- 1Department of Preventive Medicine and Health Education, School of Public Health, Fudan University, Shanghai, China
- 2Institute of Neurology, Huashan Hospital, Fudan University, Shanghai, China
- 3National Clinical Research Center for Aging Diseases, Shanghai, China
Background: Genome-wide association studies have identified many Alzheimer’s disease (AD) genetic-risk single nucleotide polymorphisms (SNPs) and indicated the important role of the cholesterol/lipid metabolism pathway in AD pathogenesis. This study aims to investigate the effects of cholesterol and genetic risk factors on progression of mild cognitive impairment (MCI) to AD.
Methods: We prospectively followed 316 MCI participants aged ≥50 years with a baseline cholesterol profile and SNP genotyping data for 4.5 years on average in a sub-cohort of the Shanghai Aging Study. Total cholesterol, low-density lipoprotein cholesterol (LDL-C), and high-density lipoprotein cholesterol in serum were measured at baseline. SNP genotyping was performed using a MassARRAY system. At follow-up, consensus diagnosis of incident dementia and AD were established based on medical, neurological, and neuropsychological examinations. Cox regression models were used to assess the association of cholesterol and SNP with incident AD.
Results: The AG/AA genotypes of PVRL2 rs6859 were significantly associated with increased incident AD in MCI participants, compared with GG genotype (adjusted hazard ratio [HR] 2.75, 95% confidence interval [CI] 1.32–5.76, p = .007, false discovery rate–adjusted p = .030). In PVRL2 rs6859 AG/AA carriers, each-1 mmol/L higher level of LDL-C was significantly associated with a 48% decreased risk of AD (adjusted HR 0.52, 95%CI 0.33–0.84, p = .007). Consistent results were obtained when using LDL-C as the categorical variable (P for trend = 0.016).
Conclusion: The relationship between LDL-C and progression of MCI may be influenced by genetic variants.
Introduction
Mild cognitive impairment (MCI) is an intermediate stage between normal cognition and Alzheimer’s disease (AD) (Scarabino et al., 2016). Reported annual conversion rates of MCI to AD range from 10% to 15% (Petersen et al., 1999) while the annual incidence of AD in people without dementia is between 2.1% and 3.9% (Ohara et al., 2017; Rajan et al., 2019). Several genetic and environmental risk factors have been demonstrated in the occurrence and progression of AD, such as the presence of the Apolipoprotein E (APOE) ε4 allele (Corder et al., 1993), AD-risk single nucleotide polymorphisms (SNPs) (Lambert et al., 2013), advancing age (Amieva et al., 2004), female sex (Li et al., 2016), low education attainment (Solfrizzi et al., 2004), diabetes mellitus (DM), and hypertension (Kryscio et al., 2013).
Previous epidemiological studies have explored the cholesterol–AD relationship but with inconsistent results, suggesting the cholesterol–cognition association was inconclusive. Some studies have found that a high level of cholesterol, especially low-density lipoprotein cholesterol (LDL-C), was significantly associated with an increased risk of AD (Benn et al., 2017; Schilling et al., 2017). However, insignificant and even opposing associations were concluded from other studies (Li et al., 2005; Reitz et al., 2005; Reitz et al., 2010; Zhou et al., 2018). Our previous studies based on the Shanghai Aging Study found that among older adults without vascular risk factors, TC and LDL-C were inversely associated with incident dementia, LDL-C was inversely associated with incident AD, and incremental TC and LDL-C showed significant correlation with slower annual decline of the MMSE score (Ding et al., 2021).
In recent years, a review of the AD related Genome-wide association study (GWAS) indicated that several AD risk SNP-related genes may cluster in cholesterol and lipid metabolism pathways (Van Cauwenberghe et al., 2016), such as APOE, clusterin (CLU), ATP-binding cassette transporter A7 (ABCA7), and poliovirus receptor–related 2 (PVRL2) (Ma et al., 2018; Mahley and Rall, 2000; Van Cauwenberghe et al., 2016). The Framingham Study explored a significant interaction between the genetic risk score (GRS) based on AD susceptibility loci and triglyceride level, but not cholesterol level, on the risk of AD in a cohort of European descent (Peloso et al., 2018a). However, studies that tested the association of cholesterol, AD-risk genetic variants that associated with lipid/cholesterol metabolism, and progression of MCI to AD (MCI-AD progression) were rarely seen in an older Chinese population. We hypothesize that AD-risk genetic variants that associated with lipid/cholesterol metabolism would influence the effect of cholesterol on MCI-AD progression and aim to test this hypothesis in a prospective, community-based cohort study.
Materials and Methods
Study Population
The Shanghai Aging Study is a longitudinal, community-based cohort study initiated in 2010 in central downtown Shanghai (Ding et al., 2014). We identified 696 individuals with MCI, aged 50 years and over, at baseline and established an MCI sub-cohort. The detailed procedures involved in an MCI diagnosis have been reported elsewhere (Ding et al., 2015).
Ethics Committee Approval
The present study was approved by the Medical Ethics Committee of Huashan Hospital at Fudan University (No. 2009-195) and the Ethics Committee of the Department of Public Health at Fudan University (No. 2018-01-0662), Shanghai, China. All participants, or their legally acceptable representative, provided written informed consent to participate in this study.
Data Collection at Baseline
At baseline, demographic and characteristic data were collected via a face-to-face questionnaire survey, including birth date, gender, height, weight, education years, Mini-Mental State Exam (MMSE) score, Center for Epidemiological Survey-Depression Scale (CES-D) score, tobacco smoking and alcohol drinking (categorized as present or never), medical histories of DM, hypertension, coronary heart diseases (CHDs), and stroke (classified as yes or no). CHDs included coronary artery disease, vascular heart disease, cardiomyopathy, heart failure, and arrhythmias. Detailed procedures of data collection have been reported elsewhere (Ding et al., 2015; Ding et al., 2016). Body mass index (BMI) was calculated as weight in kilograms divided by height in meters squared.
Assessment of Cognitive Function at Follow-Up
Follow-up was conducted until 31 October 2016, with a median follow-up time of 4.5 years (Ding et al., 2018). Research coordinators contacted all participants with MCI and asked for a clinical interview. Those who could not be traced, refused to participate, or were deceased were defined as “lost-to-follow-up.”
