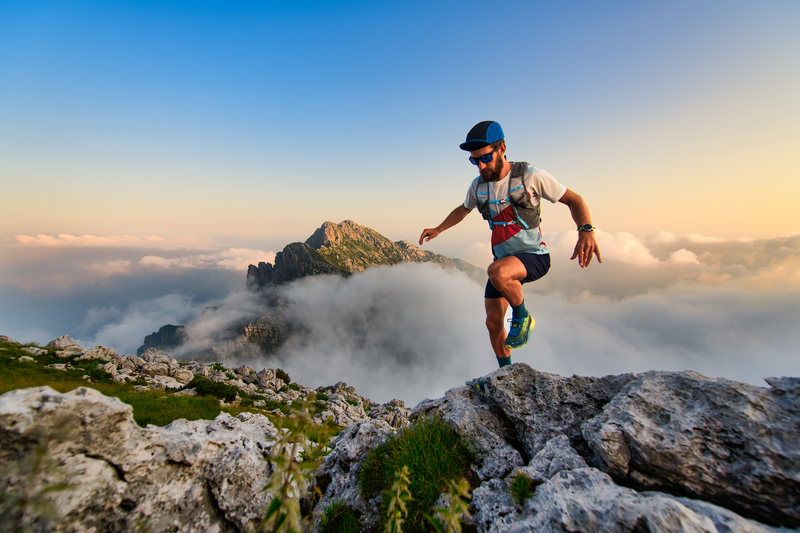
95% of researchers rate our articles as excellent or good
Learn more about the work of our research integrity team to safeguard the quality of each article we publish.
Find out more
ORIGINAL RESEARCH article
Front. Genet. , 03 March 2022
Sec. Livestock Genomics
Volume 13 - 2022 | https://doi.org/10.3389/fgene.2022.821406
Rumen development is a crucial physiological challenge for ruminants. However, the molecular mechanism regulating rumen development has not been clearly elucidated. In this study, we investigated genes involved in rumen development in 13 rumen tissues from three developmental stages (birth, youth, and adult) using RNA sequencing. We identified that 6,048 genes were differentially expressed among three developmental stages. Using weighted correlation network analysis, we found that 12 modules were significantly associated with developmental stages. Functional annotation and protein–protein interaction (PPI) network analysis revealed that CCNB1, CCNB2, IGF1, IGF2, HMGCL, BDH1, ACAT1, HMGCS2, and CREBBP involved in rumen development. Integrated transcriptome with GWAS information of carcass weight (CW), stomach weight (SW), marbling score (MS), backfat thickness (BFT), ribeye area (REA), and lean meat weight (LMW), we found that upregulated DEGs (fold change 0∼1) in birth–youth comparison were significantly enriched with GWAS signals of MS, downregulated DEGs (fold change >3) were significantly enriched with GWAS signals of SW, and fold change 0∼1 up/downregulated DEGs in birth–adult comparison were significantly enriched with GWAS signals of CW, LMW, REA, and BFT. Furthermore, we found that GWAS signals for CW, LMW, and REA were enriched in turquoise module, and GWAS signals for CW was enriched in lightgreen module. Our study provides novel insights into the molecular mechanism underlying rumen development in cattle and highlights an integrative analysis for illustrating the genetic architecture of beef complex traits.
Rumen development is an important physiological challenge for young ruminants (Lin et al., 2019). At birth, rumen is incompletely developed without high ketogenic capacity (Warner et al., 1956; Lin et al., 2020). With the rumen development and microbial colonization, calves began to transit from a milk-based diet to a grain and forage-based diet (Jami et al., 2014; Shabat et al., 2016). The development of the rumen during this transition involves three simultaneous processes (Li et al., 2019). First is the physical development of rumen including growth in rumen volume and papilla (Reynolds et al., 2004). Second, microbial communities were established and colonized (Fouts et al., 2012; Rey et al., 2014), which is highly correlated with important carcass traits, such as marbling score (MS), adjusted 12th rib fat thickness, longissimus lipid content, and carcass yield grade (Krause et al., 2020). Meanwhile, there is a functional development of fermentation capacity and enzyme activity in the rumen lumen and epimural layers (Rey et al., 2012). This whole process is not instantaneous, and the ability to ferment solid diet acquired at least 1 week after weaning (Quigley et al., 1985). However, little is known about transcriptome characteristics during these morphological changes.
Previous studies focused on the effects of nutrient, diet composition, or feeding strategy on rumen development. Encouraging calves to consume dry feedstuffs at an early age will accelerate rumen development, allowing more efficient body growth and development at maturity (Coverdale et al., 2004; Norouzian et al., 2011). Commonly rumen microorganisms proliferate and produce energy in the form of volatile fatty acids (VFAs), primarily propionate and butyrate (Reddy et al., 2017). VFAs, particularly butyrate, can stimulate papilla growth, accelerate rumen motility, and muscle growth (Tamate et al., 1962; Kristensen et al., 2007). With the development of high-throughput RNA sequencing (RNA-seq), it is allowed to investigate gene expression of certain tissue as integrity. In beef cattle, RNA-seq has been utilized to investigate the transcriptome of liver, longissimus dorsi muscle, and adipose tissues (Cesar et al., 2015; Huang et al., 2017; Kong et al., 2020). Of these, the developmental transcriptome of ruminal tissue in beef cattle was limitedly reported. Identifying genes expression during ruminal tissue development represents an important step toward understanding the biological processes of rumen growth.
Here, we performed RNA-seq to examine transcriptome profiles and identify candidate genes from rumen tissue of three developing stages. We believed that these results could help us understand the molecular mechanism of rumen development and further illuminate the relation between transcriptome of rumen development and beef complex traits.
A total of 13 Simmental half-sib individuals from three periods (birth, youth, and adult) were used in this study, including five calves at birth, five youth individuals (6 months old), and three adult cattle (18 months old). These cattle were raised under the same feeding strategies and conditions in Shayang Hanjiang cattle Co., Ltd. (Shayang County, Jingmen City, Hubei Province). After slaughtering, a 2-cm2 piece of rumen tissue was isolated immediately, rinsed with sterilized PBS buffer (pH = 6.8) and placed in a 2-ml tube. All samples were immediately frozen with liquid nitrogen for total RNA extraction.
Total RNA was extracted from rumen tissue using TRIzol reagent (Invitrogen, Life Technologies) according to the protocol of instruction. Total RNA samples were assessed for RNA integrity using the RNA Nano 6000 Assay Kit of the Bioanalyzer 2100 System (Agilent Technologices, CA, USA). RNA concentration was assayed by Qubit, and purity was assayed by Nanophotometer Spectrophotometer (Theermo Fisher Scientific, MA, USA). RNA-seq library construction was performed only for samples with RNA integrity greater than 7. The cDNA library was constructed using the Illumina TruSeqTM RNA Kit (Illumina, San Diego, CA, USA) according to the manufacturer’s instructions. RNA-seq was performed on Illumina NovaSeq 6000 platform using a pairing end strategy [read length 150 base pairs (bp)].
The clean data were mapped to the reference genome (Bos taurus ARS-UCD1.2) by the HISAT2 (v2.2.1) (Kim et al., 2015). Effective reads aligned with gene regions were statistically calculated on the basis of genomic location information specified by bovine reference genome annotations (http://ftp.ensembl.org/pub/release-103/gtf/bos_taurus/). SAMtools (v1.9) (Li et al., 2009) was used to sort the BAM alignment files that were generated from HISAT2 by name. FeatureCounts (v2.0.1) (Liao et al., 2014) and Stringtie (v2.1.1) were used to estimate read counts and normalize reads as transcripts per kilobase of exon model per million mapped reads (TPM) for each sample (Pertea et al., 2015).
