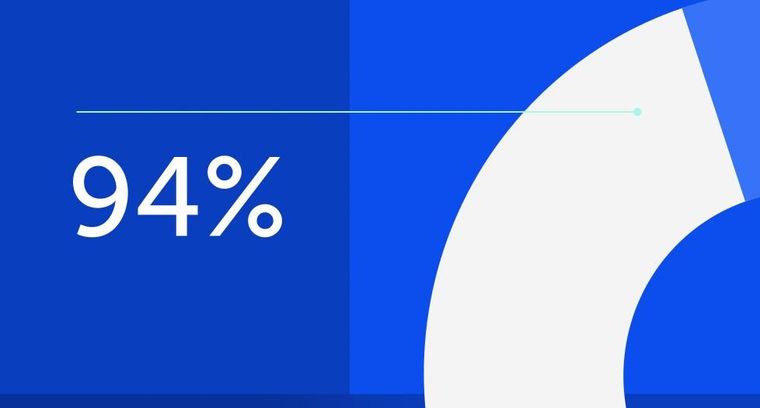
94% of researchers rate our articles as excellent or good
Learn more about the work of our research integrity team to safeguard the quality of each article we publish.
Find out more
REVIEW article
Front. Genet., 04 July 2022
Sec. Epigenomics and Epigenetics
Volume 13 - 2022 | https://doi.org/10.3389/fgene.2022.831452
This article is part of the Research TopicGenomic Imprinting and Monoallelic Gene Expression Mechanisms and ApplicationsView all 8 articles
Methylation tests have been used for decades in regular DNA diagnostics focusing primarily on Imprinting disorders or specific loci annotated to specific disease associated gene promotors. With the introduction of DNA methylation (DNAm) arrays such as the Illumina Infinium HumanMethylation450 Beadchip array or the Illumina Infinium Methylation EPIC Beadchip array (850 k), it has become feasible to study the epigenome in a timely and cost-effective way. This has led to new insights regarding the complexity of well-studied imprinting disorders such as the Beckwith Wiedemann syndrome, but it has also led to the introduction of tests such as EpiSign, implemented as a diagnostic test in which a single array experiment can be compared to databases with known episignatures of multiple genetic disorders, especially neurodevelopmental disorders. The successful use of such DNAm tests is rapidly expanding. More and more disorders are found to be associated with discrete episignatures which enables fast and definite diagnoses, as we have shown. The first examples of environmentally induced clinical disorders characterized by discrete aberrant DNAm are discussed underlining the broad application of DNAm testing in regular diagnostics. Here we discuss exemplary findings in our laboratory covering this broad range of applications and we discuss further use of DNAm tests in the near future.
Due to the Human Genome project and technological improvements, thousands of Human disorders can be diagnosed or prevented with the help of genome diagnostics, improving healthcare and reducing its costs. Genome diagnostics, however, has also confronted us with the limitations of DNA sequencing and other commonly used tests. Human health and disease are not only determined by the DNA code, but also by correct regulation of gene transcription.
Epigenetics studies this regulatory machinery. Epigenetic changes involve changes in the epigenetic modifications of chromatin, e.g., methylation of DNA or modifications of histon proteins. Also, noncoding RNAs such as microRNA or long noncoding RNAs are part of this gene transcription regulatory machinery. The modifications to the chromatin are established and recognized by proteins that either establish-, erase- or read epigenetic marks or are involved in chromatin remodeling (Bjornsson, 2015). Pathogenic mutations in these genes are associated with a large spectrum of clinical conditions often associated with intellectual disability and/or impaired neurological development.
Studying the epigenome leads to more diagnoses and better understanding of the pathogenicity of sequence variants (Aref-Eshghi et al., 2020). In addition, it leads to better understanding of environmental influences such as stress/trauma, intake of toxic substrates or inadequate nutrition in multifactorial diseases. Examples are: cardiovascular disorders, fetal alcohol syndrome, psychiatric conditions, growth malformations or the development of tumors.
Technology has moved from site specific analyses of the epigenetic status of the genome in discrete locus specific disorders to genome wide analyses of complex conditions (Ensink et al., 2021). In particular the use of the DNAm arrays, combined with improved statistics and bioinformatics approaches enables us to study the epigenome in depth, in a cost-effective way. We believe this technology has a high potential in diagnostics leading to personalized approaches in diagnostics, disease prognoses and prediction of treatment outcome.
On the other hand, there are limitations to the use of mDNA arrays in diagnostics. Carriers of Fragile X alleles for instance will not be detected until the allele becomes methylated and causes the disease. The same holds for detection of mosaic cells as seen for instance in the Beckwith Wiedemann syndrome (BWS). In addition, since gene mutations may have multiple episignatures and diseases can be multigenic, the lack of an episignature does not necessarily mean that the disorder is excluded.
During gametogenesis, fertilization, and fetal development, the epigenome drastically changes from highly unmethylated to specific DNAm patterns in specialized cells (Ischida and Moore, 2013). A special form of epigenetic gene regulation is the genomic imprinting phenomenon. For a 100 or so genes, gene regulation is determined by parental origin of the gene, i.e., paternal or maternal allele expression only. Aberrant expression of imprinted genes leads to imprinting disorders (Table 1) (Mackay and Temple, 2017) often associated with aberrant growth or even tumor development as in the Beckwith Wiedemann syndrome (Table 1).
TABLE 1. Overview on the twelve known imprinting disorders. Adapted from Mackay DJG, Temple IK (2017).
Especially in BWS, an overgrowth malformation syndrome mainly characterized by gigantism, macroglossia and exomphalos, DNA diagnostics can be complex. Two imprinted loci on the short arm of chromosome 11 are involved (i.e. imprinting center 1 (IC1) and imprinting center 2 (IC2)). Aberrant methylation can be a result of a local imprinting defect or can be caused by chromosomal abnormalities like uniparental disomies (20%), deletions (1–2%), duplication (2–4%) and translocations (rare) (Brioude et al., 2018). Aberrant methylation patterns of IC1 or IC2 lead to aberrant expression of genes associated with BWS. The best practice guidelines for BWS diagnostics have been published by Brioude et al. (2018), Such aberrant methylation patterns, consisting of loss or gain of methylation, can be studied by site specific tests such as DNAm sensitive Multiplex Ligation-dependent Probe Amplification (MS-MLPA) or alternative site-specific technologies such as MS-PCR or MSqPCR.
An important aspect of BWS diagnostics is childhood tumor prediction since imprinting aberrations in the imprinting center IC1 (also called the H19/IGF2 Differentially Methylated Region, DMR) are associated with high tumor risk whilst those in IC2 (KCNQ1OT1 DMR) are not (Maas et al., 2016; Mussa et al., 2016; Brioude et al., 2018).
Genome wide methylation studies of patients with BWS and other imprinting disorder have discovered multiple aberrant imprinting loci associated with these disorders (MLID or multiple locus imprinting disturbance) (Sanchez Delgado et al., 2016; Fontana et al., 2018). DNAm arrays enabled us to add new aberrantly imprinted loci to this MLID pattern in BWS patients or even detect hypermethylation throughout the methylome in BWS cases (Krzyzewska et al., 2019b). It can therefore be concluded that the underlying (epi)genetic cause for an imprinting disorder can vary substantially between individuals and DNAm arrays enable personalized analyses of these patients. Limitations of DNAm arrays for diagnostic use are the costs of the technique. The Multi Locus MLPA kit (ME034) is more cost effective for the detection of MLID in diagnostics. This kit however is restricted to 7 imprinted regions associated with imprinting disorders (PLAGL1, GRB10, MEST, H19, KCQ1OT1, MEG3, MEG8, SNRPN, PEG3, NESP55, GNAS-AS1, GNASXL, and GNAS A/B). The clinical relevance for the DNAm defects at secondary loci is under investigation.