At the in-person clinical interview at follow-up, participants (or the proxy) were rated on the Clinical Dementia Rating scale for cognitive complaints (Lim et al., 2007). If a person was diagnosed as having dementia or AD by neurologists at other hospitals, the time and hospital names were recorded. Participants were measured on the Lawton and Brody Activity of Daily Living (ADL) scale, and functionally intact of physical self-maintenance and instrumental activities of daily living were considered for whose ADL score >16 (Lawton and Brody, 1969). Participants with new onset of stroke were examined for their motor responses and reflexes. The onset and subtype of stroke were queried from medical records, and the results of prior computed tomography/magnetic resonance imaging images were recorded. The cognitive function of participants was tested using the same neuropsychological battery that was used at baseline (Ding et al., 2015). The test battery covered the domains of global cognition, executive function, spatial construction function, memory, language, and attention, including 1) MMSE; 2) Conflicting Instructions Task (Go/No Go Task); 3) Stick Test; 4) Modified Common Objects Sorting Test; 5) Auditory Verbal Learning Test; 6) Modified Fuld Object Memory Evaluation; 7) Trail-making tests A and B; and 8) Renminbi (Chinese currency) test. Neuropsychological tests were administered by study psychometrists according to the education level of each participant. Normative data and a detailed description of these tests have been reported elsewhere (Ding et al., 2015). All tests were conducted in Chinese within a 90-min timeframe.
Neurologists and neuropsychologists in our study group reviewed clinical and neuropsychological data and reached a consensus diagnosis of incident AD using the Diagnostic and Statistical Manual of Mental Disorders-IV and the National Institute of Neurological and Communicative Disorders and Stroke and the Alzheimer’s Disease and Related Disorders Association criteria (American Psychiatric Association, 1994). Diagnostic procedures and criteria were the same as those at baseline.
Serological Testing
A baseline blood sample was collected from each participant by research nurses in the morning after 12 h of overnight fasting. Serum cholesterol profiles were measured using a Hitachi 7600 fully automatic biochemical analyzer in the central laboratory of Huashan Hospital. Total cholesterol (TC) was measured using an oxidase method, and LDL-C and high-density lipoprotein cholesterol (HDL-C) were measured using a direct method.
SNP Selection, Genotyping, and Quality Control
For SNP selection, first, we selected the SNPs which were identified in GWAS with an AD-risk association that exceeded the threshold of a genome-wide significance level (p < 5 × 10−8) in European and Asian populations before June 2019; if multiple SNPs are in strong linkage disequilibrium (LD) and met the above criterion, defined by pairwise r2 < 0.2 estimated from the HapMap CHB (Han Chinese in Beijing, China) population, the most commonly cited SNP was selected. Next, the SNP-related genes that were reported to be involved in cholesterol/lipid metabolism pathways in publications were included in this study. Finally, only four SNPs were selected and their information is listed in Table 1. Of these SNPs, although PVRL2 rs6859 is located on chromosome 19, the same as for APOE, there is no strong linkage disequilibrium between PVRL2 rs6859 and APOE (treating ε3 and ε2 as the same allele and ε4 as another allele) with r2 = 0.13 reported in our previous study (Xiao et al., 2015), and r2 = 0.28 in this study.
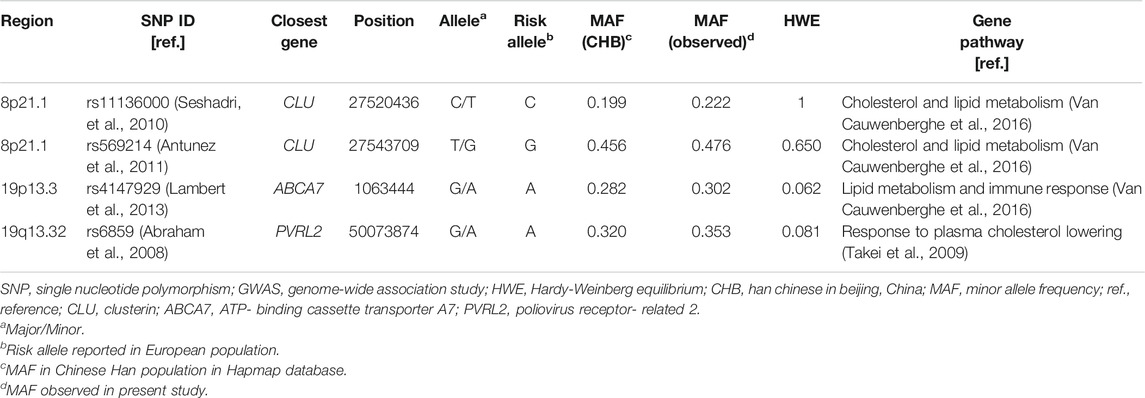
TABLE 1. Selected AD GWAS-SNPs in cholesterol/lipid metabolism–related genes evaluated in this study.
Genomic DNA was extracted from peripheral blood samples at baseline using a QIAamp DNA Blood Mini kit (QIAGEN GmbH, Hilden, Germany). Genotyping of selected SNPs was performed on a MassARRAY system (iPLEX; Sequenom Inc., San Diego, CA, United States) by using an Agena Biosciences (San Diego, CA, United States) iPLEX Gold Genotyping reagent. Four duplicate test samples and four water samples (PCR negative controls) that the technician was blinded to were included in each 384-well plate to monitor genotyping accuracy. The average concordance rate was 100% among these duplicate samples. All assays were conducted by technicians blinded to participant status. SNPs with missing SNPs >1, minor allele frequency (MAF) < 0.01, or p < .001 in a Hardy–Weinberg Equilibrium (HWE) test were removed.
APOE genotype was measured using a TaqMan SNP method (Smirnov et al., 2009). Because there are only three participants carrying APOE ε4/ε4 in our study, the presence of at least one ε4 allele was defined as APOE ε4 positive in the following analysis.
Statistical Analysis
A one-way ANOVA was used for a comparison of continuous variables, and a chi-square test was used for categorical variables. LDL-C values were divided into three levels according to tertile: low (<2.86 mmol/L), medium (2.86–3.67 mmol/L), and high (>3.67 mmol/L). Participants with MCI who had not converted to AD at the last follow-up visit were regarded as censored. The effect of SNPs on MCI-AD progression was assessed using a Cox regression model, adjusted for age and gender in model 1 and additionally adjusted for APOE ε4 status and each genetic variant in model 2. When we examined the effects of baseline cholesterol concentrations on MCI-AD progression, age, gender, APOE ε4 status, and education years were adjusted in Cox regression model 1; BMI, DM, hypertension, CHDs, stroke, smoking, drinking, and lipid-lowering medication were additionally adjusted in model 2. Covariates selected in analyses were based on the criteria of “the variable that is related to AD risk, but not in the causal pathway between cholesterol and AD risk,” and also were referred to relevant literatures (Schilling et al., 2017; Peloso et al., 2018b; Chung et al., 2018). Cumulative incidence graphs of MCI-AD conversion between LDL-C categories were drawn using a Cox regression model, adjusting for age, gender, APOE ε4 status, education years, and lipid-lowering medication, and stratified into different genetic-risk groups. The hazard ratio (HR) and 95% confidence interval (CI) were used as measures to assess the risk effect.
Statistical analyses were performed using PLINK 1.07 software and SPSS 21.0 software. All tests were two-sided and p < .05 was considered as statistically significant. The false discovery rate (FDR), as proposed by Benjamini and Hochberg, was calculated using R software for multiple comparison (Benjamini et al., 2001).
