated SNPs within genes.
The results of HIV/TB indicated that ethnolinguistic cultural groups including Bantu, Latin American, and Afro-Asiatic have the highest proportion of pathogenic variants based on 483 HIV-specific genes. From 77 TB-specific genes, we observed that Latin American and Afro-Asiatic ethnolinguistic cultural groups have the highest proportion of pathogenic variants, important among all African and African diaspora ethnolinguistic cultural groups, and only Khoesan has a high proportion of pathogenic variants within TB-specific genes. Most ethnolinguistic cultural groups from Africa (Bantu and Khoesan) have the highest HIV and TB gene-specific frequency, indicating that HIV disease risk is prevalent among African ethnolinguistic cultural groups compared with other ethnolinguistic cultural groups. Our result identifies BTNL2, MOS, CDSN, USP18, MCM8, OAS1, COG4, CCL3L1, HLA-G, HLA-E, STT3A, TMED2, and USP18 to have HIV gene-specificity in SNP frequencies ranging between 5 and 15% and those ethnolinguistic cultural groups from Africa have the highest. In addition, 33 genes have TB gene-specificity in SNP frequencies ranging between 5 and 20% of which all African ethnolinguistic cultural groups have the highest frequencies. This suggests that these genes may harbor a common effect and contribution to TB/HIV among African ethnolinguistic cultural groups. Furthermore, HIV/TB gene-specificity has a high proportion of derived alleles at low minor allele frequency (0.0–0.1) from African ethnolinguistic cultural groups and that these proportions of derived alleles vary among African ethnolinguistic cultural groups, suggesting a possible challenge in enabling cross-population actionable gene transferability and possible implementation of precision medicine within different ethnolinguistic cultural groups from Africa.
The results of malaria and sickle cell disease indicate the absence of pathogenic variants in most of the European-related ethnolinguistic cultural groups and a low proportion of pathogenic variants across all malaria-specific genes in Bantu, Afro-Asiatic, and Latin American ethnolinguistic cultural groups, except for toll-like receptor 9 (TLR9), FREM3, IL4, ICAM-1, and nitric oxide synthase 1 (neuronal), indicates that Bantu and Latin America ethnolinguistic cultural groups have a high proportion of pathogenic variants. Furthermore, Bantu, Afro-Asiatic, and Latin American ethnolinguistic cultural groups have a similar low proportion of pathogenic variants in most sickle cell disease-specific genes, except in MY O 7B, CPS1, COL6A3, MTRR, SLC22A5, ABCC1, and RPL3L. We identify four genes including GYPB, FCGR2A, IL13, and FREM3 with malaria gene-specificity in SNP frequencies ranging between 4 and 15%, while all sickle cell disease-related genes have low gene-specificity in SNP frequencies ranging between 0.1 and 0.3% among all 20 ethnolinguistic cultural groups, but all African and diaspora ethnolinguistic cultural groups have the highest in that range.
The result on ACG showed a considerably high proportion of pathogenic variants within ACG-specific genes from non-African ethnolinguistic cultural groups including Latin American, Afro-Asiatic, and European compared to most of African-related ethnolinguistic cultural groups. This result justifies and indicates that the actionability of these ACG genes may have heterogeneous effects on worldwide ethnolinguistic cultural groups, unraveling cross-ethnic group transferability and generalizability to diverse ethnic groups, particularly African from ACG-specific actionable genes daunting. Our result indicates that all ACG genes have gene-specificity in SNP frequencies lower than 0.4% in all 20 ethnolinguistic cultural groups. However, the gene-specificity in SNP frequencies from most of African ethnolinguistic cultural groups are higher than those from non-African ethnolinguistic cultural groups, supporting the potential common effect and contribution of these actionable genes to non-African ethnolinguistic cultural groups. A high ACG-gene-specific derived allele was observed in Latin-American and most Afro-related ethnolinguistic cultural groups following most of European-related ethnolinguistic cultural groups, while a low ACG--specific derived allele is observed in most of African ethnolinguistic cultural groups.
We leveraged the dbSNP database to extract SNPs associated with these genes per disease. The obtained SNPs per disease were thus extracted from the whole phased data containing 4,932 samples of these 20 ethnolinguistic cultural groups, yielding five disease-specific phased haplotype datasets. From these phased haplotype data, we conducted disease gene-specific population structure, and we examined the distribution and relationship of derived and minor allele frequency and estimated the expected and observed heterozygosity.