In the large majority of cases the loci involved in MLID, on top of the previously known disease associated loci, do not contribute to the clinical features of the primary imprinting disorder. Nonetheless, the diagnostic detection of MLID is becoming more and more relevant for routine diagnostic testing. Mutations in maternal effect genes (MEP) have been found in a small percentage of MLID patients and mutations in MEP genes are associated with reduced fertility and miscarriages (Begemann et al., 2018; Gheldof et al., 2019).
A more recent discovery is the existence of Whole Genome paternal Uniparental Disomy (GWpUPD) in an estimated 20% of BWS patients with a routine diagnosis of pUPD11. (Wilson et al., 2008; Kalish et al., 2013). DNAm arrays can be used to detect these genome wide DNAm changes of imprinted loci, but this is hampered by a low detection rate for low mosaicism. For routine diagnostics Multilocus Imprinting MLPA in combination with a SNP-array is recommended. Note that GWpUPD has been reported in association with tumor development at an age of > 7 years (reviewed in Postema et al., 2019), the age at which the increased risk of the development of childhood tumors associated with BWS falls back to the risk in the general population (Brioude et al., 2018).
The diagnostic consequence of all this is difficult to determine at present as more phenotype/(epi)genotype correlations are needed.
Several hundred genes are associated with epigenetic programming. These genes code for writers-. erasers-, readers- or remodelers of the epigenome (chromatin). These genes are important for fetal and adult development and if mutated they cause various, predominantly developmental delay disorders as reviewed by Bjornsson, (2015), Weksberg et al. (2019).
For instance, mutations in the NSD1 gene (encoding an epigenetic writer gene involved in histone methylation) cause Sotos syndrome. Mutations in KMT2D (also an epigenetic writer gene) cause Kabuki syndrome, as do mutations in KDM6A (an eraser gene involved in demethylation of histones) demonstrating the complexity of epigenetic programming. A well-known example of a reader gene causing disease once mutated is the MECP2 gene causing Rett syndrome. Finally, CHD7 is an example of a remodeler gene involved in CHARGEsyndrome.
Mutations in these genes lead to discrete aberrant genome DNAm patterns that are unique for specific disorders (Al-Jawahiri et al., 2022, Aref-Eshghi et al., 2017, 2020, Awamleh et al., 2022, Bend et al., 2019, Butcher et al., 2017, Cappuccio et al., 2020, Chater-Diehl et al., 2019, Cherik et al., 2022, Choufani et al., 2015a, 2020, Cuvertino et al., 2020, Foroutan et al., 2022, Haghshenas et al., 2016; Hood et al., 2016; Krzyzewska et al., 2019a, Levy et al., 2021a (2x), Rooney et al., 2021; Rots et al., 2021; Schenkel et al., 2017, 2018, 2021). and are detected relative to healthy controls and a growing list of various patient cohorts (Figure 1 shows an example of the methylation profile of Sotos syndrome patients relative to controls). In a single DNAm experiment, currently >60 disorders can be diagnosed and this number is growing annually (Levy et al., 2021b, website diagnostics laboratory*). This technology is available as a diagnostic test called Episign (developed by London Health Sciences, Ontario, Canada), which we have implemented in our diagnostic lab in the last few years. The latest version of this test, soon te be released, covers over 100 disorders.
FIGURE 1. Hierarchical clustering heatmap showing different methylation profiles between Sotos syndrome patients (red) and control samples (green) using the top 1,000 most differentially methylated probes between these two groups. Methylation shown from 0 to 1. Patients carrying different types of a variant of unknown significance (grey) map either with controls or with cases, confirming the diagnosis Sotos syndrome in those clustered within the true cases. Samples: publicly available data set GSE74432 (62 samples, Choufani et al., 2015) and an Amsterdam UMC cohort of 15 samples.
The EpiSign test has proven to be very useful in diagnostics in those cases where the pathogenicity of a sequence variant is unknown. If this patient presents with a discrete DNAm pattern specific for the disorder studied, that information confirms the pathogenicity of the sequence variant to a high degree. In approximately 35% of patients referred for an EpiSign test because they carried a variant of unknown significance the (presumed) diagnosis was indeed confirmed. But also, in cases where whole exome sequencing did not reveal any possible causative variant, EpiSign can help to make a diagnosis. Around 10% of such cases displayed a DNAm signature that matched one of the known episignatures (Sadikovic et al., 2021). In some of these cases, subsequent targeted analysis of the gene(s) involved revealed the causative variant after all. Absence of a specific methylation profile however does not necessarily mean that a diagnosis is excluded nor that the variant is not pathogenic. For multiple genes it has been shown that different mutations within that gene can produce different DNAm signatures. In most cases this is accompanied by differences in phenotype, for instance the different episignatures for Nicolaides Baraitser syndrome and blepharophimosis intellectual disability syndrome, both caused by variants in the SMARCA2 gene (Cappuccio et al., 2020). Other examples of genes with multiple signatures are SRCAP (Floating Harbor syndrome and DEHMBA) (Rots et al., 2021), KMT2D (Kabuki syndrome and CHARGE-like phenotype) (Cuvertino et al., 2020) and KAT6B (GTPTS and SBBYSS) (Aref-Eshghi et al., 2020). Interestingly, truncating variants in different parts of the ADNP gene do result in different signatures, however these patients do show only minimal differences in clinical presentation (Bend et al., 2019; Breen et al., 2020).
Due to the increasing number of human disorders that can be diagnosed with the EpiSign technology, these epigenetic tests have a high potential in genome diagnostics (Aref-Eshghi et al., 2020). Besides that, the same technology already enables the diagnostics of imprinted disorders and repeat sequence disorders. Moreover, episignatures may be detected and applied in human diseases associated to other genes than the aforementioned genes that code for chromatin associated proteins, as long as the disorder represents a specific methylation signature. Currently we are studying a number of such disorders such as type II diabetes mellitus (Meeks et al., 2019), obesity (Meeks et al., 2019) and Crohn’s disease (Li Yim et al., 2020), for which we detected dozens of novel disease associated loci. In addition, studies by others reported on numerous other examples of epigenetic changes associated with disease, summarized by Battram et al., 2021. These diseases are prevalent within the general population, generally reflect a heterogeneous phenotype and, obviously, a multi-factorial molecular basis, in accordance with the common variant - common disease hypothesis. The genetic component associated to these diseases often involves dozens to hundreds of genes which only partly can explain their heritability (Manolio et al., 2009). Moreover, such complex and common disorders typically involve a strong environmental component as well. Taken together, this suggests the presence of additional molecular components, for which epigenetics has been shown to be a good candidate. The multi factorial characteristic of these diseases challenges however the detection of clear epigenetic signatures tremendously. Moreover, most of these studies are based on surrogate tissue with respect to the affected tissue in disease, namely whole blood, which hampers any claims regarding causality of consequence effects. These limitations do however not necessarily prevent the detection of predictive epi signatures, in particular when statistical approaches address these adequately by means of in-depth phenotypic characterization (stratification) and application of machine learning methodology.
