- Department of Animal Science, Faculty of Agriculture, University of Tabriz, Tabriz, Iran
Accurate normalization of the gene expression assays, using housekeeping genes (HKGs), is critically necessary. To do so, selection of a proper set of HKGs for a specific experiment is of great importance. Despite many studies, there is no consensus about the suitable set of HKGs for implementing in the quantitative real-time PCR analyses of chicken tissues. A limited number of HKGs have been widely used. However, wide utilization of a little number of HKGs for all tissues is challenging. The emergence of high-throughput gene expression RNA-seq data has enabled the simultaneous comparison of the stability of multiple HKGs. Therefore, employing the average coefficient of variations of at least three datasets per tissue, we sorted all reliably expressed genes (REGs; with FPKM ≥ 1 in at least one sample) and introduced the top 10 most suitable and stable reference genes for each of the 16 chicken tissues. We evaluated the consistency of the results of five tissues using the same methodology on other datasets. Furthermore, we assessed 96 previously widely used HKGs (WU-HKGs) in order to challenge the accuracy of the previous studies. The New Tuxedo software suite was used for the main analyses. The results revealed novel, different sets of reference genes for each of the tissues with 17 common genes among the top 10 genes lists of 16 tissues. The results did disprove the suitability of WU-HKGs such as Actb, Ldha, Scd, B2m, and Hprt1 for any of the tissues examined. On the contrary, a total of 6, 13, 14, 23, and 32 validated housekeeping genes (V-HKGs) were discovered as the most stable and suitable reference genes for muscle, spleen, liver, heart, and kidney tissues, respectively. Although we identified a few new HKGs usable for multiple tissues, the selection of suitable HKGs is required to be tissue specific. The newly introduced reference genes from the present study, despite lacking experimental validation, will be able to contribute to the more accurate normalization for future expression analysis of chicken genes.
Introduction
Housekeeping genes (HKGs), by definition, are genes required for the maintenance of basal cellular function, irrespective of their specific roles in the tissue or organism. HKGs are expected to express stably in all tissues of an organism under different conditions, regardless of developmental stage, sex, or external stressors (e.g., heat stress, disease, and immunological challenge, among others). Full characterization of a minimal set of genes that are required to sustain the life of a tissue is of particular interest (Eisenberg and Levanon, 2013). The current trend of analyses of global gene expression data using microarray or RNA-seq technologies has enabled the simultaneous analysis of tens of thousands of genes. However, quantitative real-time PCR (qPCR) has remained the only valid, more preferred independent tool for validating the results of genome-wide gene expression analyses (VanGuilder et al., 2008). The reliability of the final quantification result of qPCR depends heavily on the utilization of one or multiple internal reference genes for the normalization of the expression of the genes of interest. Normalization to a set of HKGs is nowadays a current and crucial procedure and is preferred to the normalization to a single reference gene. Therefore, the identification of at least two proper, stable HKGs for a specified tissue is crucial (Bagés et al., 2015).
The simultaneous analysis of a large number of genes was not possible until the emergence of high-throughput next-generation sequencing data. Although the evaluation of expression stability of potential reference genes has been carried out earlier for several tissues of chicken (Yang et al., 2013; Olias et al., 2014; Oliveira et al., 2017; Hassanpour et al., 2018; Zhang et al., 2018), the methods of choice of almost all of them were merely based on the qPCR and utilization of the BestKeeper (Pfaffl et al., 2004), geNorm (Vandesompele et al., 2002), and NormFinder (Andersen et al., 2004) statistical algorithms. To our knowledge, the work on identifying suitable HKGs using high-throughput microarray or RNA-seq data is scarce and limited to only some plant species including olive and Arabidopsis (Zhuo et al., 2016; Carmona et al., 2017), grapevine (González-agüero et al., 2013), white campion (Zemp et al., 2014), and some insects and animals including sweet potato whitefly (Su et al., 2013), Arctic charr (Pashay Ahi et al., 2013), human (Carmona et al., 2017), and human and mouse (de Jonge et al., 2007). However, there is no comprehensive study to address the most suited HKGs in chicken using RNA-seq data. In the present work, we tested most of the reliably expressed genes (REGs) for stability in the 16 important chicken tissues using at least three RNA-seq datasets per tissue and reported 10 most stably expressed genes for each of them to be used as proper sets of HKGs in the future gene expression assays. In addition, the consistency of the results was evaluated for five tissues, namely, heart, kidney, liver, muscle, and spleen. The evaluation step was not performed for the remaining 11 tissues as a sufficient, required number of high-depth datasets were not available.
Materials and methods
Selection of the desired tissues for study
Based on the importance of the tissues in research and availability of sufficient gene expression data, we selected 16 chicken tissues, namely, adipose, blood, brain, bursa of Fabricius, duodenum, heart, ileum, jejunum, kidney, liver, lung, muscle, ovary, skin, spleen, and trachea, for the current work. There were no sufficient datasets (at least three) for other tissues in the databases.
RNA-seq data collection from databases
The required RNA-seq datasets were downloaded from NCBI (https://www.ncbi.nlm.nih.gov). At least three datasets were downloaded per tissue. Each dataset should have at least three replicated samples for case group and at least three replicated samples for control or another treatment group. The treatment of the case samples was not important as the only criterion for the comparison was to compare the expression of genes between case (treated) and control (untreated) or another treated group. For simplicity, the two mentioned groups will be called case and control, hereafter. The data were downloaded in SRA format using the SRA Toolkit and converted into fastq format using the fastq-dump tool. In Table 1, the accession numbers are shown, and in Supplementary Table S1, the meta information of the used datasets are presented.
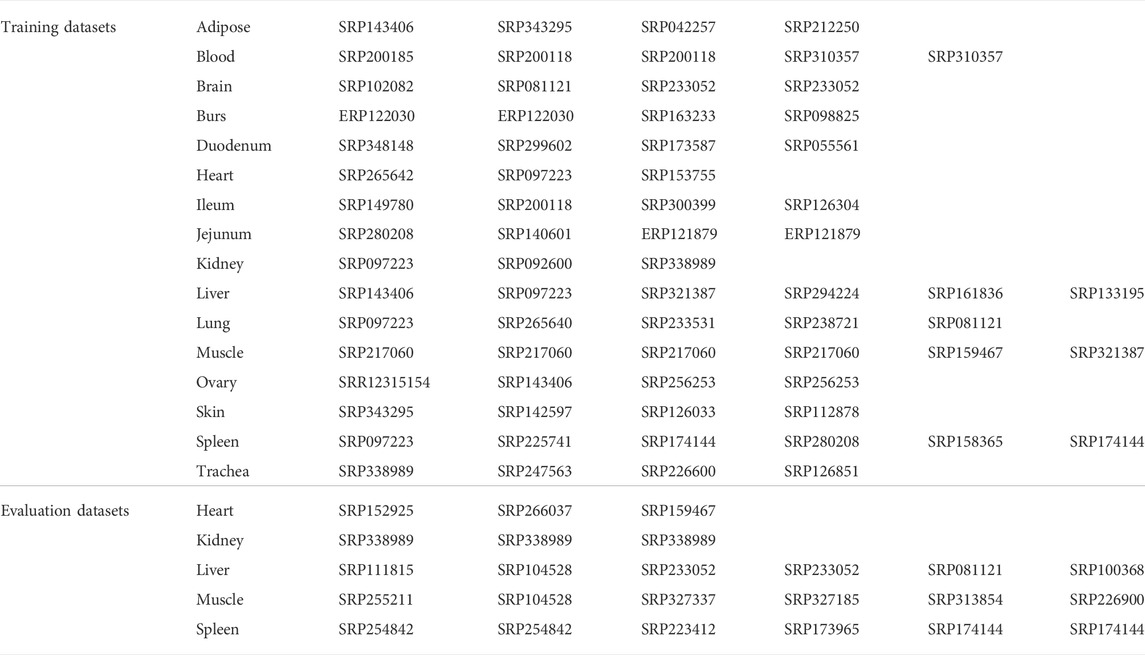
TABLE 1. Accession numbers of the used datasets for discovering the most stably expressed genes for 16 chicken tissues.
