- 1Yunnan Academy of Grassland and Animal Science, Kunming, China
- 2Key Laboratory of Animal Genetics, Breeding and Reproduction of Shaanxi Province, College of Animal Science and Technology, Northwest A&F University, Yangling, China
- 3Academy of Science and Technology, Chuxiong Normal University, Chuxiong, China
- 4National Institute for Biotechnology and Genetic Engineering, Faisalabad, Pakistan
- 5Institute of Animal Science, Tibet Academy of Agricultural and Animal Husbandry Science, Lhasa, China
Dengchuan cattle are the only dairy yellow cattle and endangered cattle among Yunnan native cattle breeds. However, its genetic background remains unclear. Here, we performed whole-genome sequencing of ten Dengchuan cattle. Integrating our data with the publicly available data, Dengchuan cattle were observed to be highly interbred than other cattle in the dataset. Furthermore, the positive selective signals were mainly manifested in candidate genes and pathways related to milk production, disease resistance, growth and development, and heat tolerance. Notably, five genes (KRT39, PGR, KRT40, ESR2, and PRKACB) were significantly enriched in the estrogen signaling pathway. Moreover, the missense mutation in the PGR gene (c.190T > C, p.Ser64Pro) showed a homozygous mutation pattern with higher frequency (83.3%) in Dengchuan cattle. In addition, a large number of strong candidate regions matched genes and QTLs related to milk yield and composition. Our research provides a theoretical basis for analyzing the genetic mechanism underlying Dengchuan cattle with excellent lactation and adaptability, crude feed tolerance, good immune performance, and small body size and also laid a foundation for genetic breeding research of Dengchuan cattle in the future.
Introduction
Chinese domestic cattle breeds have a broad genetic base and abundant genetic variation, generally consisting of Bos taurus and Bos indicus lineages. Bos taurus mainly originated from cattle in Europe and is distributed in northern China, whereas Bos indicus originated from cattle in South Asia and is mainly distributed in southern China (Zhang, 2011). Because of the intensive selection, Bos taurus have advantages on beef and milk production; however, it is not adapted to tropical environments and thus cannot make use of its full potential for production in hot and humid areas in southern China (Crouse et al., 1989; Buchanan, 2002; Pegorer et al., 2007; Satrapa et al., 2011). Compared to Bos taurus, Bos indicus is able to tolerate heat and crude feed. One of the typical and distinctive physical features includes a hump on its back (Utsunomiya et al., 2019; Zhang et al., 2021). Scientific research indicates hybridization of these sub-species can combine the strengths of Bos taurus and Bos indicus, being one of the oldest and truest ways to balance productivity with the environmental adaptability (Negussie et al., 1999; Schatz et al., 2020).
Yunnan has been one of the core regions for the migration of Indian indicine into the Chinese territory. Amongst various hybrid cattle, Dengchuan cattle are the only local dairy yellow cattle breed in China. Dengchuan shares a long history of selective breeding, dating back to the Han Dynasty (206 BC-220). Local people paid attention to selecting cattle with longer lactation periods with high milk yield, to breed their offspring, and using them to make milk fats, which is the local specialty dairy product (Zhang, 2011). With the improvement and promotion of artificial insemination and frozen semen technology of fresh semen at room temperature, native people massively introduced Holstein cattle for hybrid improvement. According to statistics data, from 1981 to 1989, the 305-day milk yield of Dengchuan cattle increased from 838.3 kg to 1,066.6 kg, with a milk fat rate of 6.89% and a dry milk matter of 13.52%, whereas the 305-day milk yield of hybrid F3 (Dengchuan × Holstein) increased from 2,111.1 kg to 3,094.4 kg, with a milk fat percentage of 4.09% and a dry milk matter of 12.0% (Zhang and Ma, 1987; Ma, 1988). However, blind hybridization and the lack of breed conservation planning caused the threat of breed degradation in Dengchuan cattle. According to a recent survey, only 212 Dengchuan cattle (206 cows and six bulls) remained, among which the original breed of Dengchuan cattle is extremely rare and endangered (Yang et al., 2021).
With the development of the next-generation sequencing technology and the enrichment of re-sequencing databases, genome-wide genetic analysis plays an increasingly significant role in the investigation and selection of germplasm resources of landraces (Shen et al., 2020; Jiang et al., 2021; Xia et al., 2021; Zhang et al., 2021). A recent study on Dengchuan cattle showed that Dengchuan cattle are a taurine–indicine mixed breed (Foissac et al., 2019). However, there are no previous studies using whole-genome sequencing data to identify genes related to milk production and disease resistance in Dengchuan cattle.
In this study, we performed whole-genome sequencing of ten individuals of Dengchuan cattle to explore the genetic diversity and population genetic structure of the autosomal genome. In order to further explore the genetic potential of Dengchuan cattle, single nucleotide polymorphisms (SNPs) of Dengchuan cattle were compared with those of commercial and native breeds previously collected from around the world.
Materials and Methods
Sample Collection and Sequencing
Ten samples of Dengchuan cattle were collected from the ear tissue samples in the Dengchuan area of Yunnan province, China. To explore the ancestry proportions of Dengchuan cattle and compare the genetic diversity with worldwide cattle breeds, additional 68 samples were collected from the Sequence Read Archive (SRA, https://www.ncbi.nlm.nih.gov/sra/) (Leinonen et al., 2011), including European cattle breeds [Angus (n = 9), Simmental (n = 8), and Holstein (n = 8)]; northeast Asia breed (Hanwoo, n = 10); southwest Chinese breeds [Dengchuan (n = 2), Dianzhong (n = 6), and Wenshan (n = 6)]; southeast Chinese breeds [Guangfeng (n = 4) and Wannan (n = 5)]; and India–Pakistan zebu cattle (Bos indicus) breeds (n = 10) (Supplementary Table S1). A total of 78 samples were used in this study.
Sequencing, Alignment, and Variant Identification
Genomic DNA was extracted using the standard phenol–chloroform method (Sterky et al., 2017). Paired-end libraries with the average insert size of 500 bp were constructed for each individual, with an average read length of 150 bp and an average sequence coverage of ∼10.7×. Sequencing was performed using Illumina NovaSeq instruments at Novogene Bioinformatics Institute, Beijing, China. Raw reads data of fastq format were quality trimmed using trimmomatic (SLIDINGWINDOW:3:15 MINLEN:35 TRAILING:20 LEADING:20 AVGQUAL:20 TOPHRED33) (Bolger et al., 2014) to remove adapters and low-quality bases. The Burrows–Wheeler Aligner BWA-MEM (v0.7.15-r1140) with default parameters (Li and Durbin, 2009) was used to align the clean reads to the Bos taurus reference assembly ARS-UCD1.2. The Picard tools (http://broadinstitute.github.io/picard) were used to filter potential duplicate reads. We used “Haplotype Caller,” “Genotype GVCFs,” and “Select Variants” modules of the Genome Analysis Toolkit (GATK, version 3.8-1-0-gf15c1c3ef) (McKenna et al., 2010) to call the SNP. The filtration of raw SNPs was conducted by using “variant Filtration” modules with the parameters “QD < 2.0, FS > 60.0, MQ < 40.0, MQRankSum < −12.5, ReadPosRankSum < −8.0, and SOR > 3.0” and the mean sequencing depth of variants (all individuals) “<1/3× and >3×”. Based on the Bos taurus reference assembly ARS-UCD1.2, SNPs were functionally annotated by ANNOVAR (Wang et al., 2010).