In case of (genome wide) significant DNAm association, complex traits generally represent aberrant methylation of loci on both alleles. Although such signal represents the average methylation signal of the set of cell (types) from which the DNA originated, allele specific methylation (ASM) is not uncommon as well. Two important ASM phenomena can be distinguished. Firstly, genomic imprinting, which represents the opposite DNAm of the paternal and maternal allele (Weksberg et al., 2019). Secondly, genotype specific methylation, which may comprise local genetic variation that affects DNAm at single and specific genomic positions at (or in vicinity of) the CpG dinucleotide site. Moreover, ASM also has been observed in relation to larger haplotypes, affecting multiple CpG dinucelotides. The average differential methylation signal as a consequence of allele specific DNAm differences is rather easy to detect (relative large effect size). For array based DNAm detection technology such as Illumina Infinium Methylation EPIC Beadchip array (850 k) genetic bias at the single base extension sites or probe sequence (Single Nucleotide Snips, SNPs) often is removed from the dataset, using Illumina’s manifest or dedicated software packages (Shan et al., 2016). However these SNPs can result in the additional detection of clustered DNAm profiles representing a proxy for genetic variance. A limited number of complex trait studies reported on mono allelic DNAm methylation, where differential methylation of the unchanged (CmpG) allele was observed while the other allele included the risk allelele (C > T) (Boks et al., 2016). Similarly, genomic imprinting defects can be detected using DNAm array profiles (Aref Esghi et al., 2020). Nevertheless, such imprinting aberrations often involve uniparental disomy (UPD) mosaicism. The detection of low mosaic (<10%) UPDs using array based technology remains limited. For in depth characterization of ASM, other technological platforms are available. Next generation sequencing (NGS) based methodology, such as whole genome or targeted bisulphite sequencing, can herein be applied. In combination with the limited read length of NGS fragments, its detection of allele specific methylation may be challenging. Recently, third generation sequencing (TGS) was introduced, where DNA (or RNA) can be sequenced directly omitting conversion of DNA and any amplification. Read length of sequenced fragments easily can cover thousands of base pairs. This way the generated genetic information can easily be used to define both alleles and haplotypes. Moreover, these technologies also enable the detection of DNA modifications as well. TGS methodology is a powerful new tool to detect ASM in relation to genetic variance or in relation to genomic imprinting and has already been applied in the field of oncogenomics and imprinting disorders (Gigante et al., 2019; McKelvey et al., 2020).
Currently it is well known that trauma and stress influence our health. At the turn of this century, Vincent Fellitti and coworkers (Fellitti et al., 1998) studied the effect of child abuse and neglect (CAN) in a large cohort of American citizens. He found that these adversechildhood experiences (ACE) resulted in a broad spectrum of health problems later in life. Animal and human studies confirmed that epigenetic changes occurred due to these environmental triggers (Weaver et al., 2004; Mc Gowan et al., 2009; McGowan et al., 2009).
In our laboratory we have studied ACEs and post-traumatic stress disorder (PTSD) in adult and childhood cohorts (Krzyzewska et al., 2018; Nawijn et al., 2019; Ensink et al., 2021) with the Illumina methylation arrays and made the following observations:
- Not all individuals are susceptible to development of PTSD and genetic factors can be found that make individuals resilient to PTSD (Krzyzewska et al., 2018). Genes such as CACNA1C, FKBP4, SDK1, and SKA2 seem to be associated with PTSD resilience. These genes are involved in stress regulation, neuron activity, psychiatric conditions, fear and suicide (Krzyzewska et al., 2018 and references therein).
- Epigenetic changes were predominantly found in genes that were associated with the neuronal dopamine system in the brain. Specific genes such as PAX8 demonstrated significant aberrant DNAm. This gene is a thyroid associated gene that might explain the disturbed thyroid function and sleeping disorders often found in individuals suffering from PTSD.
- Zooming in on PTSD in children we found that genes associated with immune response, neuronal development and stress response were aberrantly methylated compared to non-PTSD controls (healthy and trauma-exposed) (Ensink et al., 2021). Some of these differentially methylated loci correlated also with the activity in the prefrontal and hippocampus part of the brain as studied with MRI. The most significant genes were OLFM3, GDF7, and TNXB. Their cellular function fits well to the PTSD phenotype and brain changes.
- We are currently studying the effect of treatment (EMDR and cognitive therapy) on the epigenome of PTSD children. With machine learning approaches we aim to detect classifiers that enable predicting the response to PTSD intervention beforehand.
- Epigenetic changes due to child abuse and neglect may persist into adulthood. We are currently investigating this. In adults with PTSD we also noticed that aberrant DNAm of the oxytocin receptor gene was present in PTSD adult females, but not in males, which underlines differences in the etiology of PTSD between males and females as it has also been suggested between adults and children (Nawijn et al., 2019; Ensink et al., 2021). This might have consequences for treatment options.
- In a study conducted at our laboratory we analyzed the epigenome of a migrant population in Europe (e.g., residents of Ghana) and were able to associate perceived stress and ethnic discrimination to health expectation, cardiovascular risk, and diabetes (Chilunga et al., 2019; van der Laan et al., 2020). Other studies have reported a correlation between epigenetic markers and risk for cardiovascular disease. (Wada et al., 2021; Kraus et al., 2015; Agha et al., 2019).
In general, we conclude that such studies on environment-epigenome interactions demonstrate a discrete and measurable change at the molecular level. This provides a valuable tool to move forward in understanding these conditions and start diagnosing and treating them.
Alcohol abuse during pregnancy may have devastating effects. In 2019 we demonstrated that children diagnosed with fetal alcohol spectrum disorder (FASD) presented numerous differentially methylated loci compared to non-alcohol in utero exposed controls (Cobben et al.,2019). Although many of those aberrations seem to have occurred randomly, several markers (annotated to the GLI2, TNFRSG19, DTNA, and NECAB3 genes) were found to be significantly associated with the phenotype of FASD. The clinical diagnosis of FASD was based on the golden standard 4-digit score. Besides a range of different clinical features, the 4-digit score primarily takes into account the presence of alcohol exposure, facial abnormalities, brain abnormalities and growth abnormalities (Astley and Clarren, 2000). Currently we apply more sophisticated statistical models to search for a FASD specific DNAm signature and RNA expression studies are ongoing to look for eQTLs.
Apart from alcohol use, other toxic uptake, such as smoking, has a profound effect on the methylation status of the epigenome (Lee and Pausova, 2013). For this reason and together with age, it is generally corrected for as a confounder in studies with mDNA arrays on cohorts (Horvath, 2013).
Malnutrition during pregnancy can have a profound effect on health expectation for the fetus later in life especially if the famine occurred in early pregnancy (Painter et al., 2005; Roseboom et al., 2006; Roseboom et al., 2011; Veenendaal et al., 2013). In animal experiments, researchers described the influence of dietary constraints on the epigenome (Waterland and Jirtle, 2004; Dolinoy et al., 2007). In the Netherlands, two cohorts of women that were pregnant during the Dutch Famine in 1944–1945 and their offspring have been studied in Leiden and Amsterdam (Painter et al., 2005; Heijmans et al., 2008; Tobi et al., 2014).
Epigenetic differences have been linked to the Famine in these cohorts and some of these are already associated with human disease (Tobi et al., 2014). Detailed clinical information have been collected for the Amsterdam cohort consisting of the famine affected children, their mothers and their offspring. We are currently investigating the epigenetic differences and their correlation with clinical features.
Numerous publications are published describing epigenetic genomic loci, in particular regulatory regions, as markers for tumor development and progression. This field of study is too broad to cover in this manuscript. In short: some well-known examples are MLH1 for colon cancer, CDKN2A and HOXA9 for lung cancer, GSTP1 for prostate cancer, H19 for childhood tumors in Beckwith Wiedemann syndrome. Commercial tests are available to monitor markers for color cancer. DNA methylation profiling has significantly improved risk stratification in patients with adult brain tumors (Jaunmuktane et al., 2019). In our hospital, researchers are currently developing an epigenetic direct to consumer test that can be applied at home for HPV screening in relation to cervical cancer. Both array-based methylation tests as well as site specific methylation tests (e.g., promoter methylation or imprinting aberrations) are used in tumor diagnostics (for instance Jaunmuklane et al., 2019).