The result of this study suggests significant genetic variations among all non-European ethnolinguistic cultural groups, mostly African ethnolinguistic cultural groups, while all European ethnolinguistic cultural groups are genetically and consistently clustering together based on these diseases or actionable-specific variants, suggesting limitations of cross-population transferability of actionable or medically relevant genes, given the exceptional polygenicity of human traits. Furthermore, the result indicates that African and African diaspora ethnolinguistic cultural groups, particularly Bantus and Khoesan ethnolinguistic cultural groups, have the highest gene diversity in HIV, TB, malaria, sickle cell disease, and ACG-associated variants. This supports the highest genetic diversity found in individuals and communities across the African continent. Based on these findings, the use of personalized medicine including African genomics will be beneficial to both the continent and world. One of the limitations of this finding is that although these results depend greatly on laboratory experiments, the distribution of actionable genes across populations may depend on continuous genetic diversity, natural selection, and genetic drift. Such study paves the way for a continuous analysis of disease-specific actionable genes and their genetic mechanism underpinning those diseases.
Concluding Remarks
In conclusion, our findings suggest the highest genetic diversity in African ethnolinguistic cultural groups in the four African burden diseases and ACMG’s actionable genes, and that the distribution of gene-specificity (Bope et al., 2019) in SNP frequencies (Hunter et al., 2016), in the proportion of derived alleles, and (Sherry et al., 2001) in pathogenic mutations based on the obtained 77, 50, 75, 460, and 114 genes was known to associate with tuberculosis, malaria, sickle cell disease, HIV, and ACMG’s actionable genes, respectively, indicating significant variation across 20 worldwide ethnolinguistic cultural groups. This suggests (Bope et al., 2019) the limitation of transferability or generalizability; however, the use of personalized medicine will be beneficial to both the African continent and worldwide (Hunter et al., 2016), enabling a recommendation for an African-specific actionable list of genes which will further improve African and diaspora healthcare.
Materials and Methods
Data Description and Quality Check
The data Binary Alignment Map (BAM) files were obtained from the 1000 Genomes Project (1KGP) (Siva, 2008) and the African Genome Variation Project (AGVP) (Gurdasani et al., 2015), which has recently characterized the admixture across 18 ethnolinguistic groups from sub-Saharan Africa as shown in Supplementary Table S1. A quality control check was conducted on the BAM files using SAMtools (Li et al., 2009). After quality check, a total of 2,504 BAM files from the 1000 Genomes Project and 2,428 BAM files from the AGVP were retained. Based on initial sample description population and country labels, we used the population culture and ethnolinguistic information (Gudykunst and Schmidt, 1987; Michalopoulos, 2012) to group populations from the country label into 20 ethnolinguistic cultural groups (Supplementary Table S1). Supplementary Figure S1 illustrates the genetics relatedness and variation of these 20 ethnolinguistic cultural groups, supporting previous findings (Siva, 2008; Chimusa et al., 2015; Gurdasani et al., 2015; Choudhury et al., 2020), and Supplementary File 1 illustrates the genetics distance (Fst) based on disease-specific variants among the 20 ethnolinguistic cultural groups.
Variants Discovery Analysis and Annotation
LoFreq, a variant calling tool, was used to conduct joint calls across 4,932 samples in 20 worldwide ethnolinguistic cultural groups. The resulting variant sets of all 4,932 samples in the VCF file were filtered using SAMtools, and 4,932 samples remained and were considered for downstream analysis.