To investigate differentially expressed genes (DEGs) among three periods (birth vs. youth, birth vs. adult, and youth vs. adult), using DESeq2 (v1.30.1) to normalize the gene count data and calculate differential expression (Love et al., 2014). Genes with a Benjamini–Hochberg adjusted p-value < 0.05 were designated as differentially expressed.
The DEGs from three comparisons were put together and removed redundant duplicate genes and using R package WGCNA to construct co-expression network (Langfelder and Horvath 2008). Co-expressed gene modules were detected by dynamic tree cutting method (Langfelder et al., 2008). Then, modules with highly correlated GS and MM values (p-value ≤ 0.05) and module–trait relationships with a correlation coefficient >0.5 were identified as stage-specific modules (Zhang et al., 2017).
To understand the function of genes in each stage-specific module, DAVID (https://david.ncifcrf.gov/) (Huang et al., 2007) and KOBAS (http://kobas.cbi.pku.edu.cn/kobas3/) (Xie et al., 2011) were used to GO enrichment analysis and KEGG pathway analysis, respectively. GO terms and pathways with a p-value less than 0.05 were defined as significantly enriched. Genes in each stage-specific module were calculated separately by STRING (https://string-db.org/) (Szklarczyk et al., 2015), obtained the protein–protein interaction (PPI) network, and imported into Cytoscape (v3.8.2). Cytoscape depends on the “Analyze Network” function to get the degree of a node, which means how many edges connect to it (Shannon et al., 2003).
Our resource population included 1,478 Simmental beef cattle, which were born between 2008 and 2020 from in Wulagai, Inner Mongolia. All individuals were slaughtered at an average age of 20 months; four carcass traits [carcass weight (CW), lean meat weight (LMW), backfat thickness (BFT), and ribeye are (REA)], MS, and stomach weight (SW) traits were measured. Of these, SW is the total weight of the rumen, reticulum, and abomasum. All phenotypes were adjusted for the environmental fixed effects, including farm, year and sex, pre-fattening weight, and fattening days that were regarded as covariates. The DNA for each animal was isolated from blood samples and genotyped with Illumina BovineHD770kBeadchip (Illumina, San Diego, CA, USA). Before the statistical analysis, SNPs were filtered using PLINK (v1.90) (Purcell et al., 2007). SNPs were removed under strict criteria, and the standards are as follows: minor allele frequency (<0.01), missing genotypes (>0.05), and Hardy–Weinberg equilibrium (p < 10−6). Consequently, 1,432 individuals and 673,524 SNPs were remained (Xu et al., 2020). The mixed linear model was used for GWAS analysis by the GCTA (v1.93.0) (Yang et al., 2011; Yang et al., 2014) software, and the formula is as follows:
where yij is the pre-adjusted phenotypic value of the ith individual with the jth SNP; bj is the allele substitution effect of SNP j; xij is the jth SNP genotype of individual i and xij is coded as 0, 1, and 2 for genotypes BB, Bb, and bb; gij is the polygenetic effect of the ijth individual, gij∼N (0, σa2G), with σa2 being the additive genetic variance and G is the additive genetic relationship matrix constructed using all SNPs. eij is the residual effect, eij∼N (0, σe2I), with σe2 being the residual variance and I is the identity matrix.
In previous reported, rumen microbiota may affect the MS, adjusted 12th rib fat thickness, and other traits (Reddy et al., 2017; Kim et al., 2020), and its fermentation product VFAs can promote muscle growth (Kristensen et al., 2007). To investigate whether DEGs and gene co-expression modules were enriched with GWAS signals of carcass and other traits, we applied a sum-based method for GWAS signals enrichment analyses (sumGSE, https://github.com/WentaoCai/GWAS_enrichment) (Cai et al., 2021), which used signals of all markers within a pre-defined list of DEGs and then calculated the following summary statistics for the DEGs:
where Tsum was the summary statistics for a tested gene group. mg is the number of SNPs, which is within the DEGs or 5-kb up- and downstream of DEGs, and
A total of 310.8 million raw reads from 13 rumen tissues were generated by RNA-seq. After quality control, we obtained an average of 23.1 million clean reads ranged from 19.1 to 29.0 million reads. The mapping rate was about 96.60% (ranging from 95.41% to 97.51%) after aligning clean reads to the cattle reference genome (ARS-UCD1.2) in Supplementary Table S1. These findings indicated good data quality that were suitable for subsequent analysis. We observed an average of 12,124 genes (ranging from 11,660 to 13,087) that were expressed (TPM > 0 in at least seven samples) across 13 samples. The gene expression of three periods is shown in Supplementary Figure S1, and the samples between youth and adult were highly correlated, while we found obvious differences between birth and youth/adult stage (Supplementary Figure S2). Despite differences in sample characteristics, samples from the same group were clustered together on the basis of their gene expression profiles (Figure 1A).
FIGURE 1. (A) PCA of the identified genes, the red, green and blue dots represent samples of adult, birth and youth periods. (B) Volcano plot of differential genes, volcano plot for DEGs in rumen tissue comparing birth period and youth period. (C) Volcano plot for DEGs in rumen tissue comparing birth period and adult period. (D) Volcano plot for DEGs in rumen tissue comparing youth period and adult period, red and blue dots represent up/down-regulated DEGs, respectively. The gray dots represent not DEGs.
The top 20 expressed genes in the rumen tissue at the birth, youth, and adult stages are shown in Table 1 and Supplementary Table S2. The highest expressed gene in rumen of birth stage was IRS4, GINM1, PAQR5, S100A14, MDP1, HOXC4, TAF7L, RS1, FDPS, NTS, JUP, PHB, UBE2G1, SMS, HBS1L, CGAS, TPM3, TUT4, GPR153, and GLOD4, whereas COX1, PJA1, S100A5, S100A12, ILF2, COX3, EFNB1, PCYT1B, USP11, CYTB, ND6, OPHN1, EDA2R, SPAG1, NEO1, ATP6, LCTL, COX2, RPLP1, JRKL and KRTAP11-1, COX1, DDX3X, S100A12, SLC39A1, SNORA75, COX2, COX3, ND3, ATP6, MID1IP1, ATP6AP2, PIP4K2C, RPLP1, ATP8, CXHXorf38, NPR1, NOX5, DES, and CYTB were highest expressed gene in youth and adult stage, respectively. We found that seven top expressed genes were same between youth and adult, including COX1, S100A12, COX3, CYTB, ATP6, COX2, and RPLP1, whereas the top 20 expressed genes of rumen in birth stage were totally different with the other stages, which indicated that the gene expressed pattern of rumen was distinct at birth stage.
Next, differences in gene expression of rumen tissues between different periods were investigated. In the birth vs. youth comparison, 4,905 DEGs were identified, including 2,486 upregulated genes and 2,419 downregulated genes (Figure 1B and Supplementary Table S3). A total of 3,877 DEGs were identified in birth vs. adult comparison, including 1,991 upregulated genes and 1,886 downregulated genes (Figure 1C and Supplementary Table S4). However, there were fewer DEGs identified between youth and adult, and only 521 DEGs were identified, including 314 upregulated genes and 207 downregulated genes (Figure 1D and Supplementary Table S5). The hierarchical clustering heatmap of all DEGs is shown in Supplementary Figure S3.
To better understand the function of DEGs, we used WGCNA to explore the relationship between DEGs. DEGs with a low expression level (TPM < 0.05) in more than one sample in the same group were removed, obtaining 6048 DEGs for co-expression analysis (Supplementary Table S6). A scale-free network was constructed using blockwise module function, and 6048 genes were divided into 14 modules, the number genes in each module, ranging from 116 in dark red module to 1,421 in turquoise module (Supplementary Figure S4 and Supplementary Table S7).