While classical statistics, based on linear regression models, has been successfully applied to detect disease associated loci in omic based surveys (i.e., genome wide association studies), this methodology is far less suited to generate predictive outcomes. Predictive outcomes can involve prognosis and diagnosis of disease but can also be applied to detect signatures which can be used to predict the outcome of the disease treatment. The latter is a key aspect within personalized medicine and will improve patient care and decrease healthcare cost tremendously (Goecks et al., 2020). The basis of predictive algorithms is machine learning based methodologies, wherein the model is trained and tested applying tens to thousands of iterations. This approach yields a set of classifiers (loci) that together represent an optimal model for predictive purposes. Finally, the accuracy of such predictive model is then determined by its application in an independent (and blinded) cohort of cases and controls, wherein the specificity and sensitivity is validated. The performance success of machine learning methodology is dependent on the sample size. Moreover, these technologies generally work efficiently when the number of features (loci) is limited, which can be achieved by means of so-called dimension reduction. Within the field of molecular science, and in particular for genome diagnostics, both the sample size and number of features (omics) are often suboptimal. Fortunately, several methodologies and workflows have recently been developed that do enable machine learning application on smaller sample sets and high dimensional data. Currently the development of supervised (linear model) and unsupervised (variance) feature selection strategies have been shown to be successful. The methodology developed to detect DNAm signatures within the EpiSign panel is a good example, as previously described by Rooney et al. (2021). In brief, EpiSign signatures are based on a primary feature selection of often a limited but well characterized set of cases and a larger set of (standard) controls using a classic linear model. This analysis yields a limited set of individual features which is then validated using multiple iterations based on a “leave on out” strategy. Finally, the validated features are used in a support vector machine model, wherein specificity and selectivity are determined against every available control of distinct disease associated signature, yielding an accurate epigenetic signature. This strategy works for disorders associated with genes that code for chromatin associated proteins/enzymes. We are currently also applying this workflow on more complex diseases such as FASD and on predicting the response to PTSD therapy The fact that these two examples of complex and multi factorial disorders also yielded epi-signatures, indicates the great potential of machine learning technology. It is expected that future research will focus on further optimization of sample size and dimension reduction issues. Moreover, alternative approaches such as deep learning network analysis and inclusion of multi-omics (e.g. genome wide DNAm and gene expression profiles) based datasets within machine learning algorithms will be implemented (Demirel et al., 2021; Li et al., 2021; van der Vossen et al., 2021).
Epigenetics increasingly plays an important role in medical genetics. Imprinting disorders have paved the way, but on top of this limited diagnostic package we now are able to diagnose an increasing number of human disorders with a single DNAm array test or with site-specific DNAm tests for discrete disorders and cancers.
More and more epigenetic biomarkers are discovered, enabling diagnostics and prognoses. Common multifactorial diseases caused by environmental factors are better understood with epigenetic studies aiding in preventing/predicting, diagnosing and prognosing these high frequency conditions such as cardiovascular disorders or stress related diseases. Our better understanding of epigenetic processes that lead to disease will also facilitate the availability of new drug targets. Epigenetic tests are very specific and often straightforward in interpretation provided the correct test is used for the disease studied (e.g., mDNA arrays have limitations in case of nucleotide expansion disorders or mosaic conditions. Site specific tests have a higher chance of missing relevant loci). Machine learning approaches will facilitate personalized treatment of patients. As discussed, the epigenetic array technology might guide treatment choice. The reversibility of epigenetic changes holds a promise for curing human diseases through a variety of interventions such as pharmacological-, cognitive/EMDR therapy or CRISPR/Cas9 repair. For all this, as always and above all we need good and extensive epigenetics databases of controls and well characterized patient cohorts.
All authors listed have made a substantial, direct, and intellectual contribution to the work and approved it for publication.
This study received funding from the Amsterdam Reproduction Research Institute, grant no ID23230; Methylation profiling as a diagnostic test, and the Augeo Foundation, grant no ID21362; Childhood adversity and epigenetics.
The authors declare that the research was conducted in the absence of any commercial or financial relationships that could be construed as a potential conflict of interest.
All claims expressed in this article are solely those of the authors and do not necessarily represent those of their affiliated organizations, or those of the publisher, the editors and the reviewers. Any product that may be evaluated in this article, or claim that may be made by its manufacturer, is not guaranteed or endorsed by the publisher.
Andrea Venema is acknowledged for her help in preparing Figure 1.
Abreu, A. P., Dauber, A., Macedo, D. B., Noel, S. D., Brito, V. N., Gill, J. C., et al. (2013). Central Precocious Puberty Caused by Mutations in the Imprinted Gene MKRN3. N. Engl. J. Med. 368, 2467–2475. doi:10.1056/NEJMoa1302160
Agha, G., Mendelson, M. M., Ward-Caviness, C. K., Joehanes, R., Huan, T., Gondalia, R., et al. (2019). Blood Leukocyte DNA Methylation Predicts Risk of Future Myocardial Infarction and Coronary Heart Disease. Circulation 140 (8), 645–657. Epub 2019 Aug 19. PMID: 31424985; PMCID: PMC6812683. doi:10.1161/Circulation.AHA.118.039357