Population Genomic Analysis
SNPs of 78 samples were pruned in high levels of pairwise LD by PLINK v1.90b3.40 software (Purcell et al., 2007), excluding SNPs in strong LD (r2 > 0.2) within a sliding window of 50 SNPs advanced by five SNPs at the time. Principal component analysis (PCA) was carried out using the smartpca program of the EIGENSOFT v5.0 package (Patterson et al., 2006). Population structure analysis was carried out by ADMIXTURE v1.3.0 (Alexander and Lange, 2011). Based on the pairwise distance matrix, the NJ tree was constructed by MEGA v10.2.6 (Saitou and Nei, 1987; Kumar et al., 2018).
Runs of homozygosity (ROHs) were calculated by PLINK software (Purcell et al., 2007). SNPs with minor allele frequencies (MAF) < 0.05 were excluded due to instability. PLINK uses a sliding window of a minimum of 50 SNPs across the genome to identify ROHs, allowing for two missing SNPs and one heterozygous site per window. The minimum number of continuous homozygous SNPs constituting an ROH was set to 100. The minimum SNP density coverage was set to at least 50 SNPs per Kb, allowing for centromeric and SNP-poor regions to be algorithmically excluded from the analysis. The maximum gap between two consecutive homozygous SNPs was set at 100 Kb. The number and length of ROHs for each breed were estimated, and the length of ROH was divided into three categories: 0.5–1 Mb, 1–2 Mb, and 2–4 Mb, reflecting ancient, historical, and recent inbreeding, respectively (Kirin et al., 2010; Bhati et al., 2020).
Nucleotide diversity of each breed was investigated by VCFtools (Danecek et al., 2011) with the size of 50-kb non-overlapping window. The output of the --het function by VCFtools is a summary for each individual of the observed number of homozygous sites (O(hom)) and the expected number of homozygous sites (E(hom)). It also includes the total number of sites that the individual has data for and the inbreeding coefficient F, which is the canonical estimate of genomic F based on excess SNP homozygosity (Keller et al., 2011).
Linkage disequilibrium (LD) decay with the physical distance between SNPs was calculated and visualized by PopLDdecay software (Zhang et al., 2019) with default parameters.
Selective Sweep Identification
Only SNPs with less than 10% missing were used for selective sweep scanning. The nucleotide diversity (θπ) and the composite likelihood ratio (CLR) test (Nielsen et al., 2005) were used to detect the selection signatures in Dengchuan cattle and Holstein cattle. The θπ was estimated based on a sliding window of size 50 kb and a step of size 20 kb by VCFtools (Danecek et al., 2011). The CLR was calculated for sites in non-overlapping 50-kb windows by SweepFinder2 (DeGiorgio et al., 2016), reflecting the likelihood of observing SNP data under the assumption of a sweep.
We also performed the genetic differentiation (FST) and cross-population composite likelihood ratio test (XP-CLR) (Chen et al., 2010) to identify the difference in potential areas between different cattle breeds. FST analysis was estimated based on a sliding window of 50 kb and a step of size 20 kb by VCFtools (Danecek et al., 2011). XP-CLR is a likelihood method for detecting selective sweeps by jointly modeling the multilocus allele frequency differentiation between the two groups (Chen et al., 2010). The overlap of the top 1% window in each method was considered as candidate signatures of selection, and genes in those window regions were defined as potential candidate genes.
Enrichment Analyses of Candidate Genes Under Selection
Due to the complexity of biological data-mining situations, enrichment analysis was conducted to identify the possibility of biological processes associated with Dengchuan cattle (Huang et al., 2009). Online Kyoto Encyclopedia of Genes and Genomes (KEGG) pathway and Gene Ontology (GO) analyses were conducted by KOBAS 3.0 (Bu et al., 2021). Genes at p < 0.05 were considered to be significantly enriched in Kyoto Encyclopedia of Genes and Genomes (KEGG) pathways and GO (Gene Ontology) annotations.
Aligning Candidate Regions to the Quantitative Trait Loci Database
Biological processes of Dengchuan cattle could be analyzed through genes annotated from candidate regions; however, there were various candidate regions in the non-annotated genic regions, although they showed strong selective signals. We used QTLs to identify possible traits in these candidate regions. The cattle QTL database (http://www.animalgenome.org/cgi-bin/QTLdb/BT/index) contains 163,725 QTLs. The chromosome information is annotated to the cattle QTLdb to identify the regions of interest detected by selective sweep methods contained or overlapped across the QTLs. The function and information of candidate regions were determined after annotation.
Results
Analysis of the Population Structure and Genetic Diversity
Seventy-eight cattle, representing five geographically diverse cattle populations, namely, East Asian taurine, European taurine, Chinese indicine, Indian indicine (Xu et al., 2018), and local hybrid populations in Yunnan were selected for genome re-sequencing analysis (Figure 1A, Supplementary Table S1). After quality control, 147,397,064 bi-allelic autosomal SNPs (Supplementary Table S2) were used to construct genetic relationships using a neighbor-joining maximum likelihood method and PCA. Both methods revealed that these populations of regions, except Yunnan, clustered into three major genetic groups: Bos taurus, Indian indicine, and China indicine (Figure 1B, C). It was clear that Dengchuan cattle and Dianzhong cattle showed a certain degree of hybridization. Admixture analysis showed that the cattle breeds separate into Bos taurus and Bos indicus ancestries (Figure 1D, K = 2). When the number of clusters (K) was set to 4, East Asian taurine and European taurine were clearly separated. Dengchuan cattle depicted clear evidence of genetic heterogeneity with its shared genome ancestry with East Asian taurine (Hanwoo), European taurine (Angus, Simmental, and Holstein), Chinese indicine (Wannan and Guangfeng), and Indian indicine. It is rather remarkable that only half the Dengchuan cattle had a European taurine ancestry (Figure 1D, K = 4).