GS and MM of all genes in the module were calculated to investigate the period-specific modules during rumen development (Supplementary Figure S5). GS was defined as the correlation between the gene and the developing period. MM was defined as the correlation between module eigengene and the gene expression profile. A strong correlation between GS and MM (p ≤ 0.05) shows that genes highly associated with a trait are often the most important elements of the modules associated with that trait. Furthermore, we use ME to represent the gene expression level in each module. The correlations between ME and the period of differentiation were analyzed (Figure 2). Ultimately, we identified 12 period-specific modules (average module–trait relationship > 0.5 and p ≤ 0.05), among which the modules royal blue, cyan, magenta, black, and turquoise were positively correlated with birth period; the green yellow, green, yellow, lightgreen, blue, and dark red modules were positively correlated with youth period; and the brown ones were positively correlated with adult period. In contrast, the modules yellow, lightgreen, blue, and dark red were negatively correlated with birth period, and the modules royal blue and turquoise were negatively correlated with youth and adult period, repectively.
FIGURE 2. Correlation between modules and differentiation period. The color, ranging from blue through white to red, indicates negative to positive correlation.
Potential genes were obtained through PPI network and then visualized by Cytoscape (Supplementary Figure S6). The significantly enriched GO terms and the top 10 pathways of each module are shown in Figures 3 and 4 and Supplementary Figures S7 and S8. Detailed information of the GO terms and pathways is shown in Supplementary Tables S8, S9. For rumen development, the important pathways identified were butanoate metabolism, synthesis and degradation of ketone bodies, cell cycle, Wnt signaling pathway, MAPK signaling pathway, PI3K-Akt signaling pathway, and TGF-beta signaling pathway. Multiple significant GO terms are related to cell division, mitochondrial matrix, mitochondrial inner membrane, histone acetyltransferase complex, histone acetyltransferase activity, regulation of multicellular organism growth, positive regulation of phosphatidylinositol 3-kinase signaling, and positive regulation of MAPK cascade. Combining the expression level of DEGs, GO, and pathway results allows us to suggest CCNB1, CCNB2, IGF1, IGF2, HMGCL, BDH1, ACAT1, HMGCS2, and CREBBP as the promising candidate genes for rumen development. Potential genes related to rumen development and their enriched pathways and GO terms are shown in Tables 2 and 3.
FIGURE 3. Gene ontology (GO) enrichment analysis of related differentially expressed genes (DEGs). (A) GO enrichment analysis of DEGs in green module. (B) GO enrichment analysis of DEGs in cyan module. (C) GO enrichment analysis of DEGs in darkred module. (D) GO enrichment analysis of DEGs in black module. (E) GO enrichment analysis of DEGs in brown module. (F) GO enrichment analysis of DEGs in blue module.
FIGURE 4. KEGG pathway analysis of related DEGs. (A) The top 10 of pathway enrichment for green module. (B) The top 10 of pathway enrichment for cyan module. (C) The top 10 of pathway enrichment for darkred module. (D) The top 10 of pathway enrichment for black module. (E) The top 10 of pathway enrichment for brown module. (F) The top 10 of pathway enrichment for blue module.
TABLE 2. Description of potential DEGs that associated with rumen development from different module.
To investigate whether DEGs and gene co-expression modules were enriched with GWAS signals of carcass and beef quality traits, we applied enrichment analysis for all DEGs and modules across six traits (Tables 4, 5). As shown in Supplementary Figure S9A, DEGs were significantly enriched with GWAS signals of CW and LMW. In birth–youth comparison, fold change 0∼1 (slightly) upregulated DEGs were significantly enriched with GWAS signals of MS (Figure 5A), and fold change >3 (dramatically) downregulated DEGs were significantly enriched with GWAS signals of SW (Figure 5B). In birth–adult comparison, fold change 1∼2 up/downregulated DEGs were significantly enriched with GWAS signals of CW, LMW, REA, and BFT (Figures 5C,D). In youth–adult comparison, DEGs were not significantly enriched with GWAS signals. Furthermore, as shown in Supplementary Figure S9B, DEGs of turquoise module were significantly enriched with GWAS signals of CW, LMW, and REA; DEGs of lightgreen module were significantly enriched with GWAS signals of CW; and DEGs of other modules were not significantly enriched with GWAS signals (Supplementary Figures S9C,D). Of these, GOLGB1, ACVR2A, and TWIST2 belong to turquoise module and slightly upregulated DEGs, which indicated that GOLGB1 and ACVR2A might affect CW traits and TWIST2 might affect REA trait. Furthermore, IGF2 belongs to dramatically downregulated DEGs and might affect SW trait.
FIGURE 5. The GWAS enrichment for DEGs in different groups. (A) The enrichment for DEGs of four groups in birth vs youth. (B) The enrichment for DEGs of four groups in birth vs youth. (C) The enrichment for DEGs of four groups in birth vs adult. (D) The enrichment for DEGs of four groups in birth vs adult. The line means P is equal to 0.05.
In this study, we obtained a comprehensive landscape of transcriptome profiles across 13 rumen tissue samples during three different stages of growth. Importantly, we identified candidate genes and networks related to rumen development. We obtained top 20 genes expressed in the rumen tissue during three periods. Of these, COX1, COX2, COX3, CYTB, ATP6, RPLP1, and S100A12 have occurred in both youth and adult period. COX1, COX2, and COX3 are three subunits of cytochrome c oxidase that may play an important role in the production of vast majority of ATP molecules in mammalian cells (Čunátová et al., 2020). CYTB is involved in electron transport in the mitochondrial respiratory chain (Seddigh and Darabi 2018). ATP6 has transmembrane transport activity of hydrogen ions (Li et al., 2016). RPLP1 plays an important role in the elongation step of protein synthesis (Du et al., 2007). S100A12 is involved in cell cycle progression and differentiation (Fritz et al., 2010). These genes and its functions indicated that two periods are associated with energy and cell proliferation. Furthermore, the top 20 expression genes of birth period had no common genes with other periods, but S100A14 in birth period and S100A5 and S100A12 in youth or adult periods belong to the same gene family that functions in cell cycle progression and differentiation (Liu et al., 2008).
Rumen development is regulated by a various of factors. Previous studies indicated the genes of FABP7, ILK, PDGFɑ, HMGCS2, FABP3, and AKR1C1 involved in rumen development (Kato et al., 2016; Malmuthuge et al., 2019). Moreover, CREBBP, TTF2, TGFB1, and PPARɑ are capable of contributing to the development of rumen epithelium (Baldwin et al., 2012; Connor et al., 2013; Connor et al., 2014). In our study, we identified that CCNB1, CCNB2, IGF1, IGF2, HMGCL, BDH1, ACAT1, HMGCS2, and CREBBP are the potential genes involved in rumen development, some of which were reported previously. HMGCS2 plays an important role in ketogenesis in the rumen epithelium of sheep during development (Lane et al., 2002). Furthermore, HMGCS2 was identified as a downstream target of PPARα. Increased production of VFA induced by intake of solid feed during weaning might promote ketogenesis in rumen epithelial cells. In this progress, PPARα promoted papillary development by activating HMGCS2 (Connor et al., 2013). ACAT1, BDH1, HMGCL, and HMGCS2 were found involved in the pathways of synthesis and degradation of ketone bodies and butanoate metabolism, which were found as features of rumen wall development (Sun et al., 2021). Microbial fermentation of dietary carbohydrates in the rumen produces large amount of short-chain fatty acids (SCFAs) (Poulsen et al., 2012), such as butyrate, which are known to affect rumen development and stimulate rumen epithelial cells proliferation in vivo (Sakata and Tamate 1978). SCFA transport process involves many regulatory factors including insulin-like growth factor (IGF), sodium hydrogen exchangers (NHE), monocarboxylate transporters (MCTs), and epidermal growth factor (EGF), which are also involved in the regulation of rumen epithelial cell proliferation (Baldwin 1999; Yang et al., 2012; Benesch et al., 2014; Nishihara et al., 2020). Butyrate is mainly absorbed and metabolized in the rumen epithelium (Sehested et al., 1999), producing ketone body under the action of ACAT1, BDH1, HMGCS2, and HMGCL (Kostiuk et al., 2010). Of these, HMGCS2 is a rate-limiting mitochondrial enzyme in the ketogenic pathway (Lane et al., 2002) and catalyzes synthesis of 3-hydroxy-3-methylglutaryl-CoA (HMG-CoA), the central metabolite of rumen epithelial cells (Xiang et al., 2016).