The resulting joint call VCF file of 4,932 samples and samples were split into 20 VCF files per ethnolinguistic cultural group as listed in Supplementary Table S1. The independent gene-based annotation for each VCF dataset to determine whether SNPs cause protein-coding change and produce a list of amino acids that are affected was conducted using ANNOVAR (Wang et al., 2010). The following setting was used in ANOVA: the population frequency and pathogenicity for each variant were obtained from 1000 Genomes exome, Exome Aggregation Consortium (ExAC), targeted exon datasets, and COSMIC. Gene functions were obtained from RefGene, and different functional predictions were obtained from ANNOVAR’s library, which contains up to 21 different functional scores including SIFT (Ng et al., 2006), LRT (Schwarz et al., 2010), MutationTaster (Reva et al., 2011), MutationAssessor (Shihab et al., 2013), FATHMM and FATHMM-MKL (Liu et al., 2011), RadialSVM (Choi and Chan, 2015), LR (Kim et al., 2017), PROVEAN (Kim et al., 2017), MetaSVM (Dong et al., 2015), MetaLR (Rentzsch et al., 2018), CADD (Davydov et al., 2010), GERP++ (Quang et al., 2014), DANN (Jagadeesh et al., 2016), M-CAP (Ionita-Laza et al., 2016), Eigen (Lu et al., 2015), GenoCanyon (Adzhubei et al., 2010), Polyphen2-HVAR and HDIV (Doerks et al., 2002), PhyloP (Garber et al., 2009), and SiPhy (Loh et al., 2016a). In addition, conservative and segmental duplication sites were included, and the dbSNP code and clinical relevance were reported in dbSNP. From each resulting functional annotated dataset, we independently filtered for the predicted functional status, of which each predicted functional status is of “deleterious” (D), “probably damaging” (D), “disease-causing-automatic” (A), or “disease-causing” (D). The selection of mutations was carried out using the following approach: first, the casting vote approach was implemented in our custom Python script, to retain only a variant if it had at least 17 predicted functional status “D” or “A” out of 21 was used and second, the retained variants from each dataset were further filtered for rarity, exonic variants, and nonsynonymous mutations and with a high-quality call as described previously, yielding a final candidate list of predicted mutant variants in each subject group, including the replication group. We report on the aggregated SiPhy score from all identified mutant SNPs within the gene. The following sections provide details on how SNPs were mapped to genes.
Phased and Haplotypes Inference
To increase the accuracy, the resulting VCF file, containing 4,932 samples of 20 ethnolinguistic cultural groups, was used to further conduct quality control in removing all structured, indel, multi-allelic variants and those with a low minor allele frequency (MAF <0.05) prior to phasing. We first phased and inferred the haplotypes using Eagle (Loh et al., 2016b) from the resulting curated data. We further compared site discordances between these haplotype panels and independently with their original VCF file before phasing. The only site with phase switch-errors showed discrepancies in MAF and was removed.
Disease- and Actionable Gene-Specific Population Structure
We obtained the list of genes, known as medically actionable, and Actionable Genome Consortium (ACG) from https://www.coriell.org/1/NIGMS/Collections/ACMG-73-Genes. The list of genes associated with four major African diseases including malaria, TB, HIV, and sickle cell disease was collected from the GWAS Catalog (https://www.ebi.ac.uk/gwas/), and the extraction was based on phenotype classification and from databases such DisGeNET http://www.disgenet.org/and literature. We obtained 50, 77, 460, 75, and 114 genes known to be associated with tuberculosis, malaria, sickle cell anemia, HIV, and ACG, respectively. We leveraged the dbSNP database to extract SNPs associated with these genes per disease, as shown in Table 1. The obtained SNPs per disease were extracted from the whole phased data containing 4,932 samples of these 20 ethnolinguistic cultural groups, yielding five disease-specific phased haplotype datasets (Table 1).
To evaluate the extent of substructures within disease-specific polymorphism across worldwide ethnolinguistic cultural groups, we leverage each constructed disease-specific phased haplotype dataset, to perform genetic structure analysis based on principal component analysis (PCA) using smartpca, part of the EIGENSOFT 3.0 package (Patterson and Price, 2006). Genesis software http://www.bioinf.wits.ac.za/software/genesis was used to plot PCA.
Proportion of Ancestral/Derived Alleles Among Risk-Conferring Alleles
Each of these four disease-specific phased haplotype datasets was used to analyze the fraction of derived and ancestral alleles and at-risk alleles within each ethnolinguistic cultural group. A previous work showed that derived alleles are more often minor alleles (<50% allele frequency) and associated with risk than ancestral alleles (Gorlova et al., 2012). Therefore, we define risk alleles as follows: if a gene is reported to increase the risk of disease (odd ratio >1) from either the DisGeNET or GWAS Catalog, the risk allele was defined as a minor allele (for all SNPs associated with the gene); otherwise (odd ratio <1), it is defined as a major allele (for all SNPs associated with the gene).