Al-Jawahiri, R., Foroutan, A., Kerkhof, J., McConkey, H., Levy, M., Haghshenas, S., et al. (2022). SOX11 Variants Cause a Neurodevelopmental Disorder with Infrequent Ocular Malformations and Hypogonadotropic Hypogonadism and with Distinct DNA Methylation Profile. Genet. Med. 24 (6), 1261–1273. Epub 2022 Mar 24. PMID: 35341651. doi:10.1016/j.gim.2022.02.013
Andrews, S. V., Ladd-Acosta, C., Feinberg, A. P., Hansen, K. D., and Fallin, M. D. (2016). "Gap Hunting" to Characterize Clustered Probe Signals in Illumina Methylation Array Data. Epigenetics Chromatin 9, 56. PMID: 27980682. doi:10.1186/s13072-016-0107-z
Aref-Eshghi, E., Kerkhof, J., Pedro, V. P., Barat-Houari, M., Ruiz-Pallares, N., Andrau, J.-C., et al. (2020). Evaluation of DNA Methylation Episignatures for Diagnosis and Phenotype Correlations in 42 Mendelian Neurodevelopmental Disorders. Am. J. Hum. Genet. 106 (3), 356–370. doi:10.1016/j.ajhg.2020.01.019
Aref-Eshghi, E., Schenkel, L. C., Lin, H., Skinner, C., Ainsworth, P., Paré, G., et al. (2017). The Defining DNA Methylation Signature of Kabuki Syndrome Enables Functional Assessment of Genetic Variants of Unknown Clinical Significance. Epigenetics 12 (11), 923–933. Epub 2017 Nov 7. PMID: 28933623; PMCID: PMC5788422. doi:10.1080/15592294.2017.1381807
Astley, S. J., and Clarren, S. K. (2000). Diagnosing the Full Spectrum of Fetal Alcohol-Exposed Individuals: Introducing the 4-digit Diagnostic Code. Alcohol 35 (4), 400–410. PMID: 10906009. doi:10.1093/alcalc/35.4.400
Awamleh, Z., Chater-Diehl, E., Choufani, S., Wei, E., Kianmahd, R. R., Yu, A., et al. (2022). DNA Methylation Signature Associated with Bohring-Opitz Syndrome: a New Tool for Functional Classification of Variants in ASXL Genes. Eur. J. Hum. Genet. 30, 695–702. Epub ahead of print. PMID: 35361921. doi:10.1038/s41431-022-01083-0
Barel, O., Shalev, S. A., Ofir, R., Cohen, A., Zlotogora, J., Shorer, Z., et al. (2008). Maternally Inherited Birk Barel Mental Retardation Dysmorphism Syndrome Caused by a Mutation in the Genomically Imprinted Potassium Channel KCNK9. Am. J. Hum. Genet. 83 (2), 193–199. PMID 18678320. doi:10.1016/ajhg.2008.07.010
Battram, T., Yousefi, P., Crawford, G., Prince, C., Babaei, M. S., Sharp, G., et al. (2021). The EWAS Catalog: A Database of Epigenome-wide Association Studies. OSF Prepr. 7, 41. doi:10.12688/wellcomeopenres.17598.2
Begemann, M., Rezwan, F. I., Beygo, J., Docherty, L. E., Kolarova, J., Schroeder, C., et al. (2018). Maternal Variants in NLRP and Other Maternal Effect Proteins Are Associated with Multilocus Imprinting Disturbance in Offspring. J. Med. Genet. 55 (7), 497–504. Epub 2018 Mar 24. PMID: 29574422; PMCID: PMC6047157. doi:10.1136/jmedgenet-2017-105190
Bend, E. G., Aref-Eshghi, E., Everman, D. B., Rogers, R. C., Cathey, S. S., Prijoles, E. J., et al. (2019). Gene Domain-specific DNA Methylation Episignatures Highlight Distinct Molecular Entities of ADNP Syndrome. Clin. Epigenet 11, 64. doi:10.1186/s13148-019-0658-5
Bjornsson, H. T. (2015). The Mendelian Disorders of the Epigenetic Machinery. Genome Res. 25 (10), 1473–1481. PMID: 26430157; PMCID: PMC4579332. doi:10.1101/gr.190629.115
Boks, M. P., Rutten, B. P. F., Geuze, E., Houtepen, L. C., Vermetten, E., Kaminsky, Z., et al. (2016). SKA2 Methylation Is Involved in Cortisol Stress Reactivity and Predicts the Development of Post-Traumatic Stress Disorder (PTSD) after Military Deployment. Neuropsychopharmacol 41 (5), 1350–1356. doi:10.1038/npp.2015.286
Breen, M. S., Garg, P., Tang, L., Mendonca, D., Levy, T., Barbosa, M., et al. (2020). Episignatures Stratifying Helsmoortel-Van Der Aa Syndrome Show Modest Correlation with Phenotype. Am. J. Hum. Genet. 107 (3), 555–563. Epub 2020 Aug 5. PMID: 32758449; PMCID: PMC7477006. doi:10.1016/j.ajhg.2020.07.003
Brioude, F., Kalish, J. M., Mussa, A., Foster, A. C., Bliek, J., Ferrero, G. B., et al. (2018). Clinical and Molecular Diagnosis, Screening and Management of Beckwith-Wiedemann Syndrome: an International Consensus Statement. Nat. Rev. Endocrinol. 14 (4), 229–249. PMID: 29377879; PMCID: PMC6022848. doi:10.1038/nrendo.2017.166
Buiting, K. (2010). Prader-Willi Syndrome and Angelman Syndrome. Am. J. Med. Genet. 154C, 365–376. doi:10.1002/ajmg.c.30273
Butcher, D. T., Cytrynbaum, C., Turinsky, A. L., Siu, M. T., Inbar-Feigenberg, M., Mendoza-Londono, R., et al. (2017). CHARGE and Kabuki Syndromes: Gene-specific DNA Methylation Signatures Identify Epigenetic Mechanisms Linking These Clinically Overlapping Conditions. Am. J. Hum. Genet. 100 (5), 773–788. PMID: 28475860; PMCID: PMC5420353. doi:10.1016/j.ajhg.2017.04.004
Cappuccio, G., Sayou, C., Tanno, P. L., Tisserant, E., Bruel, A.-L., and Kennani, S. E. (2020). De Novo SMARCA2 Variants Clustered outside the Helicase Domain Cause a New Recognizable Syndrome with Intellectual Disability and Blepharophimosis Distinct from Nicolaides-Baraitser Syndrome. Genet. Med. 22 (11), 1838–1850. Epub 2020 Jul 22.PMID: 32694869-. doi:10.1038/s41436-020-0898-y
Chater-Diehl, E., Ejaz, R., Cytrynbaum, C., Siu, M. T., Turinsky, A., Choufani, S., et al. (20192019). New Insights into DNA Methylation Signatures: SMARCA2 Variants in Nicolaides-Baraitser Syndrome. BMC Med. Genomics 12 (1), 105. PMID: 31288860; PMCID: PMC6617651. doi:10.1186/s12920-019-0555-y
Cherik, S., Reilly, J., Low, K. J., Dias, C., Havlovicová, M., Hančárová, M., et al. (2022). DNA Methylation Episignature in Gabriele-De Vries Syndrome. Genet. Med. 4 (4), 905–914. Epub 2022 Jan 10. PMID: 35027293. doi:10.1016/j.gim.2021.12.003
Chilunga, F. P., Boateng, D., Henneman, P., Beune, E., Requena-Méndez, A., Meeks, K., et al. (2019). Perceived Discrimination and Stressful Life Events Are Associated with Cardiovascular Risk Score in Migrant and Non-migrant Populations: The RODAM Study. Int. J. Cardiol. 286, 169–174. Epub 2018 Dec 21. PMID: 30638750. doi:10.1016/j.ijcard.2018.12.056
Choufani, S., Cytrynbaum, C., Chung, B. H., Turinsky, A. L., Grafodatskaya, D., Chen, Y. A., et al. (2015). NSD1 Mutations Generate a Genome-wide DNA Methylation Signature. Nat. Commun. 6 (6), 10207. doi:10.1038/ncomms10207
Choufani, S., Gibson, W. T., Turinsky, A. L., Chung, B. H. Y., Wang, T., Garg, K., et al. (2020). DNA Methylation Signature for EZH2 Functionally Classifies Sequence Variants in Three PRC2 Complex Genes. Am. J. Hum. Genet. 106 (5), 596–610. Epub - 2020 Apr 2. PMID: 32243864; PMCID: PMC7212265. doi:10.1016/j.ajhg.2020.03.008