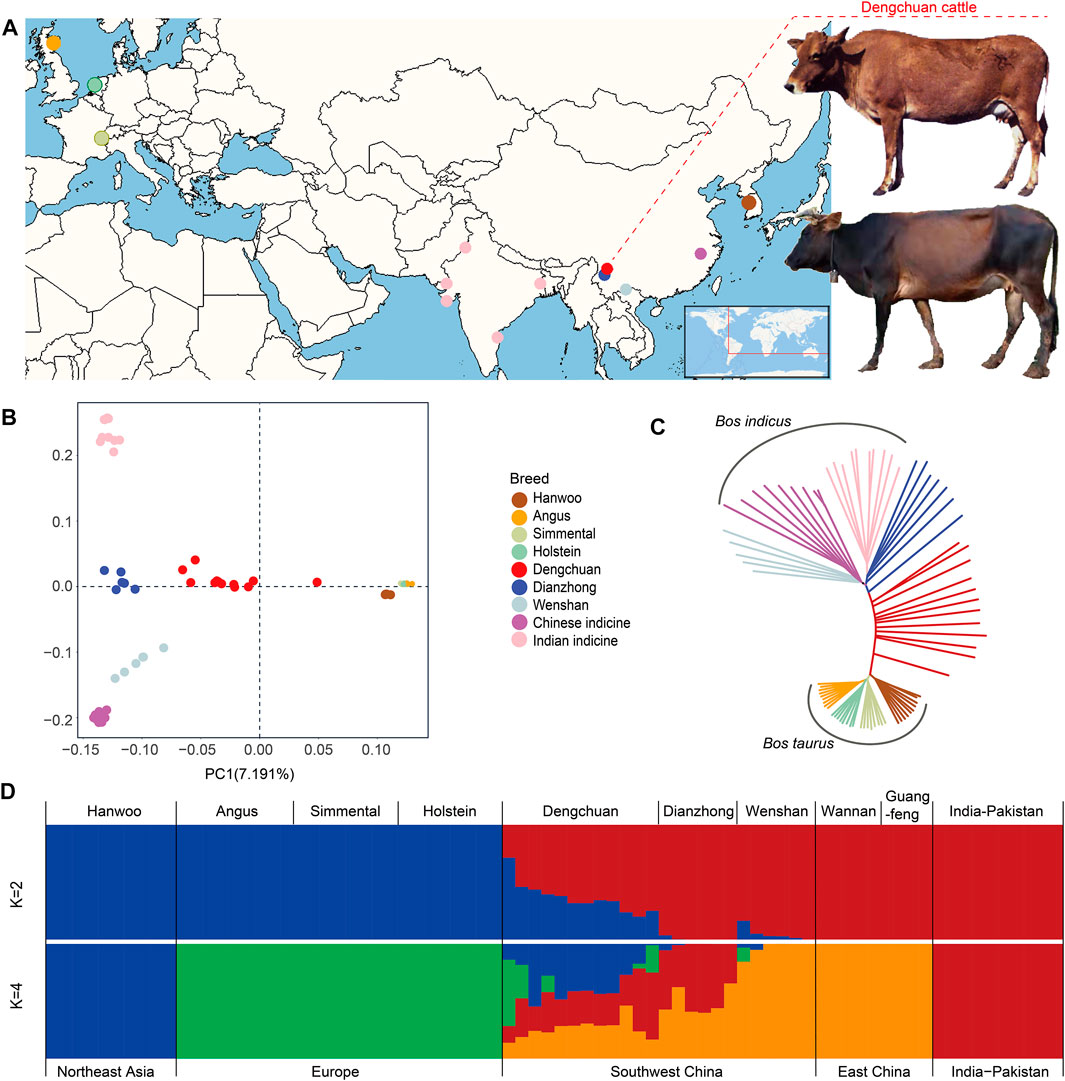
FIGURE 1. Population genetic analysis of Dengchuan cattle. (A) Geographic map indicating the origins of Dengchuan cattle and other cattle analyzed in this study. (B) Principal component analysis of cattle with PC1 against PC2. (C) Neighbor-joining tree of the 78 domesticated cattle. (D) Admixture plot (K = 2, 4) for the 78 cattle individuals. Each individual is shown as a vertical bar divided into K colors.
Patterns of Genomic Variation
ROH analysis revealed that the vast majority of ROHs identified in all breeds were between 0.5–1 Mb in length, but European commercial breeds (Angus, Holstein, and Simmental) had medium (1–2 Mb) and long ROHs (2–4 Mb). Besides, the total lengths of ROHs in Dengchuan cattle were much longer than those of the other two cattle in Yunnan (Figure 2A). This could indicate that European commercial breeds and Dengchuan cattle had undergone artificial selection for a long time. Similarly, the inbreeding coefficient based on genome heterozygosity was the highest in Angus (0.67) and lowest in Chinese indicine (−0.22) (Figure 2B). The average nucleotide diversity among Dengchuan cattle and other cattle groups revealed that Chinese indicine was the highest (3.10e−3), followed by Wenshan cattle (2.81e−3), Dianzhong cattle (2.72e−3), and Dengchuan cattle (2.58e−3). In comparison to Bos indicus, it was concluded that Bos taurus possessed a low level and high density of nucleotide diversity (Figure 2C). In contrast, the lowest average genome-wide LD was observed in Dengchuan cattle and Indian indicine. Besides, the LD decay in Bos indicus was faster than Bos taurus when the physical distance of SNP was less than 10 KB (Figure 2D).
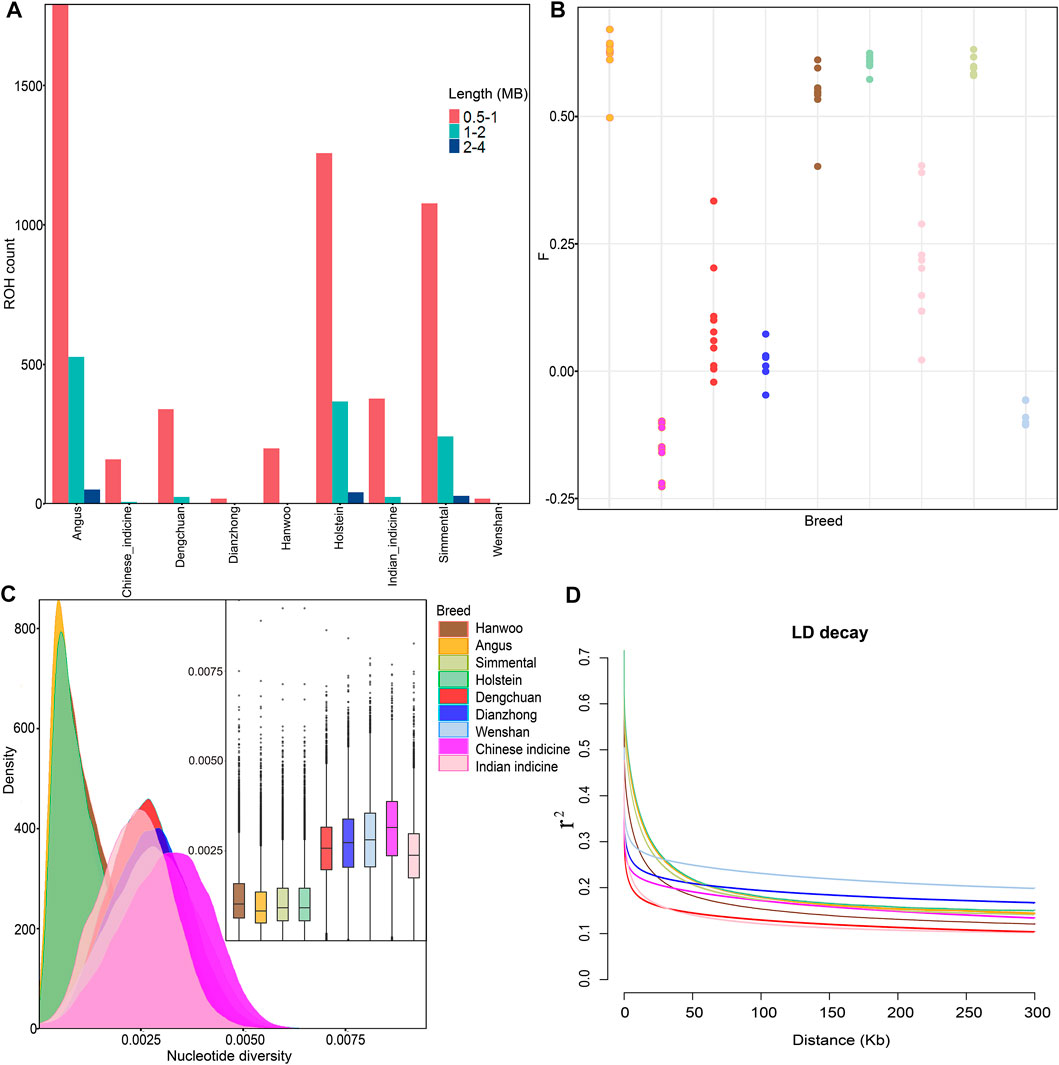
FIGURE 2. Genetic diversity among 78 samples from nine populations. (A) Estimation of the total number of ROH for each group. The three categories of ROH length: 0.5–1 Mb, 1–2 Mb, and 2–4 Mb, reflecting ancient, historical, and recent inbreeding, respectively. (B) Inbreeding coefficient for each individual. (C) Density plots and Box plots of the nucleotide diversity for each group. (D) Genome-wide average LD decay estimated from each group.