Required conditions for the datasets to be chosen for the analyses
The main question of the current work was “Which HKGs are the most suitable?” It is obvious that genes with the lowest expression differences between the case and control groups within a specified dataset as well as with expression sustainability among all datasets of a specified tissue could be considered as the most suitable HKGs. Since at least three datasets were analyzed per tissue, genes with the highest consistency of expression across all the experiments were finally introduced as the most proper sets of HKGs. We screened the NCBI database exhaustively to download only the datasets that address the question of research. Therefore, we downloaded only the datasets with the following conditions: 1) Illumina paired-end RNA-seq data (no single-end data used); 2) each of the tissues should have at least three datasets; 3) each dataset could be subset into only two case and control groups; and 4) each case group and control group should have at least three replicate samples.
Analyses of individual datasets
The individual datasets were analyzed separately using the New Tuxedo software suite employing the Ensembl Gallus gallus Build 6.0 reference genome (https://asia.ensembl.org/Gallus_gallus/info/index). At first, Fastqc (Andrews, 2010) and Trimmomatic (Bolger et al., 2014) software tools were employed for quality control and trimming, respectively. Datasets with insufficient quality metrics were excluded. The data were trimmed using ILLUMINACLIP, SLIDING WINDOW (window size 3–5 and Phred quality mean of 20–28), CROP (to trim 3–10 left-end nucleotides), AVGQUAL (minimum Phred quality of 20–25), and MINLEN (read length ≥ 40–45) options. Depending on the dataset, the values were varied. The Hisat2 software (Kim et al., 2019) (available at https://daehwankimlab.github.io/hisat2) was used for both indexing of the genome and mapping of the clean reads onto the indexed reference genome. The Stringtie software (Kovaka et al., 2019) (available at http://ccb.jhu.edu/software/stringtie) was used for assembly of transcripts of each sample using the -G option that forces the assembly to be limited to only the known genes. The transcripts of all samples of all experiments were assembled using the merge option of Stringtie. The Cuffdiff software (Trapnell et al., 2010) (available in http://cole-trapnell-lab.github.io/cufflinks) was used for differential expression analysis between the case and control groups with the multiread and bias correction options enabled. Genes with considerable expression differences between the two mentioned groups resulted in statistically significant differences, while genes with constant expressions between the two groups have p-values approximate to 1. Genes with unreliable expression (FPKM < 1) in all samples of a dataset were excluded. The expression (FPKM) values of all samples within a specified experiment were gathered, and mean, variation, and coefficient of variation (CV) statistics were calculated for each gene. Only REGs (i.e., genes passing the aforementioned filter in all datasets of a tissue) were considered for the discovery of the stably expressed genes. Then, average CV was calculated for each gene across all datasets within a specified tissue. Genes were sorted in ascending order and ranked based on the average CV, and those with the lowest average CV values were reported as the most stable and suitable housekeeping genes. The flowchart of the analyses is shown in Figure 1. This process was repeated for all 16 tissues, and the top 10 most stably expressed genes were reported for each tissue separately.
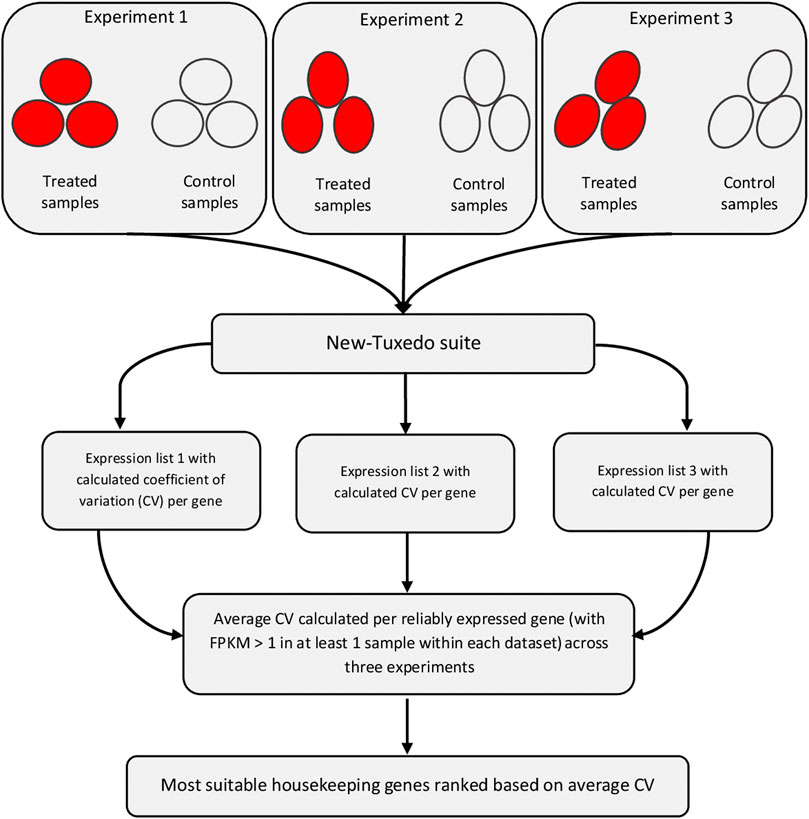
FIGURE 1. Flowchart of detection of the most suitable housekeeping genes for a specific tissue. This workflow was repeated for all tissues separately. At least three datasets were analyzed per tissue. The comparisons within each experiment were performed between the treated and control groups, each with at least three replicates. All the used datasets are publicly available data generated using Illumina paired-end RNA-Sequencing method. Software programs within the New Tuxedo suite were hisat2 (for mapping of reads onto reference genome), Stringtie (for assembly and read counting), and Cuffdiff (for differentiall expression analysis). Expression stability was monitored per gene based on the coefficient of variation (CV), and ranking of most stably expressed genes were performed based on the average CV criterion.
Gene ontology and pathway enrichment analyses of the most stably expressed genes
All the top 10 genes of the 16 tissues were gathered, and the duplicated genes were deduplicated. In the end, a total of 139 unique genes were submitted to gene ontology and pathway enrichment analyses using the DAVID web-based software (Jiao et al., 2012) (available at https://david.ncifcrf.gov) in order to understand the functions and to gain insight into the pathways that the less variable genes are involved in.