Results
Key Demographic and Clinical Information of Participants
Of 696 MCI participants at baseline, 311 were lost-to-follow-up, 39 lacked baseline blood samples, and 30 were failed in genomic DNA extraction or SNP genotyping. After an average of 4.5 years [standard deviation (SD) 1.3] of follow-up, we diagnosed 58 with incident AD, 11 with other types of dementia, and 247 as non-demented. We therefore included 305 participants with incident AD and non-dementia in our dataset for analysis (Figure 1). The excluded MCI participants were slightly but statistically significantly older (74.7 vs. 72.7, p = .003), with lower MMSE score (p < .001), lower education years (p = .016), and lower BMI level (p = .035), than the included MCI participants in the analysis. There was no significant difference between these two groups in sex, APOE ε4, CES-D score, medical history, cholesterol concentrations at baseline, and smoking and drinking status (Supplementary Table S1).
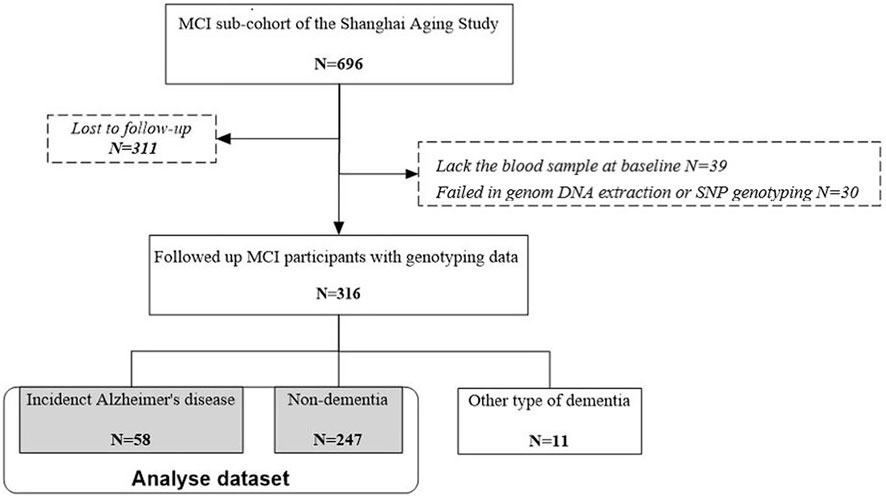
FIGURE 1. Flow chart of participant recruitment for the present study. MCI, mild cognitive impairment.
As shown in Table 2, the mean age of the included 305 MCI participants at baseline was 72.7 (SD 8.0) years, the mean education attainment was 10.5 (SD 4.5) years, and the mean MMSE score was 27.1 (SD 2.5). It was found that 20.9% of MCI participants were APOE ε4 positive. Participants with a medium LDL-C level were older than those with low or high LDL-C (p = .012). Participants with high LDL-C were more likely to be women (p = .023) and to have higher TC (p < .001) and HDL-C (p = .003) levels at baseline. Of the three groups with different LDL-C levels, there was no significant difference in APOE ε4, education year, MMSE score, CES-D score, lipid-lowering medication, BMI, medical history, and smoking and drinking status at baseline, and AD onset during follow-up.
Genotypes and MCI-AD Progression
All four SNPs passed the quality control standards. The associations of genetic variants with MCI-AD progression were analyzed using a Cox regression model adjusted for age and gender in model 1 and additionally adjusted for APOE ε4 status and other three SNPs in model 2. The PVRL2 rs6859 AG, AA, and AG/AA genotypes were significantly associated with increased MCI-AD progression, compared with GG genotype (AG vs. GG, HR (95%CI) = 2.53 (1.14–5.58), p = .022, FDR (P) = .040; AA vs. GG, HR (95%CI) = 3.12 (1.30–7.83), p = .011, FDR (P) = .030; AG/AA vs. GG, HR (95%CI) = 2.75 (1.32–5.76), p = .007, FDR (P) = .030, Table 3, model 2). In APOE ε4 non-carriers, PVRL2 rs6859 still had a significant association with MCI-AD progression adjusting for age and gender (AG/AA vs. GG, HR (95% CI) = 2.23 (1.07–4.65), p = .032); however, PVRL2 rs6859 and APOE had no interactive effect on MCI-AD progression (Pinter rs6859×APOE = 0.567) (Supplementary Table S2). In addition, in our study, APOE ε4 was found to be associated with increased risk of incident AD in univariate analysis (p = .045) but not in multivariate analysis adjusting for age and gender (p = .077), compared to the APOE ε4 non-carriers (Supplementary Table S3). These findings suggested that AG/AA in PVRL2 rs6859 was a genetic risk factor of conversion from MCI to AD.
Cholesterol and MCI-AD Progression
The distributions of cholesterol in different PVRL2 rs6859 genotypes or APOE ε4 status were examined before further analysis, and no significantly different distributions were found (Supplementary Table S4). The association of cholesterol with MCI-AD progression is detailed in Figure 2. Among PVRL2 rs6859 AG/AA carriers, each 1 mmol/L higher level of LDL-C was significantly associated with 48% decreased risk of AD in model 2 (HR (95%CI) = 0.52 (0.33–0.84), p = .007, model 2). However, no significant associations between HDL-C, LDL-C, TC concentration, and MCI-AD progression were found in PVRL2 rs6859 GG carriers. Among total samples, LDL-C (per mmol/L) level was inversely associated with incident AD in Cox regression model 1 (HR = 0.69, 95%CI = 0.47–1.00, p = .048), but the association was no longer significant in model 2 (p = .079). Meanwhile, we examined the association of cholesterol with MCI-AD progression in subgroups stratified by APOE ε4 status and found that LDL-C was also inversely associated with incident AD in APOE ε4 carriers in Cox regression (HR = 0.43, 95%CI = 0.19–0.97, p = .043; Supplementary Figure S1, model 2).