Positive Selective Signature
A total of 217 candidate genes were detected by both θπ and the CLR test in Dengchuan cattle (Figure 3A, Supplementary Tables S3, S4). Some positively selected genes were reported to be associated with lactation function and disease resistance, such as the butterfat rate [PPARGC1A (Weikard et al., 2005; Schennink et al., 2009)], milk production [B4GALT1 (Asadollahpour Nanaei et al., 2020; Valsalan et al., 2021)], immunity [IL2 (McCoard et al., 2019) and NFATC3 (Hu et al., 2018)], and mastitis resistance [ITSN2 (Miles and Huson, 2020)]. In particular, ITSN2 was located at the strongest selection signal on BTA11 (11:74700001-74900000). The result of strong positive selection was further verified by Tajima’s D and nucleotide diversity analysis (Figure 3C). Moreover, 217 candidate genes were compared with 224 candidate genes detected by both θπ and the CLR test in Holstein cattle (Supplementary Tables S5, S6), whereas seven genes (RERE, SLC45A1, RAB11FIP2, PTDSS1, MTERF3, KDM4C, and COL27A1) were shared in both Dengchuan cattle and Holstein cattle.
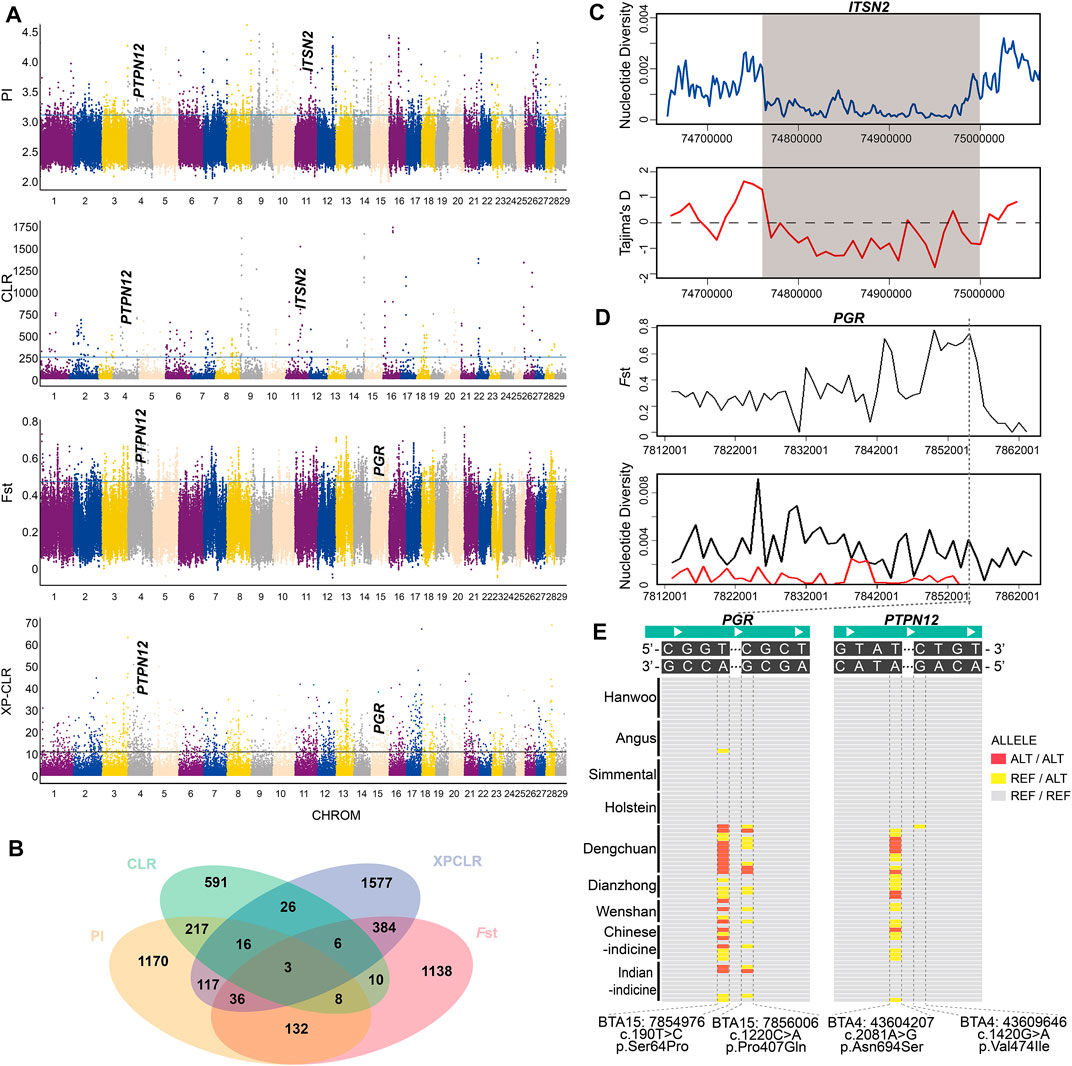
FIGURE 3. Analysis of the signatures of positive selection in the genome of Dengchuan cattle. (A) Manhattan plot of selective sweeps in Dengchuan cattle. (B) Venn diagram showing the gene overlaps among θπ, CLR, Fst, and XP-CLR. (C) Nucleotide diversity and Tajima’s D plots at the ITSN2 gene region. (D) Fst and Tajima’s D plots at the PGR gene region. (E) Regional highlight of the missense mutation of genes enriched in the estrogen signaling pathway. PGR gene showed a high-frequency homozygous mutation site (c.190T > C) in Dengchuan cattle.