Evaluation of the consistency of the results
For five of the tissues, there were six or more datasets. For each of heart and kidney tissues, there were six datasets, and for each of liver, muscle, and spleen tissues, there were 12 datasets. We analyzed half of the datasets of the mentioned tissues as training and the second half for the evaluation of the consistency of the results of the training datasets. We named the second set as evaluation datasets. As for the training datasets, the REGs of the evaluation datasets were also ranked based on the average CV. The top 100 genes of the training datasets were compared with those of the evaluation datasets and those genes that were in common in both of the top 100 genes lists were reported as validated housekeeping genes (V-HKGs). The more the counts of matched genes between the two top 100 genes lists, the greater the accuracy and repeatability of discovering the stable genes.
Assessment of the suitability of widely used housekeeping genes
A total of 96 WU-HKGs were selected from the literature. The list of WU-HKGs and their corresponding citations are shown in Supplementary Table S2. Gene expression analysis using a real-time PCR assay was the main subject of the reviewed papers. The main objective of the current section was to challenge the accuracy of the previously conducted gene expression studies that had used nonproper HKGs.
Results and discussion
We used 94 datasets (70 for training and 24 for evaluation) sourced from 16 tissues, namely, adipose, blood, brain, bursa of Fabricius, duodenum, heart, ileum, jejunum, kidney, liver, lung, muscle, ovary, skin, spleen, and trachea. A total of 23,403 genes were analyzed in each dataset, and almost 3,000–11,000 REGs (with FPKM value ≥ 1 in at least one sample of a datasets) were assessed for the stability of expression. In contrast, nearly 50–85% of genes were filtered out because of the inconsistency of expression or because of low coverage of sequencing in some of the datasets. It is worth mentioning that only REGs of all datasets within a tissue were allowed for the final analysis. For tissues with more available datasets (such as spleen, liver, and muscle), we only chose the datasets with sufficiently deep sequencing, because the low coverage datasets would not guarantee the possibility of the evaluation of all REGs. In contrast, for less studied tissues that had no abundant datasets available, we decided to utilize all available datasets regardless of their sequencing depths. It is obvious that the insufficient coverage of datasets will cause the number of analyzed genes to be reduced. The total number of datasets and REGs for each of the tissues are reported in Supplementary Table S3.
To identify the most stable reference genes, we first checked the relation of mean of expression and variation. We observed no relation between the mentioned coefficients, and thereby, the suggested reference genes can be used for the normalization of the interested genes irrespective of their expression levels. In Figure 2, a scatterplot demonstrating the relation of mean and CV of one experiment of adipose tissue is shown. Scatterplots of the remaining experiments were similar and therefore were not shown here.
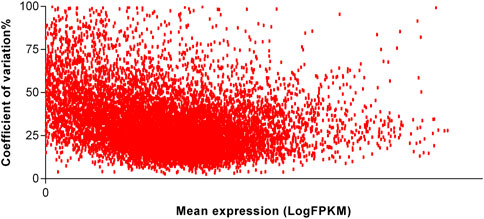
FIGURE 2. Scatterplot of the reliably expressed genes of one dataset of adipose tissue. The relationship of mean (in log10 scale) and variation of expression of genes were assessed in order to understand whether the variability of expression is increased with the mean of expression. The expression values are given as fragments per kilobase of transcript per million reads in log10 scale.
The methodology used in the present work to find the most stable reference genes revealed interesting results. Almost all of the introduced reference genes (i.e., top 10 most stably expressed genes) were new, indicating that the previously used HKGs were not as stable as required for a gene to be considered as a proper HKG. Only eight (8.3%) of the WU-HKGs were present in the top 10 lists of five tissues including Rpl4 for adipose; Oaz1, Rpl27a, and Gapdh for blood; Rpl6 for jejunum; Gusb and Polr2b for kidney; and Ap2m1 for liver. The top 10 most stably expressed genes introduced here for the remaining tissues were completely novel. In Table 2, the top 10 most stable reference genes are reported for the studied tissues.
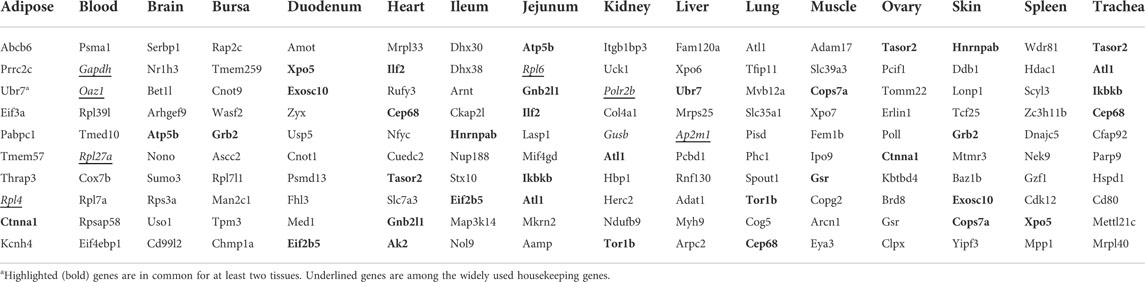
TABLE 2. Top 10 most suitable reference genes for chicken tissues based on average coefficient of variations (CVs) across at least three experiments.
As can be seen in Table 2, some of the introduced reference genes (17 genes) were in common in the top 10 lists of at least two tissues. For example, Atl1 was identified as suitable for four tissues. Cep68 and Tasor2 genes were in common in the lists of three tissues, and Ubr7, Ctnna1, Atp5b, Grb2, Xpo5, Exosc10, Eif2b5, Ilf2, Gnb2l1, Hnrnpab, Ikbkb, Tor1b, cops7a, and Gsr genes were in common in the top 10 lists of two tissues. It is obvious that genes with more frequent occurrence in multiple top 10 genes lists are more likely suggested as suitable HKGs than are those with only one occurrence. Therefore, these 17 genes are strongly suggested to be used as HKGs for the mentioned tissues.
Gene ontology and Kyoto Encyclopedia of Genes and Genomes pathway analyses of the most stably expressed genes
Understanding the functionality of the most stable reference genes is of great importance. In the current work, the top 10 most stable genes of all 16 studied tissues (totally 134 unique genes) were subjected to gene ontology and KEGG pathway enrichment analyses using the DAVID web tool. The results revealed that the most stably expressed genes that enriched significantly (p < 0.05) in molecular function terms, biological processes terms, and KEGG pathways were generally related with the transcription and translation of proteins (e.g., RNA binding, protein binding, structural constituent of ribosome, translational initiation, positive regulation of transcription, cytoplasmic translation, nucleocytoplasmic transport, RNA degradation, and ribosome, among others). In other words, as compared to genes of other processes or pathways, it seems that genes associated with transcription and translation are less variable under different conditions and are more suited as HKGs. These results are in accordance with the findings of Kouadjo et al. (2007) and de Jonge et al. (2007), who reported the protein biosynthesis–related genes as the most stably expressed genes. Gene ontology terms as well as KEGG pathways enriched by the top 10 most stably expressed genes are reported in Supplementary Table S4.