Cell proliferation is an important part of rumen development and is affected by various aspects (Naeem et al., 2012). In our study, CCNB1 and CCNB2 were enriched in the cell division, cell cycle pathway. Previous study indicated that the CCNB1 expression promoted ruminal cell cycle progression in goat (Gui and Shen 2016). CCNB2 was also found playing important roles in the acceleration of cell cycle and rumen development (Cui et al., 2018; Sun et al., 2021). CREBBP functions as a transcriptional coactivator of RNA polymerase II–mediated transcription and plays an important role in cell growth (Kalkhoven 2004; Baldwin et al., 2012). Furthermore, butyrate has been shown to affect the rumen by regulating the transcription factor CREBBP (Baldwin et al., 2012). CREBBP was enriched in the cell cycle, TGF-beta signaling pathway, and Wnt signaling pathway, part of which were highly associated with cell proliferation, apoptosis, and differentiation (Huang and Huang 2005; Kabiri et al., 2018; Guo et al., 2021). Kim et al. (2013) reported that the activity of IGF-I or IGF-II can regulate epithelial cell proliferation and differentiation in some tissues. Bach et al. (1995) suggested that IGF-II stimulates the proliferation and differentiation of rat myoblasts. Besides, these pathways are highly correlated with cell proliferation, apoptosis, and differentiation (Sun et al., 2015; Wang et al., 2018). In our study, IGF-I was enriched in the GO terms of positive regulation of phosphatidylinositol 3-kinase signaling and regulation of multicellular organism growth, and IGF-II was enriched in the GO terms of positive regulation of MAPK cascade. Hence, IGF-I and IGF-II may affect the proliferation and differentiation of rumen cells.
Rumen and its microbial abundance are closely related to the growth traits of ruminants (Andersen et al., 2021). Large amounts of VFAs and microbial proteins were produced in rumen with the assistance of rumen microbes (Krause et al., 2020; Newbold and Ramos-Morales 2020; Chai et al., 2021), which are important sources of energy, fatty acids, and amino acids for ruminants (Bergman 1990; Pathak 2008; Li et al., 2013; Liu et al., 2018). Fatty acids are associated with the deposition of marbling and backfat (Smith and Johnson 2015; Ueda et al., 2019; Zhang et al., 2019). Amino acids are the building blocks of proteins that are necessary for body protein synthesis such as muscle growth or milk protein secretion (Abdoun et al., 2006; Wu 2014). Previous research reported that SORT1, ITGA6, and TMEM39B were associated with intramuscular fat deposition (Cesar et al., 2018; Hérault et al., 2018; Ueda et al., 2021). Chromosome locations of PAQR3 co-located with QTL associated with fat deposition and one missense variant likely affect intramuscular fat via the LNPEP gene (Pena et al., 2013; Derks et al., 2021). We integrated differential genes obtained from the transcriptome with GWAS data using sum-based marker-set test method, which have been shown to be more potent or at least equivalent to most commonly used marker-set test methods for polygenic traits (Sørensen et al., 2017; Cai et al., 2021). In birth vs. youth comparison, the GWAS signals of MS trait were significantly enriched in fold change 0∼1 upregulated genes, which implied that these slightly upregulated genes were related to beef quality. Interestingly, we found that GWAS signals of SW trait were significantly enriched in fold change >3 downregulated genes, indicating that these dramatically downregulated genes may be correlated with weight of stomach. Furthermore, SORT1, ITGA6, TMEM39B, PAQR3, and LNPEP belong to fold change 0∼1 upregulated genes, and IGF2 belongs to fold change >3 downregulated genes. We can make a hypothesis that SORT1, ITGA6, TMEM39B, PAQR3, and LNPEP may be related to MS trait and IGF2 related to SW, which needs to be verified in future study. In birth vs. adult comparison, GWAS signals for CW, LMW, REA, and BFT were significantly enriched in fold change 0∼1 up/downregulated genes (Figures 5C,D). This implied that these slightly upregulated genes may be associated with CW, LMW, REA, and BFT traits. Many genes were reported to be associated with CW in previous studies. Fu et al. (2020) reported that a novel 65-bp indel in the GOLGB1 gene is related to chicken growth and body weight. PLA2R1 was associated with fat deposition and body weight, and the haplotype of ACVR2A gene had a significant effect on CW (Gheyas et al., 2015; Bhattacharya et al., 2016). Moreover, BMP7 and NFIA are associated with hot CW and LMW traits in beef cattle (Wang et al., 2020). TWIST2 and ACSL1 genes may be related to REA trait (Carvalho et al., 2019; Silva-Vignato et al., 2019). Positive correlations were found between FBP1 and PCCA gene expressions with BFT (Fassah et al., 2018). In addition, PECR and ACAT1 genes may be related to BFT trait (Piórkowska et al., 2017; Silva-Vignato et al., 2019). Moreover, these above 11 genes included in fold change 0∼1 up/downregulated genes group implied that these genes may be involved in regulating economic traits in beef cattle. This need for further investigation. Besides, the differential genes were divided into modules for enrichment analysis. We found that GWAS signals for CW, LMW, and REA were enriched in turquoise module, and GWAS signals for CW were enriched in lightgreen module (Supplementary Figures S8B,C). These implied that turquoise module genes might be related to CW, LMW, and REA traits and lightgreen module genes might be related to CW trait. We also found that GOLGB1, ACVR2A, and TWIST2 belong to turquoise module, which was consistent with our above hypothesis that GOLGB1 and ACVR2A might affect CW traits and TWIST2 affect REA trait.
This study explored the genes of CCNB1, CCNB2, IGF1, IGF2, HMGCL, BDH1, ACAT1, HMGCS2, and CREBBP on rumen proliferation and development. On the basis of our results, we can make a hypothesis that SORT1, ITGA6, TMEM39B, PAQR3, and LNPEP may regulate MS trait; GOLGB1, PLA2R1, and ACVR2A may regulate CW trait; IGF2 may regulate SW trait; BMP7 and NFIA may regulate LMW trait; TWIST2 and ACSL1 may regulate REA trait; and FBP1, PCCA, PECR, and ACAT1 may regulate BFT trait.
The raw sequence data reported in this paper have been deposited in the Genome Sequence Archive (Genomics, Proteomics & Bioinformatics 2021) in National Genomics Data Center (Nucleic Acids Res 2021), China National Center for Bioinformation/Beijing Institute of Genomics, Chinese Academy of Sciences (GSA: CRA005438) that are publicly accessible at https://ngdc.cncb.ac.cn/gsa. Genotype data have been submitted to Dryad: doi:10.5061/dryad.4qc06.
The animal study was reviewed and approved by the Animal Ethics Committee of the Institute of Animal Sciences, Chinese Academy of Agricultural Sciences (No. IAS 2020-33; 4/8/2020). All animals used in the study were treated following the guidelines established by the Council of China Animal Welfare.
YZ simulated and analyzed the data and wrote the manuscript. JL and LZ designed and supervised the experiments. WC, QL, and ZW helped to conduct the study. YW, LX, XH, QZ, LX, BZ, XG, YC, and HG discussed and improved the manuscript. All authors have read and approved the final manuscript.
This study was supported by the Agricultural Science and Technology Innovation Program in the Chinese Academy of Agricultural Sciences (ASTIP-IAS03 and CAAS-ZDRW202102), China Agriculture Research System of MOF and MARA, and the National Beef Cattle Industrial Technology System (CARS-37). The project was also partly supported by the Science and Technology Project of Inner Mongolia Autonomous Region (2020GG0210 and KJXM2020002-01) for the data analysis and interpretation of the study.