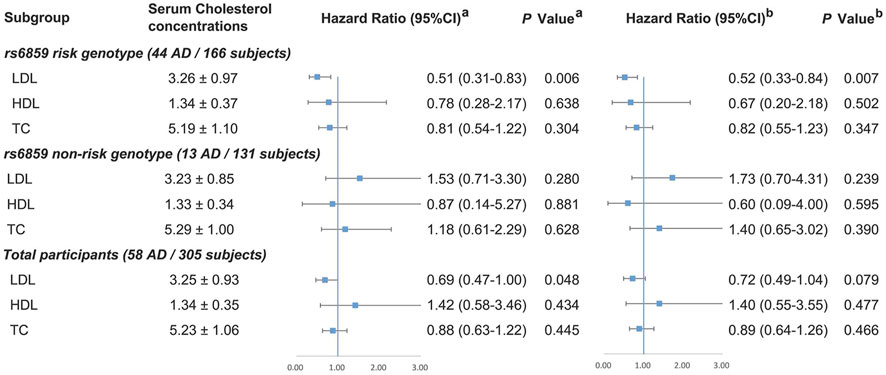
FIGURE 2. Association between cholesterol and MCI-AD progression among total participants and in subgroups stratified by PVRL2 rs6859 genotypes. PVRL2 rs6859 AG/AA was defined as risk genotype and GG was non-risk genotype. a Model 1 was adjusted for age, gender, APOE ε4 status, and education years. b Model 2 was additionally adjusted for vascular risk factors (body mass index, diabetes mellitus, hypertension, coronary heart disease, stroke, smoking, and drinking) and lipid-lowering medication. APOE, Apolipoprotein E; TC, total cholesterol; HDL-C, high-density lipoprotein cholesterol; LDL-C, low-density lipoprotein cholesterol. Note: There were eight missing data of genotyping failing for PVRL2 rs6859.
We further divided the LDL-C concentration into categorical scales by tertile. As shown in Figure 3, among MCI participants with PVRL2 rs6859 AG/AA genotype, those maintaining medium/high LDL-C (2.86-3.67 mmol/L/> 3.67 mmol/L) had a significantly decreased cumulative risk of AD compared with those with low LDL-C (<2.86 mmol/L) (P for trend = .016, Pmedium vs. low = .002, Phigh vs. low = .015; Figure 3A). No significant association between LDL-C and cumulative risk of AD was found in PVRL2 rs6859 GG carriers (Figure 3B). These findings indicated that among MCI with PVRL2 rs6859 AG/AA genotypes, LDL-C was a risk factor of progression from MCI to AD.
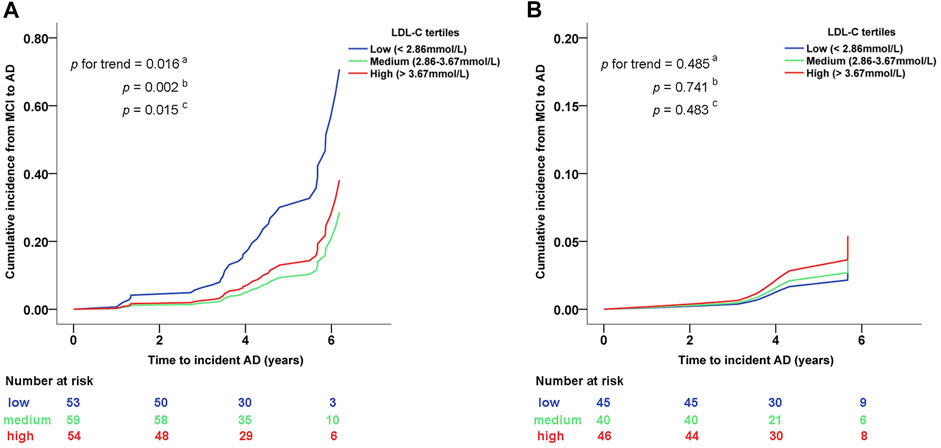
FIGURE 3. Cumulative conversion rate from MCI to AD between different LDL-C category levels in subgroups stratified by PVRL2 rs6859 genotypes. (A) PVRL2 rs6859 AG/AA genotype subgroup. (B) PVRL2 rs6859 GG genotype subgroup. Cumulative incidence graphs are based on age, gender, APOE ε4 status, education years, and lipid-lowering medication adjusted Cox models (from which indicated p values were extracted). a Trend p of cumulative incidence across the three groups of participants with different levels of LDL-C. b medium vs. low LDL-C. c high vs. low LDL-C. AD, Alzheimer’s disease; MCI, mild cognitive impairment; LDL-C, low-density lipoprotein cholesterol.
Discussion
The present community-based prospective study suggests an inverse association between LDL-C at baseline and risk of incident AD in MCI participants with an AD genetic risk factor of PVLR2 rs6859 AG/AA. However, no significant association was found in those with PVRL2 rs6859 GG genotype or total MCI participants. Our results suggest that genetic factors might influence the relationship between LDL-C and risk of incident AD, providing a possible explanation for inconsistent LDL-C-cognition association from previous population-based study (Li et al., 2005; Reitz et al., 2005; Reitz et al., 2010; Benn et al., 2017; Schilling et al., 2017; Zhou et al., 2018) and suggesting that among individuals with PVRL2 rs6859 AG/AA, aggressively lowering LDL-C might not be beneficial to prevent cognition impairment.
The mechanism by which PVRL2 rs6859 influences the relationship between LDL-C and risk of AD remains elusive. PVRL2 rs6859 was previously reported to be associated with AD risk (Hollingworth et al., 2011) and poor cognitive performance (Cruz-Sanabria et al., 2018) and also involved in cholesterol and lipid metabolism. Based on the HaploReg database, (http://pubs.broadinstitute.org/mammals/haploreg/haploreg.php), the rs6859 locates in the 3′UTR region of PVRL2 gene, and the 3′UTR can control gene expression by affecting the localization, stability, and translation of mRNAs (Decker and Parker, 1995). The Genotype-Tissue Expression (GTEx) database (http://www.gtexportal.org/) also shows that the different genotypes of rs6859 were significantly correlated with the expression level of PVRL2 gene in many tissues, including brain hippocampus, whole blood, artery-aorta, artery-tibial tissues, and artery-coronary tissue (Supplementary Figure S2). Specifically, the association of rs6859 genotypes with PVRL2 gene expression remained significant in whole blood, artery-aorta, and artery-tibial tissues even after multiple test correction. Compared to those with GG genotypes, the PVRL2 gene expression level in patients with AG and AA genotype was significantly decreased (Supplementary Figure S3). Furthermore, PVRL2 knockout mice were reported to have less atherosclerosis (Rossignoli, Shang, Gladh, Moessinger, and Foroughi et al., 2017). Importantly, our previous study based on the Shanghai Aging Study found that among older adults without vascular risk factors, LDL-C was inversely associated with incident AD (Ding et al., 2021). All these findings above might provide a reasonable explanation for the results of the present study, that is, older adults with rs6859 AA/AG genotypes might experience decreased expression of PVRL2 in the body and then have less cardiovascular risk. Therefore, among older adults with rs6859 AA/AG genotypes, LDL-C is inversely associated with incident AD.