Consistency of the results of the training datasets
As mentioned above, other sets of data of heart (three datasets), kidney (three datasets), liver (six datasets), muscle (six datasets), and spleen (six datasets) tissues were analyzed in the same way as the training datasets in order to evaluate the consistency of the results of the training datasets. The average CVs of the training datasets strongly correlated with that of the evaluation datasets with Pearson correlation coefficient ranging from 0.64 to 0.88 and Spearman correlation coefficients ranging from 0.67 to 0.82, indicating the relatively high accuracy of the discovering stable genes. The top 100 genes of the training datasets were compared with that of the evaluation datasets. We found that the top 100 genes of the training datasets also took place in higher ranks in the evaluation datasets. Except for muscle, we found relatively consistent results for the remaining four tissues. There were 6, 13, 14, 23, and 32 genes in common in the top 100 genes of the training and evaluation datasets of muscle, spleen, liver, heart, and kidney tissues, respectively. In total, 80% of the top 100 genes for kidney tissue and almost 50% of the top 100 genes for heart, liver, and spleen tissues were present in the top 500 genes of the evaluation datasets. It should be noted that in the case of random distribution of genes in the various ranks of the evaluation datasets, less than 10% of the top 100 genes of training datasets would be present in the top 500 genes of the evaluation datasets [(500/number of analyzed genes) × 100 = ∼4.6–8%]. These findings indicate that the identification of the most stably expressed genes with the used method is, to some extent, accurate, and the repeatability of the results is considerable. In Table 3, the number of common genes in the top 100 genes of the training datasets and top 500 genes of the evaluation datasets are presented. Although the employed approach identified reliable, stable reference genes for all tissues, we recommend the utilization of the reported HKGs cautiously. In addition, we invite related researchers to further validate the reported HKGs using real-time PCR as this was not possible in the current work.

TABLE 3. Number of genes that are in common in the top 100 genes list of the training datasets and the top 100 to top 500 genes lists of the evaluation datasets.
Here, we introduced only those genes that were in common between the top 100 genes of the training and evaluation datasets as V-HKGs. Six of the V-HKGs, namely, Ankrd16, Strada, Phc1, Atl1, Mkrn2, and Uck1, were observed commonly for at least two tissues. Ankrd16 gene was identified as suitable for heart, liver, and spleen tissues. The remaining five genes were identified as the best for both heart and kidney tissues. In Table 4, the official names of the V-HKGs are reported. In addition, in Figure 3, the boxplots of the V-HKGs are illustrated. As can be seen in Figure 3, the expression variations of the V-HKGs were negligible.
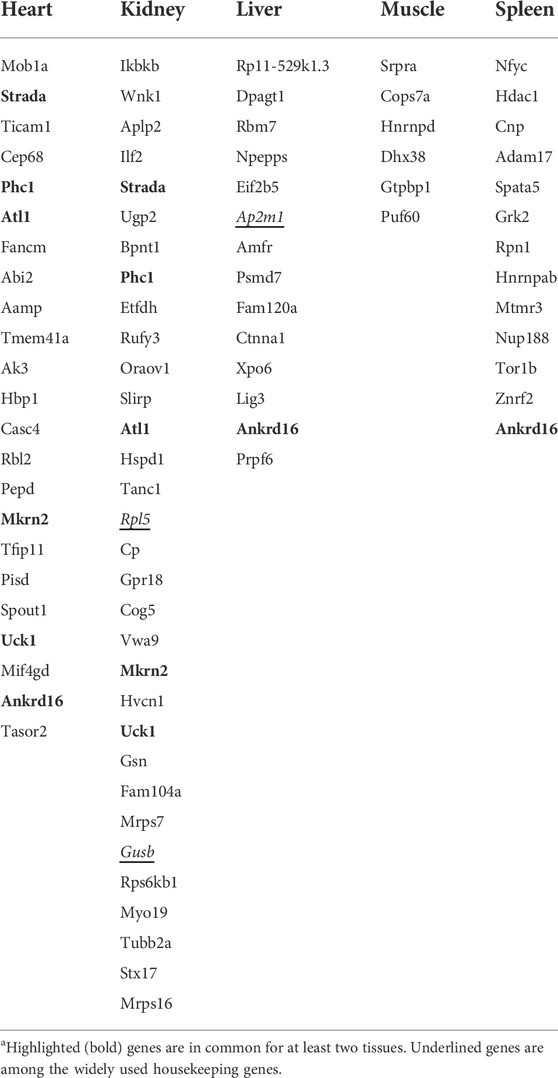
TABLE 4. Most stably expressed genes of five chicken tissues that are in common between the top 100 genes of the training dataset and the top 100 genes of the evaluation dataset.
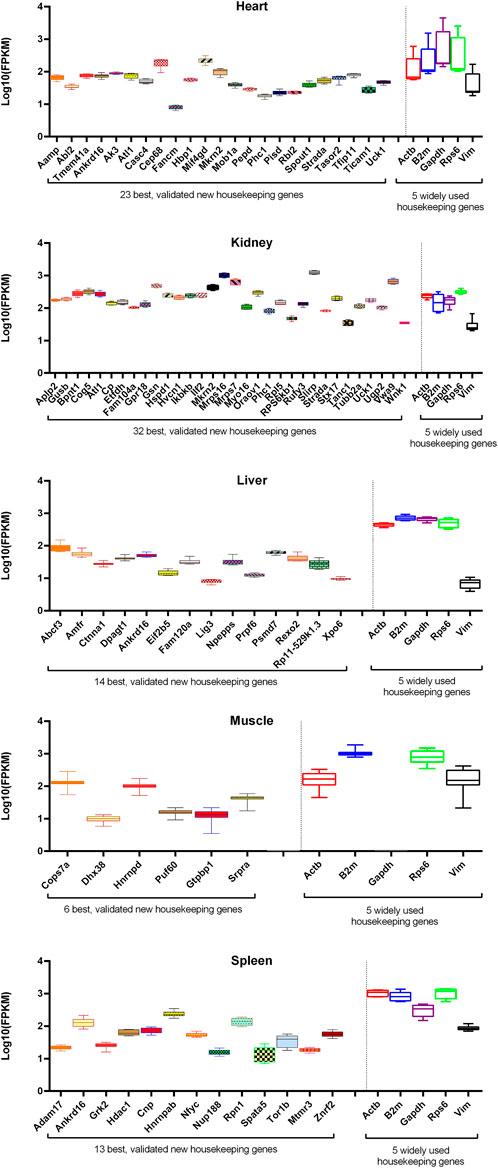
FIGURE 3. Box plots of the expression of most stable, validated housekeeping genes in comparison with that of five randomly selected widely used housekeeping genes of five chicken tissues. For each tissue, the expression variation of newly introduced housekeeping genes (left side of the vertical line) was compared with that of five old and widely used housekeeping genes (right side of the vertical line). The expression values are given as fragments per kilobase of transcript per million reads in log10 scale (whiskers: min to max).
Assessing the suitability of 96 widely used housekeeping genes
In Supplementary Tables S5 and S6, the average CVs and rankings of the WU-HKGs among the REGs are reported, respectively. The expression profile of most of the 96 WU-HKGs showed inconsistency in some of the chicken tissues. Eight genes (i.e., Mb, Dimt1, Rps29, Stx5, Gys1, Il6, Rbx1, and Rnasek) showed no expression in more than five tissues. It is obvious that the mentioned genes do not have merit to be suggested as proper HKGs, although some of them have been traditionally used widely for the normalization of real-time PCR assays. In contrast, only 13 genes showed consistent expression in all 16 tissues, including Ap2m1, Gusb, Polr2b, Rpl6, Eif4a3, Rpl4, Eef1a1, Gapdh, Rpl19, Rpl27a, Rpl31, and Rps6. Six out of the 13 mentioned genes (i.e., Eef1a1, Gapdh, Rpl19, Rpl27a, Rpl31, and Rps6) showed relatively consistent, stable expression and ranked among the best 100 most stably expressed genes of two tissues. Although not detected in three datasets, Ap2m1 performed well in seven tissues, which was followed by Gusb, Polr2b, Rpl6, Rpl4, and Eif4a3. These WU-HKGs were high-ranked in three or more tissues and seem to be suitable for further utilization.