Biological Process and Pathway Between Dengchuan Cattle and Angus Cattle
FST and XP-CLR tests were performed to detect the positive selection signatures between Dengchuan and Angus cattle (Figure 3A, Supplementary Tables S7, S8). A total of 384 genes were overlapped by both methods, which were enriched using GO annotation and KEGG pathway terms to further analyze their biological functions. The results represented significant enrichment of 220 GO terms and 29 KEGG pathways (p < 0.05; Supplementary Tables S9, S10). Gene list analysis revealed the involvement of various genes in protein synthesis (GO:0042802), endoplasmic reticulum (GO:0005783), temperature homeostasis (GO:0001659), and neutral amino acid transport (GO:0015804). Similarly, the enriched KEGG pathways included the estrogen signaling pathway, protein processing in the endoplasmic reticulum, biosynthesis of amino acids, glycan biosynthesis, and metabolic pathways. Moreover, PGR, a gene enriched in the estrogen signaling pathway, showed strong positive selection in Dengchuan cattle (Figure 3D).
It is worth noting that three overlapped genes (PTPN12, KIAA1109, and ADAD1) were detected among the four mentioned selection methods (Figure 3B), indicating that these genes were strongly selected in Dengchuan cattle. We checked mutations of eight genes (five estrogen signaling pathway enrichment genes (KRT39, PGR, KRT40, ESR2, and PRKACB) and three overlapped genes (PTPN12, KIAA1109, and ADAD1)) in Dengchuan cattle, two missense mutations in PGR (c.190T > C, p.Ser64Pro; c.1220C > A, p.Pro407Gln), and two missense mutations in PTPN12 (c.2081A > G, p.Asn694Ser; c.1420G > A, p.Val474Ile), which showed distinct allelic patterns in Dengchuan cattle (Figure 3E).
QTLs Based on Identified Regions
QTLs and selection signatures at the same location indicated that phenotypes and traits were influenced by the joint action of large numbers of polygenes and environmental effects (Georges et al., 1995). Thus, the top 50 candidate regions were studied for each method of scanning in Dengchuan cattle. These candidate regions also included regions that annotated gene failures and were used to extract relevant QTLs from the cattle QTLdb. Since the candidate region might overlap with several QTLs associated with different traits, one result with the most consistent chromosome fragment for each candidate region was picked. As shown in the Supplementary Table S11, 94 genomic regions for 100 candidate regions (θπ and CLR) overlapped with QTLs: 49 candidate regions overlapped milk, 21 candidate regions overlapped reproduction and production, 18 candidate regions overlapped health, and six candidate regions overlapped meat and carcass. Simultaneously, 99 genomic regions for 100 candidate regions (FST and XP-CLR) overlapped with QTLs: 50 candidate regions overlapped milk, 38 candidate regions overlapped reproduction and production, six candidate regions overlapped meat and carcass, four candidate regions overlapped health, and one candidate region overlapped exterior conformation (Supplementary Table S12).
Discussion
Genomic information is the instruction of life construction. Here, we have conducted the whole-genome sequence-based study for the genomic diversity and selective signatures in Dengchuan cattle. The ancestral contributions of Dengchuan cattle came from East Asian taurine (∼34%), Chinese indicine (∼22%), European taurine (10%), and Indian indicine (∼34%). It is worth noting that Dengchuan cattle have not been able to cluster completely, showing some differences amongst its individuals (Figure 1B). Similar outliers can be seen for the inbreeding coefficient (F) based on ROH, which may be from hybrid lineages or the introduction of crossbreeding. In addition, the ROH distribution and nucleotide diversity of Dengchuan cattle were basically consistent with other native Yunnan breeds (Foissac et al., 2019; Zhang et al., 2021). The LD decay pattern of Dengchuan cattle was similar to that of Indian indicine, confirming the high genetic diversity of Dengchuan cattle.
Dairy cows in hot and humid areas are naturally more prone to environmental mastitis due to bacterial growth (Alain et al., 2009). ITSN2 is a member of a family of proteins involved in clathrin-mediated endocytosis that encodes a cytoplasmic protein which contains SH3 domains. ITSN2 is thought to regulate the formation of clathrin-coated vesicles and may also function in the induction of T-cell antigen receptor (TCR) endocytosis (National Center for Biotechnology Information, 2017). Furthermore, PTPN12 is a protein tyrosine phosphatase that contributes to the stable 3D acinar formation of mammary epithelial cells (Sun et al., 2011). PGR promotes alveologenesis in the pregnant mammary gland for milk production (Aikawa et al., 2020). In addition, among the seven genes overlapped between Dengchuan cattle and Holstein cattle, we examined the scanning signal of PTDSS1 and found that the CLR value in Dengchuan cattle (∼638) was much higher than that in Holstein cattle (∼431). PTDSS1 is used for the catalytic synthesis of lecithin. The remaining six genes were also reported to be highly associated with milk production: RERE and SLC45A1 are reported to be associated with milk production (Buaban et al., 2022); MTERF3 is associated with milk fatty acid composition (Palombo et al., 2018); KDM4C is associated with breast cancer (Garcia and Lizcano, 2016); RAB11FIP2 is associated with transcytosis (Ducharme et al., 2007); and COL27A1 is associated with the sternum (Maddirevula et al., 2019). The strong selection of these genes may be the reason why Dengchuan cattle are a good dairy breed in hot and humid climates.
The comparative analysis of genetic differentiation between Dengchuan cattle and other breeds revealed the estimated value of Dengchuan cattle and Angus cattle to be the highest (∼0.22), which was suitable for subsequent analysis (Supplementary Table S13). Interestingly, the KEGG pathway with significant enrichment of differential signals included the estrogen signaling pathway. Activation of the estrogen signaling pathway results in prolonged lactation and high milk yield (Lawrence, 2022). Additionally, in the current study, the missense mutations in PGR (c.190T > C, p.Ser64Pro) (Figure 3E) likely play an important role in elevated milk production in Dengchuan cattle.