The authors declare that the research was conducted in the absence of any commercial or financial relationships that could be construed as a potential conflict of interest.
All claims expressed in this article are solely those of the authors and do not necessarily represent those of their affiliated organizations or those of the publisher, the editors, and the reviewers. Any product that may be evaluated in this article, or claim that may be made by its manufacturer, is not guaranteed or endorsed by the publisher.
The Supplementary Material for this article can be found online at: https://www.frontiersin.org/articles/10.3389/fgene.2022.821406/full#supplementary-material
Abdoun, K., Stumpff, F., and Martens, H. (2006). Ammonia and Urea Transport across the Rumen Epithelium: a Review. Anim. Health Res. Rev. 7, 43–59. doi:10.1017/s1466252307001156
Andersen, T. O., Kunath, B. J., Hagen, L. H., Arntzen, M. Ø., and Pope, P. B. (2021). Rumen Metaproteomics: Closer to Linking Rumen Microbial Function to Animal Productivity Traits. Methods 186, 42–51. doi:10.1016/j.ymeth.2020.07.011
Bach, L. A., Salemi, R., and Leeding, K. S. (1995). Roles of Insulin-like Growth Factor (IGF) Receptors and IGF-Binding Proteins in IGF-II-Induced Proliferation and Differentiation of L6A1 Rat Myoblasts. Endocrinology 136, 5061–5069. doi:10.1210/endo.136.11.7588242
Baldwin, R. L., Wu, S., Li, W., Li, C., Bequette, B. J., and Li, R. W. (2012). Quantification of Transcriptome Responses of the Rumen Epithelium to Butyrate Infusion Using RNA-Seq Technology. Gene Regul. Syst. Bio 6, 67–80. doi:10.4137/GRSB.S9687
Baldwin, R. L. (1999). The Proliferative Actions of Insulin, Insulin-like Growth Factor-I, Epidermal Growth Factor, Butyrate and Propionate on Ruminal Epithelial Cells In Vitro. Small Ruminant Res. 32, 261–268. doi:10.1016/s0921-4488(98)00188-6
Benesch, F., Dengler, F., Masur, F., Pfannkuche, H., and Gäbel, G. (2014). Monocarboxylate Transporters 1 and 4: Expression and Regulation by PPARα in Ovine Ruminal Epithelial Cells. Am. J. Physiology-Regulatory, Integr. Comp. Physiol. 307, R1428–R1437. doi:10.1152/ajpregu.00408.2013
Bergman, E. N. (1990). Energy Contributions of Volatile Fatty Acids from the Gastrointestinal Tract in Various Species. Physiol. Rev. 70, 567–590. doi:10.1152/physrev.1990.70.2.567
Bhattacharya, T. K., Chatterjee, R. N., Dushyanth, K., Paswan, C., and Guru Vishnu, P. (2016). Activin Receptor 2A and Activin Receptor 2B Genes in Chicken: Effect on Carcass Traits. J. Appl. Anim. Res. 44, 480–486. doi:10.1080/09712119.2015.1091321
Cai, W., Li, C., Li, J., Song, J., and Zhang, S. (2021). Integrated Small RNA Sequencing, Transcriptome and GWAS Data Reveal microRNA Regulation in Response to Milk Protein Traits in Chinese Holstein Cattle. Front. Genet. 12. doi:10.3389/fgene.2021.726706
Carvalho, M., Baldi, F., Alexandre, P., Santana, M., and Ventura, R. (2019). Genomic Regions and Genes Associated with Carcass Quality in Nelore Cattle. Genet. Mol. Res. GMR 18, gmr18226. doi:10.4238/gmr18226
Cesar, A. S. M., Regitano, L. C. A., Koltes, J. E., Fritz-Waters, E. R., Lanna, D. P. D., Gasparin, G., et al. (2015). Putative Regulatory Factors Associated with Intramuscular Fat Content. PLoS One 10, e0128350. doi:10.1371/journal.pone.0128350
Cesar, A. S. M., Regitano, L. C. A., Reecy, J. M., Poleti, M. D., Oliveira, P. S. N., de Oliveira, G. B., et al. (2018). Identification of Putative Regulatory Regions and Transcription Factors Associated with Intramuscular Fat Content Traits. BMC Genomics 19, 499. doi:10.1186/s12864-018-4871-y
Chai, J., Lv, X., Diao, Q., Usdrowski, H., and Zhuang, Y. (2021). Solid Diet Manipulates Rumen Epithelial Microbiota and its Interactions with Host Transcriptomic in Young Ruminants. Environ. Microbiol. n/a. doi:10.1111/1462-2920.15757
Connor, E. E., Baldwin, R. L., Walker, M. P., Ellis, S. E., Li, C., Kahl, S., et al. (2014). Transcriptional Regulators Transforming Growth Factor-Β1 and Estrogen-Related Receptor-α Identified as Putative Mediators of Calf Rumen Epithelial Tissue Development and Function during Weaning. J. Dairy Sci. 97, 4193–4207. doi:10.3168/jds.2013-7471
Connor, E. E., Baldwin, R. L., Li, C.-j., Li, R. W., and Chung, H. (2013). Gene Expression in Bovine Rumen Epithelium during Weaning Identifies Molecular Regulators of Rumen Development and Growth. Funct. Integr. Genomics 13, 133–142. doi:10.1007/s10142-012-0308-x
Coverdale, J. A., Tyler, H. D., Quigley, J. D., and Brumm, J. A. (2004). Effect of Various Levels of Forage and Form of Diet on Rumen Development and Growth in Calves. J. Dairy Sci. 87, 2554–2562. doi:10.3168/jds.s0022-0302(04)73380-9
Cui, C., Li, L., and Zhen, J. (2018). Bioinformatic Analysis Reveals the Key Pathways and Genes in Early-Onset Breast Cancer. Med. Oncol. 35, 67. doi:10.1007/s12032-018-1130-7
Čunátová, K., Reguera, D. P., Houštěk, J., Mráček, T., and Pecina, P. (2020). Role of Cytochrome C Oxidase Nuclear-Encoded Subunits in Health and Disease. Physiol. Res. 69, 947–965.