For blood, 17 WU-HKGs were relatively suitable, which was followed by adipose (seven genes), jejunum (six genes), kidney (six genes), and liver (five genes), indicating that the WU-HKGs are more suitable for blood than for other tissues. For the remaining tissues, almost none of the WU-HKGs is suitable, and further use of them is not suggested.
The variation of expression of the 96 WU-HKGs were considerably more than that of the stable reference genes that were introduced in the present work. In Figure 3, the expression variations of five randomly selected WU-HKGs (i.e., Gapdh, Actb, Vim, Rps6, and B2m) are illustrated. As can be seen in Figure 3, some of the mentioned genes were not stably expressed across all experiments of 16 tissues. Two of them (i.e., Gapdh and Rps6) were among the top 100 stable genes, while the remaining three (i.e., Vim, B2m, and Actb) were not.
Previous studies have reported relatively high variability for the expression of WU-HKGs in a varied range of tissues and organs in livestock animals (Gromboni et al., 2020; Lozano-Villegas et al., 2021) as well as in humans (Mahoney et al., 2004; Caracausi et al., 2017; Cadenas et al., 2022), mice (Fu et al., 2020; Muñoz et al., 2021), and insects (Shi et al., 2016), among others.
For adipose tissue, only Rpl4 gene took place within the top 10 stable genes among 10,343 REGs (Table 2). Other WU-HKGs were not even within the best 100 genes. We found it interesting that having fold changes of ∼9.7 and 12.0, respectively, Acta1 and Scd genes showed significant difference between the case and control groups in one experiment of adipose (q-value < 0.05). Therefore, these genes are no longer suggested for further utilization. Unlike Rpl4 gene, which is also proposed here, Tbp has previously been reported elsewhere as a suitable reference gene for the normalization of gene expression data of adipose tissue (Wang et al., 2020). Tbp was the only WU-HKG that was reliably expressed in all 16 tissues with nonsignificant differential expression in all of the 94 studied datasets. However, Tbp ranked 1,092 among the 10,343 REGs of adipose tissue. Other studies have reported Rpl32 and B2m genes as two suitable HKGs for abdominal fat, compared with the other three genes, namely, Sdha, Tbp, and Ywhaz (Bagés et al., 2015). Neither of them, however, were identified as suitable HKGs for adipose in the current work. Na et al. (2021) compared 14 chicken reference genes and reported both Tbp and Hmbs genes as the most stably expressed genes during the growth and development of abdominal adipose tissue of broilers. They also reported Tbp and Rpl13 genes as the most stable during the differentiation of primary preadipocytes and Tbp and Hmbs genes in preadipocytes and mature adipocytes.
In the present work, as compared to those of other tissues, greater number of WU-HKGs were relatively constant across all datasets of blood. Studying human peripheral blood, Martínez-Sánchez et al. (2019) found Hprt and Tbp as the most reliable genes. They suggested the utilization of Gapdh, B2m, and Rpl13a genes to be avoided. Likewise, Dheda et al. (2004) emphasized the avoidance of employment of Gapdh, B2m, and Actb genes (each with ∼10- to 30-fold variability across conditions) for normalizing mRNA levels in human pulmonary tuberculosis. For blood, we found the following four WU-HKGs as relatively stable: Gapdh (rank = 2), Rpl13 (rank = 80), Oaz1 (rank = 3), and Rpl27a (rank = 6).
For heart tissue, except Gusb (rank = 50) and Tbp (rank = 57) genes, further utilization of other WU-HKGs genes is not suggested. TBP has been also identified as a suitable reference gene for lung and heart (Hassanpour et al., 2018) and abdominal fat (Wang et al., 2020) tissues. Because of the low coverage of the used datasets of heart tissue in the current work, only 3,334 (6,235) genes in the training (evaluation) datasets were reliably expressed. Therefore, as expected, many of the WU-HKGs with little expression were not detected in heart datasets. On that account, only 33 WU-HKGs were analyzed. Gromboni et al. (2020) assessed the suitability of eight HKGs for heart. Their studied WU-HKGs were present in neither the top 10 genes nor the list of 23 V-HKGs of heart tissue in the current study. Hassanpour et al. (2018) investigated a panel of nine HKGs and introduced Ywhaz and Rpl13 genes as suitable for chicken heart (Hassanpour et al., 2018). All of their studied genes were filtered out in the current study as lacking the criteria we employed to categorize the stable reference genes.
For kidney tissue, only Polr2b and Gusb genes (rankings of 3 and 5, respectively) and Eef1a1, Nelfcd, Rpl5, and Rpl6 (rankings of less than 100) outperformed other WU-HKGs within 7,527 REGs. On the contrary, Il6, Scd, and Dimt1 were among the worst genes and identified as the most inappropriate HKGs for kidney. Other WU-HKGs were also not stable and therefore not recommended for further use.
For liver tissue, only Ap2m1 gene appeared suitable with a ranking of 5 among 8,428 REGs. Out of 94 analyzed datasets, Ap2m1 was reliably expressed in 80, while it was significant (q-value < 0.05) in only two datasets belonging to blood and brain tissues. Therefore, although not suggested for brain, blood, heart, and lung tissues, Ap2m1 seems to be suggested for more than one tissue. In yellow feathered broilers, Zhang et al. (2018) reported Rpl13 gene as the most proper HKG for liver, compared with only six other candidate genes. In another research, Ywhaz and Tbp were found more stable than B2m, Rpl32, and Sdha genes (Bagés et al., 2015). In the present work, being significant in one, two, two, and three experiments among 12 experiments of liver, Actb, B2m, Gapdh, and Rpl13 genes, respectively, were not proved to be suitable HKGs.
For lung tissue, only 34 WU-HKGs showed reliable expression. Almost 95% of these 34 genes were not stable. Only Gusb (rank 30 among 3,562 REGs) was identified as relatively suitable. In an attempt, Kriegova et al. (2008) investigated 10 candidate HKGs and introduced Rpl32 as the most suitable HKG for lung tissue. Being excluded from the analyses in the filtration steps, Rpl32 was not identified as a suitable HKG in the current work for neither lung tissue nor other tissues. The results of Fu et al. (2020) indicated that none of the 15 WU-HKGs that they studied were sufficiently good as reference genes. However, they suggested the combination of Grcc10 and Ppia genes as a proper choice for the lung tissue of mouse infected with IAV.