Milk yield and composition are typical polygenic traits (Georges et al., 1995). For a more comprehensive explanation of the function in strong candidate regions, the most promising QTLs were matched for two-hundred candidate regions. Because of the window size of scanning methods and the different fragment sizes of different genes and QTLs, some regions were separated and calculated several times and were matched to the same QTL. This results in a large number of milk-related QTLs matching our candidate regions. Overall, about half of the candidate regions matched QTLs associated with milk yield and its composition. Furthermore, the candidate regions corresponded with the health QTLs, which were mainly related to the somatic cell score and heat tolerance. Most of the QTLs related to reproduction were associated with the body size in our result. For example, some growth-related QTLs have been annotated on chromosome 14 (Supplementary Table S11). Similarly, the PLAG1 gene on chromosome 14 has been shown to be associated with the body size (Karim et al., 2011). Fst results showed that there exists significant genetic differentiation of the PLAG1 gene between Dengchuan cattle and Angus cattle (Supplementary Table S7). It is worth noting that Dengchuan cattle are small in body stature, approximately 105 cm tall and weighing 225 kg in adulthood (Zhang, 2011).
In conclusion, this study provides a theoretical basis for analyzing the genetic mechanism of Dengchuan cattle with excellent lactation and adaptability, crude feed tolerance, good immune performance, and small body size, which also lays a foundation for genetic breeding research of Dengchuan cattle in the future.
Data Availability Statement
The datasets presented in this study can be found in online repositories. The names of the repository/repositories and accession number(s) can be found in the article/Supplementary Material.
Ethics Statement
The study was approved by the Institutional Animal Care and Use Committee of Northwest A&F University following the recommendation of the Regulations for the Administration of Affairs Concerning Experimental Animals of China. Specific consent procedures were not required for this study following the recommendations of the Regulations for the Administration of Affairs Concerning Experimental Animals of China.
Author Contributions
NC and CL conceived and designed the experiments. LJ and KQ performed the experiments. JZ, JL, and QS contributed analysis tools. LJ wrote the manuscript. QH and CL revised the manuscript and provided suggestions. KQ, CL, and BH contributed to the funding for the research. All authors contributed to the article and approved the submitted version.
Funding
This work was funded by the China Agriculture Research System of MOF and MARA (CARS-37), the Program of Yunling Scholar, the Young and Middle-aged Academic Technology Leader Backup Talent Cultivation Program in Yunnan Province, China (No. 2018HB045), and Yunnan Provincial Major S&T Project (No. 2019ZG007), and the Doctoral Startup Project of Chuxiong Normal University (No. BSQD2101).
Conflict of Interest
The authors declare that the research was conducted in the absence of any commercial or financial relationships that could be construed as a potential conflict of interest.
Publisher’s Note
All claims expressed in this article are solely those of the authors and do not necessarily represent those of their affiliated organizations, or those of the publisher, the editors, and the reviewers. Any product that may be evaluated in this article, or claim that may be made by its manufacturer, is not guaranteed or endorsed by the publisher.
Acknowledgments
Thanks to high-performance computing (HPC) of Northwest A&F University (NWAFU) for providing computing resources.
Supplementary Material
The Supplementary Material for this article can be found online at: https://www.frontiersin.org/articles/10.3389/fgene.2022.833475/full#supplementary-material
References
Aikawa, S., Yuan, J., Dewar, A., Sun, X., and Dey, S. K. (2020). Scribble Promotes Alveologenesis in the Pregnant Mammary Gland for Milk Production. Reproduction 159, 719–731. doi:10.1530/rep-20-0108
Alain, K., Karrow, N. A., Thibault, C., St-Pierre, J., Lessard, M., and Bissonnette, N. (2009). Osteopontin: an Early Innate Immune Marker of Escherichia coli Mastitis Harbors Genetic Polymorphisms with Possible Links with Resistance to Mastitis. BMC Genomics 10, 444. doi:10.1186/1471-2164-10-444
Alexander, D. H., and Lange, K. (2011). Enhancements to the ADMIXTURE Algorithm for Individual Ancestry Estimation. BMC Bioinformatics 12, 246. doi:10.1186/1471-2105-12-246
Asadollahpour Nanaei, H., Dehghani Qanatqestani, M., and Esmailizadeh, A. (2020). Whole-genome Resequencing Reveals Selection Signatures Associated with Milk Production Traits in African Kenana Dairy Zebu Cattle. Genomics 112, 880–885. doi:10.1016/j.ygeno.2019.06.002
Bhati, M., Kadri, N. K., Crysnanto, D., and Pausch, H. (2020). Assessing Genomic Diversity and Signatures of Selection in Original Braunvieh Cattle Using Whole-Genome Sequencing Data. BMC Genomics 21, 27. doi:10.1186/s12864-020-6446-y
Bolger, A. M., Lohse, M., and Usadel, B. (2014). Trimmomatic: a Flexible Trimmer for Illumina Sequence Data. Bioinformatics 30, 2114–2120. doi:10.1093/bioinformatics/btu170
Bu, D., Luo, H., Huo, P., Wang, Z., Zhang, S., He, Z., et al. (2021). KOBAS-i: Intelligent Prioritization and Exploratory Visualization of Biological Functions for Gene Enrichment Analysis. Nucleic Acids Res. 49, W317–w325. doi:10.1093/nar/gkab447