The SNP ancestral alleles were downloaded from the Ensembl, a 59 comparative 32 species alignment (Paten et al., 2008), and we further checked the SNPs for those present in the dbSNP database. Each of these four disease-specific phased haplotype datasets was further annotated using the VCFtools “fillOaa” script (Danecek et al., 2011) with the ancestral allele recorded using the “AA” INFO tag. For each disease-specific dataset, we determined the proportion of risk alleles that were ancestral or derived alleles. We first computed, for each SNP, the fraction of the ancestral allele, which was calculated by dividing the number of times the defined risk allele matched with the ancestral allele by the total number of copies of all alternative alleles across all samples (within each ethnolinguistic cultural group per disease) for a particular SNP. The fraction of the derived allele is equivalent to one minus the fraction of the ancestral allele. As mentioned earlier, derived alleles are more often minor alleles and associated with risk rather than ancestral alleles. Therefore, we investigated the relationship between the fraction of derived alleles, at-risk alleles, and ethnolinguistic cultural group SNP minor allele frequency. To this end, the alternative (minor) alleles were categorized into six bins, (0–0.05, >0.05–0.1, >0.1–0.2, >0.2–0.3, >0.3–0.4, and >0.4–0.5) with respect to each ethnolinguistic cultural dataset frequencies and independently computed the fractions of derived alleles in each bin. Furthermore, we computed the fraction of ancestral/derived alleles for all these known disease-specific genes. To this end, we aggregated the fraction of ancestral/derived alleles at the SNP-based level to gene, considering all SNPs located within the genes’ downstream or upstream region (Chimusa et al., 2015).
Distribution of Minor Allele Frequency and Gene-Specificity in SNP Frequencies
To examine the extent of common variants across these 20 ethnolinguistic cultural groups within a specific disease (TB, HIV, sickle cell anemia, and malaria) and known actionable genes from ACG, the distribution of the minor allele frequency was investigated. To this end, the proportion of minor alleles was categorized into six bins (0–0.05, >0.05–0.1, >0.1–0.2, >0.2–0.3, >0.3–0.4, and >0.4–0.5) with respect to each ethnolinguistic cultural group with a disease. The minor allele frequency (MAF) per SNP for each category was computed using Plink software (Purcell et al., 2007). Furthermore, the fraction of gene-specific in SNP frequency for each gene was computed. To this end, the fraction of gene-specific SNP frequency was computed, assuming that SNPs in upstream and downstream within a gene region are close and possibly in linkage disequilibrium (LD). Minor allele frequency per SNP has aggregated a gene level.
Aggregating SNP Summary Statistics at the Gene Level
SNP-specific allele frequencies or the proportion of ancestral/derived alleles from SNPs 40 kb downstream and upstream within a gene region as per the dbSNP database were aggregated (Chimusa et al., 2016). Under the null hypothesis, frequency/proportion
Key Points
• Personalized medicine including African genomics will be beneficial both to the continent and worldwide.
• Generalizability and transferability of actionable genes are challenging but will improve clinical population healthcare.
• Investigating the distribution of gene-specificity in SNP frequencies, gene-specificity in proportion of derived alleles, and gene-specificity in burden of pathogenic mutations will reveal population-specific actionable genes.
Data Availability Statement
The original contributions presented in the study are included in the article/Supplementary Material; further inquiries can be directed to the corresponding author.
Author Contributions
The authors have equally contributed to writing, interpretation of the results and revision of the manuscript. However, SA and EC carried out all the analysis, and EC designed, administrated and supervised the manuscript.
Funding
The authors are supported in part by the DAAD, the German Academic Exchange Programme, under reference number 91653117, the National Institutes of Health Common Fund under grant number 1U2RTW012131-01 (COBIP), the National Research Foundation of South Africa for funding (NRF) (Grant #RA171111285157/119,056), and the Sub-Saharan African Network for TB/HIV Research Excellence (SANTHE), a DELTAS African Initiative (grant # DEL-15-006). Responsibility for the information and views expressed in the article lies entirely with the authors.
Conflict of Interest
The authors declare that the research was conducted in the absence of any commercial or financial relationships that could be construed as a potential conflict of interest.
Publisher’s Note
All claims expressed in this article are solely those of the authors and do not necessarily represent those of their affiliated organizations, or those of the publisher, the editors, and the reviewers. Any product that may be evaluated in this article, or claim that may be made by its manufacturer, is not guaranteed or endorsed by the publisher.