Derks, M. F. L., Gross, C., Lopes, M. S., Reinders, M. J. T., Bosse, M., Gjuvsland, A. B., et al. (2021). Accelerated Discovery of Functional Genomic Variation in Pigs. Genomics 113, 2229–2239. doi:10.1016/j.ygeno.2021.05.017
Du, Y.-J., Luo, X.-Y., Hao, Y.-Z., Zhang, T., and Hou, W.-R. (2007). cDNA Cloning and Overexpression of Acidic Ribosomal Phosphoprotein P1 Gene (RPLP1) from the Giant Panda. Int. J. Biol. Sci. 3, 428–433. doi:10.7150/ijbs.3.428
Fassah, D. M., Jeong, J. Y., and Baik, M. (2018). Hepatic Transcriptional Changes in Critical Genes for Gluconeogenesis Following Castration of Bulls. Asian-australas J. Anim. Sci. 31, 537–547. doi:10.5713/ajas.17.0875
Fouts, D. E., Szpakowski, S., Purushe, J., Torralba, M., Waterman, R. C., MacNeil, M. D., et al. (2012). Next Generation Sequencing to Define Prokaryotic and Fungal Diversity in the Bovine Rumen. PloS one 7, e48289. doi:10.1371/journal.pone.0048289
Fritz, G., Botelho, H. M., Morozova-Roche, L. A., and Gomes, C. M. (2010). Natural and Amyloid Self-Assembly of S100 Proteins: Structural Basis of Functional Diversity. FEBS J. 277, 4578–4590. doi:10.1111/j.1742-4658.2010.07887.x
Fu, R., Ren, T., Li, W., Liang, J., and Mo, G. (2020). A Novel 65-bp Indel in the GOLGB1 Gene Is Associated with Chicken Growth and Carcass Traits, Animals (Basel) 10, 475. doi:10.3390/ani10030475
Gheyas, A. A., Boschiero, C., Eory, L., Ralph, H., Kuo, R., Woolliams, J. A., et al. (2015). Functional Classification of 15 Million SNPs Detected from Diverse Chicken Populations. DNA Res. 22, 205–217. doi:10.1093/dnares/dsv005
Gui, H., and Shen, Z. (2016). Concentrate Diet Modulation of Ruminal Genes Involved in Cell Proliferation and Apoptosis Is Related to Combined Effects of Short-Chain Fatty Acid and pH in Rumen of Goats. J. Dairy Sci. 99, 6627–6638. doi:10.3168/jds.2015-10446
Guo, S., Quan, S., and Zou, S. (2021). Roles of the Notch Signaling Pathway in Ovarian Functioning. Reprod. Sci. 28, 2770–2778. doi:10.1007/s43032-021-00610-6
Hérault, F., Damon, M., Cherel, P., and Le Roy, P. (2018). Combined GWAS and LDLA Approaches to Improve Genome-wide Quantitative Trait Loci Detection Affecting Carcass and Meat Quality Traits in Pig. Meat Sci. 135, 148–158. doi:10.1016/j.meatsci.2017.09.015
Huang, D. W., Sherman, B. T., Tan, Q., Kir, J., Liu, D., Bryant, D., et al. (2007). DAVID Bioinformatics Resources: Expanded Annotation Database and Novel Algorithms to Better Extract Biology from Large Gene Lists. Nucleic Acids Res. 35, W169–W175. doi:10.1093/nar/gkm415
Huang, S. S., and Huang, J. S. (2005). TGF-β Control of Cell Proliferation. J. Cel. Biochem. 96, 447–462. doi:10.1002/jcb.20558
Huang, W., Guo, Y., Du, W., Zhang, X., Li, A., and Miao, X. (2017). Global Transcriptome Analysis Identifies Differentially Expressed Genes Related to Lipid Metabolism in Wagyu and Holstein Cattle. Sci. Rep. 7, 5278. doi:10.1038/s41598-017-05702-5
Jami, E., White, B. A., and Mizrahi, I. (2014). “Potential Role of the Bovine Rumen Microbiome in Modulating Milk Composition and Feed Efficiency, PLoS ONE, 9, e85423. doi:10.1371/journal.pone.0085423
Kabiri, Z., Greicius, G., Zaribafzadeh, H., Hemmerich, A., Counter, C. M., and Virshup, D. M. (2018). Wnt Signaling Suppresses MAPK-Driven Proliferation of Intestinal Stem Cells. J. Clin. Invest. 128, 3806–3812. doi:10.1172/jci99325
Kalkhoven, E. (2004). CBP and P300: HATs for Different Occasions. Biochem. Pharmacol. 68, 1145–1155. doi:10.1016/j.bcp.2004.03.045
Kato, D., Suzuki, Y., Haga, S., So, K., Yamauchi, E., Nakano, M., et al. (2016). Utilization of Digital Differential Display to Identify Differentially Expressed Genes Related to Rumen Development. Anim. Sci. J. 87, 584–590. doi:10.1111/asj.12448
Kim, D., Shin, H., Joung, J. G., Lee, S. Y., and Kim, J. H. (2013). Intra-relation Reconstruction from Inter-relation: miRNA to Gene Expression. BMC Syst. Biol. 7 Suppl 3 (Suppl. 3), S8. doi:10.1186/1752-0509-7-S3-S8
Kim, D., Langmead, B., and Salzberg, S. L. (2015). HISAT: a Fast Spliced Aligner with Low Memory Requirements. Nat. Methods 12, 357–360. doi:10.1038/nmeth.3317
Kim, M., Park, T., Jeong, J. Y., Baek, Y., and Lee, H. J. (2020). Association between Rumen Microbiota and Marbling Score in Korean Native Beef Cattle, Animals (Basel) 10, 712. doi:10.3390/ani10040712
Kong, L., Liu, G., Deng, M., Lian, Z., Han, Y., Sun, B., et al. (2020). Growth Retardation-Responsive Analysis of mRNAs and Long Noncoding RNAs in the Liver Tissue of Leiqiong Cattle. Sci. Rep. 10, 14254. doi:10.1038/s41598-020-71206-4
Kostiuk, M. A., Keller, B. O., and Berthiaume, L. G. (2010). Palmitoylation of Ketogenic Enzyme HMGCS2 Enhances its Interaction with PPARα and Transcription at theHmgcs2PPRE. FASEB j. 24, 1914–1924. doi:10.1096/fj.09-149765
Krause, T. R., Lourenco, J. M., Welch, C. B., Rothrock, M. J., Callaway, T. R., and Pringle, T. D. (2020). The Relationship between the Rumen Microbiome and Carcass merit in Angus Steers. J. Anim. Sci. 98. doi:10.1093/jas/skaa287
Kristensen, N. B., Sehested, J., Jensen, S. K., and Vestergaard, M. (2007). Effect of Milk Allowance on Concentrate Intake, Ruminal Environment, and Ruminal Development in Milk-Fed Holstein Calves. J. Dairy Sci. 90, 4346–4355. doi:10.3168/jds.2006-885
Lane, M. A., Baldwin, R. L., and Jesse, B. W. (2002). Developmental Changes in Ketogenic Enzyme Gene Expression during Sheep Rumen Development1. J. Anim. Sci. 80, 1538–1544. doi:10.2527/2002.8061538x
Langfelder, P., and Horvath, S. (2008). WGCNA: an R Package for Weighted Correlation Network Analysis. BMC Bioinformatics 9, 559. doi:10.1186/1471-2105-9-559
Langfelder, P., Zhang, B., and Horvath, S. (2008). Defining Clusters from a Hierarchical Cluster Tree: the Dynamic Tree Cut Package for R. Bioinformatics 24, 719–720. doi:10.1093/bioinformatics/btm563
Li, C., Cai, W., Zhou, C., Yin, H., Zhang, Z., Loor, J. J., et al. (2016). RNA-seq Reveals 10 Novel Promising Candidate Genes Affecting Milk Protein Concentration in the Chinese Holstein Population, Scientific Rep., 6, 26813. doi:10.1038/srep26813
Li, H., Handsaker, B., Wysoker, A., Fennell, T., Ruan, J., Homer, N., et al. (2009). The Sequence Alignment/Map Format and SAMtools. Bioinformatics 25, 2078–2079. doi:10.1093/bioinformatics/btp352
Li, W., Edwards, A., Riehle, C., Cox, M. S., Raabis, S., Skarlupka, J. H., et al. (2019). Transcriptomics Analysis of Host Liver and Meta-Transcriptome Analysis of Rumen Epimural Microbial Community in Young Calves Treated with Artificial Dosing of Rumen Content from Adult Donor Cow. Sci. Rep. 9, 790. doi:10.1038/s41598-018-37033-4
Li, X., Chen, H., Guan, Y., Li, X., Lei, L., Liu, J., et al. (2013). Acetic Acid Activates the AMP-Activated Protein Kinase Signaling Pathway to Regulate Lipid Metabolism in Bovine Hepatocytes. PLoS One 8, e67880. doi:10.1371/journal.pone.0067880