Buaban, S., Lengnudum, K., Boonkum, W., and Phakdeedindan, P. (2022). Genome-wide Association Study on Milk Production and Somatic Cell Score for Thai Dairy Cattle Using Weighted Single-step Approach with Random Regression Test-Day Model. J. Dairy Sci. 105, 468–494. doi:10.3168/jds.2020-19826
Buchanan, D. S. (2002). “Animals that Produce Dairy Foods | Major Bos Taurus Breeds,” in Encyclopedia of Dairy Sciences. Editor J. W. Fuquay. Second Edition (San Diego: Academic Press), 284–292. doi:10.1016/b978-0-12-374407-4.00032-7
Chen, H., Patterson, N., and Reich, D. (2010). Population Differentiation as a Test for Selective Sweeps. Genome Res. 20, 393–402. doi:10.1101/gr.100545.109
Crouse, J. D., Cundiff, L. V., Koch, R. M., Koohmaraie, M., and Seideman, S. C. (1989). Comparisons of and Inheritance for Carcass Beef Characteristics and Meat Palatability. J. Anim. 67, 2661. doi:10.2527/jas1989.67102661x
Danecek, P., Auton, A., Abecasis, G., Albers, C. A., Banks, E., Depristo, M. A., et al. (2011). The Variant Call Format and VCFtools. Bioinformatics 27, 2156–2158. doi:10.1093/bioinformatics/btr330
DeGiorgio, M., Huber, C. D., Hubisz, M. J., Hellmann, I., and Nielsen, R. (2016). SweepFinder2: Increased Sensitivity, Robustness and Flexibility. Bioinformatics 32, 1895–1897. doi:10.1093/bioinformatics/btw051
Ducharme, N. A., Williams, J. A., Oztan, A., Apodaca, G., Lapierre, L. A., and Goldenring, J. R. (2007). Rab11-FIP2 Regulates Differentiable Steps in Transcytosis. Am. J. Physiol. Cel Physiol. 293 (3), C1059–C1072. doi:10.1152/ajpcell.00078.2007
Foissac, S., Djebali, S., Munyard, K., Vialaneix, N., Rau, A., Muret, K., et al. (2019). Multi-species Annotation of Transcriptome and Chromatin Structure in Domesticated Animals. BMC Biol. 17, 108. doi:10.1186/s12915-019-0726-5
Garcia, J., and Lizcano, F. (2016). KDM4C Activity Modulates Cell Proliferation and Chromosome Segregation in Triple-Negative Breast Cancer. Breast Cancer (Auckl) 10, 169–175. doi:10.4137/BCBCR.S40182
Georges, M., Nielsen, D., Mackinnon, M., Mishra, A., Okimoto, R., Pasquino, A. T., et al. (1995). Mapping Quantitative Trait Loci Controlling Milk Production in Dairy Cattle by Exploiting Progeny Testing. Genetics 139, 907–920. doi:10.1093/genetics/139.2.907
Hu, L., He, F., Huang, M., Peng, M., Zhou, Z., Liu, F., et al. (2018). NFATc3 Deficiency Reduces the Classical Activation of Adipose Tissue Macrophages. J. Mol. Endocrinol. 61, 79–89. doi:10.1530/jme-18-0070
Huang, D. W., Sherman, B. T., and Lempicki, R. A. (2009). Bioinformatics Enrichment Tools: Paths toward the Comprehensive Functional Analysis of Large Gene Lists. Nucleic Acids Res. 37, 1–13. doi:10.1093/nar/gkn923
Jiang, L., Kon, T., Chen, C., Ichikawa, R., Zheng, Q., Pei, L., et al. (2021). Whole-genome Sequencing of Endangered Zhoushan Cattle Suggests its Origin and the Association of MC1R with Black Coat Colour. Sci. Rep. 11, 17359. doi:10.1038/s41598-021-96896-2
Karim, L., Takeda, H., Lin, L., Druet, T., Arias, J. A. C., Baurain, D., et al. (2011). Variants Modulating the Expression of a Chromosome Domain Encompassing PLAG1 Influence Bovine Stature. Nat. Genet. 43, 405–413. doi:10.1038/ng.814
Keller, M. C., Visscher, P. M., and Goddard, M. E. (2011). Quantification of Inbreeding Due to Distant Ancestors and its Detection Using Dense Single Nucleotide Polymorphism Data. Genetics 189, 237–249. doi:10.1534/genetics.111.130922
Kirin, M., Mcquillan, R., Franklin, C. S., Campbell, H., Mckeigue, P. M., and Wilson, J. F. (2010). Genomic Runs of Homozygosity Record Population History and Consanguinity. PloS One 5, e13996. doi:10.1371/journal.pone.0013996
Kumar, S., Stecher, G., Li, M., Knyaz, C., and Tamura, K. (2018). MEGA X: Molecular Evolutionary Genetics Analysis across Computing Platforms. Mol. Biol. Evol. 35, 1547–1549. doi:10.1093/molbev/msy096
Lawrence, R. A. (2022). “3-Physiology of Lactation,” in Breastfeeding. Editors R. A. Lawrence,, and R. M. Lawrence. Ninth Edition (Philadelphia: Elsevier), 58–92. doi:10.1016/b978-0-323-68013-4.00003-1
Leinonen, R., Sugawara, H., and Shumway, M, International Nucleotide Sequence Database Collaboration (2011). The Sequence Read Archive. Nucleic Acids Res. 39, D19–D21. doi:10.1093/nar/gkq1019
Li, H., and Durbin, R. (2009). Fast and Accurate Short Read Alignment with Burrows-Wheeler Transform. Bioinformatics 25, 1754–1760. doi:10.1093/bioinformatics/btp324
Ma, Y. (1988). Crossing Improvement Effect of Holstein Cattle and Dengchuan Cattle (In Chinese). Yunnan J. Anim. Sci. Vet. Med. 03, 12–13.
Maddirevula, S., Alzahrani, F., Al-Owain, M., Al Muhaizea, M. A., Kayyali, H. R., Alhashem, A., et al. (2019). Autozygome and High Throughput Confirmation of Disease Genes Candidacy. Genet. Med. 21 (3), 736–742. doi:10.1038/s41436-018-0138-x
McCoard, S., Heiser, A., Lowe, K., Molenaar, A., Maclean, P., Johnstone, P., et al. (2019). Effect of Weaning Age on Growth, Mammary Gland Development, and Immune Function in Holstein Friesian Calves Fed Conserved Alfalfa (FiberStart). J. Dairy Sci. 102, 6076–6087. doi:10.3168/jds.2018-15615
McKenna, A., Hanna, M., Banks, E., Sivachenko, A., Cibulskis, K., Kernytsky, A., et al. (2010). The Genome Analysis Toolkit: a MapReduce Framework for Analyzing Next-Generation DNA Sequencing Data. Genome Res. 20, 1297–1303. doi:10.1101/gr.107524.110
Miles, A. M., and Huson, H. J. (2020). Time- and Population-dependent Genetic Patterns Underlie Bovine Milk Somatic Cell Count. J. Dairy Sci. 103, 8292–8304. doi:10.3168/jds.2020-18322
National Center for Biotechnology Information (2017). Gene: ITSN2 Intersectin 2. Available at: https://www.ncbi.nlm.nih.gov/gene/50618 (Accessed November, 2021).