Acknowledgments
We are grateful to H3ABioNet consortium members for helpful discussions and the CHPC (https://www.chpc.ac.za/) facility for providing a computing platform. We thank Dr. Denis Awany, Ms Jacquiline Mugo and Mr. Joel Defo to have read our manuscript and for their advises and supports during the preparation of this manuscript.
Supplementary Material
The Supplementary Material for this article can be found online at: https://www.frontiersin.org/articles/10.3389/fgene.2022.835713/full#supplementary-material
References
Adzhubei, I. A., Schmidt, S., Peshkin, L., Ramensky, V. E., Gerasimova, A., Bork, P., et al. (2010). A Method and Server for Predicting Damaging Missense Mutations. Nat. Methods 7, 248–249. doi:10.1038/nmeth0410-248
Amendola, L. M., Dorschner, M. O., Robertson, P. D., Salama, J. S., Hart, R., Shirts, B. H., et al. (2015). Actionable Exomic Incidental Findings in 6503 Participants: Challenges of Variant Classification. Genome Res. 25, 305–315. doi:10.1101/gr.183483.114
Bope, C. D., Chimusa, E. R., Nembaware, V., Mazandu, G. K., de Vries, J., and Wonkam, A. (2019). Dissecting In Silico Mutation Prediction of Variants in African Genomes: Challenges and Perspectives. Front. Genet. 10 (601), 601. doi:10.3389/fgene.2019.00601
Chimusa, E. R., Mbiyavanga, M., Mazandu, G. K., and Mulder, N. J. (2016). ancGWAS: a Post Genome-wide Association Study Method for Interaction, Pathway and Ancestry Analysis in Homogeneous and Admixed Populations. Bioinformatics 32 (4), 549–556. doi:10.1093/bioinformatics/btv619
Chimusa, E. R., Meintjies, A., Tchanga, M., Mulder, N., Seoighe, C., Soodyall, H., et al. (2015). A Genomic Portrait of Haplotype Diversity and Signatures of Selection in Indigenous Southern African Populations. PLoS Genet. 11, e1005052. doi:10.1371/journal.pgen.1005052
Choi, Y., and Chan, A. P. (2015). PROVEAN Web Server: a Tool to Predict the Functional Effect of Amino Acid Substitutions and Indels. Bioinformatics 31 (16), 2745–2747. doi:10.1093/bioinformatics/btv195
Choudhury, A., Aron, S., Botigué, L. R., Sengupta, D., Botha, G., Bensellak, T., et al. (2020). High-depth African Genomes Inform Human Migration and Health. Nature 586 (7831), 741–748. doi:10.1038/s41586-020-2859-7
Danecek, P., Auton, A., Abecasis, G., Albers, C. A., Banks, E., DePristo, M. A., et al. (2011). The Variant Call Format and VCFtools. Bioinformatics 27, 2156–2158. doi:10.1093/bioinformatics/btr330
Davydov, E. V., Goode, D. L., Sirota, M., Cooper, G. M., Sidow, A., and Batzoglou, S. (2010). Identifying a High Fraction of the Human Genome to Be under Selective Constraint Using GERP++. PLoS Comput. Biol. 6, e1001025. doi:10.1371/journal.pcbi.1001025
Doerks, T., Copley, R. R., Schultz, J., Ponting, C. P., and Bork, P. (2002). Systematic Identification of Novel Protein Domain Families Associated with Nuclear Functions. Genome Res. 12, 47–56. doi:10.1101/gr.203201
Dong, C., Wei, P., Jian, X., Gibbs, R., Boerwinkle, E., Wang, K., et al. (2015). Comparison and Integration of Deleteriousness Prediction Methods for Nonsynonymous SNVs in Whole Exome Sequencing Studies. Hum. Mol. Genet. 24, 2125–2137. doi:10.1093/hmg/ddu733
Dorschner, M. O., Amendola, L. M., Turner, E. H., Robertson, P. D., Shirts, B. H., and Gallego, C. J. Actionable, Pathogenic Incidental Findings in 1,000 Participants' Exomes. Am. J. Hum. Genet. 93, 631–640. doi:10.1016/j.ajhg.2013.08.006