Liao, Y., Smyth, G. K., and Shi, W. (2014). featureCounts: an Efficient General Purpose Program for Assigning Sequence Reads to Genomic Features. Bioinformatics 30, 923–930. doi:10.1093/bioinformatics/btt656
Lin, L., Xie, F., Sun, D., Liu, J., Zhu, W., and Mao, S. (2019). Ruminal Microbiome-Host Crosstalk Stimulates the Development of the Ruminal Epithelium in a Lamb Model. Microbiome 7, 83. doi:10.1186/s40168-019-0701-y
Lin, S., Fang, L., Kang, X., Liu, S., Liu, M., Connor, E. E., et al. (2020). Establishment and Transcriptomic Analyses of a Cattle Rumen Epithelial Primary Cells (REPC) Culture by Bulk and Single-Cell RNA Sequencing to Elucidate Interactions of Butyrate and Rumen Development. Heliyon 6, e04112. doi:10.1016/j.heliyon.2020.e04112
Liu, J., Li, X., Dong, G.-L., Zhang, H.-W., Chen, D.-L., Du, J.-J., et al. (2008). In Silico analysis and Verification of S100 Gene Expression in Gastric Cancer. BMC Cancer 8, 261. doi:10.1186/1471-2407-8-261
Liu, Q., Wang, C., Guo, G., Huo, W. J., Zhang, Y. L., Pei, C. X., et al. (2018). Effects of Branched-Chain Volatile Fatty Acids Supplementation on Growth Performance, Ruminal Fermentation, Nutrient Digestibility, Hepatic Lipid Content and Gene Expression of Dairy Calves. Anim. Feed Sci. Technol. 237, 27–34. doi:10.1016/j.anifeedsci.2018.01.006
Love, M. I., Huber, W., and Anders, S. (2014). Moderated Estimation of Fold Change and Dispersion for RNA-Seq Data with DESeq2. Genome Biol. 15, 550. doi:10.1186/s13059-014-0550-8
Malmuthuge, N., Liang, G., and Guan, L. L. (2019). Regulation of Rumen Development in Neonatal Ruminants through Microbial Metagenomes and Host Transcriptomes. Genome Biol. 20, 172. doi:10.1186/s13059-019-1786-0
Naeem, A., Drackley, J. K., Stamey, J., and Loor, J. J. (2012). Role of Metabolic and Cellular Proliferation Genes in Ruminal Development in Response to Enhanced Plane of Nutrition in Neonatal Holstein Calves. J. Dairy Sci. 95, 1807–1820. doi:10.3168/jds.2011-4709
Newbold, C. J., and Ramos-Morales, E. (2020). Review: Ruminal Microbiome and Microbial Metabolome: Effects of Diet and Ruminant Host. Animal 14, s78–s86. doi:10.1017/s1751731119003252
Nishihara, K., Suzuki, Y., and Roh, S. (2020). Ruminal Epithelial Insulin-like Growth Factor-Binding Proteins 2, 3, and 6 Are Associated with Epithelial Cell Proliferation. Anim. Sci. J. 91, e13422. doi:10.1111/asj.13422
Norouzian, M. A., Valizadeh, R., and Vahmani, P. (2011). Rumen Development and Growth of Balouchi Lambs Offered Alfalfa hay Pre- and post-weaning. Trop. Anim. Health Prod. 43, 1169–1174. doi:10.1007/s11250-011-9819-z
Pathak, A. (2008). Various Factors Affecting Microbial Protein Synthesis in the Rumen. Veterinary World 1 (6), 186–189.
Pena, R. N., Noguera, J. L., Casellas, J., Díaz, I., Fernández, A. I., Folch, J. M., et al. (2013). Transcriptional Analysis of Intramuscular Fatty Acid Composition in the Longissimus Thoracis Muscle of Iberian × Landrace Back-Crossed Pigs. Anim. Genet. 44, 648–660. doi:10.1111/age.12066
Pertea, M., Pertea, G. M., Antonescu, C. M., Chang, T.-C., Mendell, J. T., and Salzberg, S. L. (2015). StringTie Enables Improved Reconstruction of a Transcriptome from RNA-Seq Reads. Nat. Biotechnol. 33, 290–295. doi:10.1038/nbt.3122
Piórkowska, K., Tyra, M., Ropka-Molik, K., and Podbielska, A. (2017). Evolution of Peroxisomal Trans-2-enoyl-CoA Reductase ( PECR ) as Candidate Gene for Meat Quality. Livestock Sci. 201, 85–91. doi:10.1016/j.livsci.2017.05.004
Poulsen, M., Jensen, B. B., and Engberg, R. M. (2012). The Effect of Pectin, Corn and Wheat Starch, Inulin and pH on In Vitro Production of Methane, Short Chain Fatty Acids and on the Microbial Community Composition in Rumen Fluid. Anaerobe 18, 83–90. doi:10.1016/j.anaerobe.2011.12.009
Purcell, S., Neale, B., Todd-Brown, K., Thomas, L., Ferreira, M. A. R., Bender, D., et al. (2007). PLINK: a Tool Set for Whole-Genome Association and Population-Based Linkage Analyses. Am. J. Hum. Genet. 81, 559–575. doi:10.1086/519795
Quigley, J. D., Schwab, C. G., and Hylton, W. E. (1985). Development of Rumen Function in Calves: Nature of Protein Reaching the Abomasum. J. Dairy Sci. 68, 694–702. doi:10.3168/jds.s0022-0302(85)80875-4
Reddy, K. E., Jeong, J., Baek, Y.-C., Oh, Y. K., Kim, M., So, K. M., et al. (2017). Early Weaning of Calves after Different Dietary Regimens Affects Later Rumen Development, Growth, and Carcass Traits in Hanwoo Cattle. Asian-australas J. Anim. Sci. 30, 1425–1434. doi:10.5713/ajas.17.0315
Rey, M., Enjalbert, F., Combes, S., Cauquil, L., Bouchez, O., and Monteils, V. (2014). Establishment of Ruminal Bacterial Community in Dairy Calves from Birth to Weaning Is Sequential. J. Appl. Microbiol. 116, 245–257. doi:10.1111/jam.12405
Rey, M., Enjalbert, F., and Monteils, V. (2012). Establishment of Ruminal Enzyme Activities and Fermentation Capacity in Dairy Calves from Birth through Weaning. J. Dairy Sci. 95, 1500–1512. doi:10.3168/jds.2011-4902
Reynolds, C. K., Dürst, B., Lupoli, B., Humphries, D. J., and Beever, D. E. (2004). Visceral Tissue Mass and Rumen Volume in Dairy Cows during the Transition from Late Gestation to Early Lactation. J. Dairy Sci. 87, 961–971. doi:10.3168/jds.s0022-0302(04)73240-3
Sakata, T., and Tamate, H. (1978). Rumen Epithelial Cell Proliferation Accelerated by Rapid Increase in Intraruminal Butyrate. J. Dairy Sci. 61, 1109–1113. doi:10.3168/jds.s0022-0302(78)83694-7
Seddigh, S., and Darabi, M. (2018). Functional, Structural, and Phylogenetic Analysis of Mitochondrial Cytochrome B (Cytb) in Insects. Mitochondrial DNA A 29, 236–249. doi:10.1080/24701394.2016.1275596
Sehested, J., Diernæs, L., Møller, P. D., and Skadhauge, E. (1999). Ruminal Transport and Metabolism of Short-Chain Fatty Acids (SCFA) In Vitro: Effect of SCFA Chain Length and pH. Comp. Biochem. Physiol. A: Mol. Integr. Physiol. 123, 359–368. doi:10.1016/s1095-6433(99)00074-4
Shabat, S. K. B., Sasson, G., Doron-Faigenboim, A., Durman, T., Yaacoby, S., Berg Miller, M. E., et al. (2016). Specific Microbiome-dependent Mechanisms Underlie the Energy Harvest Efficiency of Ruminants. Isme J. 10, 2958–2972. doi:10.1038/ismej.2016.62
Shannon, P., Markiel, A., Ozier, O., Baliga, N. S., Wang, J. T., Ramage, D., et al. (2003). Cytoscape: a Software Environment for Integrated Models of Biomolecular Interaction Networks. Genome Res. 13, 2498–2504. doi:10.1101/gr.1239303
Silva-Vignato, B., Coutinho, L. L., Poleti, M. D., Cesar, A. S. M., Moncau, C. T., Regitano, L. C. A., et al. (2019). Gene Co-expression Networks Associated with Carcass Traits Reveal New Pathways for Muscle and Fat Deposition in Nelore Cattle. BMC Genomics 20, 32. doi:10.1186/s12864-018-5345-y
Smith, S. B., and Johnson, B. J. (2016). 0794 Marbling: Management of Cattle to Maximize the Deposition of Intramuscular Adipose Tissue. Journal of Animal Science 94, 382.