Choufani, S., Shuman, C., and Weksberg, R. (2010). Beckwith-Wiedemann Syndrome. Am. J. Med. Genet. 154C, 343–354. doi:10.1002/ajmg.c.30267
Cobben, J. M., Krzyzewska, I. M., Venema, A., Mul, A. N., Polstra, A., Postma, A. V., et al. (2019). DNA Methylation Abundantly Associates with Fetal Alcohol Spectrum Disorder and its Subphenotypes. Epigenomics 11 (7), 767–785. Epub 2019 Mar 15. PMID: 30873861. doi:10.2217/epi-2018-0221
Cuvertino, S., Hartill, V., Colyer, A., Garner, T., Nair, N., Al-Gazali, L., et al. (2020). A Restricted Spectrum of Missense KMT2D Variants Cause a Multiple Malformations Disorder Distinct fromKabuki Syndrome. Genet. Med. 22 (5), 867–877. doi:10.1038/s41436-019-0743-3
Demirel, H. C., Arici, M. K., and Tuncbag, N. (2021). Computational Approaches Leveraging Integrated Connections of Multi-Omic Data toward Clinical Applications. Mol. Omics 18, 7–18. Online ahead of print. PMID: 34734935. doi:10.1039/d1mo00158b
Dolinoy, D. C., Huang, D., and Jirtle, R. L. (2007). Maternal Nutrient Supplementation Counteracts Bisphenol A-Induced DNA Hypomethylation in Early Development. Proc. Natl. Acad. Sci. U.S.A. 104 (32), 13056–13061. Epub 2007 Aug 1. PMID: 17670942; PMCID: PMC1941790. doi:10.1073/pnas.0703739104
Eggermann, T. (2010). Russell-silver Syndrome. Am. J. Med. Genet. 154C, 355–364. doi:10.1002/ajmg.c.30274
Elli, F. M., Linglart, A., Garin, I., de Sanctis, L., Bordogna, P., Grybek, V., et al. (2016). The Prevalence of GNAS Deficiency-Related Diseases in a Large Cohort of Patients Characterized by the EuroPHP Network. J. Clin. Endocrinol. Metab. 101 (101), 3657–3668. doi:10.1210/jc.2015-4310
Ensink, J. B. M., Keding, T. J., Henneman, P., Venema, A., Papale, L. A., Alisch, R. S., et al. (2021). Differential DNA Methylation Is Associated with Hippocampal Abnormalities in Pediatric Posttraumatic Stress Disorder. Biol. Psychiatry Cognitive Neurosci. Neuroimaging 6 (11), 1063–1070. Epub 2021 May 5. PMID: 33964519; PMCID: PMC8568739. doi:10.1016/j.bpsc.2021.04.016
Felitti, V. J., Anda, R. F., Nordenberg, D., Williamson, D. F., Spitz, A. M., Edwards, V., et al. (1998). Relationship of Childhood Abuse and Household Dysfunction to Many of the Leading Causes of Death in Adults. Am. J. Prev. Med. 14 (4), 245–258. PMID: 9635069. doi:10.1016/s0749-3797(98)00017-8
Fontana, L., Bedeschi, M. F., Maitz, S., Cereda, A., Faré, C., Motta, S., et al. (2018). Characterization of Multi-Locus Imprinting Disturbances and Underlying Genetic Defects in Patients with Chromosome 11p15.5 Related Imprinting Disorders. Epigenetics 13 (9), 897–909. PMID: 30221575; PMCID: PMC6284780. doi:10.1080/15592294.2018.1514230
Foroutan, A., Haghshenas, S., Bhai, P., Levy, M. A., Kerkhof, J., and McConkey, H. (2022). Clinical Utility of a Unique Genome-wide DNA Methylation Signature for KMT2A-Related Syndrome. Int. J. Mol. Sci. 23 (3), 1815. doi:10.3390/ijms23031815PMID: 35163737; PMCID: PMC8836705
Fountain, M. D., Aten, E., Cho, M. T., Juusola, J., Walkiewicz, M. A., Ray, J. W., et al. (2017). The Phenotypic Spectrum of Schaaf-Yang Syndrome: 18 New Affected Individuals from 14 Families. Genet. Med. 19 (1), 45–52. Epub 2016 May 19.PMID: 27195816. doi:10.1038/gim.2016.53
Gheldof, A., Mackay, D. J. G., Cheong, Y., and Verpoest, W. (2019). Genetic Diagnosis of Subfertility: the Impact of Meiosis and Maternal Effects. J. Med. Genet. 56 (5), 271–282. Epub 2019 Feb 6. PMID: 30728173; PMCID: PMC6581078. doi:10.1136/jmedgenet-2018-105513
Gigante, S., Gouil, Q., Lucattini, A., Keniry, A., Beck, T., Tinning, M., et al. (2019). Using Long-Read Sequencing to Detect Imprinted DNA Methylation. Nucleic Acids Res. 47 (8), 46. doi:10.1093/nar/gkz107
Goecks, J., Jalili, V., Heiser, L. M., and Gray, J. W. (2020). How Machine Learning Will Transform Biomedicine. Cell Apr. 181 (1), 92101. doi:10.1016/j.cell2020.03.022
Haghshenas, S., Levy, M. A., Kerkhof, J., Aref-Eshghi, E., McConkey, H., Balci, T., et al. (2016). The Defining DNA Methylation Signature of Floating-Harbor Syndrome. Sci. Rep. 6, 38803. PMID: 27934915; PMCID:PMC5146968. doi:10.1038/srep38803
Heijmans, B. T., Tobi, E. W., Stein, A. D., Putter, H., Blauw, G. J., Susser, E. S., et al. (2008). Persistent Epigenetic Differences Associated with Prenatal Exposure to Famine in Humans. Proc. Natl. Acad. Sci. U. S. A. 51 (44), 495–501. Epub 2008 Oct 27. PMID: 18955703; PMCID: PMC2579375. doi:10.1073/pnas.0806560105
Horvath, S. (2013). DNA Methylation Age of Human Tissues and Cell Types. Genome Biol. 14, R115. doi:10.1186/gb-2013-14-10-r115
Hood, R. L., Schenkel, L. C., Nikkel, S. M., Ainsworth, P. J., Pare, G., Boycott, K. M., et al. (2016). The Defining DNA Methylation Signature of Floating-Harbor Syndrome. Sci. Rep. 6, 38803. doi:10.1038/srep38803
Ioannides, Y., Lokulo-Sodipe, K., Mackay, D. J., Davies, J. H., and Temple, I. K. (2014). Temple Syndrome: Improving the Recognition of an Underdiagnosed Chromosome 14 Imprinting Disorder: an Analysis of 51 Published Cases. J. Med. Genet. doi:10.1136/jmedgenet-2014-102396.pii:jmedgenet2014-102396
Ishida, M., and Moore, G. E. (2013). The Role of Imprinted Genes in Humans. Mol. Aspects Med. 34 (4), 826–840. PMID: 22771538. doi:10.1016/j.mam.2012.06.009
Jaunmuktane, Z., Capper, D., Jones, D. T. W., Schrimpf, D., Sill, M., Dutt, M., et al. (2019). Methylation Array Profiling of Adult Brain Tumours: Diagnostic Outcomes in a Large, Single Centre. Acta Neuropathol. Commun. 7 (1), 24. PMID: 30786920; PMCID: PMC6381711. doi:10.1186/s40478-019-0668-8
Kagami, M., Matsubara, K., Nakabayashi, K., Nakamura, A., Sano, S., Okamura, K., et al. (2017). Genome-wide Multilocus Imprinting Disturbance Analysis in Temple Syndrome and Kagami-Ogata Syndrome. Genet. Med. 19 (4), 476–482. doi:10.1038/gim.2016.123
Kalish, J. M., Conlin, L. K., Bhatti, T. R., Dubbs, H. A., Harris, M. C., Izumi, K., et al. (2013). Clinical Features of Three Girls with Mosaic Genome-wide Paternal Uniparental Isodisomy. Am. J. Med. Genet. 161 (8), 1929–1939. Epub 2013 Jun 26. PMID: 23804593; PMCID: PMC4082120. doi:10.1002/ajmg.a.36045
Kraus, W. E., Muoio, D. M., Stevens, R., Craig, D., Bain, J. R., Grass, E., et al. (2015). Metabolomic Quantitative Trait Loci (mQTL) Mapping Implicates the Ubiquitin Proteasome System in Cardiovascular Disease Pathogenesis. PLoS Genet. 11 (11), e1005553. PMID: 26540294; PMCID: PMC4634848. doi:10.1371/journal.pgen.1005553