Garber, M., Guttman, M., Clamp, M., Zody, M. C., Friedman, N., and Xie, X. (2009). Identifying Novel Constrained Elements by Exploiting Biased Substitution Patterns. Bioinformatics 25, i54–i62. doi:10.1093/bioinformatics/btp190
Gorlova, O. Y., Ying, J., Amos, C. I., Spitz, M. R., Peng, B., and Gorlov, I. P. (2012). Derived SNP Alleles Are Used More Frequently Than Ancestral Alleles as Risk-Associated Variants in Common Human Diseases. J. Bioinform Comput. Biol. 10 (2), 1241008. doi:10.1142/S0219720012410089
Gudykunst, W. B., and Schmidt, K. L. (1987). Language and Ethnic Identity: An Overview and Prologue. J. Lang. Soc. Psychol. 6 (3-4), 157–170. doi:10.1177/0261927x8763001
Gurdasani, D., Carstensen, T., Tekola-Ayele, F., Pagani, L., Tachmazidou, I., Hatzikotoulas, K., et al. (2015). The African Genome Variation Project Shapes Medical Genetics in Africa. Nature 517, 327–332. doi:10.1038/nature13997
Hunter, J. E., Irving, S. A., Biesecker, L. G., Buchanan, A., Jensen, B., Lee, K., et al. (2016). A Standardized, Evidence-Based Protocol to Assess Clinical Actionability of Genetic Disorders Associated with Genomic Variation. Genet. Med. 18, 1258–1268. doi:10.1038/gim.2016.40
Ionita-Laza, I. M. K., McCallum, K., Xu, B., and Buxbaum, J. D. (2016). A Spectral Approach Integrating Functional Genomic Annotations for Coding and Noncoding Variants. Nat. Genet. 48 (2), 214–220. doi:10.1038/ng.3477
Jagadeesh, K. A., Wenger, A. M., Berger, M. J., Guturu, H., Stenson, P. D., Cooper, D. N., et al. (2016). M-CAP Eliminates a Majority of Variants of Uncertain Significance in Clinical Exomes at High Sensitivity. Nat. Genet. 48 (12), 1581–1586. doi:10.1038/ng.3703
Kim, S. J., Jhong, J. H., Lee, J., and Koo, J. Y. (2017). Erratum to: Meta-Analytic Support Vector Machine for Integrating Multiple Omics Data. BioData Min. 10 (2), 8. doi:10.1186/s13040-017-0128-6
Landrum, M. J., Lee, J. M., Benson, M., Brown, G., Chao, C., Chitipiralla, S., et al. (2016). ClinVar: Public Archive of Interpretations of Clinically Relevant Variants. Nucleic Acids Res. 44, D862–D868. doi:10.1093/nar/gkv1222
Lebeko, K., Manyisa, N., Chimusa, E. R., Mulder, N., Dandara, C., and Wonkam, A. (2017). A Genomic and Protein-Protein Interaction Analyses of Nonsyndromic Hearing Impairment in Cameroon Using Targeted Genomic Enrichment and Massively Parallel Sequencing. OMICS A J. Integr. Biol. 21, 90–99. doi:10.1089/omi.2016.0171
Li, H., Handsaker, B., Wysoker, A., Fennell, T., Ruan, J., Homer, N., et al. (2009). The Sequence Alignment/Map Format and SAMtools. Bioinformatics 25, 2078–2079. doi:10.1093/bioinformatics/btp352
Liu, X., Jian, X., and Boerwinkle, E. (2011). dbNSFP: a Lightweight Database of Human Nonsynonymous SNPs and Their Functional Predictions. Hum. Mutat. 32, 894–899. doi:10.1002/humu.21517
Loh, P.-R., Danecek, P., Palamara, P. F., Fuchsberger, C., A Reshef, Y., K Finucane, H., et al. (2016). Reference-based Phasing Using the Haplotype Reference Consortium Panel. Nat. Genet. 48 (11), 1443–1448. doi:10.1038/ng.3679
Loh, P.-R., Palamara, P. F., and Price, A. L. (2016). Fast and Accurate Long-Range Phasing in a UK Biobank Cohort. Nat. Genet. 48, 811–816. doi:10.1038/ng.3571
Lu, Q. H. Y., Hu, Y., Sun, J., Cheng, Y., Cheung, K. H., and Zhao, H. (2015). A Statistical Framework to Predict Functional Non-coding Regions in the Human Genome through Integrated Analysis of Annotation Data. Sci. Rep. 5, 10576. doi:10.1038/srep10576
McLaren, W., Gil, L., Hunt, S. E., Riat, H. S., Ritchie, G. R. S., Thormann, A., et al. (2016). The Ensembl Variant Effect Predictor. Genome Biol. 17, 122. doi:10.1186/s13059-016-0974-4