Importantly, our results showed that PVRL2 rs6859 was significantly associated with MCI-AD progression. Even in APOE ε4 non-carriers, PVRL2 rs6859 still had a significant association with MCI-AD progression (Supplementary Table S2). This finding is particularly important in the assessment of MCI-AD progression. APOE has been irrefutably recognized as the major genetic risk factor for late-onset AD (Chartier-Harlin et al., 1994). Although Asian populations have a lower APOE ε4 frequency (6.9%) (Hu et al., 2011) than Europeans (10–15% in the south to 40–50% in the north) (Ewbank, 2004), the prevalence of cognitive impairment in the Chinese population (Hilal et al., 2013) is still in a similar range to the prevalence reported in Caucasians (Alexander et al., 2015). Therefore, other genetic variants may also contribute to the pathological process of AD and influence the susceptibility of AD in the Chinese population. The evidence for the frequency APOE ε4 in MCI is mostly unexplored. Only a few studies have been conducted with, as yet, small sample sizes (ranging from 28 to 583 participants) and with the APOE ε4 frequency ranging from 7.5% to 39.6% in China, France, Spain/Catalonia, Switzerland, Belgium, and Cuba, as summarized by Tsolak (Tsolaki et al., 2018). MCI participants in our study also showed low prevalence of APOE ε4 carriers (20.9%, 60/290, Table 2) but a high prevalence of PVRL2 rs6859 AG/AA carriers (55.9%, 166/297, Table 3). Even among non-APOE ε4 carriers, 46.2% (102/221) participants carried the PVRL2 rs6859 AG/AA genotype (Supplementary Table S2). Therefore, PVRL2 rs6859 may supplement APOE for better assessing the AD genetic risk in the Chinese population.
Differing from previous prospective cohort studies that examined the cholesterol effect on AD progression from cognitive normal (Ancelin et al., 2013; Reitz et al., 2005; Schilling et al., 2017), we prospectively followed up a cohort of MCI participants and assessed the risk of incident AD. MCI is an intermediate transition stage between cognitive normal and dementia; thus, our study on MCI-AD progression may provide the evidence for the second AD prevention and have the advantage of saving observation time and improving efficiency. Another advantage is that we provided a precise evaluation of AD genetic susceptibility when examining the cholesterol effect on MCI-AD progression, that is, the genetic polymorphisms selected in this study were both identified in an AD GWAS and reported to be involved in cholesterol/lipid metabolism.
PVRL2, also known as NECTIN2, is a cell membrane protein located in the LDL-C GWAS locus APOE. The relationship between PVRL2 and LDL-C level is complicated. Takeiet al. reported that PVRL2 is a cholesterol-responsive gene expressed in many organs, including the brain (Takei et al., 2009). PVRL2 was also found to be markedly downregulated in response to plasma cholesterol lowering in atherosclerosis-prone mice with a humanlike plasma cholesterol profile (Rossignoli et al., 2017). Recently, a Mendelian randomization study reported a negative causal relationship between PVRL2 expression LDL-C uptake in hepatic cells, but a positive causal relationship between PVRL2 expression in liver and LDL-C levels in plasma (van der Graaf et al., 2020). In our study, the LDL-C levels neither differ in different PVRL2 rs6859 genotypes nor in different APOE ε4 status (Supplementary Table S4).
Several longitudinal studies have examined the effects of lipids on AD. However, the results are conflicting (Li et al., 2005; Reitz et al., 2005; Reitz et al., 2010; Ancelin et al., 2013; Proitsi et al., 2014; Benn et al., 2017; Schilling et al., 2017) due to ethics differences, different stages when lipids were measured, onset age, and follow-up duration (Benn et al., 2017; Reitz et al., 2010; Schilling et al., 2017). The Framingham Heart Study (n = 3040) examined the effects of mid-life lipid levels on AD based on AD-risk genetic variants and found that the effect of triglycerides on AD varies according to the sortilin-related receptor 1 (SORL1) SNP rs11218343, but no interaction occurred between AD-risk SNPs and the LDL-C level on the risk of AD. However, this study was conducted in older European adults, aged 65 years and over, and with a 10-year follow-up from cognitive normal to AD (Peloso et al., 2018a). Previous Mendelian randomization studies have explored the causal nature of the relationship between lipids and AD using lipid metabolism–related genetic variants (Benn et al., 2017; Proitsi et al., 2014). One Mendelian randomization study, including participants from the Copenhagen City Heart Study (n = 11,201) and Copenhagen General Population Study (n = 99,993), reported that low LDL-C, due to genes responsible for LDL-C metabolism and biosynthesis, was associated with decreased AD risk in the European population with a median follow-up time of 8.2 years (Benn et al., 2017). However, another Mendelian randomization study, combining British Birth Cohort (n = 9398), Institute of Psychiatry Plus group (n = 663), and Alzheimer’s Disease Neuroimaging Initiative data (n = 517), suggested that lipid-related genetic variants were not causally associated with late-onset AD risk through changes in the LDL-C level in the European population (Proitsi et al., 2014). Our findings offered evidence that lipid metabolism–related SNPs may affect the role of cholesterol in MCI-AD progression in older Chinese people.
In analysis of the cumulative conversion rate from MCI to AD between different LDL-C category levels in high-risk groups stratified by PVRL2 rs6859 genotypes (Table 2), patients with medium LDL-C concentration have the lowest risk of conversion from MCI to AD. LDL-C is a well-established causal risk factor for cardiovascular disease (Silverman et al., 2016), which in turn is recognized as a risk factor of AD. Therefore, the reason for the non–dose-dependent manner in the relationship between LDL-C concentration and the conversion rate may be that excessively low level of LDL-C may partially neutralize the beneficial role of LDL-C for MCI-AD progression through contributing to the risk of cardiovascular disease (de la Torre, 2004; Kelleher and Soiza, 2013).
Limitations should be acknowledged. First, although our study involved a population-based cohort, its sample size was relatively small, which may skew our results to be statistically insignificant. For example, APOE was not observed as a significant risk factor on MCI-AD progression in our study in the multivariate Cox regression model. Second, the mean follow-up time of MCI participants was relatively short. However, as an intermediate state between cognitive normal and AD, MCI has a relative higher conversion rate to AD than a cognitive normal status (18% in our study) within a short follow-up time. Therefore, this average 4.5-year follow-up study with 305 participants was able to achieve a sufficient statistical power in analysis. Third, the Shanghai Aging Study was composed of people with Han Chinese backgrounds, and therefore, these results may not be generalizable to other ethnic populations. Finally, because the excluded participants were older and have a worse baseline cognitive performance than the included participants, the association between LDL-C and the risk of MCI progressing to AD in our study may be underestimated due to the lower follow up rate among very old residents. This should be taken into account when generalizing our results to the general population.
In summary, results from this study suggest that the effect of LDL-C on MCI-AD progression may influenced by genetic variants. Among older people with genetic risk of PVRL2 rs6859 AG/GG, maintaining a moderate and slightly high LDL-C level might be beneficial in preventing AD onset in this subgroup. Larger studies in other ethnic populations with longer follow-up are needed to validate our findings and to explore the potential pathological mechanisms.