Krzyzewska, I. M., Maas, S. M., Henneman, P., Lip, K. V. D., Venema, A., Baranano, K., et al. (2019a). A Genome-wide DNA Methylation Signature for SETD1B-Related Syndrome. ClinEpigenetics 11 (1), 156. PMID:31685013; PMCID: PMC6830011. doi:10.1186/s13148-019-0749-3
Krzyzewska, I. M., Alders, M., Maas, S. M., Bliek, J., Venema, A., Henneman, P., et al. (2019b). Genome-wide Methylation Profiling of Beckwith-Wiedemann Syndrome Patients without Molecular Confirmation after Routine Diagnostics. Clin. Epigenet 11 (1), 53. PMID: 30898153; PMCID: PMC6429826. doi:10.1186/s13148-019-0649-6
Krzyzewska, I. M., Ensink, J. B. M., Nawijn, L., Mul, A. N., Koch, S. B., Venema, A., et al. (2018). Genetic Variant in CACNA1C Is Associated with PTSD in Traumatized Police Officers. Eur. J. Hum. Genet. 26 (2), 247–257. PMID: 29362489; PMCID: PMC5838973. doi:10.1038/s41431-017-0059-1
Lee, K. W. K., and Pausova, Z. (2013). Cigarette Smoking and DNA Methylation. Front. Genet. 4 (4), 132. PMID 23882278. doi:10.3389/fgene.2013.00132
Levy, M. A., Beck, D. B., Metcalfe, K., Douzgou, S., Sithambaram, S., Cottrell, T., et al. (2021). Deficiency of TET3 Leads to a Genome-wide DNA Hypermethylation Episignature in Human Whole Blood. NPJ Genom Med. 6 (1), 92. doi:10.38/s41525-021-00256-y
Levy, M. A., McConkey, H., Kerkhof, J., Barat-Houari, M., Bargiacchi, S., Biamino, E., et al. (2022). Novel Diagnostic DNA Methylation Episignatures Expand and Refine the Epigenetic Landscapes of Mendelian Disorders. Hum. Genet. Genomics Adv. 3 (1), 100075. doi:10.1016/j.xhgg.2021.100075
Li, R., Li, L., Xu, Y., and Yang, J. (2021). Machine Learning Meets Omics: Applications and Perspectives. Brief. Bioinform. Nov. 23, bbab460. Online ahead of print. PMID: 34791021. doi:10.1093/bib/bbab460
Li Yim, A. Y. F., Duijvis, N. W., Ghiboub, M., Sharp, C., Ferrero, E., Mannens, M. M. A. M., et al. (2020). Whole-Genome DNA Methylation Profiling of CD14+ Monocytes Reveals Disease Status and Activity Differences in Crohn's Disease Patients. Jcm 9 (4), 1055. PMID: 32276386. doi:10.3390/jcm9041055
Maas, S. M., Vansenne, F., Kadouch, D. J. M., Ibrahim, A., Bliek, J., Hopman, S., et al. (2016). Phenotype, Cancer Risk, and Surveillance in Beckwith-Wiedemann Syndrome Depending on Molecular Genetic Subgroups. Am. J. Med. Genet. 170 (9), 2248–2260. PMID: 27419809. doi:10.1002/ajmg.a.37801
Mackay, D. J. G., and Temple, I. K. (2017). Human Imprinting Disorders: Principles, Practice, Problems and Progress. Eur. J. Med. Genet. 60 (11), 618–626. PMID: 28818477. doi:10.1016/j.ejmg.2017.08.014
Mackay, D. J. G., and Temple, I. K. (2010). Transient Neonatal Diabetes Mellitus Type 1. Am. J. Med. Genet. 154C (3), 335–342. PMID: 20803656. doi:10.1002/ajmg.c.30272.Review
Manolio, T. A., Collins, F. S., Cox, N. J., Goldstein, D. B., Hindorff, L. A., Hunter, D. J., et al. (2009). Finding the Missing Heritability of Complex Diseases. Nature 461 (7265), 747–753. PMID: 19812666. doi:10.1038/nature08494
Mantovani, G., Spada, A., and Elli, F. M. (2016). Pseudohypoparathyroidism and Gsα-cAMP-Linked Disorders: Current View and Open Issues. Nat. Rev. Endocrinol. 12, 347–356. doi:10.1038/nrendo.2016.52
McGowan, P. O., Sasaki, A., D'Alessio, A. C., Dymov, S., Labonté, B., Szyf, M., et al. (2009). Epigenetic Regulation of the Glucocorticoid Receptor in Human Brain Associates with Childhood Abuse. Nat. Neurosci. 12 (3), 342–348. PMID: 19234457; PMCID: PMC2944040. doi:10.1038/nn.2270
McKelvey, B. A., Gilpatrick, T., Wang, Y., Timp, W., Umbricht, C. B., and Zeiger, M. A. (2020). Characterization of Allele-specific Regulation of Telomerase Reverse Transcriptase in Promoter Mutant Thyroid Cancer Cell Lines. Thyroid 30 (10), 1470–1481. PMID: 32228178. doi:10.1089/thy.2020.0055
Meeks, K. A. C., Henneman, P., Venema, A., Addo, J., Bahendeka, S., and Burr, T. (2019). Epigenome-wide Association Study in Whole Blood on Type 2 Diabetes Among Sub-saharan African Individuals: Findings from the RODAM Study. Int. J. Epidemiol. 48 (1), 58–70. PMID: 301075McGowan PO. doi:10.1093/ije/dyy171
Mulchandani, S., Bhoj, E. J., Luo, M., Powell-Hamilton, N., Jenny, K., Gripp, K. W., et al. (2015). Maternal Uniparental Disomy of Chromosome 20: a Novel Imprinting Disorder of Growth Failure. Genet. Med. 18, 309. doi:10.1038/gim.2015.103
Mussa, A., Molinatto, C., Baldassarre, G., Riberi, E., Russo, S., Larizza, L., et al. (2016). Cancer Risk in Beckwith-Wiedemann Syndrome: A Systematic Review and Meta-Analysis Outlining a Novel (Epi)Genotype Specific Histotype Targeted Screening Protocol. J. Pediatr. 176, 142–149.e1. Epub 2016 Jun 29. PMID: 27372391. doi:10.1016/j.jpeds.2016.05.038
Nawijn, L., Krzyzewska, I. M., van Zuiden, M., Henneman, P., Koch, S. B. J., Mul, A. N., et al. (2019). Oxytocin Receptor Gene Methylation in Male and Female PTSD Patients and Trauma-Exposed Controls. Eur. Neuropsychopharmacol. 29 (1), 147–155. Epub 2018 Nov 8. PMID: 30415783. doi:10.1016/j.euroneuro.2018.10.006
Ogata, T., and Kagami, M. (2016). Kagami-Ogata Syndrome: a Clinically Recognizable Upd(14)pat and Related Disorder Affecting the Chromosome 14q32.2 Imprinted Region. J. Hum. Genet. 61, 87–94. doi:10.1038/jhg.2015.113
Painter, R. C., Roseboom, T. J., and Bleker, O. P. (2005). Prenatal Exposure to the Dutch Famine and Disease in Later Life: an Overview. Reprod. Toxicol. 20 (3), 345–352. PMID: 15893910. doi:10.1016/j.reprotox.2005.04.005
Postema, F. A. M., Bliek, J., van Noesel, C. J. M., Van Zutven, L. J. C. M., Oosterwijk, J. C., Hopman, S. M. J., et al. (2019). Multiple Tumors Due to Mosaic Genome-wide Paternal Uniparental Disomy. Pediatr. Blood Cancer 66 (6), e27715. Epub 2019 Mar 18. PMID:30882989. doi:10.1002/pbc.27715
Rooney, K., Levy, M. A., Haghshenas, S., Kerkhof, J., Rogaia, D., Tedesco, M. G., et al. (2021). Identification of a DNA Methylation Episignature in the 22q11.2 Deletion Syndrome. Int. J. Mol. Sci. 22 (16), 8611. PMCID: PMC8395258. PMID: 34445317. doi:10.3390/ijms22168611
Roseboom, T., de Rooij, S., and Painter, R. (2006). The Dutch Famine and its Long-Term Consequences for Adult Health. Early Hum. Dev. 82 (8), 485–491. Epub 2006 Jul 28. PMID: 16876341. doi:10.1016/j.earlhumdev.2006.07.001
Roseboom, T. J., Painter, R. C., van Abeelen, A. F. M., Veenendaal, M. V. E., and de Rooij, S. R. (2011). Hungry in the Womb: what Are the Consequences? Lessons from the Dutch Famine. Maturitas 70 (2), 141–145. Epub 2011 Jul 28. PMID: 21802226. doi:10.1016/j.maturitas.2011.06.017