Michalopoulos, S. (2012). The Origins of Ethnolinguistic Diversity. Am. Econ. Rev. 102 (4), 1508–1539. doi:10.1257/aer.102.4.1508
Ng, P. C., Henikoff, S., Chun, S., and Fay, J. C. (2006). Predicting the Effects of Amino Acid Substitutions on Protein functionIdentification of Deleterious Mutations within Three Human Genomes. Annu. Rev. Genom. Hum. Genet.Genome Res. 719, 611553–801561. doi:10.1146/annurev.genom.7.080505.115630
Paten, B., Herrero, J., Fitzgerald, S., Beal, K., Flicek, P., Holmes, I., et al. (2008). Genome-wide Nucleotide-Level Mammalian Ancestor Reconstruction. Genome Res. 18, 1829–1843. doi:10.1101/gr.076521.108
Patterson, N. P. A. L., and Price, D. (2006). Population Structure and Eigenanalysis. PLoS Genet. 2 (12), e190. doi:10.1371/journal.pgen.0020190
Purcell, S., Neale, B., Todd-Brown, K., Thomas, L., Ferreira, M. A. R., Bender, D., et al. (2007). PLINK: a Tool Set for Whole-Genome Association and Population-Based Linkage Analyses. Am. J. Hum. Genet. 81, 559–575. doi:10.1086/519795
Quang, D., Chen, Y., Dann, X., and Xie, X. (2014). DANN: a Deep Learning Approach for Annotating the Pathogenicity of Genetic Variants. Bioinformatics 31 (5), 761–763. doi:10.1093/bioinformatics/btu703
Rentzsch, P., Witten, D., Cooper, G. M., Shendure, J., and Kircher, M. (2018). CADD: Predicting the Deleteriousness of Variants throughout the Human Genome. Nucleic Acids Res. 47, D886. doi:10.1093/nar/gky1016
Reva, B., Antipin, Y., and Sander, C. (2011). Predicting the Functional Impact of Protein Mutations: Application to Cancer Genomics. Nucleic Acids Res. 39, e118. doi:10.1093/nar/gkr407
Schwarz, J. M., Rödelsperger, C., Schuelke, M., and Seelow, D. (2010). MutationTaster Evaluates Disease-Causing Potential of Sequence Alterations. Nat. Methods 7, 575–576. doi:10.1038/nmeth0810-575
Sherry, S. T., Ward, M. H., Kholodov, M., Baker, J., Phan, L., Smigielski, E. M., et al. (2001). dbSNP: the NCBI Database of Genetic Variation. Nucleic Acids Res. 29 (1), 308–311. doi:10.1093/nar/29.1.308
Shihab, H. A., Gough, J., Cooper, D. N., Day, I. N. M., and Gaunt, T. R. (2013). Predicting the Functional Consequences of Cancer-Associated Amino Acid Substitutions. Bioinformatics 29, 1504–1510. doi:10.1093/bioinformatics/btt182
Keywords: actionable gene, incidental finding, whole-genome sequencing, next-generation sequencing, genetic diversity, population genetics actionable gene, population genetics
Citation: Chimusa ER, Alosaimi S and Bope CD (2022) Dissecting Generalizability and Actionability of Disease-Associated Genes From 20 Worldwide Ethnolinguistic Cultural Groups. Front. Genet. 13:835713. doi: 10.3389/fgene.2022.835713
Received: 12 January 2022; Accepted: 29 April 2022;
Published: 24 June 2022.
Edited by:
Guiyou Liu, Chinese Academy of Sciences (CAS), ChinaReviewed by:
Segun Fatumo, University of London, United KingdomJuan Carlos Fernandez-Lopez, Instituto Nacional de Medicina Genómica (INMEGEN), Mexico
Copyright © 2022 Chimusa, Alosaimi and Bope. This is an open-access article distributed under the terms of the Creative Commons Attribution License (CC BY). The use, distribution or reproduction in other forums is permitted, provided the original author(s) and the copyright owner(s) are credited and that the original publication in this journal is cited, in accordance with accepted academic practice. No use, distribution or reproduction is permitted which does not comply with these terms.
*Correspondence: Emile R. Chimusa, emile.chimusa@.uct.ac.za