Data Availability Statement
Data in the current study are available from the corresponding author on reasonable request and with permission of Huashan Hospital. Additionally, the genotype data of participants can be shared with a signed data confidentiality contract to ensure that the use of the data will meet the requirements of China's Ministry of Science and Technology (MOST) and the data will not be leaked to a third party.
Ethics Statement
The present study was approved by the Medical Ethics Committee of Huashan Hospital at Fudan University (No. 2009-195) and the Ethics Committee of the Department of Public Health at Fudan University (No. 2018-01-0662), Shanghai, China. The patients/participants provided their written informed consent to participate in this study.
Author Contributions
Study conception and design: QX and DD. Clinical diagnosis and interpretation of the data: QZ, LZ, QG, and DD. Acquisition, analysis, or interpretation of data: JX, RW, XL, and WW. Statistical analysis: QX and JX. Manuscript drafting: QX and JX. Critical revision and commentary on manuscript: DD, HF, and ZH. All authors read and approved the final manuscript.
Funding
This work was supported by the grant from the National Natural Science Foundation of China (82173592 and 81773513), the Shanghai Municipal Science and Technology Major Project (2018SHZDZX01), and ZJ LAB, Key Project of the Ministry of Science and Technology, China (2020YFC2005003 and 2021YFE0111800).
Conflict of Interest
The authors declare that the research was conducted in the absence of any commercial or financial relationships that could be construed as a potential conflict of interest.
Publisher’s Note
All claims expressed in this article are solely those of the authors and do not necessarily represent those of their affiliated organizations, or those of the publisher, the editors, and the reviewers. Any product that may be evaluated in this article, or claim that may be made by its manufacturer, is not guaranteed or endorsed by the publisher.
Acknowledgments
The authors thank all the study coordinators, research nurses, psychometrists, and lab technicians for their efforts toward this study and all the participants for their cooperation.
Supplementary Material
The Supplementary Material for this article can be found online at: https://www.frontiersin.org/articles/10.3389/fgene.2022.823406/full#supplementary-material
References
Abraham, R, Moskvina, V, Sims, R, Hollingworth, P, Morgan, A, Georgieva, L, et al. A genome-wide association study for late-onset Alzheimer's disease using DNA pooling. Bmc Med Genomics (2008) 1:44. doi:10.1186/1755-8794-1-44
Alexander, M., Perera, G., Ford, L., Arrighi, H. M., Foskett, N., Debove, C., et al. (2015). Age-Stratified Prevalence of Mild Cognitive Impairment and Dementia in European Populations: A Systematic Review. Jad 48 (2), 355–359. doi:10.3233/JAD-150168
American Psychiatric Association (1994). Diagnostic and Statistical Manual of Mental Disorders. 4th ed., Washington, DC. 143–147.
Amieva, H., Letenneur, L., Dartigues, J.-F., Rouch-Leroyer, I., Sourgen, C., D’Alchée-Birée, F., et al. (2004). Annual Rate and Predictors of Conversion to Dementia in Subjects Presenting Mild Cognitive Impairment Criteria Defined According to a Population-Based Study. Dement Geriatr. Cogn. Disord. 18 (1), 87–93. doi:10.1159/000077815
Ancelin, M.-L., Ripoche, E., Dupuy, A.-M., Barberger-Gateau, P., Auriacombe, S., Rouaud, O., et al. (2013). Sex Differences in the Associations between Lipid Levels and Incident Dementia. Jad 34 (2), 519–528. doi:10.3233/JAD-121228
Antunez, C, Boada, M, Gonzalez-Perez, A, Gayan, J, Ramirez-Lorca, R, Marin, J, et al. The membrane-spanning 4-domains, subfamily A (MS4A) gene cluster contains a common variant associated with Alzheimer’s disease. Genome Med (2011) 3:33. doi:10.1186/gm249
Benjamini, Y., Drai, D., Elmer, G., Kafkafi, N., and Golani, I. (2001). Controlling the False Discovery Rate in Behavior Genetics Research. Behav. Brain Res. 125 (1-2), 279–284. doi:10.1016/s0166-4328(01)00297-2
Benn, M., Nordestgaard, B. G., Frikke-Schmidt, R., and Tybjærg-Hansen, A. (2017). Low LDL cholesterol,PCSK9andHMGCRgenetic Variation, and Risk of Alzheimer's Disease and Parkinson's Disease: Mendelian Randomisation Study. BMJ 357, j1648. doi:10.1136/bmj.j1648
Chartier-Harlin, M. C., Parfitt, M., Legrain, S., Pérez-Tur, J., Brousseau, T., Evans, A., et al. (1994). Apolipoprotein E, Epsilon 4 Allele as a Major Risk Factor for Sporadic Early and Late-Onset Forms of Alzheimer's Disease: Analysis of the 19q13.2 Chromosomal Region. Hum. Mol. Genet. 3 (4), 569–574. doi:10.1093/hmg/3.4.569
Chung, C.-P., Chou, K.-H., Peng, L.-N., Liu, L.-K., Lee, W.-J., Chen, L.-K., et al. (2018). Associations between Low Circulatory Low-Density Lipoprotein Cholesterol Level and Brain Health in Non-stroke Non-demented Subjects. Neuroimage 181, 627–634. doi:10.1016/j.neuroimage.2018.07.049
Corder, E. H., Saunders, A. M., Strittmatter, W. J., Schmechel, D. E., Gaskell, P. C., and Small, G. W. (1993). Gene Dose of Apolipoprotein E Type 4 Allele and the Risk of Alzheimer's Disease in Late Onset Families. Science 261 (5123), 921–923. doi:10.1126/science.8346443
Cruz-Sanabria, F., Bonilla-Vargas, K., Estrada, K., Mancera, O., Vega, E., Guerrero, E., et al. (2021). Análisis de desempeños cognitivos y polimorfismos en SORL, PVRL2, CR1, TOMM40, APOE, PICALM, GWAS_14q, CLU y BIN1 en pacientes con trastorno neurocognitivo leve y en sujetos cognitivamente sanos. Neurologia 36, 681–691. doi:10.1016/j.nrl.2018.07.002