The datasets of muscle in the current work appeared very variable. Although we only used datasets belonging to pectoral major muscle tissue, as compared to other tissues, there were less genes in common between the training and evaluation datasets. It seems that sampling of the tissues of the different studies had been done differently and not from the same section of the pectoral major muscle. The six identified V-HKGs (i.e., Srpra, Cops7a, Hnrnpd, Dhx38, Gtpbp1, and Puf60), however, showed sufficiently less variability within the used 12 different datasets and seem to be a suitable set of reference genes for muscle. The findings of Nascimento et al. (2015) showed Hmbs and Hprt1 genes as the most stable while Tfrc and B2m as the least stable reference genes for the pectoralis major muscle of chicken. Their results, also, revealed that Hmbs and Hprt1 gene expression did not change owing to dietary variations and thus were recommended for accurate normalization of RT-qPCR data of chicken pectoralis major muscle. In our results, the best WU-HKG was MIF (rank = 89). Hprt1, although expressed nonsignificantly in all six training analyses of pectoral muscle and its fold change ranged 1.0–1.3 between the case and control groups, appeared relatively variable within the case or control groups of the evaluation datasets and ranked 1,172 among 10,745 REGs. In accordance with the findings of the current work, Barber et al. (2005) analyzed Gapdh expression in a panel of 72 human tissues and observed a 15-fold difference in Gapdh mRNA copy numbers between the skeletal muscle and the breast. Their results confirmed previous reports of the marked variability of Gapdh expression between tissue types. On the contrary, Mahoney et al. (2004) concluded that B2M and ACTB were the most stably expressed HKGs in human skeletal muscle following resistance exercise, while B2m and Gapdh were the most stable following endurance exercise.
For ovary, we discovered three genes (i.e., Tasor2, Ctnna1, and Gsr) within its top 10 genes that were also identified as the most stable for other tissues. These stable genes along with all the top 10 genes of brain, bursa of Fabricius, duodenum, ileum, jejunum, lung, skin, and trachea were completely new and, to our knowledge, are first reported in the present study. Hassanpour et al. (2019) reported Ywhaz, Hprt1, and Hmbs genes as most stable. They suggested the combination of Ywhaz, Hprt1, and Hmbs as the best set of reference genes for ovarian and uterine tissues of laying hens under control and heat stress conditions. Cadenas et al. (2022) found that the stability of all reference genes differs among ovarian cell types in humans. They identified Actb as the best reference gene for oocytes and cumulus cells and B2m for medulla tissue and isolated follicles. They concluded that using a single validated reference gene may be sufficient when the available testing material is limited. For the ovarian cortex, depending on culture conditions, Gapdh or Actb were found to be the most stably expressed genes. Their reported stable genes were not confirmed in the current work.
Similar to the tissues discussed above, we evaluated the 96 HKGs for spleen transcriptome data as well. Having a relatively low CV and high rank (58 among 9,904 genes), only Rpl6 proved to be relatively suitable for spleen. In a previous study, 10 HKGs were assessed and 2 genes, i.e., Tbp and Ywhaz, were identified as the most suitable HKGs for spleen tissue (Khan et al., 2017). None of them, however, was in the list of best, high-ranked HKGs in the current work. For spleen, liver, and cecum of different-aged specific-pathogen-free layer chickens and commercial turkeys, Mitra et al. (2016) suggested Rpl13 and Tbp as the most stable reference genes. They also observed a stable expression of Rpl13 and Tfrc genes in the mentioned tissue samples of turkey. In the current work, both Rpl13 and Tfrc genes were expressed differently between the case and control groups of two experiments of evaluation datasets (q-value < 0.05). Therefore, our results did not prove the consistency of expression of Rpl13 and Tfrc genes. Likewise, we could not approve the suitability of spleen HKGs introduced by Khan et al. (2017) and Borowska et al. (2016).
To our knowledge, the present work is the first comprehensive study that investigated all REGs for 16 most important chicken tissues. Most of the previous studies have compared only a handful of HKGs in which the used genes were in common (Zinzow-Kramer et al., 2014; Bagés et al., 2015; Mitra et al., 2016; Khan et al., 2017; Hassanpour et al., 2018; Zhang et al., 2018; Gromboni et al., 2020). The employed methodologies of the mentioned studies were NormFinder, GeNorm, BestKeeper, RefFinder, and delta CT (Mitra et al., 2016). Each of them has its own strengths and weaknesses. In RefFinder, PCR efficiencies are not taken into account. The NormFinder software is influenced by sample size (Spiegelaere et al., 2015). GeNorm ranking for genes is based on the highest degree of similarity in their expression profile and does not take the amount of variation into account (Andersen et al., 2004). BestKeeper utilizes Pearson correlation analysis and is just valid for normally distributed data with a homogeneous variance. In general, the ranking of HKGs is different based on the output of RefFinder, NormFinder, GeNorm, and BestKeeper, and there is little overlap (Kou et al., 2017). Owing to the dynamic and high-throughput nature of the next-generation sequencing data, the methodology that was introduced in the current work seems to overcome the weakness of the previously used methods. Moreover, utilization of different datasets that belong to different studies, instead of real-time PCR data, is the superiority of the current work over the previous research. In addition, the integration of the results of at least three datasets per tissue seems to increase the reliability of the results.
Conclusion
In the present work, we, for the first time, conducted a comprehensive genome-wide gene expression evaluation of 3,000–11,000 genes, analyzing 94 experiments in order to assess the suitability of previously known HKGs as well as to discover the most stable, new housekeeping genes for each of 16 chicken tissues. The results clearly revealed novel reference genes with more stable expressions in different experimental conditions. On the basis of the definition of ubiquitous and stable expression, our results suggest that no single gene qualifies as a real HKG. In addition, although we identified some genes that were suited for more than one tissue, most of the introduced new and validated HKGs were tissue specific. Thus, instead of one suitable HKG, we reported 10 high-ranked, stable genes for each tissue to provide future studies with more options to choose from. The identified new HKGs were predominantly involved in transcription, translation, and protein biosynthesis. There were 17 common HKGs that were suitable for more than one tissue. We strongly suggest them as well as the V-HKGs for normalization in all future qRT-PCR experiments. We believe that the results of the present work will contribute to more accurate normalization of chicken gene expression data, especially for the data of heart, liver, kidney, spleen, and muscle tissues, and that their results will be validated by analyzing additional sets of datasets.
Data availability statement
The original contributions presented in the study are included in the article/Supplementary Material, and further inquiries can be directed to the corresponding author.
Ethics statement
Ethical review and approval were not required for the animal study because we used the publicly available RNA-seq datasets and we did not collect the data ourselves.
Author contributions
KH: research concept and design, data analysis and interpretation, wrote the article, and final approval of the article. AM and SH: data analysis and wrote the article. SA: critical revision of the article and final approval of the article.
Conflict of interest
The authors declare that the research was conducted in the absence of any commercial or financial relationships that could be construed as a potential conflict of interest.
Publisher’s note
All claims expressed in this article are solely those of the authors and do not necessarily represent those of their affiliated organizations, or those of the publisher, the editors, and the reviewers. Any product that may be evaluated in this article, or claim that may be made by its manufacturer, is not guaranteed or endorsed by the publisher.
Supplementary material
The Supplementary Material for this article can be found online at: https://www.frontiersin.org/articles/10.3389/fgene.2022.827538/full#supplementary-material
References
Andersen, C. L., Jensen, J. L., and Ørntoft, T. F. (2004). Normalization of real-time quantitative reverse transcription-PCR data: A model-based variance estimation approach to identify genes suited for normalization, applied to bladder and colon cancer data sets. Cancer Res. 64, 5245–5250. doi:10.1158/0008-5472.CAN-04-0496
Andrews, S. (2010). FastQC: a quality control tool for high throughput sequence data. Available at: http://www.bioinformatics.babraham.ac.uk/projects/fastqc/.