Sørensen, I. F., Edwards, S. M., Rohde, P. D., and Sørensen, P. (2017). Multiple Trait Covariance Association Test Identifies Gene Ontology Categories Associated with Chill Coma Recovery Time in Drosophila melanogaster. Sci. Rep. 7, 2413.
Sun, D., Yin, Y., Guo, C., Liu, L., Mao, S., Zhu, W., et al. (2021). Transcriptomic Analysis Reveals the Molecular Mechanisms of Rumen wall Morphological and Functional Development Induced by Different Solid Diet Introduction in a Lamb Model. J. Anim. Sci Biotechnol 12, 33. doi:10.1186/s40104-021-00556-4
Sun, Y., Liu, W.-Z., Liu, T., Feng, X., Yang, N., and Zhou, H.-F. (2015). Signaling Pathway of MAPK/ERK in Cell Proliferation, Differentiation, Migration, Senescence and Apoptosis. J. Receptors Signal Transduction 35, 600–604. doi:10.3109/10799893.2015.1030412
Szklarczyk, D., Franceschini, A., Wyder, S., Forslund, K., Heller, D., Huerta-Cepas, J., et al. (2015). STRING V10: Protein-Protein Interaction Networks, Integrated over the Tree of Life. Nucleic Acids Res. 43, D447–D452. doi:10.1093/nar/gku1003
Tamate, H., McGilliard, A. D., Jacobson, N. L., and Getty, R. (1962). Effect of Various Dietaries on the Anatomical Development of the Stomach in the Calf. J. Dairy Sci. 45, 408–420. doi:10.3168/jds.s0022-0302(62)89406-5
Ueda, S., Hosoda, M., Yoshino, K.-I., Yamanoue, M., and Shirai, Y. (2021). Gene Expression Analysis Provides New Insights into the Mechanism of Intramuscular Fat Formation in Japanese Black Cattle. Genes 12, 1107. doi:10.3390/genes12081107
Ueda, S., Iwamoto, E., Kato, Y., Shinohara, M., Shirai, Y., and Yamanoue, M. (2019). Comparative Metabolomics of Japanese Black Cattle Beef and Other Meats Using Gas Chromatography-Mass Spectrometry. Biosci. Biotechnol. Biochem. 83, 137–147. doi:10.1080/09168451.2018.1528139
Wang, Y., Zhang, F., Mukiibi, R., Chen, L., Vinsky, M., Plastow, G., et al. (2020). Genetic Architecture of Quantitative Traits in Beef Cattle Revealed by Genome Wide Association Studies of Imputed Whole Genome Sequence Variants: II: Carcass merit Traits. BMC Genomics 21, 38. doi:10.1186/s12864-019-6273-1
Wang, Y., Zhao, H., Shao, Y., Liu, J., Li, J., and Xing, M. (2018). Interplay between Elemental Imbalance-Related PI3K/Akt/mTOR-Regulated Apoptosis and Autophagy in Arsenic (III)-induced Jejunum Toxicity of Chicken. Environ. Sci. Pollut. Res. 25, 18662–18672. doi:10.1007/s11356-018-2059-2
Warner, R. G., Flatt, W. P., and Loosli, J. K. (1956). Ruminant Nutrition, Dietary Factors Influencing Development of Ruminant Stomach. J. Agric. Food Chem. 4, 788–792. doi:10.1021/jf60067a003
Wu, G. (2014). Dietary Requirements of Synthesizable Amino Acids by Animals: a Paradigm Shift in Protein Nutrition. J. Anim. Sci Biotechnol 5, 34. doi:10.1186/2049-1891-5-34
Xiang, R., Oddy, V. H., Archibald, A. L., Vercoe, P. E., and Dalrymple, B. P. (2016). Epithelial, Metabolic and Innate Immunity Transcriptomic Signatures Differentiating the Rumen from Other Sheep and Mammalian Gastrointestinal Tract Tissues. PeerJ 4, e1762. doi:10.7717/peerj.1762
Xie, C., Mao, X., Huang, J., Ding, Y., Wu, J., Dong, S., et al. (2011). KOBAS 2.0: a Web Server for Annotation and Identification of Enriched Pathways and Diseases. Nucleic Acids Res. 39, W316–W322. doi:10.1093/nar/gkr483
Xu, L., Gao, N., Wang, Z., Xu, L., Liu, Y., Chen, Y., et al. (2020). Incorporating Genome Annotation into Genomic Prediction for Carcass Traits in Chinese Simmental Beef Cattle. Front. Genet. 11, 481. doi:10.3389/fgene.2020.00481
Yang, J., Lee, S. H., Goddard, M. E., and Visscher, P. M. (2011). GCTA: a Tool for Genome-wide Complex Trait Analysis. Am. J. Hum. Genet. 88, 76–82. doi:10.1016/j.ajhg.2010.11.011
Yang, J., Zaitlen, N. A., Goddard, M. E., Visscher, P. M., and Price, A. L. (2014). Advantages and Pitfalls in the Application of Mixed-Model Association Methods. Nat. Genet. 46, 100–106. doi:10.1038/ng.2876
Yang, W., Shen, Z., and Martens, H. (2012). An Energy-Rich Diet Enhances Expression of Na+/H+ Exchanger Isoform 1 and 3 Messenger RNA in Rumen Epithelium of Goat1. J. Anim. Sci. 90, 307–317. doi:10.2527/jas.2011-3854
Zhang, T., Zhang, X., Han, K., Zhang, G., Wang, J., Xie, K., et al. (2017). Analysis of Long Noncoding RNA and mRNA Using RNA Sequencing during the Differentiation of Intramuscular Preadipocytes in Chicken. PLoS One 12, e0172389. doi:10.1371/journal.pone.0172389
Keywords: rumen development, carcass traits, beef cattle, transcriptome, GWAS enrichment analysis
Citation: Zhang Y, Cai W, Li Q, Wang Y, Wang Z, Zhang Q, Xu L, Xu L, Hu X, Zhu B, Gao X, Chen Y, Gao H, Li J and Zhang L (2022) Transcriptome Analysis of Bovine Rumen Tissue in Three Developmental Stages. Front. Genet. 13:821406. doi: 10.3389/fgene.2022.821406
Received: 24 November 2021; Accepted: 21 January 2022;
Published: 03 March 2022.
Edited by:
Jiuzhou Song, University of Maryland, College Park, United StatesReviewed by:
Xiaomei Sun, Yangzhou University, ChinaCopyright © 2022 Zhang, Cai, Li, Wang, Wang, Zhang, Xu, Xu, Hu, Zhu, Gao, Chen, Gao, Li and Zhang. This is an open-access article distributed under the terms of the Creative Commons Attribution License (CC BY). The use, distribution or reproduction in other forums is permitted, provided the original author(s) and the copyright owner(s) are credited and that the original publication in this journal is cited, in accordance with accepted academic practice. No use, distribution or reproduction is permitted which does not comply with these terms.
*Correspondence: Junya Li, bGlqdW55YUBjYWFzLmNu; Lupei Zhang, emhhbmdsdXBlaUBjYXNzLmNu
Disclaimer: All claims expressed in this article are solely those of the authors and do not necessarily represent those of their affiliated organizations, or those of the publisher, the editors and the reviewers. Any product that may be evaluated in this article or claim that may be made by its manufacturer is not guaranteed or endorsed by the publisher.
Research integrity at Frontiers
Learn more about the work of our research integrity team to safeguard the quality of each article we publish.