Rots, D., Chater-Diehl, E., Dingemans, A. J. M., Goodman, S. J., Siu, M. T., Cytrynbaum, C., et al. (2021). Truncating SRCAP Variants outside the Floating-Harbor Syndrome Locus Cause a Distinct Neurodevelopmental Disorder with a Specific DNA Methylation Signature. Am. J. Hum. Genet. 108 (6), 1053–1068. Epub 2021 Apr 27.PMID: 33909990. doi:10.1016/j.ajhg.2021.04.008
Sadikovic, B., Levy, M. A., Kerkhof, J., Aref-Eshghi, E., Schenkel, L., Stuart, A., et al. (2021). Clinical Epigenomics: Genome-wide DNA Methylation Analysis for the Diagnosis of Mendelian Disorders. Genet. Med. 23 (6), 1065–1074. Erratum in: Genet Med. 2021 Feb 26;: PMID: 33547396; PMCID: PMC8187150. doi:10.1038/s41436-020-01096-4
Sanchez-Delgado, M., Riccio, A., Eggermann, T., Maher, E. R., Lapunzina, P., Mackay, D., et al. (2016). Causes and Consequences of Multi-Locus Imprinting Disturbances in Humans. Trends Genet. 32 (7), 444–455. Epub 2016 May 24. PMID: 27235113. doi:10.1016/j.tig.2016.05.001
Schenkel, L. C., Aref-Eshghi, E., Rooney, K., Kerkhof, J., Levy, M. A., McConkey, H., et al. (2021). DNA Methylation Epi-Signature Is Associated with Two Molecularly and Phenotypically Distinct Clinical Subtypes of Phelan-McDermid Syndrome. Clin. Epigenet 13 (1), 2. PMID: 33407854; PMCID: PMC7789817. doi:10.1186/s13148-020-00990-7
Schenkel, L. C., Aref-Eshghi, E., Skinner, C., Ainsworth, P., Lin, H., Paré, G., et al. (2018). Peripheral Blood Epi-Signature of Claes-Jensen Syndrome Enables Sensitive and Specific Identification of Patients and Healthy Carriers with Pathogenic Mutations in KDM5C. Clin. Epigenet 10, 21. PMID: 29456765; PMCID:- PMC5813334. doi:10.1186/s13148-018-0453-8
Schenkel, L. C., Kernohan, K. D., McBride, A., Reina, D., Hodge, A., Ainsworth, P. J., et al. (2017). Identification of Epigenetic Signature Associated with Alpha Thalassemia/mental Retardation X-Linked Syndrome. Epigenetics Chromatin 10, 10. PMID: 28293299; PMCID: PMC5345252. doi:10.1186/s13072-017-0118-4
Tobi, E. W., Goeman, J. J., Monajemi, R., Gu, H., Putter, H., Zhang, Y., et al. (2014). DNA Methylation Signatures Link Prenatal Famine Exposure to Growth and Metabolism. Nat. Commun. Nov. 5 (5), 5592. PMID: 25424739; PMCID: PMC4246417. doi:10.1038/ncomms6592.Erratum
Shan, V. A., Ladd-Acosta, C. L., Feinberg, A. P., Hansen, K. D., and Fallin, D. (2016). Gap Hunting to Characterize Clustered Probe Signals in Illumina Methylation Array Date. Epigenetic chromatin dec. 7 (9), 6. doi:10.1186/s13072-016-0107-z
van der Laan, L. C., Meeks, K. A. C., Chilunga, F. P., Agyemang, C., Venema, A., Mannens, M. M. A. M., et al. (2020). Epigenome-wide Association Study for Perceived Discrimination Among Sub-saharan African Migrants in Europe - the RODAM Study. Sci. Rep. 10 (1), 4919. PMID: 32188935; PMCID: PMC7080832. doi:10.1038/s41598-020-61649-0
van der Vossen, E. W. J., Bastos, D., Stols-Gonçalves, D., de Goffau, M. C., Davids, M., Pereira, J. P. B., et al. (2021). Effects of Fecal Microbiota Transplant on DNA Methylation in Subjects with Metabolic Syndrome. Gut Microbes 13 (1), 1993513. PMID: 34747338. doi:10.1080/19490976.2021.1993513
Veenendaal, M., Painter, R., de Rooij, S., Bossuyt, P., van der Post, J., Gluckman, P., et al. (2013). Transgenerational Effects of Prenatal Exposure to the 1944-45 Dutch Famine. Bjog 120 (5), 548–554. PMID: 23346894. doi:10.1111/1471-0528.12136
Wada, K., Misaka, T., Yokokawa, T., Kimishima, Y., Kaneshiro, T., Oikawa, M., et al. (2021). Blood‐Based Epigenetic Markers of FKBP5 Gene Methylation in Patients with Dilated Cardiomyopathy. Jaha 10 (21), e021101. Epub 2021 Oct 29. PMID: 34713710. doi:10.1161/JAHA.121.021101
Wakeling, E. L., Brioude, F., Lokulo-Sodipe, O., O'Connell, S. M., Salem, J., Bliek, J., et al. (2016). Diagnosis and Management of Silver-Russell Syndrome: First International Consensus Statement. Nat. Rev. Endocrinol. 13, 105–124. doi:10.1038/nrendo.2016.138
Waterland, R. A., and Jirtle, R. L. (2004). Early Nutrition, Epigenetic Changes at Transposons and Imprinted Genes, and Enhanced Susceptibility to Adult Chronic Diseases. Nutrition 20 (1), 63–68. PMID: 14698016. doi:10.1016/j.nut.2003.09.011
Weaver, I. C. G., Cervoni, N., Champagne, F. A., D'Alessio, A. C., Sharma, S., Seckl, J. R., et al. (2004). Epigenetic Programming by Maternal Behavior. Nat. Neurosci. 7 (8), 847–854. Epub 2004 Jun 27. doi:10.1038/nn1276
Weksberg, R., Butcher, D. T., Cytrynbaum, C., Siu, M. T., Choufani, S., and Tycko, B. (2019) Epigenetics Pp 79-123 in “Emery & Rimoin’s Principles and Practice of Medical Genetics. Foundations. in Churchill Livingstone. Editors R. E. Pyeritz, B. R. Korf, and W. W. Grody. 7 edition (Philadelphia: Elsevier).
Keywords: genomic imprinting, DNAm arrays, episign, epigenome, genome diagnostics
Citation: Mannens MMAM, Lombardi MP, Alders M, Henneman P and Bliek J (2022) Further Introduction of DNA Methylation (DNAm) Arrays in Regular Diagnostics. Front. Genet. 13:831452. doi: 10.3389/fgene.2022.831452
Received: 08 December 2021; Accepted: 08 June 2022;
Published: 04 July 2022.
Edited by:
Benjamin Tycko, Center for Discovery and Innovation, Hackensack Meridian Health, United StatesReviewed by:
Jeffrey Mark Craig, Murdoch Childrens Research Institute, AustraliaCopyright © 2022 Mannens, Lombardi, Alders, Henneman and Bliek. This is an open-access article distributed under the terms of the Creative Commons Attribution License (CC BY). The use, distribution or reproduction in other forums is permitted, provided the original author(s) and the copyright owner(s) are credited and that the original publication in this journal is cited, in accordance with accepted academic practice. No use, distribution or reproduction is permitted which does not comply with these terms.
*Correspondence: M. M. A. M. Mannens, bS5hLm1hbm5lbnNAYW1zdGVyZGFtdW1jLm5s
Disclaimer: All claims expressed in this article are solely those of the authors and do not necessarily represent those of their affiliated organizations, or those of the publisher, the editors and the reviewers. Any product that may be evaluated in this article or claim that may be made by its manufacturer is not guaranteed or endorsed by the publisher.
Research integrity at Frontiers
Learn more about the work of our research integrity team to safeguard the quality of each article we publish.