de la Torre, J. C. (2004). Is Alzheimer's Disease a Neurodegenerative or a Vascular Disorder? Data, Dogma, and Dialectics. Lancet Neurol. 3 (3), 184–190. doi:10.1016/S1474-4422(04)00683-0
Decker, C. J., and Parker, R. (1995). Diversity of Cytoplasmic Functions for the 3′ Untranslated Region of Eukaryotic Transcripts. Curr. Opin. Cel Biol. 7 (3), 386–392. doi:10.1016/0955-0674(95)80094-8
Ding, D., Xiong, Y., Zhao, Q., Guo, Q., Chu, S., Chu, W. W. C., et al. (2018). White Matter Hyperintensity Predicts the Risk of Incident Cognitive Decline in Community Dwelling Elderly. Jad 61 (4), 1333–1341. doi:10.3233/JAD-170876
Ding, D., Zhao, Q., Guo, Q., Liang, X., Luo, J., Yu, L., et al. (2016). Progression and Predictors of Mild Cognitive Impairment in Chinese Elderly: A Prospective Follow‐up in the Shanghai Aging Study. Alzheimers Dement (Amst). 4, 28–36. doi:10.1016/j.dadm.2016.03.004
Ding, D., Zhao, Q., Guo, Q., Meng, H., Wang, B., and Luo, J. (2015). Prevalence of Mild Cognitive Impairment in an Urban Community in China: A Cross‐sectional Analysis of the Shanghai Aging Study. Alzheimer's Demen. 11 (3), 300–309. doi:10.1016/j.jalz.2013.11.002
Ding, D., Zhao, Q., Guo, Q., Meng, H., Wang, B., Yu, P., et al. (2014). The Shanghai Aging Study: Study Design, Baseline Characteristics, and Prevalence of Dementia. Neuroepidemiology 43 (2), 114–122. doi:10.1159/000366163
Ding, D., Zhou, F., Cao, Y., Liang, X., Wu, W., Xiao, Z., et al. (2021). Cholesterol Profiles and Incident Cognitive Decline Among Older Adults: the Shanghai Aging Study. Age Ageing 50 (2), 472–479. doi:10.1093/ageing/afaa140
Ewbank, D. C. (2004). The APOE Gene and Differences in Life Expectancy in EuropeJournal Article; Research Support. J. Gerontol. A. Biol. Sci. Med. Sci. 59 (1), 16–20. doi:10.1093/gerona/59.1.b16
Hilal, S., Ikram, M. K., Saini, M., Tan, C. S., Catindig, J. A., Dong, Y. H., et al. (2013). Prevalence of Cognitive Impairment in Chinese: Epidemiology of Dementia in Singapore Study. J. Neurol. Neurosurg. Psychiatry 84 (6), 686–692. doi:10.1136/jnnp-2012-304080
Hollingworth, P., Harold, D., Sims, R., Gerrish, A., Lambert, J. C., and Carrasquillo, M. M. (2011). Common Variants at ABCA7, MS4A6A/MS4A4E, EPHA1, CD33 and CD2AP Are Associated with Alzheimer's Disease. Nat. Genet. 43 (5), 429–435. doi:10.1038/ng.803
Hu, P., Qin, Y. H., Jing, C. X., Lu, L., Hu, B., and Du, P. F. (2011). Does the Geographical Gradient of ApoE4 Allele Exist in China? A Systemic Comparison Among Multiple Chinese Populations. Mol. Biol. Rep. 38 (1), 489–494. doi:10.1007/s11033-010-0132-0
Kelleher, R. J., and Soiza, R. L. (2013). Evidence of Endothelial Dysfunction in the Development of Alzheimer's Disease: Is Alzheimer's a Vascular Disorder? Am. J. Cardiovasc. Dis. 3 (4), 197–226.
Kryscio, R. J., Abner, E. L., Lin, Y., Cooper, G. E., Fardo, D. W., Jicha, G. A., et al. (2013). Adjusting for Mortality when Identifying Risk Factors for Transitions to Mild Cognitive Impairment and Dementia. J. Alzheimers Dis. 35 (4), 823–832. doi:10.3233/JAD-122146
Lambert, J. C., Ibrahim-Verbaas, C. A., Harold, D., Naj, A. C., Sims, R., Bellenguez, C., et al. (2013). Meta-analysis of 74,046 Individuals Identifies 11 New Susceptibility Loci for Alzheimer's Disease. Nat. Genet. 45 (12), 1452–1458. doi:10.1038/ng.2802
Lawton, M. P., and Brody, E. M. (1969). Assessment of Older People: Self-Maintaining and Instrumental Activities of Daily Living. Gerontologist 9 (3), 179–186. doi:10.1093/geront/9.3_part_1.179
Li, G., Shofer, J. B., Kukull, W. A., Peskind, E. R., Tsuang, D. W., Breitner, J. C., et al. (2005). Serum Cholesterol and Risk of Alzheimer Disease: A Community-Based Cohort Study. Neurology 65 (7), 1045–1050. doi:10.1212/01.wnl.0000178989.87072.11
Li, J. Q., Tan, L., Wang, H. F., Tan, M. S., Tan, L., Xu, W., et al. (2016). Risk Factors for Predicting Progression from Mild Cognitive Impairment to Alzheimer's Disease: A Systematic Review and Meta-Analysis of Cohort Studies. J. Neurol. Neurosurg. Psychiatry 87 (5), 476–484. doi:10.1136/jnnp-2014-310095
Lim, W. S., Chong, M. S., and Sahadevan, S. (2007). Utility of the Clinical Dementia Rating in Asian Populations. Clin. Med. Res. 5 (1), 61–70. doi:10.3121/cmr.2007.693
Ma, F., Wang, H., Cao, X., Tan, C., Tan, L., and Yu, J. (2018). Meta-Analysis of the Association between Variants in ABCA7 and Alzheimer’s Disease. J. Alzheimer's Dis. 63 (4), 1261–1267. doi:10.3233/JAD-180107
Mahley, R. W., and Rall, S. C. (2000). APOLIPOPROTEIN e: Far more Than a lipid transport protein. Annu. Rev. Genom. Hum. G. 1 (1), 507–537. doi:10.1146/annurev.genom.1.1.507
Ohara, T., Hata, J., Yoshida, D., Mukai, N., Nagata, M., Iwaki, T., et al. (2017). Trends in Dementia Prevalence, Incidence, and Survival Rate in a Japanese Community. Neurology 88 (20), 1925–1932. doi:10.1212/WNL.0000000000003932
Peloso, G. M., Beiser, A. S., Destefano, A. L., and Seshadri, S. (2018b). Genetic Interaction with Plasma Lipids on Alzheimer's Disease in the framingham Heart Study. J. Alzheimers Dis. 66 (3), 1275–1282. doi:10.3233/JAD-180751
Peloso, G. M., Beiser, A. S., Destefano, A. L., and Seshadri, S. (2018a). Genetic Interaction with Plasma Lipids on Alzheimer's Disease in the framingham Heart Study. [Journal Article]. J. Alzheimers Dis. 66 (3), 1275–1282. doi:10.3233/JAD-180751
Petersen, R. C., Smith, G. E., Waring, S. C., Ivnik, R. J., Tangalos, E. G., and Kokmen, E. (1999). Mild Cognitive Impairment: Clinical Characterization and Outcome. Arch. Neurol. 56 (3), 303–308. doi:10.1001/archneur.56.3.303