Negussie, E., Brannang, E., and Rottmann, O. J. (1999). Reproductive Performance and Herd Life of Dairy Cattle at Asella Livestock Farm, Arsi, Ethiopia. II. Crossbreds with 50, 75 and 87.5% European Inheritance. J. Anim. Breed. Genet. 116 (3), 225–234. doi:10.1046/j.1439-0388.1999.00191.x
Nielsen, R., Williamson, S., Kim, Y., Hubisz, M. J., Clark, A. G., and Bustamante, C. (2005). Genomic Scans for Selective Sweeps Using SNP Data. Genome Res. 15, 1566–1575. doi:10.1101/gr.4252305
Palombo, V., Milanesi, M., Sgorlon, S., Capomaccio, S., Mele, M., Nicolazzi, E., et al. (2018). Genome-wide Association Study of Milk Fatty Acid Composition in Italian Simmental and Italian Holstein Cows Using Single Nucleotide Polymorphism Arrays. J. Dairy Sci. 101, 11004–11019. doi:10.3168/jds.2018-14413
Patterson, N., Price, A. L., and Reich, D. (2006). Population Structure and Eigenanalysis. Plos Genet. 2, e190. doi:10.1371/journal.pgen.0020190
Pegorer, M. F., Vasconcelos, J. L. M., Trinca, L. A., Hansen, P. J., and Barros, C. M. (2007). Influence of Sire and Sire Breed (Gyr versus Holstein) on Establishment of Pregnancy and Embryonic Loss in Lactating Holstein Cows during Summer Heat Stress. Theriogenology 67, 692–697. doi:10.1016/j.theriogenology.2006.09.042
Purcell, S., Neale, B., Todd-Brown, K., Thomas, L., Ferreira, M. A. R., Bender, D., et al. (2007). PLINK: a Tool Set for Whole-Genome Association and Population-Based Linkage Analyses. Am. J. Hum. Genet. 81, 559–575. doi:10.1086/519795
Saitou, N., and Nei, M. (1987). The Neighbor-Joining Method: a New Method for Reconstructing Phylogenetic Trees. Mol. Biol. Evol. 4, 406–425. doi:10.1093/oxfordjournals.molbev.a040454
Satrapa, R. A., Nabhan, T., Silva, C. F., Simões, R. A. L., Razza, E. M., Puelker, R. Z., et al. (2011). Influence of Sire Breed (Bos indicus versus Bos taurus) and Interval from slaughter to Oocyte Aspiration on Heat Stress Tolerance of In Vitro-produced Bovine Embryos. Theriogenology 76, 1162–1167. doi:10.1016/j.theriogenology.2011.05.026
Schatz, T., Thomas, S., Reed, S., and Hearnden, M. (2020). Crossbreeding with a Tropically Adapted Bos taurus Breed (Senepol) to Improve Meat Quality and Production from Brahman Herds in Northern Australia. 1. Steer Performance. Anim. Prod. Sci. 60, 487–491. doi:10.1071/AN18609
Schennink, A., Bovenhuis, H., Léon-Kloosterziel, K. M., Van Arendonk, J. A. M., and Visker, M. H. P. W. (2009). Effect of Polymorphisms in the FASN, OLR1, PPARGC1A, PRL and STAT5 Agenes on Bovine Milk-Fat Composition. Anim. Genet. 40, 909–916. doi:10.1111/j.1365-2052.2009.01940.x
Shen, J., Hanif, Q., Cao, Y., Yu, Y., Lei, C., Zhang, G., et al. (2020). Whole Genome Scan and Selection Signatures for Climate Adaption in Yanbian Cattle. Front. Genet. 11, 94. doi:10.3389/fgene.2020.00094
Sterky, F. H., Trotter, J. H., Lee, S.-J., Recktenwald, C. V., Du, X., Zhou, B., et al. (2017). Carbonic Anhydrase-Related Protein CA10 Is an Evolutionarily Conserved Pan-Neurexin Ligand. Proc. Natl. Acad. Sci. U.S.A. 114, E1253–E1262. doi:10.1073/pnas.1621321114
Sun, T., Aceto, N., Meerbrey, K. L., Kessler, J. D., Zhou, C., Migliaccio, I., et al. (2011). Activation of Multiple Proto-Oncogenic Tyrosine Kinases in Breast Cancer via Loss of the PTPN12 Phosphatase. Cell 144, 703–718. doi:10.1016/j.cell.2011.02.003
Utsunomiya, Y. T., Milanesi, M., Fortes, M. R. S., Porto‐Neto, L. R., Utsunomiya, A. T. H., Silva, M. V. G. B., et al. (2019). Genomic Clues of the Evolutionary History of Bos indicus Cattle. Anim. Genet. 50, 557–568. doi:10.1111/age.12836
Valsalan, J., Sadan, T., Venkatachalapathy, T., Anilkumar, K., and Aravindakshan, T. V. (2021). Identification of Novel Single-Nucleotide Polymorphism at Exon1 and 2 Region of B4GALT1 Gene and its Association with Milk Production Traits in Crossbred Cattle of Kerala, India. Anim. Biotechnol. 3, 1–9. doi:10.1080/10495398.2020.1866591
Wang, K., Li, M., and Hakonarson, H. (2010). ANNOVAR: Functional Annotation of Genetic Variants from High-Throughput Sequencing Data. Nucleic Acids Res. 38, e164. doi:10.1093/nar/gkq603
Weikard, R., Kühn, C., Goldammer, T., Freyer, G., and Schwerin, M. (2005). The Bovine PPARGC1A Gene: Molecular Characterization and Association of an SNP with Variation of Milk Fat Synthesis. Physiol. Genomics 21, 1–13. doi:10.1152/physiolgenomics.00103.2004
Xia, X., Zhang, S., Zhang, H., Zhang, Z., Chen, N., Li, Z., et al. (2021). Assessing Genomic Diversity and Signatures of Selection in Jiaxian Red Cattle Using Whole-Genome Sequencing Data. BMC Genomics 22, 43. doi:10.1186/s12864-020-07340-0
Xu, J., Dong, Q., Yu, Y., Niu, B., Ji, D., Li, M., et al. (2018). Mass Spider Silk Production through Targeted Gene Replacement in Bombyx mori. Proc. Natl. Acad. Sci. U.S.A. 115, 8757–8762. doi:10.1073/pnas.1806805115
Yang, L., Su, Z., Li, H., and Zhao, T. (2021). Investigation and Analysis of the Present Situation of Dengchuan Cattle. (In Chinese). Heilongjiang Anim. Sci. Vet. Med. 13, 58–61. doi:10.13881/j.cnki.hljxmsy.2020.01.0281
Zhang, G., and Ma, Y. (1987). Benefit of Progressive Crossing of Dungchuan Cattle (In Chinese). Chin. J. Anim. Sci. 02, 31–32.
Zhang, C., Dong, S.-S., Xu, J.-Y., He, W.-M., and Yang, T.-L. (2019). PopLDdecay: a Fast and Effective Tool for Linkage Disequilibrium Decay Analysis Based on Variant Call Format Files. Bioinformatics 35, 1786–1788. doi:10.1093/bioinformatics/bty875
Zhang, X., Qu, K., Jia, P., Zhang, J., Liu, J., Lei, C., et al. (2021). Assessing Genomic Diversity and Productivity Signatures in Dianzhong Cattle by Whole-Genome Scanning. Front. Genet. 12. doi:10.3389/fgene.2021.719215
Keywords: whole-genome resequencing, Chinese cattle, genetic diversity, population structure, selection signatures, lactation function
Citation: Jin L, Qu K, Hanif Q, Zhang J, Liu J, Chen N, Suolang Q, Lei C and Huang B (2022) Whole-Genome Sequencing of Endangered Dengchuan Cattle Reveals Its Genomic Diversity and Selection Signatures. Front. Genet. 13:833475. doi: 10.3389/fgene.2022.833475
Received: 11 December 2021; Accepted: 04 March 2022;
Published: 29 March 2022.
Edited by:
Tiago do Prado Paim, Goiano Federal Institute (IFGOIANO), BrazilReviewed by:
Ranjit Singh Kataria, National Bureau of Animal Genetic Resources (NBAGR), IndiaQianjun Zhao, Institute of Animal Sciences (CAAS), China
Copyright © 2022 Jin, Qu, Hanif, Zhang, Liu, Chen, Suolang, Lei and Huang. This is an open-access article distributed under the terms of the Creative Commons Attribution License (CC BY). The use, distribution or reproduction in other forums is permitted, provided the original author(s) and the copyright owner(s) are credited and that the original publication in this journal is cited, in accordance with accepted academic practice. No use, distribution or reproduction is permitted which does not comply with these terms.
*Correspondence: Chuzhao Lei, bGVpY2h1emhhbzExMThAMTI2LmNvbQ==; Bizhi Huang, aGJ6QHluYnAuY24=