Bagés, S., Estany, J., Tor, M., and Pena, R. N. (2015). Investigating reference genes for quantitative real-time PCR analysis across four chicken tissues. Gene 561, 82–87. doi:10.1016/j.gene.2015.02.016
Barber, R. D., Harmer, D. W., Coleman, R. A., and Clark, B. J. (2005). GAPDH as a housekeeping gene: Analysis of GAPDH mRNA expression in a panel of 72 human tissues. Physiol. Genomics 21, 389–395. doi:10.1152/physiolgenomics.00025.2005
Bolger, A. M., Lohse, M., and Usadel, B. (2014). Trimmomatic: A flexible trimmer for Illumina sequence data. Bioinformatics 30, 2114–2120. doi:10.1093/bioinformatics/btu170
Borowska, D., Rothwell, L., Bailey, R. A., Watson, K., and Kaiser, P. (2016). Identification of stable reference genes for quantitative PCR in cells derived from chicken lymphoid organs. Vet. Immunol. Immunopathol. 170, 20–24. doi:10.1016/j.vetimm.2016.01.001
Cadenas, J., Pors, S. E., Nikiforov, D., Zheng, M., Subiran, C., Bøtkjær, J. A., et al. (2022). Validating reference gene expression stability in human ovarian follicles, oocytes, cumulus cells, ovarian medulla, and ovarian cortex tissue. Int. J. Mol. Sci. 23, 886. doi:10.3390/ijms23020886
Caracausi, M., Piovesan, A., Antonaros, F., Strippoli, P., Vitale, L., and Pelleri, M. C. (2017). Systematic identification of human housekeeping genes possibly useful as references in gene expression studies. Mol. Med. Rep. 16, 2397–2410. doi:10.3892/mmr.2017.6944
Carmona, R., Arroyo, M., José, M., Quesada, J., Seoane, P., Zafra, A., et al. (2017). Automated identification of reference genes based on RNA - seq data. Biomed. Eng. Online 16, 65–33. doi:10.1186/s12938-017-0356-5
de Jonge, H. J. M., Fehrmann, R. S. N., de Bont, E. S. J. M., Hofstra, R. M. W., Gerbens, F., Kamps, W. A., et al. (2007). Evidence based selection of housekeeping genes. PLoS ONE 2, e898–5. doi:10.1371/journal.pone.0000898
Dheda, K., Huggett, J. F., Bustin, S. A., Johnson, M. A., Rook, G., and Zumla, A. (2004). Validation of housekeeping genes for normalizing RNA expression in real-time PCR. Biotechniques 37, 112–114. doi:10.2144/04371RR03
Eisenberg, E., and Levanon, E. Y. (2013). Human housekeeping genes, revisited. Trends Genet. 29, 569–574. doi:10.1016/j.tig.2013.05.010
Fu, Y., Yang, J., Fan, S., Zhao, S., Shah, S. M. A., Akram, M., et al. (2020). A novel strategy facilitates reference gene selection by RT-qPCR analysis in kidney yang deficiency syndrome mice infected with the influenza A (H1N1) virus. Biomed. Res. Int. 2020, 9075165. doi:10.1155/2020/9075165
González-agüero, M., García-rojas, M., Di Genova, A., Correa, J., Maass, A., Orellana, A., et al. (2013). Identification of two putative reference genes from grapevine suitable for gene expression analysis in berry and related tissues derived from RNA-Seq data. BMC Genomics 14, 878. doi:10.1186/1471-2164-14-878
Gromboni, J. G. G., de Oliveira, H. C., Marques, D. B. D., Junior, A. A. P. G., Filho, R. V. F., Gromboni, C. F., et al. (2020). Influence of heat stress on reference genes stability in heart and liver of two chickens genotypes. PLoS ONE 15, e0228314–15. doi:10.1371/journal.pone.0228314
Hassanpour, H., Bahadoran, S., Farhadfar, F., Chamali, Z. F., Nazari, H., and Kaewduangta, W. (2018). Identification of reliable reference genes for quantitative real-time PCR in lung and heart of pulmonary hypertensive chickens. Poult. Sci. 97, 4048–4056. doi:10.3382/ps/pey258
Hassanpour, H., Aghajani, Z., Bahadoran, S., Farhadi, N., Nazari, H., and Kaewduangta, W. (2019). Identification of reliable reference genes for quantitative real-time PCR in ovary and uterus of laying hens under heat stress. Stress 22, 387–394. doi:10.1080/10253890.2019.1574294
Jiao, X., Sherman, B. T., Huang, D. W., Stephens, R., Baseler, M. W., Lane, H. C., et al. (2012). DAVID-WS: A stateful web service to facilitate gene/protein list analysis. Bioinformatics 28, 1805–1806. doi:10.1093/bioinformatics/bts251
Khan, S., Roberts, J., and Wu, S. B. (2017). Reference gene selection for gene expression study in shell gland and spleen of laying hens challenged with infectious bronchitis virus. Sci. Rep. 7, 14271–14312. doi:10.1038/s41598-017-14693-2
Kim, D., Paggi, J. M., Park, C., Bennett, C., and Salzberg, S. L. (2019). Graph-based genome alignment and genotyping with HISAT2 and HISAT-genotype. Nat. Biotechnol. 37, 907–915. doi:10.1038/s41587-019-0201-4
Kou, X., Zhang, L., Yang, S., Li, G., and Ye, J. (2017). Selection and validation of reference genes for quantitative RT-PCR analysis in peach fruit under different experimental conditions. Sci. Hortic. 225, 195–203. doi:10.1016/j.scienta.2017.07.004
Kouadjo, K. E., Nishida, Y., Cadrin-Girard, J. F., Yoshioka, M., and St-Amand, J. (2007). Housekeeping and tissue-specific genes in mouse tissues. BMC Genomics 8, 127. doi:10.1186/1471-2164-8-127
Kovaka, S., Zimin, A. V., Pertea, G. M., Razaghi, R., Salzberg, S. L., and Pertea, M. (2019). Transcriptome assembly from long-read RNA-seq alignments with StringTie2. Genome Biol. 20, 278. doi:10.1186/s13059-019-1910-1
Kriegova, E., Arakelyan, A., Fillerova, R., Zatloukal, J., Mrazek, F., Navratilova, Z., et al. (2008). PSMB2 and RPL32 are suitable denominators to normalize gene expression profiles in bronchoalveolar cells. BMC Mol. Biol. 9, 69–14. doi:10.1186/1471-2199-9-69
Lozano-Villegas, K. J., Rodríguez-Hernández, R., Herrera-Sánchez, M. P., Uribe-García, H. F., Naranjo-Gómez, J. S., Otero-Arroyo, R. J., et al. (2021). Identification of reference genes for expression studies in the whole-blood from three cattle breeds under two states of livestock weather safety. Animals 11, 3073. doi:10.3390/ani11113073
Mahoney, D. J., Carey, K., Fu, M. H., Snow, R., Cameron-Smith, D., Parise, G., et al. (2004). Real-time RT-PCR analysis of housekeeping genes in human skeletal muscle following acute exercise. Physiol. Genomics 18, 226–231. doi:10.1152/physiolgenomics.00067.2004
Martínez-Sánchez, M., Hernandez-Monge, J., Moctezuma-Dávila, M., Rangel-Charqueño, M., and Olivares-Illana, V. (2019). Analysis of housekeeping genes in the peripheral blood of retinoblastoma patients. bioRxiv. doi:10.1101/693101