Proitsi, P., Lupton, M. K., Velayudhan, L., Newhouse, S., Fogh, I., Tsolaki, M., et al. (2014). Genetic Predisposition to Increased Blood Cholesterol and Triglyceride Lipid Levels and Risk of Alzheimer Disease: A Mendelian Randomization Analysis. Plos Med. 11 (9), e1001713. doi:10.1371/journal.pmed.1001713
Rajan, K. B., Weuve, J., Barnes, L. L., Wilson, R. S., and Evans, D. A. (2019). Prevalence and Incidence of Clinically Diagnosed Alzheimer's Disease Dementia from 1994 to 2012 in a Population Study. Alzheimer's Demen. 15 (1), 1–7. doi:10.1016/j.jalz.2018.07.216
Reitz, C., Luchsinger, J., Tang, M. X., Manly, J., and Mayeux, R. (2005). Impact of Plasma Lipids and Time on Memory Performance in Healthy Elderly without Dementia. Neurology 64 (8), 1378–1383. doi:10.1212/01.WNL.0000158274.31318.3C
Reitz, C., Tang, M. X., Schupf, N., Manly, J. J., Mayeux, R., and Luchsinger, J. A. (2010). Association of Higher Levels of High-Density Lipoprotein Cholesterol in Elderly Individuals and Lower Risk of Late-Onset Alzheimer Disease. Arch. Neurol. 67 (12), 1491–1497. doi:10.1001/archneurol.2010.297
Rossignoli, A., Shang, M. M., Gladh, H., Moessinger, C., Foroughi, A. H., Talukdar, H. A., et al. (2017). Poliovirus Receptor-Related 2: A Cholesterol-Responsive Gene Affecting Atherosclerosis Development by Modulating Leukocyte Migration. Arterioscler Thromb. Vasc. Biol. 37 (3), 534–542. doi:10.1161/ATVBAHA.116.308715
Scarabino, D., Broggio, E., Gambina, G., Maida, C., Gaudio, M. R., and Corbo, R. M. (2016). Apolipoprotein E Genotypes and Plasma Levels in Mild Cognitive Impairment Conversion to Alzheimer's Disease: A Follow-Up Study. Am. J. Med. Genet. B: Neuropsychiatr. Genet. 171 (8), 1131–1138. doi:10.1002/ajmg.b.32495
Schilling, S., Tzourio, C., Soumaré, A., Kaffashian, S., Dartigues, J. F., Ancelin, M. L., et al. (2017). Differential Associations of Plasma Lipids with Incident Dementia and Dementia Subtypes in the 3C Study: A Longitudinal, Population-Based Prospective Cohort Study. Plos Med. 14 (3), e1002265. doi:10.1371/journal.pmed.1002265
Seshadri, S, Fitzpatrick, AL, Ikram, MA, DeStefano, AL, Gudnason, V, Boada, M, et al. Genome-wide analysis of genetic loci associated with Alzheimer disease. JAMA (2010) 303:1832–40. doi:10.1001/jama.2010.574
Silverman, M. G., Ference, B. A., Im, K., Wiviott, S. D., Giugliano, R. P., Grundy, S. M., et al. (2016). Association between Lowering LDL-C and Cardiovascular Risk Reduction Among Different Therapeutic Interventions: A Systematic Review and Meta-Analysis. JAMA 316 (12), 1289–1297. doi:10.1001/jama.2016.13985
Smirnov, D. A., Morley, M., Shin, E., Spielman, R. S., and Cheung, V. G. (2009). Genetic Analysis of Radiation-Induced Changes in Human Gene Expression. Nature 459 (7246), 587–591. doi:10.1038/nature07940
Solfrizzi, V., Panza, F., Colacicco, A. M., D'Introno, A., Capurso, C., Torres, F., et al. (2004). Vascular Risk Factors, Incidence of MCI, and Rates of Progression to Dementia. Neurology 63 (10), 1882–1891. doi:10.1212/01.wnl.0000144281.38555.e3
Takei, N., Miyashita, A., Tsukie, T., Arai, H., Asada, T., Imagawa, M., et al. (2009). Genetic Association Study on in and Around the APOE in Late-Onset Alzheimer Disease in Japanese. Genomics 93 (5), 441–448. doi:10.1016/j.ygeno.2009.01.003
Tsolaki, A. C., Gatzima, O., Daniilidou, M., Lazarou, E., Bamidis, P. D., Verykouki, E., et al. (2018). Prevalence of apolipoprotein e polymorphisms in alzheimer’s disease, mild cognitive impairment, and healthy elderly: A northern greece study. Neurodegener. Dis. 18 (4), 216–224. doi:10.1159/000491764
Van Cauwenberghe, C., Van Broeckhoven, C., and Sleegers, K. (2016). The Genetic Landscape of Alzheimer Disease: Clinical Implications and Perspectives. Genet. Med. 18 (5), 421–430. doi:10.1038/gim.2015.117
van der Graaf, A., Claringbould, A., Rimbert, A., Westra, H. J., Li, Y., Wijmenga, C., et al. (2020). Mendelian Randomization while Jointly Modeling Cis Genetics Identifies Causal Relationships between Gene Expression and Lipids. Nat. Commun. 11 (1), 4930. doi:10.1038/s41467-020-18716-x
Xiao, Q., Liu, Z. J., Tao, S., Sun, Y. M., Jiang, D., Li, H. L., et al. (2015). Risk Prediction for Sporadic Alzheimer's Disease Using Genetic Risk Score in the Han Chinese Population. [Comparative Study. Oncotarget 6 (35), 36955–36964. doi:10.18632/oncotarget.6271
Keywords: mild cognitive impairment, progression, Alzheimer’s disease, rs6859, low-density lipoprotein
Citation: Xiao Q, Xi J, Wang R, Zhao Q, Liang X, Wu W, Zheng L, Guo Q, Hong Z, Fu H and Ding D (2022) The Relationship Between Low-Density Lipoprotein Cholesterol and Progression of Mild Cognitive Impairment: The Influence of rs6859 in PVRL2. Front. Genet. 13:823406. doi: 10.3389/fgene.2022.823406
Received: 27 November 2021; Accepted: 21 January 2022;
Published: 21 February 2022.
Edited by:
Zhenjian Zhuo, Guangzhou Medical University, ChinaReviewed by:
Haitao Chen, Sun Yat-sen University, ChinaScott Sundseth, Global Genomic Medicine Collaborative, United States
Copyright © 2022 Xiao, Xi, Wang, Zhao, Liang, Wu, Zheng, Guo, Hong, Fu and Ding. This is an open-access article distributed under the terms of the Creative Commons Attribution License (CC BY). The use, distribution or reproduction in other forums is permitted, provided the original author(s) and the copyright owner(s) are credited and that the original publication in this journal is cited, in accordance with accepted academic practice. No use, distribution or reproduction is permitted which does not comply with these terms.
*Correspondence: Qianyi Xiao, eGlhb3FpYW55aUBmdWRhbi5lZHUuY24=; Ding Ding, ZGluZ2RpbmdAaHVhc2hhbi5vcmcuY24=