Mitra, T., Bilic, I., Hess, M., and Liebhart, D. (2016). The 60S ribosomal protein L13 is the most preferable reference gene to investigate gene expression in selected organs from turkeys and chickens, in context of different infection models. Vet. Res. 47, 105–109. doi:10.1186/s13567-016-0388-z
Muñoz, J. J., Anauate, A. C., Amaral, A. G., Ferreira, F. M., Watanabe, E. H., Meca, R., et al. (2021). Ppia is the most stable housekeeping gene for qRT-PCR normalization in kidneys of three Pkd1-deficient mouse models. Sci. Rep. 11, 19798–19821. doi:10.1038/s41598-021-99366-x
Na, W., Wang, Y., Gong, P., Zhang, X., Zhang, K., Zhang, H., et al. (2021). Screening of reference genes for RT-qPCR in chicken adipose tissue and adipocytes. Front. Physiol. 12, 676864–676913. doi:10.3389/fphys.2021.676864
Nascimento, C. S., Barbosa, L. T., Brito, C., Fernandes, R. P. M., Mann, R. S., Pinto, A. P. G., et al. (2015). Identification of suitable reference genes for real time quantitative polymerase chain reaction assays on Pectoralis major muscle in chicken (Gallus gallus). PLoS ONE 10, e0127935–15. doi:10.1371/journal.pone.0127935
Olias, P., Adam, I., Meyer, A., Scharff, C., and Gruber, A. D. (2014). Reference genes for quantitative gene expression studies in multiple avian species. PLoS ONE 9, e99678. doi:10.1371/journal.pone.0099678
Oliveira, H. C. De, Garcia, A. A. P., Gromboni, J. G. G., Filho, R. V. F., Nascimento, C. S. Do, and Wenceslau, A. A. (2017). Influence of heat stress, sex and genetic groups on reference genes stability in muscle tissue of chicken. PLoS ONE 12, e0176402–15. doi:10.1371/journal.pone.0176402
Pashay Ahi, E., Guobrandsson, J., Kapralova, K. H., Snorrason, S. S., and Maier, V. H. (2013). Validation of reference genes for expression studies during craniofacial development in arctic charr. PLos One 8, e66389. doi:10.1371/journal.pone.0066389
Pfaffl, M. W., Tichopad, A., Prgomet, C., and Neuvians, T. P. (2004). Determination of stable housekeeping genes, differentially regulated target genes and sample integrity: BestKeeper - excel-based tool using pair-wise correlations. Biotechnol. Lett. 26, 509–515. doi:10.1023/B:BILE.0000019559.84305.47
Shi, C., Yang, F., Zhu, X., Du, E., Yang, Y., Wang, S., et al. (2016). Evaluation of housekeeping genes for quantitative real-time PCR analysis of bradysia odoriphaga (Diptera: Sciaridae). Int. J. Mol. Sci. 17, E1034. doi:10.3390/ijms17071034
Spiegelaere, W. De, Dern-Wieloch, J., Weigel, R., Schumacher, V., Schorle, H., Nettersheim, D., et al. (2015). Reference gene validation for RT-qPCR, a note on different available software packages. PLoS ONE 10, e0122515–13. doi:10.1371/journal.pone.0122515
Su, Y., He, W., Wang, J., Li, J., Liu, S., Wang, X., et al. (2013). Selection of endogenous reference genes for gene expression analysis in the mediterranean species of the bemisia tabaci ( Hemiptera : Aleyrodidae ) complex. J. Econ. Entomol. 106, 1446–55. doi:10.1603/EC12459
Trapnell, C., Williams, B. A., Pertea, G., Mortazavi, A., Kwan, G., van Baren, M. J., et al. (2010). Transcript assembly and quantification by RNA-Seq reveals unannotated transcripts and isoform switching during cell differentiation. Nat. Biotechnol. 28, 511–515. doi:10.1038/nbt.1621
Vandesompele, J., De Preter, K., Pattyn, F., Poppe, B., Van Roy, N., De Paepe, A., et al. (2002). Accurate normalization of real-time quantitative RT-PCR data by geometric averaging of multiple internal control genes. Genome Biol. 3, RESEARCH0034. doi:10.1186/gb-2002-3-7-research0034
VanGuilder, H. D., Vrana, K. E., and Freeman, W. M. (2008). Twenty-five years of quantitative PCR for gene expression analysis. BioTechniques 44, 619–626. doi:10.2144/000112776
Wang, L., Leng, L., Ding, R., Gong, P., Liu, C., Wang, N., et al. (2020). Integrated transcriptome and proteome analysis reveals potential mechanisms for differential abdominal fat deposition between divergently selected chicken lines. J. Proteomics 241, 1–22. doi:10.21203/rs.3.rs-36558/v1
Yang, F., Lei, X., Rodriguez-Palacios, A., Tang, C., and Yue, H. (2013). Selection of reference genes for quantitative real-time PCR analysis in chicken embryo fibroblasts infected with avian leukosis virus subgroup J. BMC Res. Notes 6, 402. doi:10.1186/1756-0500-6-402
Zemp, N., Minder, A., and Widmer, A. (2014). Identification of internal reference genes for gene expression normalization between the two sexes in dioecious white campion. PLoS One 9, e92893. doi:10.1371/journal.pone.0092893
Zhang, J., Gao, Y. Y., Huang, Y. Q., Fan, Q., Lu, X. T., and Wang, C. K. (2018). Selection of housekeeping genes for quantitative gene expression analysis in yellow-feathered broilers. Italian J. Animal Sci. 17, 540–546. doi:10.1080/1828051X.2017.1365633
Zhuo, B., Emerson, S., Chang, J. H., and Di, Y. (2016). Identifying stably expressed genes from multiple RNA-Seq data sets. PeerJ 4, e2791. doi:10.7717/peerj.2791
Keywords: housekeeping genes, chicken, New Tuxedo, RNA-sequencing, coefficient of variation
Citation: Hasanpur K, Hosseinzadeh S, Mirzaaghayi A and Alijani S (2022) Investigation of chicken housekeeping genes using next-generation sequencing data. Front. Genet. 13:827538. doi: 10.3389/fgene.2022.827538
Received: 02 December 2021; Accepted: 21 July 2022;
Published: 13 September 2022.
Edited by:
Wenfei Jin, University of Science and Technology, ChinaCopyright © 2022 Hasanpur, Hosseinzadeh, Mirzaaghayi and Alijani. This is an open-access article distributed under the terms of the Creative Commons Attribution License (CC BY). The use, distribution or reproduction in other forums is permitted, provided the original author(s) and the copyright owner(s) are credited and that the original publication in this journal is cited, in accordance with accepted academic practice. No use, distribution or reproduction is permitted which does not comply with these terms.
*Correspondence: Karim Hasanpur, karimhasanpur@yahoo.com